If you are like me, and you have devoted a good chunk of your professional career to the world of data analytics in the enterprise, I guess the following story will sound very familiar to you:
如果您像我一样,并且已经将自己的职业生涯的很大一部分投入到企业中的数据分析领域,那么我想以下故事对您来说将是非常熟悉的:
“You are tasked with a new mandate: — coming from the top management of the company — to transform the organization towards [insert most hyped buzzword at that time] lets say data-centric or data-driven. After a saga of countless meetings and long committees, great news! It is finally decided to invest a couple of million or so in software, hardware and consulting/implementation services.
“您肩负着一项新的任务:—来自公司的高层管理人员–要使组织转变为[当时插入最被大肆宣传的流行语],以数据为中心或以数据为驱动力。 经过无数次会议和冗长的委员会的传奇,好消息! 最终决定在软件,硬件和咨询/实施服务上投资几百万美元左右。
Following a few months of hard work, your team and yourself manage to implement a shiny new Data Warehouse. The quality of its data is acceptable, at best, but hey!, the reports and dashboards that your IT department has created look pretty good.
经过几个月的努力,您的团队和您自己都设法实现了一个崭新的数据仓库。 数据的质量充其量是可以接受的,但是,嘿,您的IT部门创建的报告和仪表板看起来还不错。
The BI sugar rush lasts for a couple weeks but then … you discover that something is happening: business processes remains the same! The groups of users that have to make decisions — (hopefully now) based on the insights provided by your reports — neither know nor want to access these new tools.
BI交易高峰持续了几个星期,但随后……您发现正在发生某些事情:业务流程保持不变! 必须(根据现在的情况而定)基于报表提供的见解做出决定的用户组既不知道也不希望访问这些新工具。
Your truly human nature shows up and undoubtedly you direct the blame to this collective of business users — they don’t want to change. It scares them — you say. They are not as smart as we are in technology — you think to yourself “
您真正的人性显现出来,毫无疑问,您应该将责任推给这群企业用户-他们不想改变。 您说,这使他们感到恐惧。 他们不像我们在技术上那么聪明-您认为自己“
I think that the analysis of why data-driven transformation projects fail cannot be taken lightly, there are many factors and specificities that make each situation unique.But surely one of the most common problems is failing to take into account the current capabilities and training — data analytics alphabetisation/literacy maturity — of the so-called last mile of business.
