机器学习 数据模型
My previous post Machine Learning in SQL using PyCaret 1.0 provided details about integrating PyCaret with SQL Server. In this article, I will provide step-by-step details on how to train and deploy a Supervised Machine Learning Classification model in SQL Server using PyCaret 2.0 (PyCaret is a low-code ML library in Python).
我以前的文章使用PyCaret 1.0在SQL中进行机器学习提供了有关将PyCaret与SQL Server集成的详细信息。 在本文中,我将提供有关如何使用PyCaret 2.0在SQL Server中训练和部署监督机器学习分类模型的分步详细信息。 (PyCaret是Python中的低代码ML库) 。
Things to be covered in this article:
本文涉及的内容:
1. How to load data into SQL Server table
1.如何将数据加载到SQL Server表中
2. How to create and save a model in SQL Server table
2.如何在SQL Server表中创建和保存模型
3. How to make model predictions using the saved model and store results in the table
3.如何使用保存的模型进行模型预测并将结果存储在表中
I.导入/加载数据 (I. Import/Load Data)
You will now have to import CSV file into a database using SQL Server Management Studio.
现在,您将必须使用SQL Server Management Studio将CSV文件导入数据库。
Create a table “cancer” in the database
在数据库中创建一个表“ Cancer ”
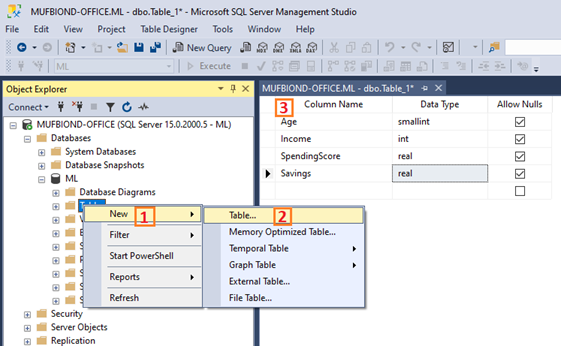
Right-click the database and select Tasks -> Import Data
右键单击数据库,然后选择任务 -> 导入数据
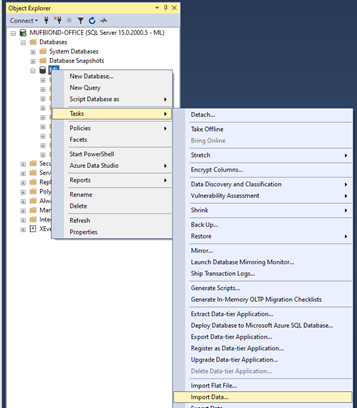
For Data Source, select Flat File Source. Then use the Browse button to select the CSV file. Spend some time configuring the data import before clicking the Next button.
对于数据源,选择平面文件源 。 然后使用浏览按钮选择CSV文件。 在单击“ 下一步”按钮之前,请花一些时间配置数据导入。
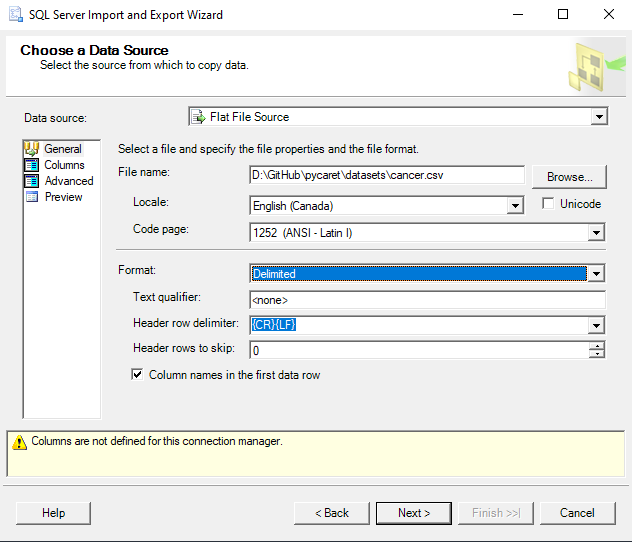
For Destination, select the correct database provider (e.g. SQL Server Native Client 11.0). Enter the Server name; check Use SQL Server Authentication, enter the Username, Password, and Database before clicking the Next button.
