一、背景说明
目前Flink包含8个重分区算子,对应8个分区器(7个官方定义及1个自定义),均继承与父类StreamPartitioner。
RebalancePartitioner RescalePartitioner KeyGroupStreamPartitioner GlobalPartitioner ShufflePartitioner ForwardPartitioner CustomPartitionerWrapper BroadcastPartitioner
二、各分区器说明
1. 概览图
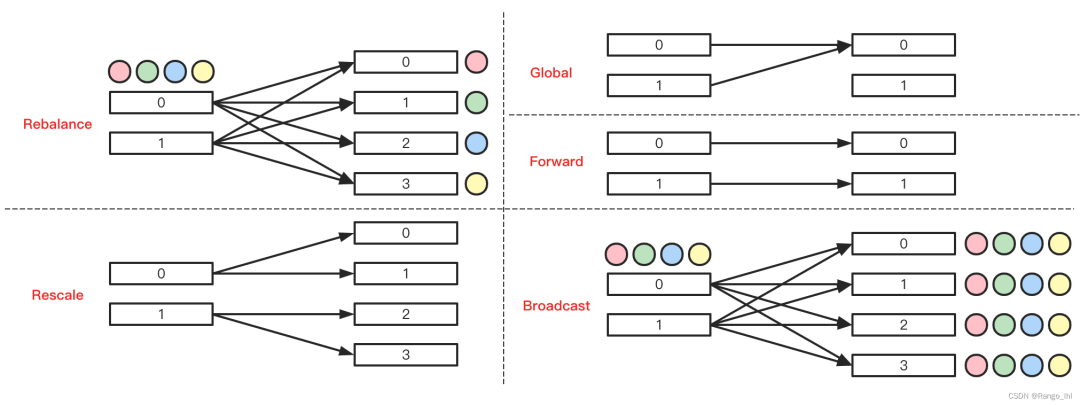
2. RebalancePartitioner
Partitioner that distributes the data equally by cycling through the output channels.
rebalance()算子是真正意义上的轮询操作,上游数据轮询下发到下游算子,注意与broadcast()算子的区别,上图颜色点代表两者数据分发的区别。
private int nextChannelToSendTo;
// 下游channel选择器,第一个数据是随机选择下游其中一个channel
@Override
public void setup(int numberOfChannels) {
super.setup(numberOfChannels);
nextChannelToSendTo = ThreadLocalRandom.current().nextInt(numberOfChannels);
}
// 后续+1取模的方式开始轮询下发
@Override
public int selectChannel(SerializationDelegate<StreamRecord<T>> record) {
nextChannelToSendTo = (nextChannelToSendTo + 1) % numberOfChannels;
return nextChannelToSendTo;
}
// 分发模式为 ALL_TO_ALL
@Override
public boolean isPointwise() { return false; }
FLink 将任务的执行计划分为 StreamGraph–>JobGraph–>ExecutionGraph,其中的StreamingJobGraphGenerator类用以实现将StreamGraph转化为JobGraph,在该类中会调用分区器的isPointwise()方法实现分发模式的选择 :POINTWISE / ALL_TO_ALL。
JobEdge jobEdge;
if (partitioner.isPointwise()) {
jobEdge =
downStreamVertex.connectNewDataSetAsInput(
headVertex, DistributionPattern.POINTWISE, resultPartitionType);
} else {
jobEdge =
downStreamVertex.connectNewDataSetAsInput(
headVertex, DistributionPattern.ALL_TO_ALL, resultPartitionType);
}
3. RescalePartitioner
The subset of downstream operations to which the upstream operation sends elements depends on the degree of parallelism of both the upstream and downstream operation. For example, if the upstream operation has parallelism 2 and the downstream operation has parallelism 4, then one upstream operation would distribute elements to two downstream operations while the other upstream operation would distribute to the other two downstream operations. If, on the other hand, the downstream operation has parallelism 2 while the upstream operation has parallelism 4 then two upstream operations will distribute to one downstream operation while the other two upstream operations will distribute to the other downstream operations. In cases where the different parallelisms are not multiples of each other one or several downstream operations will have a differing number of inputs from upstream operations.
根据源码里面的注释可知道,rescale的上下游交互取决于他们的并行度,上游为2下游为4,则一个上游对应两个下游,上游为4下游为2,则两个上游对应一个下游。如若是不同倍数的并行度,则下游会有不同数量的输入。
区别于rebalance有两点,轮询从下游第一个分区开始以及是点对点分发模式。
rescale可以增加数据本地处理,减少了网络io性能更高,但数据均衡性不如rebalance。
private int nextChannelToSendTo = -1;
// 下游channel选择器,从0开始轮询
@Override
public int selectChannel(SerializationDelegate<StreamRecord<T>> record) {
if (++nextChannelToSendTo >= numberOfChannels) {
nextChannelToSendTo = 0;
}
return nextChannelToSendTo;
}
// 分发模式 POINTWISE 点到点,一个下游只会有一个输入
@Override
public boolean isPointwise() { return true; }
4. GlobalPartitioner
Partitioner that sends all elements to the downstream operator with subtask ID=0.