我认为对数据驱动的转换项目失败的原因不能轻易分析,有许多因素和特殊性使每种情况都与众不同。但是,最常见的问题之一肯定是没有考虑到当前的能力和培训—数据分析字母/扫盲成熟度-所谓的最后一英里业务。
>最后一英里-也就是发生事情的地方 (> the last mile — a.k.a where stuff happens)
Parcel delivery drivers, front-line employees in a fashion retail store, financial advisors in a bank branch or a real-estate salesperson are examples of employees located in this last mile. And is in this last mile is where things happen, in here, the customer commits to buy a new pair of jeans or signs a 30 year long mortgage.
包裹运送司机,时装零售店的一线员工,银行分行的财务顾问或房地产销售人员都是最后一英里的员工。 而在这最后一英里就是事情发生,在这里,客户提交新买的牛仔裤或体征有30年之久的抵押贷款。
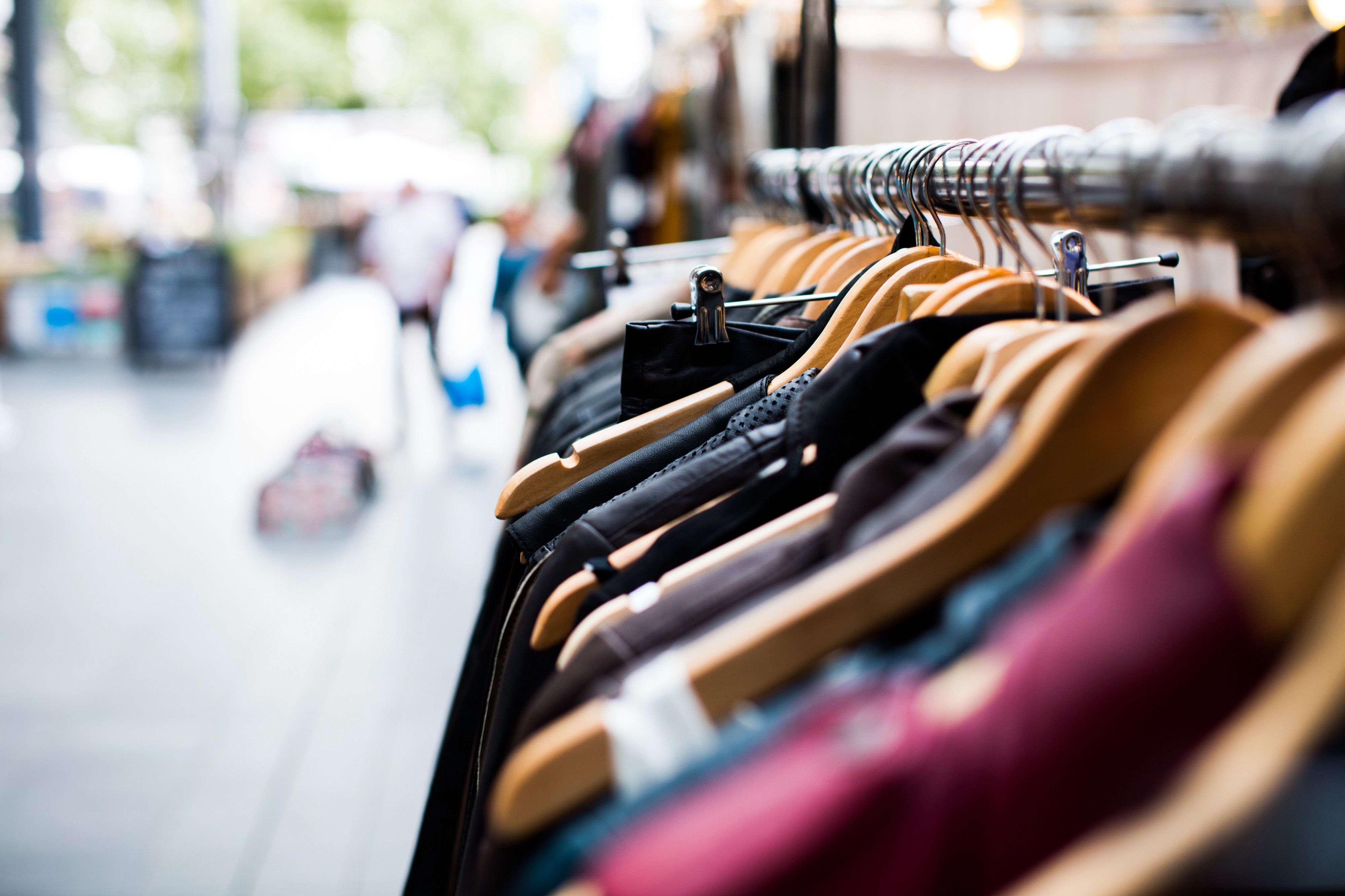
Generally speaking, the decision-making power (spectrum) of a front-line salesperson is, let’s face it, very limited (e.g. cannot decide on a 30% price cut of those jeans unilaterally) , but, how many micro-decisions (e.g. proactively showing up a t-shirt that matches perfectly your style and the new jeans) are made per day in the last mile? What if the aggregated added value of these decisions is greater than the value of a strategic decision made at headquarters?
通常来说,一线销售员的决策权(频谱)非常有限(例如,无法单方面决定将这些牛仔裤的价格下调30%),但是,有多少个微决策(例如,主动展示出与您的风格完全匹配的T恤,而新牛仔裤每天都在最后一英里内制作? 如果这些决策的总增加值大于总部做出的战略决策的价值,该怎么办?
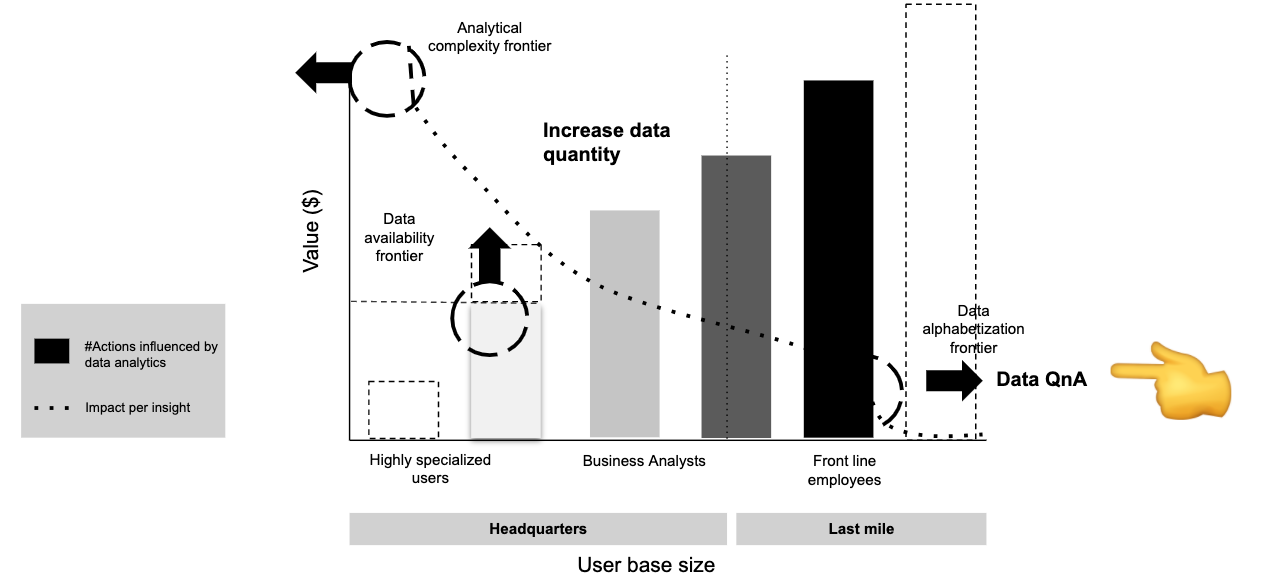
From my point of view, depending on the type of persona, there might be different approaches to increase the returned value in terms of data analytics: for highly experienced users (e.g. data scientists), perhaps the best way is to increase the analytical complexity, introducing for example more complex models, such as Machine Learning. For the intermediate user (e.g. business analysts) , perhaps the main focus of improvement, would be to increase the number of data sources available to them, maintaining of course the data quality standards, and for the user we are focusing on today (e.g. front line), without a doubt the main barrier to tear down it is analytical literacy.
