上节课我们一起学习了Hadoop自定义分区功能,这节课我们一起学习Hadoop的自定义排序,Hadoop是有一套默认的排序规则的,但是这往往不能满足我们多样化的需求,为了让排序更多样化,这就需要用到我们本节课所要学习的自定义排序功能。枯燥的说理论我相信大家都很难记住,我们一起来通过一个例子来更好的理解学习它。
我们所要用到的数据如下所示,有四列,分别代表账户、收入、支出、时间。我们想要实现的功能是按照用户的收入金额排序收入金额越大排序越靠前,如果收入金额一样则比较支出,支出越少排名越靠前。
zhangsan@163.com 6000 0 2014-02-20
lisi@163.com 2000 0 2014-02-20
lisi@163.com 0 100 2014-02-20
zhangsan@163.com 3000 0 2014-02-20
wangwu@126.com 9000 0 2014-02-20
wangwu@126.com 0 200 2014-02-20
我们通过上面的数据可以知道我们最终要得到的结果如下,最后一列的意思是收入减去支出而得到的结余多少钱。
zhangsan@163.com 9000 0 9000
wangwu@126.com 9000 200 8800
lisi@163.com 2000 100 1900
我们使用Maven来管理我们的jar包,为此我们需要创建一个Maven工程,如下图所示
点击上图的“Maven Project”之后,我们会看到如下图所示的界面,我们勾选上第一个复选框(Create a simple project),然后点击Next
点击上图的"Next"之后,我们会进入到如下图所示的界面,我们在Group Id和Artifact Id中输入组ID和工程ID,然后点击“Finish”
点击上图的"Finish"之后我们便创建了一个Maven工程,不过需要说明的是,如果你是第一次在Eclipse中创建Maven工程,它会报错,其实是找不到对应的依赖包,关于这个问题的解决方法是在root根目录下放一个m2.tar.gz本地仓库并解压它,然后在你创建的工程上右键,鼠标放到Maven上,在其子菜单中点击“Update Project”,错误就消失了。如果大家还不是很清楚如何操作,请参考:http://blog.csdn.net/u012453843/article/details/52600313这篇博客进行学习。如果已经创建过Maven工程了,第二次再创建便不会报错了。
创建好了Maven工程,接下来我们需要在pom.xml中配置依赖项,由于我们要用到的是MapReduce的功能,因此我们需要把MapReduce所需要依赖的包给导进来,还要说明的是无论用到HDFS也好还是MapReduce也好,都需要依赖hadoop-common的包,因此也需要配置进来,由于我用的Hadoop版本是2.2.0,因此包的版本也写成2.2.0,如下所示:
<modelVersion>4.0.0</modelVersion>
<groupId>com.myhadoop.mr</groupId>
<artifactId>sortdatacount</artifactId>
<version>0.0.1-SNAPSHOT</version>
<dependencies>
<dependency>
<groupId>org.apache.hadoop</groupId>
<artifactId>hadoop-common</artifactId>
<version>2.2.0</version>
</dependency>
<groupId>org.apache.hadoop</groupId>
<artifactId>hadoop-mapreduce-client-core</artifactId>
<version>2.2.0</version>
</dependency>
</dependencies>
</project>
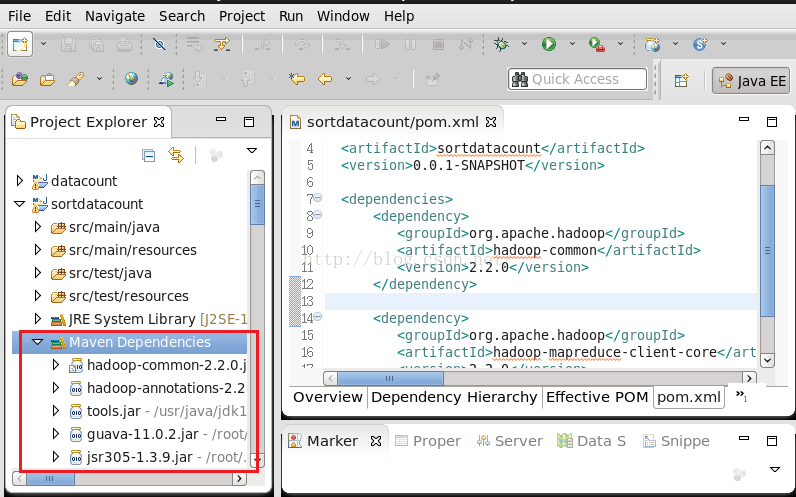
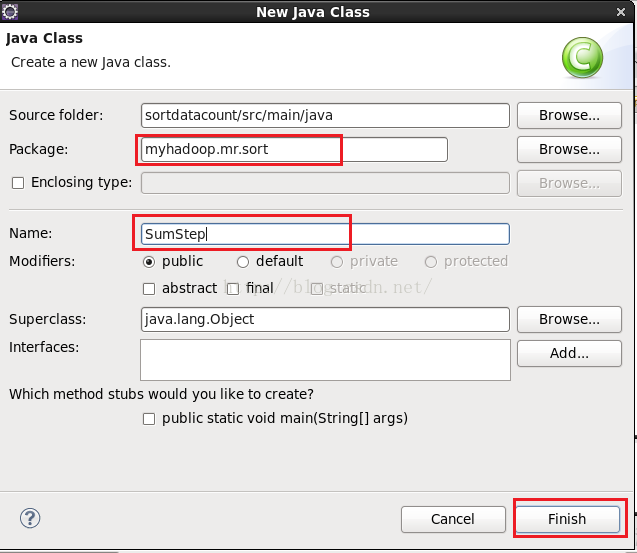
import java.io.DataOutput;
import java.io.IOException;
private String account;//帐号
private double income;//收入,这里我们不考虑多么精准,因我我们用到的数据都是整数