如源码注释所写,所有上游数据下发到下游第一个分区。
// 下游channel选择器,均返回0
@Override
public int selectChannel(SerializationDelegate<StreamRecord<T>> record) { return 0;}
// 分发模式为 ALL_TO_ALL
@Override
public boolean isPointwise() { return false;}
5. ForwardPartitioner
Partitioner that forwards elements only to the locally running downstream operation.
仅将元素转发到本地运行的下游操作的分区器。
// 下游channel选择器,均返回0
@Override
public int selectChannel(SerializationDelegate<StreamRecord<T>> record) { return 0;}
// 分发模式 POINTWISE 点到点,一个下游只会有一个输入
@Override
public boolean isPointwise() { return true;}
与global一样的channel选择方法,区别在于isPointwise()方法为点到点。因此实现了下游仅有一个输入,通过概览图可以清晰看到两者区别。
6. BroadcastPartitioner
Partitioner that selects all the output channels.
上游数据会分发给下游所有分区,故源码里面也提示了不支持select channel。
/**
* Note: Broadcast mode could be handled directly for all the output channels in record writer,
* so it is no need to select channels via this method.
*/
@Override
public int selectChannel(SerializationDelegate<StreamRecord<T>> record) {
throw new UnsupportedOperationException(
"Broadcast partitioner does not support select channels.");
}
7. KeyGroupStreamPartitioner
Partitioner selects the target channel based on the key group index.
总结下来就是,按照分区键根据hashCode()一次哈希,再murmurHash(keyHash)二次哈希,按照最大并行度(默认128)取模生成keyGroupId,最后根据keyGroupId * parallelism / maxParallelism 得出下游分区index,作为数据分发的依据。
// 核心逻辑,其中最大并行度由系统定义,DEFAULT_LOWER_BOUND_MAX_PARALLELISM = 1 << 7 为128
public KeyedStream(
DataStream<T> dataStream,
KeySelector<T, KEY> keySelector,
TypeInformation<KEY> keyType) {
this(
dataStream,
new PartitionTransformation<>(
dataStream.getTransformation(),
new KeyGroupStreamPartitioner<>(
keySelector,
StreamGraphGenerator.DEFAULT_LOWER_BOUND_MAX_PARALLELISM)),
keySelector,
keyType);
}
KeyGroupStreamPartitioner
// key为分组键,maxParallelism由系统定义默认128,numberOfChannels为用户定义并行度
@Override
public int selectChannel(SerializationDelegate<StreamRecord<T>> record) {
K key;
try {
key = keySelector.getKey(record.getInstance().getValue());
} catch (Exception e) {
throw new RuntimeException(
"Could not extract key from " + record.getInstance().getValue(), e);
}
return KeyGroupRangeAssignment.assignKeyToParallelOperator(
key, maxParallelism, numberOfChannels);
}
KeyGroupRangeAssignment
public static int assignKeyToParallelOperator(Object key, int maxParallelism, int parallelism) {
Preconditions.checkNotNull(key, "Assigned key must not be null!");
return computeOperatorIndexForKeyGroup(
maxParallelism, parallelism, assignToKeyGroup(key, maxParallelism));
}
// 第一次hash
public static int assignToKeyGroup(Object key, int maxParallelism) {
Preconditions.checkNotNull(key, "Assigned key must not be null!");
return computeKeyGroupForKeyHash(key.hashCode(), maxParallelism);
}
// 第二次hash(murmurhash)
public static int computeKeyGroupForKeyHash(int keyHash, int maxParallelism) {
return MathUtils.murmurHash(keyHash) % maxParallelism;
}
// 根据公式获取目标下游分区index
public static int computeOperatorIndexForKeyGroup(
int maxParallelism, int parallelism, int keyGroupId) {
return keyGroupId * parallelism / maxParallelism;
}
8. ShufflePartitioner
Partitioner that distributes the data equally by selecting one output channel randomly.
shuffle()算子按Random()方法随机选择下游分区。
// 随机分发
private Random random = new Random();
@Override
public int selectChannel(SerializationDelegate<StreamRecord<T>> record) { return random.nextInt(numberOfChannels);}
@Override
public boolean isPointwise() { return false;}
9. CustomPartitionerWrapper
Partitions a DataStream on the key returned by the selector, using a custom partitioner. This method takes the key selector to get the key to partition on, and a partitioner that accepts the key type.
partitionCustom()方法顾名思义就是就是自定义分区器,其中主要是重写里面两个方法Partitioner(定义分区行为)及KeySelector(定义key)
public <K> DataStream<T> partitionCustom(
Partitioner<K> partitioner, KeySelector<T, K> keySelector) {
return setConnectionType(
new CustomPartitionerWrapper<>(clean(partitioner), clean(keySelector)));
}
如果这个文章对你有帮助,不要忘记 「在看」 「点赞」 「收藏」 三连啊喂!
2022年全网首发|大数据专家级技能模型与学习指南(胜天半子篇)
Flink CDC我吃定了耶稣也留不住他!| Flink CDC线上问题小盘点