对于“目标”,选择正确的数据库提供程序(例如SQL Server Native Client 11.0)。 输入服务器名称 ; 选中“ 使用SQL Server身份验证” ,然后输入“ 用户名” ,“ 密码 ”和“ 数据库”,然后单击“ 下一步”按钮。
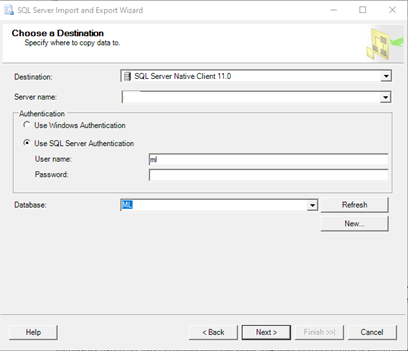
In the Select Source Tables and Views window, you can Edit Mappings before clicking the Next button.
在“选择源表和视图”窗口中,可以在单击“ 下一步”之前编辑“映射” 。
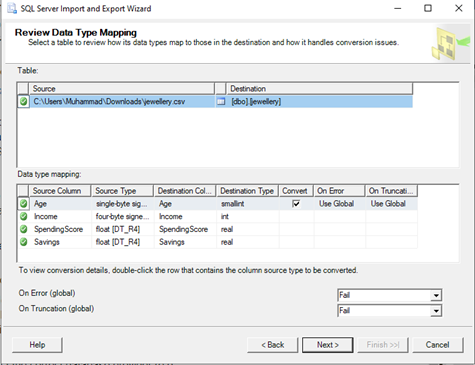
Check Run immediately and click the Next button
选中立即运行,然后单击下一步按钮
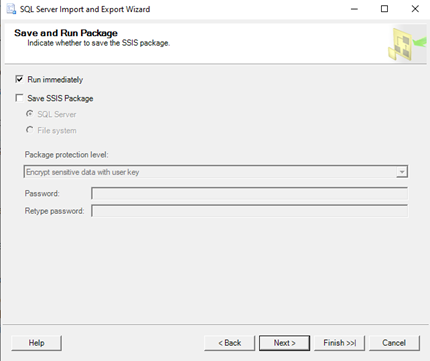
Click the Finish button to run the package
单击完成按钮运行程序包
二。 创建ML模型并保存在数据库表中 (II. Create ML Model & Save in Database Table)
Classification is a type of supervised machine learning to predict the categorical class labels which are discrete and unordered. The module available in the PyCaret package can be used for binary or multiclass problems.
分类是一种有监督的机器学习,用于预测离散且无序的分类类别标签 。 PyCaret软件包中提供的模块可用于解决二进制或多类问题。
In this example, we will be using a ‘Breast Cancer Dataset’. Creating and saving a model in a database table is a multi-step process. Let’s go by them step by step:
在此示例中,我们将使用“ 乳腺癌数据集 ”。 在数据库表中创建和保存模型是一个多步骤的过程。 让我们一步一步地走:
i. Create a stored procedure to create a trained model in this case an Extra Trees Classifier algorithm. The procedure will read data from the cancer table created in the previous step.
一世。 在这种情况下,使用Extra Trees Classifier算法创建存储过程以创建训练模型。 该程序将从上一步创建的癌症表中读取数据。
Below is the code used to create the procedure:
以下是用于创建该过程的代码:
-- Stored procedure that generates a PyCaret model using the cancer data using Extra Trees Classifier AlgorithmDROP PROCEDURE IF EXISTS generate_cancer_pycaret_model;GoCREATE PROCEDURE generate_cancer_pycaret_model (@trained_model varbinary(max) OUTPUT) ASBEGINEXECUTE sp_execute_external_script@language = N'Python', @script = N'import pycaret.classification as cpimport pickletrail1 = cp.setup(data = cancer_data, target = "Class", silent = True, n_jobs=None)# Create Model
et = cp.create_model("et", verbose=False)#To improve our model further, we can tune hyper-parameters using tune_model function.
