题目:从UCI下载到一个数据集,通过病人的体温、是否恶心、是否腰椎疼痛、是否尿推(连续需要排尿)、排尿是否疼痛、尿道口是否有灼烧,痒,肿的感觉。来判断该病人是否得了膀胱炎,或是得了肾盂原产肾炎。
1.分类器参数设置
-M
设置迭代更新参数Θ的最大次数为number
eg:-M 3表示用
此公式运算3次以得到分类器的关键参数Θ。默认值为-M -1,表示不断迭代直到Θ的各个值收敛。
-R
Cost函数中的一个预设参数ridge,这个算法的cost函数为:
L = -sum[i=1..n]{
sum[j=1..(k-1)](Yij * ln(Pj(Xi)))
+(1 - (sum[j=1..(k-1)]Yij))
* ln(1 - sum[j=1..(k-1)]Pj(Xi))
} + ridge * (B^2)
从weka源代码中的相关代码是这样的:
for(int offset=0; offset
for(int r=1; r
nll += m_Ridge*x[offset*dim+r]*x[offset*dim+r];
}
其中nll即为上式的L。
算法通过不断迭代最小化这个L值,这里的ridge用来调整数组B(也就是我们要求的参数Θ)的模长对cost函数影响的大小。默认值为1E-8。与之前提到的梯度下降法不同,Logistic类使用拟牛顿法来不断优化参数Θ。
分类器参数设置代码如下:
Logistic m_classifier=new Logistic();//Logistic用以建立一个逻辑回归分类器
String options[]=new String[4];//训练参数数组
options[0]="-R";//cost函数中的预设参数 影响cost函数中参数的模长的比重
options[1]="1E-5";//设为1E-5
options[2]="-M";//最大迭代次数
options[3]="10";//最多迭代计算10次
m_classifier.setOptions(options);
2.这次的程序和之前稍有不同,这次的数据格式为:
@attribute Temperature real
@attribute Nausea {yes,no}
@attribute Lumbar_pain {yes,no}
@attribute Urine_pushing {yes,no}
@attribute Micturition_pains {yes,no}
@attribute Burning_feeling {yes,no}
@attribute Inflammation_of_urinary_bladder {yes,no}
@attribute Nephritis_of_renal_pelvis_origin {yes,no}
最后两个参数都是需要进行分类的类别,所以使用过滤器每次过滤掉一个然后进行一次算法训练以及评估。然后过滤另一个类别构造分类器。过滤部分代码如下:
String[] removeOptions = new String[2];
removeOptions[0] = "-R"; // "range"
removeOptions[1] = "7"; // 7th attribute
Remove remove1 = new Remove(); // new instance of filter
remove1.setOptions(removeOptions); // set options
remove1.setInputFormat(instancesTrain);
Instances newInstancesTrain1 = Filter.useFilter(instancesTrain, remove1); // 得到新的数据
3.程序运行结果如下:
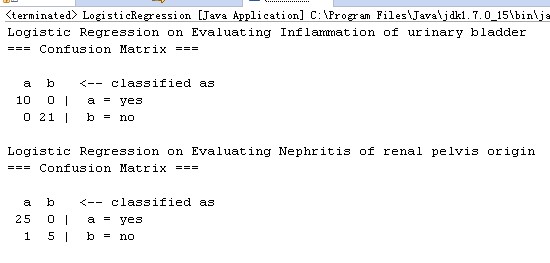
代码:
import java.io.File;
import java.io.IOException;
import weka.classifiers.Classifier;
import weka.classifiers.Evaluation;
import weka.classifiers.functions.Logistic;
import weka.classifiers.trees.J48;
import weka.core.Instances;
import weka.core.converters.ArffLoader;
import weka.filters.Filter;
import weka.filters.unsupervised.attribute.Remove;
public class LogisticRegression {
public static void main(String[] args) throws Exception {
// TODOAuto-generated method stub
ArffLoader atf = new ArffLoader(); //Reads a source that is in arff (attribute relation file format) format.