private double outcome;//支出
private double surplus;//结余
/**
* Text类中专门有个set方法让我们给它赋值,我们模仿Text类,也定义一个set方法来初始化InfoBean
* @param account 帐号
* @param income 收入
* @param outcome 支出
*/
public void set(String account,double income,double outcome){
this.account=account;
this.income=income;
this.outcome=outcome;
this.surplus=income-outcome;
}
/**
* 序列化Bean
*/
public void write(DataOutput out) throws IOException {
out.writeUTF(account);
out.writeDouble(income);
out.writeDouble(outcome);
out.writeDouble(surplus);
}
/**
* 反序列化Bean
*/
public void readFields(DataInput in) throws IOException {
this.account=in.readUTF();
this.income=in.readDouble();
this.outcome=in.readDouble();
this.surplus=in.readDouble();
}
/**
* 自定义比较方法,返回到int值是参数Bean与当前Bean的比较结果
* 如果传过来到Bean的收入值小于当前Bean的收入值,那么应该返回-1;
* 表示传过来的Bean应该排在当前Bean的后面。
* 如果传过来的Bean与当前Bean的收入值一样,那么就再比较支出的值,
* 如果传过来的Bean的支出值小于当前Bean的支出值的话,应该返回1,
* 表示传过来的Bean应该排在当前Bean的前面。
*/
public int compareTo(InfoBean o) {
if(this.income==o.getIncome()){
return this.getOutcome()>o.getOutcome() ? 1:-1;
}else{
return this.getIncome()>o.getIncome() ? -1:1;
}
}
/**
* 重写toString方法
*/
@Override
public String toString() {
return this.income+"\t"+this.outcome+"\t"+this.surplus;
}
return account;
}
this.account = account;
}
return income;
}
this.income = income;
}
return outcome;
}
this.outcome = outcome;
}
return surplus;
}
this.surplus = surplus;
}
}
import java.io.IOException;
import org.apache.hadoop.conf.Configuration;
import org.apache.hadoop.fs.Path;
import org.apache.hadoop.io.LongWritable;
import org.apache.hadoop.io.Text;
import org.apache.hadoop.mapreduce.Job;
import org.apache.hadoop.mapreduce.Mapper;
import org.apache.hadoop.mapreduce.Reducer;
import org.apache.hadoop.mapreduce.lib.input.FileInputFormat;
import org.apache.hadoop.mapreduce.lib.output.FileOutputFormat;
public class SumStep {
public static void main(String[] args) throws Exception {
Configuration conf=new Configuration();