#We can also optimize tuning based on an evaluation metric. As our choice of metric is F1-score, lets optimize our algorithm!tuned_et = cp.tune_model(et, optimize = "F1", verbose=False)#The finalize_model() function fits the model onto the complete dataset.
#The purpose of this function is to train the model on the complete dataset before it is deployed in productionfinal_model = cp.finalize_model(tuned_et)# Before saving the model to the DB table, convert it to a binary objecttrained_model = []
prep = cp.get_config("prep_pipe")
trained_model.append(prep)
trained_model.append(final_model)
trained_model = pickle.dumps(trained_model)', @input_data_1 = N'select "Class", "age", "menopause", "tumor_size", "inv_nodes", "node_caps", "deg_malig", "breast", "breast_quad", "irradiat" from dbo.cancer', @input_data_1_name = N'cancer_data', @params = N'@trained_model varbinary(max) OUTPUT', @trained_model = @trained_model OUTPUT;END;GO
ii. Create a table that is required to store the trained model object
ii。 创建存储训练的模型对象所需的表
DROP TABLE IF EXISTS dbo.pycaret_models;GOCREATE TABLE dbo.pycaret_models (
model_id INT NOT NULL PRIMARY KEY,
dataset_name VARCHAR(100) NOT NULL DEFAULT('default dataset'),
model_name VARCHAR(100) NOT NULL DEFAULT('default model'),
model VARBINARY(MAX) NOT NULL
);GO
iii. Invoke stored procedure to create a model object and save into a database table
iii。 调用存储过程以创建模型对象并保存到数据库表中
DECLARE @model VARBINARY(MAX);
EXECUTE generate_cancer_pycaret_model @model OUTPUT;
INSERT INTO pycaret_models (model_id, dataset_name, model_name, model) VALUES(2, 'cancer', 'Extra Trees Classifier algorithm', @model);
The output of this execution is:
该执行的输出为:
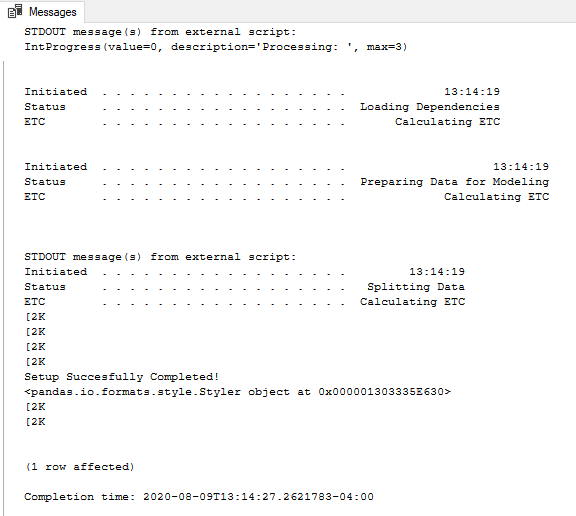
The view of table results after saving model
保存模型后的表结果视图

三, 运行预测 (III. Running Predictions)
The next step is to run the prediction for the test dataset based on the saved model. This is again a multi-step process. Let’s go through all the steps again.