在我看来,根据角色类型的不同,可能存在不同的方法来提高数据分析的返回值:对于经验丰富的用户(例如数据科学家),最好的方法可能是增加分析的复杂性,引入更复杂的模型,例如机器学习。 对于中级用户(例如业务分析师)来说,改进的主要重点可能是增加可供他们使用的数据源的数量,当然还要保持数据质量标准,而对于我们今天关注的用户(例如前端毫无疑问,拆除它的主要障碍是分析素养。
We have to make things easy for the busy residents in the last mile. Why do you require a sales assistant to understand your brand new BI self service platform? As beautiful as the UI is, concepts such as Facts, Dimensions or aggregation functions sound like differential equations for the last mile.
我们必须让忙碌的居民在最后一英里处变得轻松。 为什么需要销售助理来了解全新的BI自助服务平台? 与用户界面一样漂亮,事实,维度或聚合函数之类的概念听起来像是最后一英里的微分方程。
So, we want to influence decision-making in the last mile, but the gap in analytical literacy is insurmountable. What can we do? If not even a drag and drop interface can do the job. What technology can we use?
因此,我们希望影响最后一英里的决策,但是分析素养的差距是无法克服的。 我们能做什么? 如果不是这样,甚至拖放界面也可以完成这项工作。 我们可以使用什么技术?
>输入BigQuery Data QnA (> enter BigQuery Data QnA)
SQL, is without a doubt the lingua-franca to accessing data, all these interfaces that we have talked about, end in one way or another, transferring the user’s designs to an SQL query. On the other hand, it is quite obvious that only a very small percentage of employees in the last mile are going to be able to generate SQL statements. Isn’t there then another language that the majority of this group knows?
毫无疑问,SQL是访问数据的通用语言,我们已经讨论过的所有这些接口都以一种或另一种方式结束,从而将用户的设计转移到SQL查询中。 另一方面,很明显,最后一英里中只有很小一部分的员工将能够生成SQL语句。 那不是大多数人都知道的另一种语言吗?
I assure you that ALL the employees in the last mile are excellent in one language and no … I’m not talking about python or SQL, I’m talking about natural language: English!
我向您保证,最后一英里的所有员工都精通一种语言,而且没有……我不是在谈论python或SQL,而是在谈论自然语言:英语!
So, you are telling me that there in an English to SQL translator then? That’s right, and it’s called BigQuery Data QnA.
那么,您告诉我,那里有英语到SQL的翻译器吗? 没错,它叫做BigQuery Data QnA。
First, BigQuery is an enterprise data warehouse that solves the problem of storing and querying massive datasets by enabling super-fast SQL queries using the processing power of Google’s infrastructure. Simply move your data into BigQuery and let us handle the hard work.
首先,BigQuery是一个企业数据仓库,它通过利用Google基础架构的处理能力实现超快速SQL查询,解决了存储和查询海量数据集的问题。 只需将您的数据移至BigQuery中,让我们处理艰苦的工作即可。
Secondly, Data QnA is a natural language interface for BigQuery data analysis (on private alpha at the time of writing this article). Data QnA enables your last mile users to get answers to their analytical queries through questions in natural language, without relying on IT/BI . From now on, last-mile employees have at their disposal all the analytical potential that it has taken the organization so much time and effort to build.
其次,Data QnA是用于BigQuery数据分析的自然语言界面(在撰写本文时为私有alpha)。 数据QnA使您的最后一英里用户能够通过自然语言的问题获得对他们的分析查询的答案,而无需依赖IT / BI。 从现在开始,最后一英里的员工可以利用所有的分析潜力,这花费了组织大量的时间和精力。
Data QnA is based on the Google Research Analyza research project. Analyza uses semantic parsing for analyzing and exploring data using conversation, i.e., doing entity and intent recognition, then mapping to the underlying business datasets.