Job job=Job.getInstance(conf);
job.setJarByClass(SumStep.class);
job.setMapperClass(SumMapper.class);
job.setMapOutputKeyClass(Text.class);
job.setMapOutputValueClass(InfoBean.class);
FileInputFormat.setInputPaths(job, new Path(args[0]));
job.setReducerClass(SumReducer.class);
job.setOutputKeyClass(Text.class);
job.setOutputValueClass(InfoBean.class);
FileOutputFormat.setOutputPath(job, new Path(args[1]));
job.waitForCompletion(true);
}
public static class SumMapper extends Mapper<LongWritable, Text, Text, InfoBean>{
private Text k=new Text();
private InfoBean v=new InfoBean();
/**
* 重写map方法
*/
@Override
protected void map(LongWritable key, Text value, Context context)
throws IOException, InterruptedException {
//首先获取一行的数据
String line=value.toString();
//由于我们的数据是以空格分割的,因此我们以空格进行分割
String[] fields=line.split("\t");
//获取帐号
String account=fields[0];
//获取收入的值
double income=Double.parseDouble(fields[1]);
//获取支出的值
double outcome=Double.parseDouble(fields[2]);
//给k赋值
k.set(account);
//给v赋值
v.set(account, income, outcome);
//输出
context.write(k, v);
}
}
public static class SumReducer extends Reducer<Text, InfoBean, Text, InfoBean>{
private InfoBean v=new InfoBean();
/**
* 重写Reduce方法
*/
@Override
protected void reduce(Text key, Iterable<InfoBean> values,Context context)
throws IOException, InterruptedException {
//每个账户总的收入
double sum_income=0;
//每个账户总的支出
double sum_outcome=0;
for(InfoBean bean:values){
sum_income+=bean.getIncome();
sum_outcome+=bean.getOutcome();
}
//由于key就是账户,因此这里不用传account进去也没问题
v.set("", sum_income, sum_outcome);
//输出
context.write(key, v);
}
}
}
import org.apache.hadoop.fs.Path;
import org.apache.hadoop.io.LongWritable;
import org.apache.hadoop.io.NullWritable;
import org.apache.hadoop.io.Text;
import org.apache.hadoop.mapreduce.Job;
import org.apache.hadoop.mapreduce.Mapper;
import org.apache.hadoop.mapreduce.Reducer;
import org.apache.hadoop.mapreduce.lib.input.FileInputFormat;