File inputFile = new File("diagnosis_part1.arff");//读入训练文件
atf.setFile(inputFile);
Instances instancesTrain = atf.getDataSet(); // 得到格式化的训练数据
//这个文件最后两个属性都是用来分类的 所以我们一个一个来做,先过滤掉一个
String[] removeOptions = newString[2];
removeOptions[0] = "-R"; // "range"
removeOptions[1] = "8"; // 8th attribute去掉第8 个
Remove remove1 = new Remove(); // new instance of filter
remove1.setOptions(removeOptions); // set options
remove1.setInputFormat(instancesTrain);
Instances newInstancesTrain1 = Filter.useFilter(instancesTrain, remove1); // 得到新的数据
newInstancesTrain1.setClassIndex(newInstancesTrain1.numAttributes()-1);//设置类别位置
inputFile = new File("diagnosis_part2.arff");//读入测试文件
atf.setFile(inputFile);
Instances instancesTest = atf.getDataSet(); // 得到格式化的测试数据
remove1.setInputFormat(instancesTest);
Instances newInstancesTest1=Filter.useFilter(instancesTest, remove1);//得到新的测试数据
newInstancesTest1.setClassIndex(newInstancesTest1.numAttributes() - 1); //设置分类属性所在行号(第一行为0号),instancesTest.numAttributes()可以取得属性总数
Logistic m_classifier=new Logistic();//Logistic用以建立一个逻辑回归分类器
String options[]=new String[4];//训练参数数组
options[0]="-R";//cost函数中的预设参数 影响cost函数中参数的模长的比重
options[1]="1E-5";//设为1E-5
options[2]="-M";//最大迭代次数
options[3]="10";//最多迭代计算10次
m_classifier.setOptions(options);
m_classifier.buildClassifier(newInstancesTrain1); //训练
Evaluation eval = newEvaluation(newInstancesTrain1); //构造评价器
eval.evaluateModel(m_classifier, newInstancesTest1);//用测试数据集来评价m_classifier
System.out.println("Logistic Regression on Evaluating Inflammation of urinary bladder");
//System.out.println(eval.toSummaryString("=== Summary ===\n",false)); //输出信息
System.out.println(eval.toMatrixString("=== Confusion Matrix ===\n"));//Confusion Matrix
//对第二个分类 Nephritis of renal pelvis origin 进行逻辑回归
Remove remove2 = new Remove(); // new instance of filter
removeOptions[0] = "-R"; // "range"
removeOptions[1] = "7"; // 7th attribute
remove2.setOptions(removeOptions);
remove2.setInputFormat(instancesTrain);
Instances newInstancesTrain2 = Filter.useFilter(instancesTrain, remove2); // 得到新的数据
newInstancesTrain2.setClassIndex(newInstancesTrain2.numAttributes()-1);//设置类别位置
//System.out.println(newInstancesTrain2.numAttributes()+"ssss");
remove2.setInputFormat(instancesTest);
Instances newInstancesTest2=Filter.useFilter(instancesTest, remove2);
newInstancesTest2.setClassIndex(newInstancesTest2.numAttributes() - 1); //设置分类属性所在行号(第一行为0号),instancesTest.numAttributes()可以取得属性总数
Logistic m_classifier2=new Logistic();//Logistic用以建立一个逻辑回归分类器
m_classifier2.setOptions(options);
m_classifier2.buildClassifier(newInstancesTrain2); //训练
Evaluation eval2 = newEvaluation(newInstancesTrain2); //构造评价器
eval2.evaluateModel(m_classifier2, newInstancesTest2);//用测试数据集来评价m_classifier
System.out.println("Logistic Regression on Evaluating Nephritis of renal pelvis origin");
//System.out.println(eval2.toSummaryString("=== Summary ===\n",false)); //输出信息
System.out.println(eval2.toMatrixString("=== Confusion Matrix ===\n"));//Confusion Matrix
}
}
数据集及源代码下载:https://onedrive.live.com/redir?resid=DF3DA11703A9CA4C!165&authkey=!AEcOfvERlJSEtdU&ithint=file,.rar