数据QnA基于Google Research Analyza研究项目。 Analyza使用语义解析来使用对话来分析和探索数据,即进行实体和意图识别,然后映射到基础业务数据集。
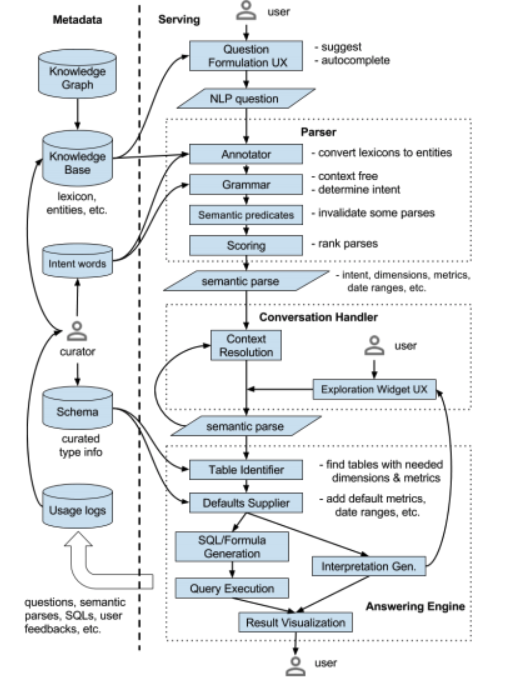
>如何运作?(> how does it work?)
We are going to use data from the BigQuery Public Dataset program, to see an example. Specifically, the dataset of the birth data in the United States.
我们将使用BigQuery Public Dataset程序中的数据,以查看示例。 具体来说,是美国出生数据的数据集。
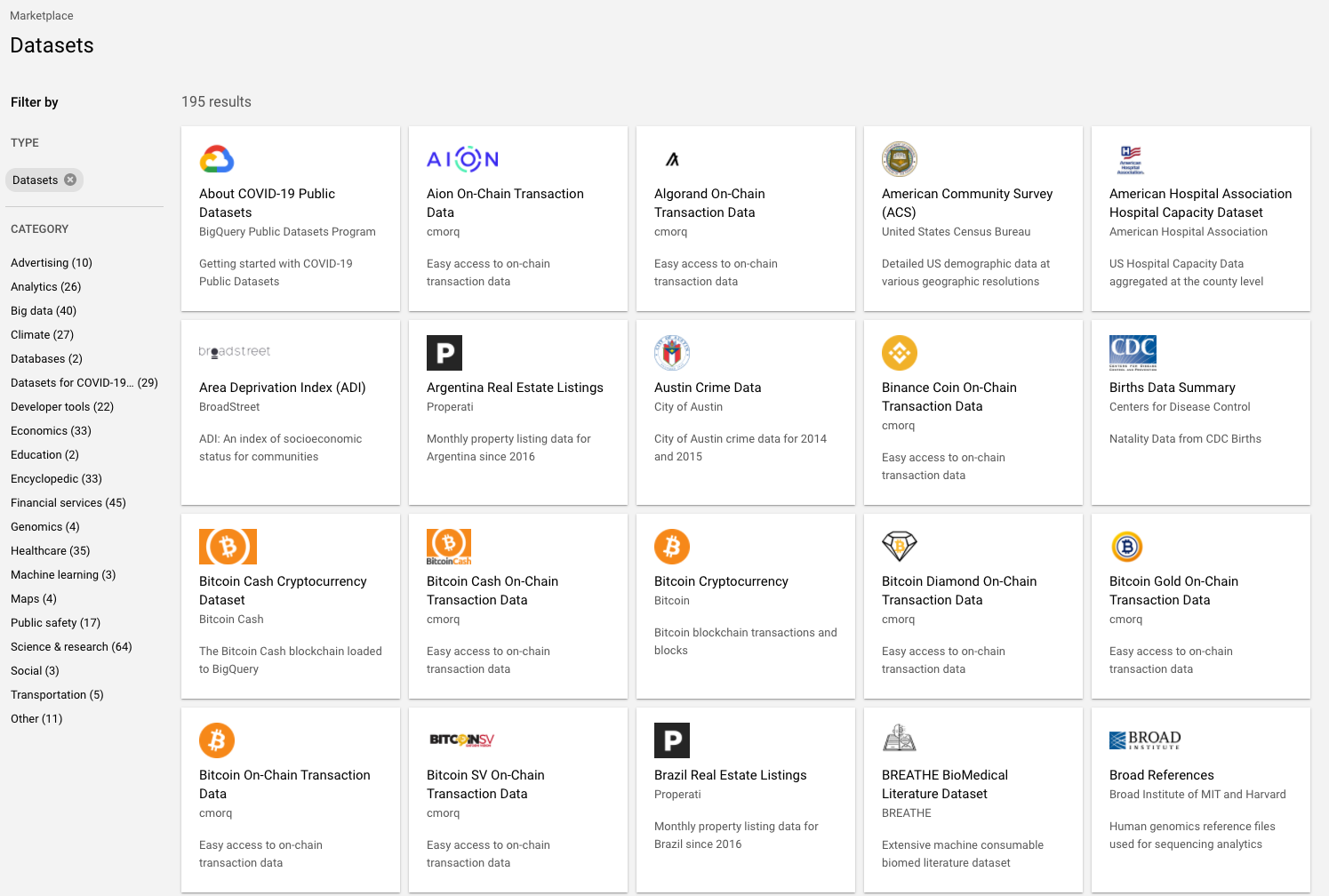
This is the schema of the table that we will analyze
这是我们将分析的表的架构
