1.2 AUTOGRAD: AUTOMATIC DIFFERENTIATION
The autograd package provides automatic differentiation for all operations on Tensors.
It is a define-by-run framework, which means that your backprop is defined by how your code is run, and that every single iteration can be different.
1.2.1 Tensors
torch.Tensor
is the central class of the package.
requires_grad=True
- Track computation
x = torch.ones(2, 2, requires_grad=True)
print(x)
tensor([[1., 1.],
[1., 1.]], requires_grad=True)
.grad_fn attribute
Tensor and Function are interconnected and build up an acyclic graph, that encodes a complete history of computation.
Each tensor has a .grad_fn attribute that references a Function that has created the Tensor (except for Tensors created by the user - their grad_fn is None).
Do a tensor operation, and it has a .grad_fn
attribute.
y = x + 2
print(y)
print(y.grad_fn)
y = x + 2
print(y)
print(y.grad_fn)
tensor([[3., 3.],
[3., 3.]], grad_fn=<AddBackward0>)
<AddBackward0 object at 0x12646dc18>
requires_grad
defaults to False, in this condition, .grad_fn
is None.
It can be changed by requires_grad_
.
a = torch.randn(2, 2)
a = ((a * 3) / (a - 1))
print(a.requires_grad)
b = (a * a).sum()
print(b.grad_fn)
a.requires_grad_(True)
print(a.requires_grad)
c = (a * a).sum()
print(c.grad_fn)
False
None
True
<SumBackward0 object at 0x126479978>
1.2.2 Gradients
When you finish the computation you can call .backward() and have all the gradients computed automatically.
The gradient for this tensor will be accumulated into .grad attribute.
If Tensor is a scalar (i.e. it holds a one element data), you don’t need to specify any arguments to backward(),
else you need to specify a gradient argument that is a tensor of matching shape.
Calculate
(1) Tensor is a scalar
z = y * y * 3
out = z.mean()
print(z)
print(out)
tensor([[27., 27.],
[27., 27.]], grad_fn=<MulBackward0>)
tensor(27., grad_fn=<MeanBackward0>)
print(x.grad)
# don’t need to specify arguments
out.backward()
print(x.grad)
None
tensor([[4.5000, 4.5000],
[4.5000, 4.5000]])
(2) Tensor isn’t a scalar
x = torch.tensor([1.0,2.0,3.0],requires_grad=True)
y = (x + 2)**2
z = 4*y
z.backward(torch.tensor([1,1,1]))
print(x.grad)
tensor([24., 32., 40.])
计算推导如下:
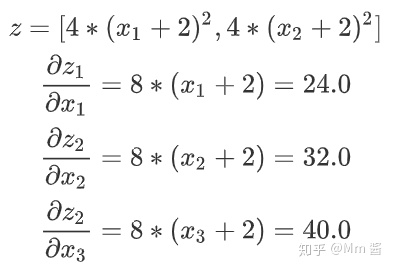
其中,添加的tensor[1,1,1],是待求x梯度的系数。
替换系数tensor后,结果如下:
x = torch.tensor([1.0,2.0,3.0],requires_grad=True)
y = (x + 2)**2
z = 4*y
z.backward(torch.tensor([1,10,100]))
print(x.grad)
tensor([ 24., 320., 4000.])
Stop autograd
(1) torch.no_grad(): stop tracking history
print(x.requires_grad)
print((x ** 2).requires_grad)
with torch.no_grad():
print((x ** 2).requires_grad)
True
True
False
(2) .detach(): get a new Tensor with same content but without gradients
print(x.requires_grad)
y = x.detach()
print(y.requires_grad)
print(x.eq(y).all())
True
False
tensor(True)