import org.apache.hadoop.mapreduce.lib.output.FileOutputFormat;
public static void main(String[] args) throws Exception {
Configuration conf=new Configuration();
Job job=Job.getInstance(conf);
job.setJarByClass(SortStep.class);
job.setMapperClass(SortMapper.class);
job.setMapOutputKeyClass(InfoBean.class);
job.setMapOutputValueClass(NullWritable.class);
FileInputFormat.setInputPaths(job, new Path(args[0]));
job.setReducerClass(SortReducer.class);
job.setOutputKeyClass(Text.class);
job.setOutputValueClass(InfoBean.class);
FileOutputFormat.setOutputPath(job, new Path(args[1]));
job.waitForCompletion(true);
}
public static class SortMapper extends Mapper<LongWritable, Text, InfoBean, NullWritable>{
private InfoBean k=new InfoBean();
@Override
protected void map(LongWritable key, Text value, Context context)
throws IOException, InterruptedException {
//首先获取一行的数据
String line=value.toString();
//由于我们第一个MapReduce处理完之后toString方法是以\t为分割写的,因此我们这里分割数据应该用\t来分割
String[] fields=line.split("\t");
//获取帐号
String account=fields[0];
//获取收入的值
double income=Double.parseDouble(fields[1]);
//获取支出的值
double outcome=Double.parseDouble(fields[2]);
//给v赋值
k.set(account, income, outcome);
//输出,把Bean当作key它自己就具有排序功能,因此我们只需要Bean就可以了,value的值我们可以用NullWritable来代替
context.write(k, NullWritable.get());
}
}
public static class SortReducer extends Reducer<InfoBean, NullWritable, Text, InfoBean>{
private Text k=new Text();
@Override
protected void reduce(InfoBean bean, Iterable<NullWritable> values,Context context)
throws IOException, InterruptedException {
String account=bean.getAccount();
k.set(account);
context.write(k, bean);
}
}
}
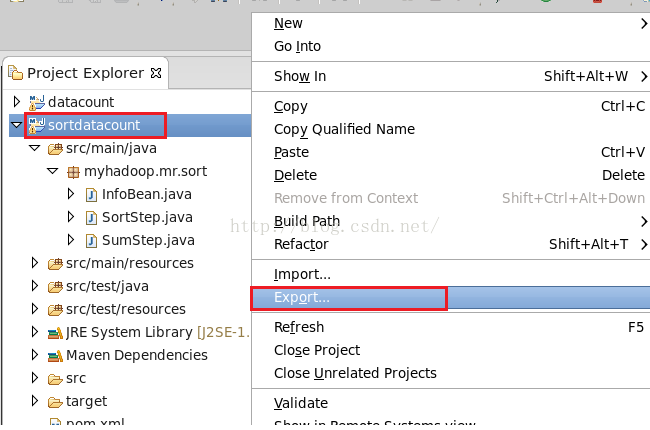
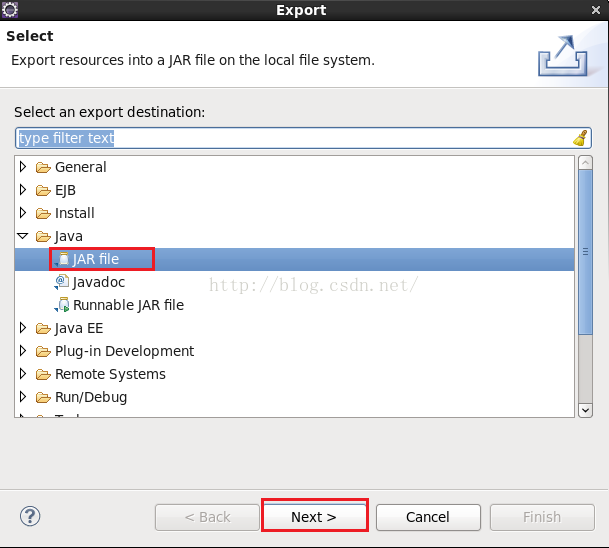
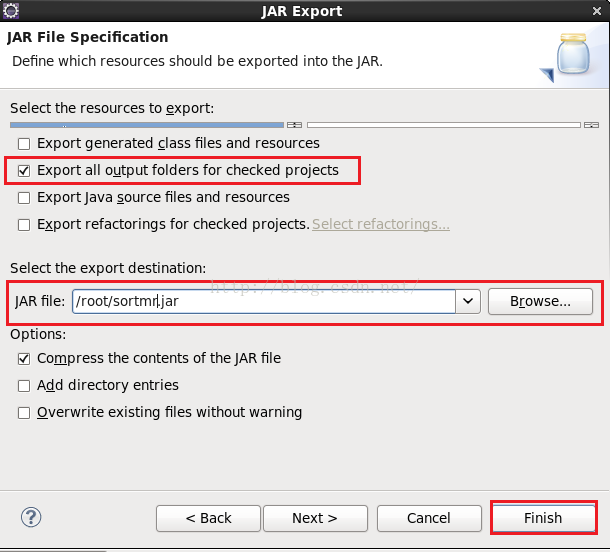

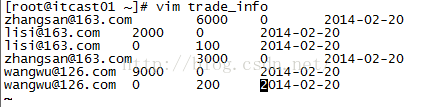
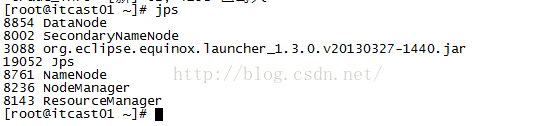
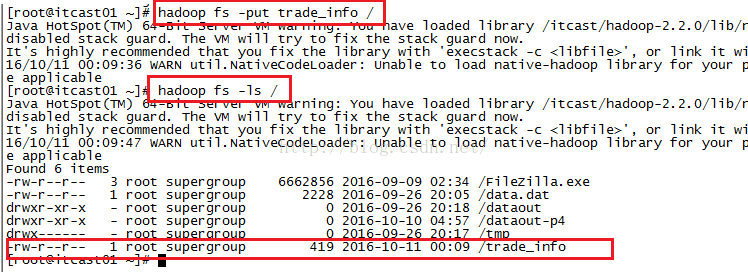
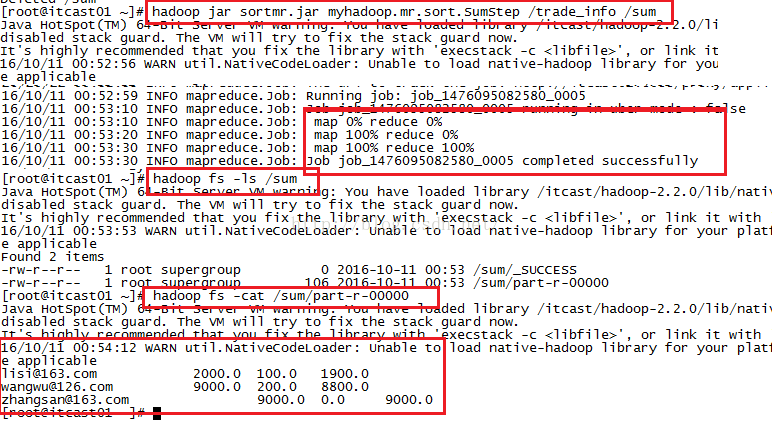
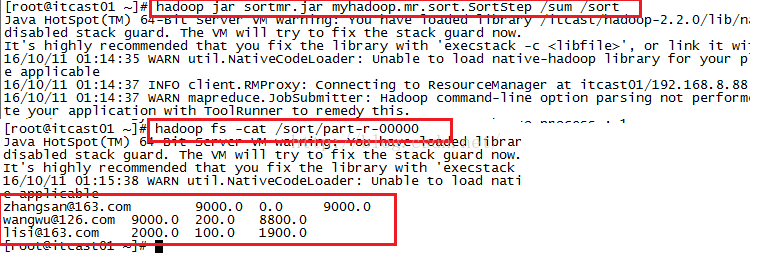