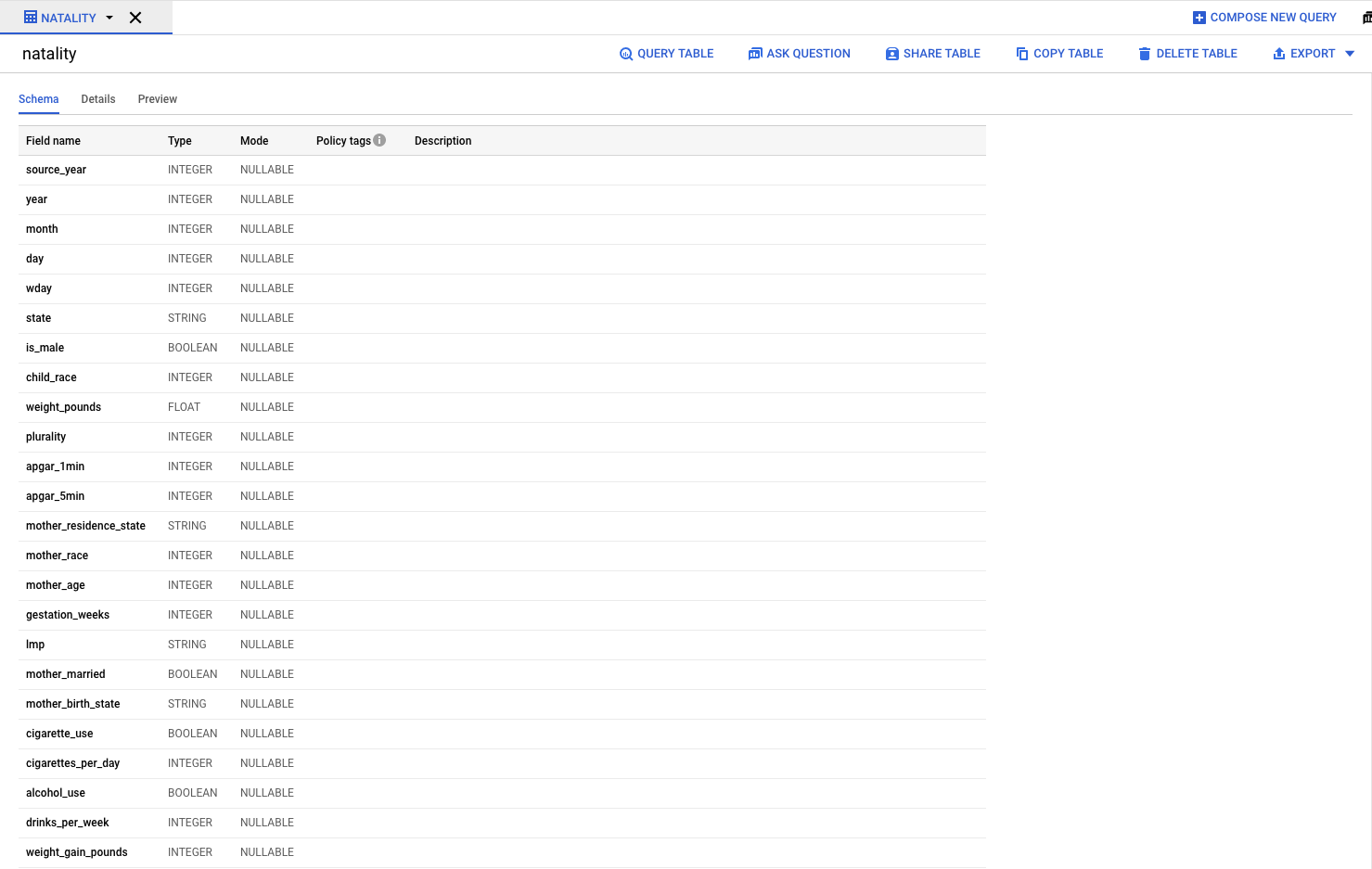
To activate Data QnA we simply have to click “ASK QUESTION” button next to “QUERY TABLE”.Next, we must perform a small configuration that basically consists of:
要激活Data QnA,我们只需单击“ QUERY TABLE”旁边的“ ASK QUESTION”按钮。接下来,我们必须执行一个小的配置,该配置主要包括:
- Mark each column as dimension (aggregation criteria) or metric (values to aggregate) 将每列标记为维度(汇总条件)或指标(要汇总的值)
- Add synonyms of column, so the parser integrates differents entities together 添加列的同义词,以便解析器将不同实体集成在一起
- Mark columns containing dates标记包含日期的列
- Specify the default value to return — in case of not specifying anything, for example row count指定要返回的默认值-如果未指定任何内容,例如行数
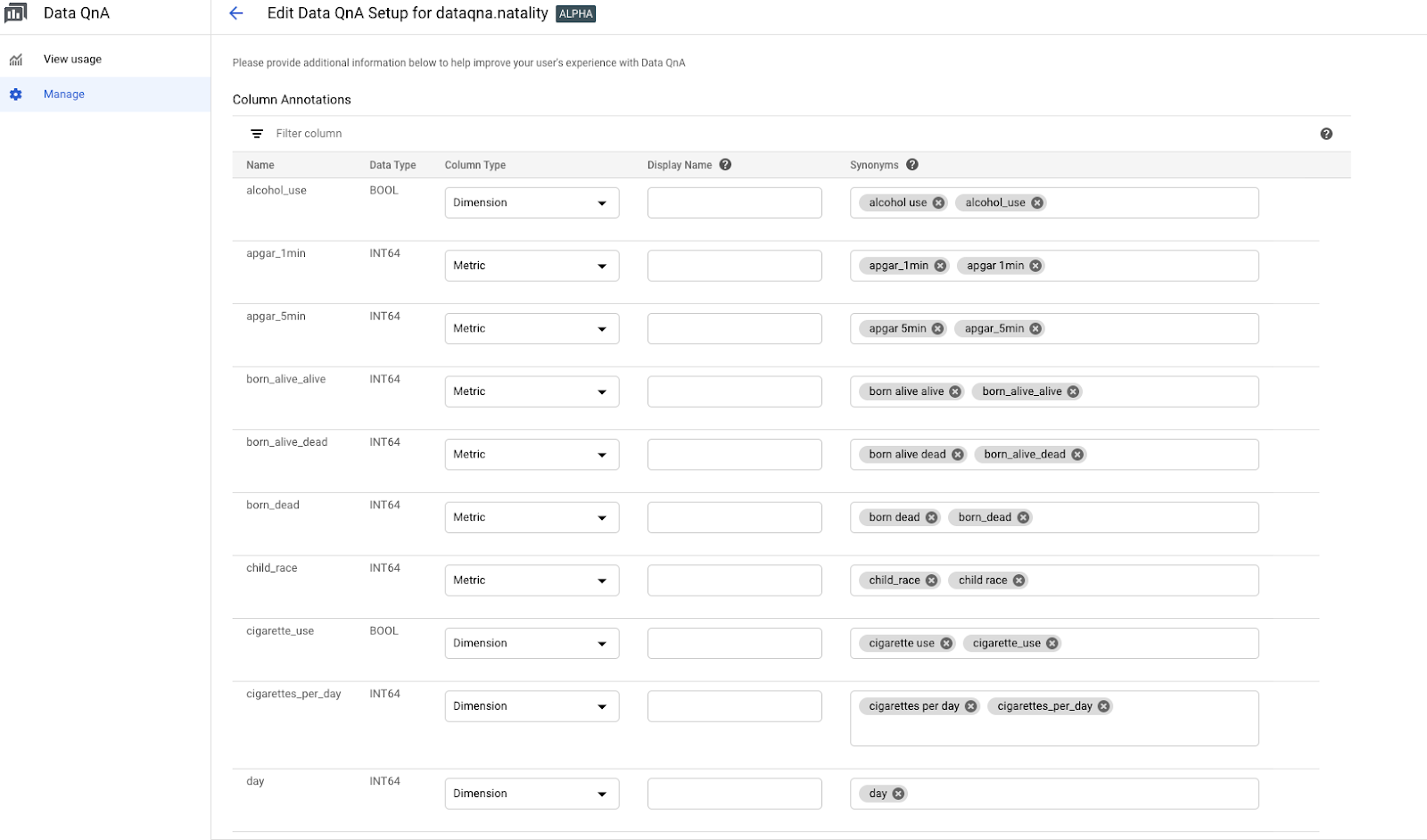
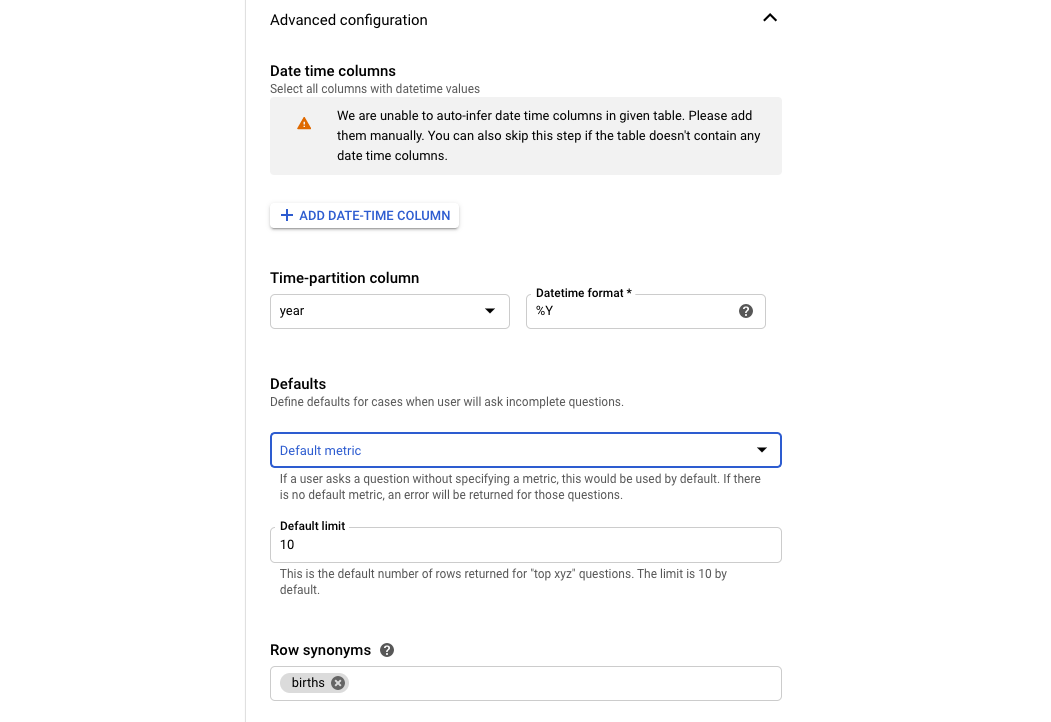
This is it, lets try it out!
就是这样,让我们尝试一下!
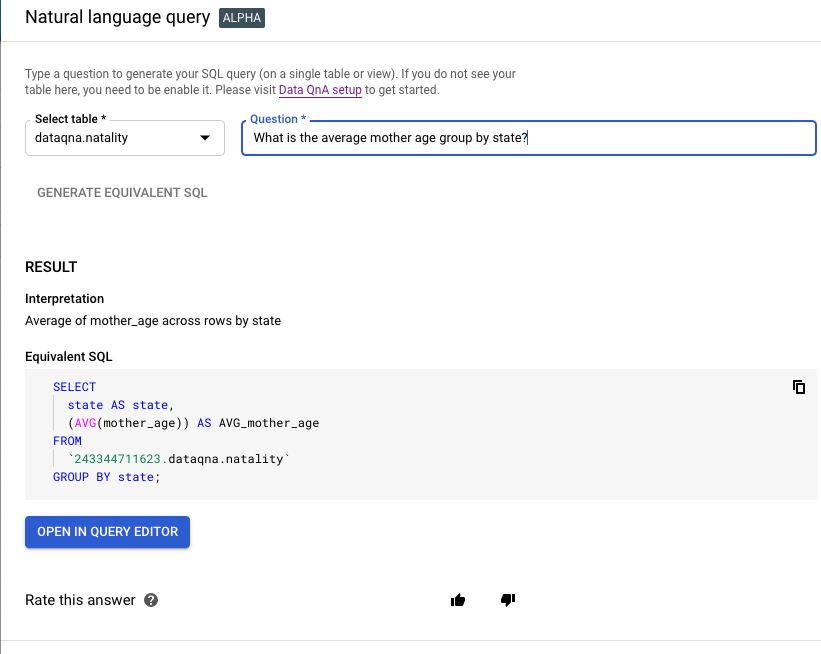
Q: What is the average mother age group by state sort by mother age?
问:按州按母亲年龄排序的平均母亲年龄组是多少?
SELECTstate AS state,(AVG(mother_age)) AS AVG_mother_ageFROM`dataqna.natality`GROUP BY stateORDER BY AVG_mother_age DESCLIMIT 10;
Q: Calculate number of births by year
问:按年份计算出生人数
SELECTyear AS year,(COUNT(*)) AS COUNT__ROWS_FROM`dataqna.natality`GROUP BY year;
Q: How is average gestation weeks affected by drinking?
问:饮酒如何影响平均孕周?
SELECTalcohol_use AS alcohol_use,(AVG(gestation_weeks)) AS AVG_gestation_weeksFROM`dataqna.natality`GROUP BY alcohol_use;
Q: What is the most frequent month?
问:什么是最频繁的月份?
SELECTmonth AS month,(COUNT(*)) AS COUNT__ROWS_FROM`dataqna.natality`GROUP BY monthORDER BY COUNT__ROWS_ DESCLIMIT 10;
Q: Calculate top record weight by different child race
问:按不同的子种族计算最高体重记录
SELECTchild_race AS child_race,(SUM(record_weight)) AS SUM_record_weightFROM`dataqna.natality`GROUP BY child_raceORDER BY SUM_record_weight DESCLIMIT 10;
Finally, a detail that cannot be neglected is the access channel to this tool, again the simpler and more natural this channel is, the more engagement we will have.
最后,一个无法忽视的细节是该工具的访问渠道,同样,这个渠道越简单和自然,我们将拥有更多的参与度。
Data QnA offers an API and client libraries that can be used to embed it in other interfaces, for instance you can integrate Data QnA into experiences built with Google Dialogflow. Data QnA enforces all underlying customer-defined data access policies, automatically restricting access of data to the right users.
Data QnA提供了API和客户端库,可用于将其嵌入其他界面,例如,您可以将Data QnA集成到使用Google Dialogflow构建的体验中。 数据QnA会强制执行所有基础的客户定义的数据访问策略,从而自动将数据访问限制为正确的用户。
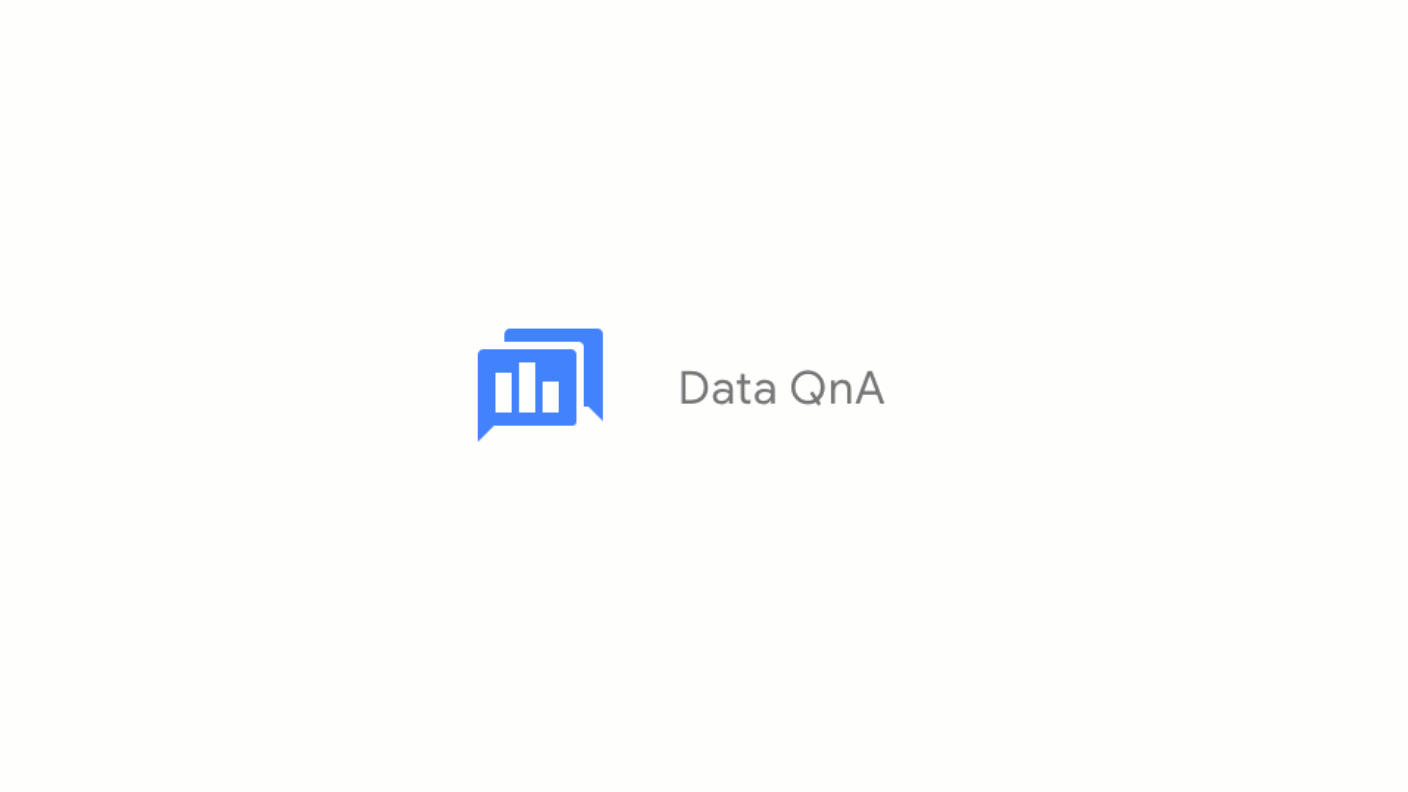
Now, go and democratize data analytics.
现在,使数据分析民主化。
Yours truly,
敬上,