下一步是根据保存的模型为测试数据集运行预测。 这又是一个多步骤的过程。 让我们再次完成所有步骤。
i. Create a stored procedure that will use the test dataset to detect cancer for a test datapoint
一世。 创建一个存储过程,该过程将使用测试数据集来检测测试数据点的癌症
Below is the code to create a database procedure:
下面是创建数据库过程的代码:
DROP PROCEDURE IF EXISTS pycaret_predict_cancer;
GOCREATE PROCEDURE pycaret_predict_cancer (@id INT, @dataset varchar(100), @model varchar(100))
ASBEGINDECLARE @py_model varbinary(max) = (select modelfrom pycaret_modelswhere model_name = @modeland dataset_name = @datasetand model_id = @id);EXECUTE sp_execute_external_script@language = N'Python',@script = N'# Import the scikit-learn function to compute error.import pycaret.classification as cpimport picklecancer_model = pickle.loads(py_model)# Generate the predictions for the test set.predictions = cp.predict_model(cancer_model, data=cancer_score_data)OutputDataSet = predictionsprint(OutputDataSet)', @input_data_1 = N'select "Class", "age", "menopause", "tumor_size", "inv_nodes", "node_caps", "deg_malig", "breast", "breast_quad", "irradiat" from dbo.cancer', @input_data_1_name = N'cancer_score_data', @params = N'@py_model varbinary(max)', @py_model = @py_modelwith result sets (("Class" INT, "age" INT, "menopause" INT, "tumor_size" INT, "inv_nodes" INT,"node_caps" INT, "deg_malig" INT, "breast" INT, "breast_quad" INT,"irradiat" INT, "Class_Predict" INT, "Class_Score" float ));END;GO
ii. Create a table to save the predictions along with the dataset
ii。 创建一个表以将预测与数据集一起保存
DROP TABLE IF EXISTS [dbo].[pycaret_cancer_predictions];GOCREATE TABLE [dbo].[pycaret_cancer_predictions]([Class_Actual] [nvarchar] (50) NULL,[age] [nvarchar] (50) NULL,[menopause] [nvarchar] (50) NULL,[tumor_size] [nvarchar] (50) NULL,[inv_nodes] [nvarchar] (50) NULL,[node_caps] [nvarchar] (50) NULL,[deg_malig] [nvarchar] (50) NULL,[breast] [nvarchar] (50) NULL,[breast_quad] [nvarchar] (50) NULL,[irradiat] [nvarchar] (50) NULL,[Class_Predicted] [nvarchar] (50) NULL,[Class_Score] [float] NULL) ON [PRIMARY]GO
iii. Call pycaret_predict_cancer procedure to save predictions result into a table
iii。 调用pycaret_predict_cancer过程将预测结果保存到表中
--Insert the results of the predictions for test set into a tableINSERT INTO [pycaret_cancer_predictions]EXEC pycaret_predict_cancer 2, 'cancer', 'Extra Trees Classifier algorithm';
iv. Execute the SQL below to view the result of the prediction
iv。 执行以下SQL以查看预测结果
-- Select contents of the tableSELECT * FROM [pycaret_cancer_predictions];
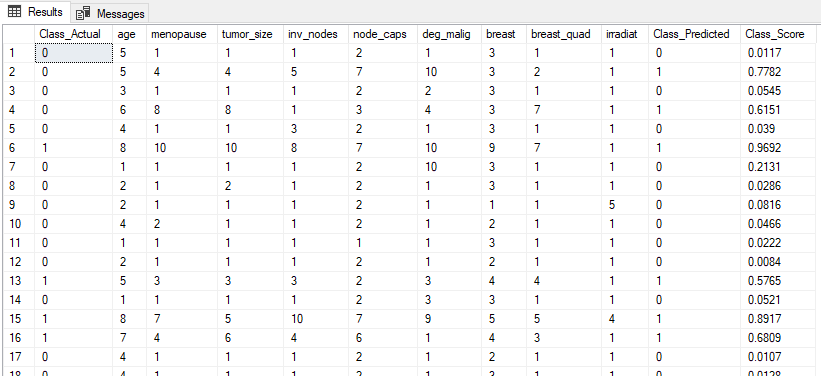
IV。 结论 (IV. Conclusion)
In this post, we learnt how to build a classification model using a PyCaret in SQL Server. Similarly, you can build and run other types of supervised and unsupervised ML models depending on the need of your business problem.
在本文中,我们学习了如何在SQL Server中使用PyCaret构建分类模型。 同样,您可以根据业务问题的需要来构建和运行其他类型的受监督和不受监督的ML模型。
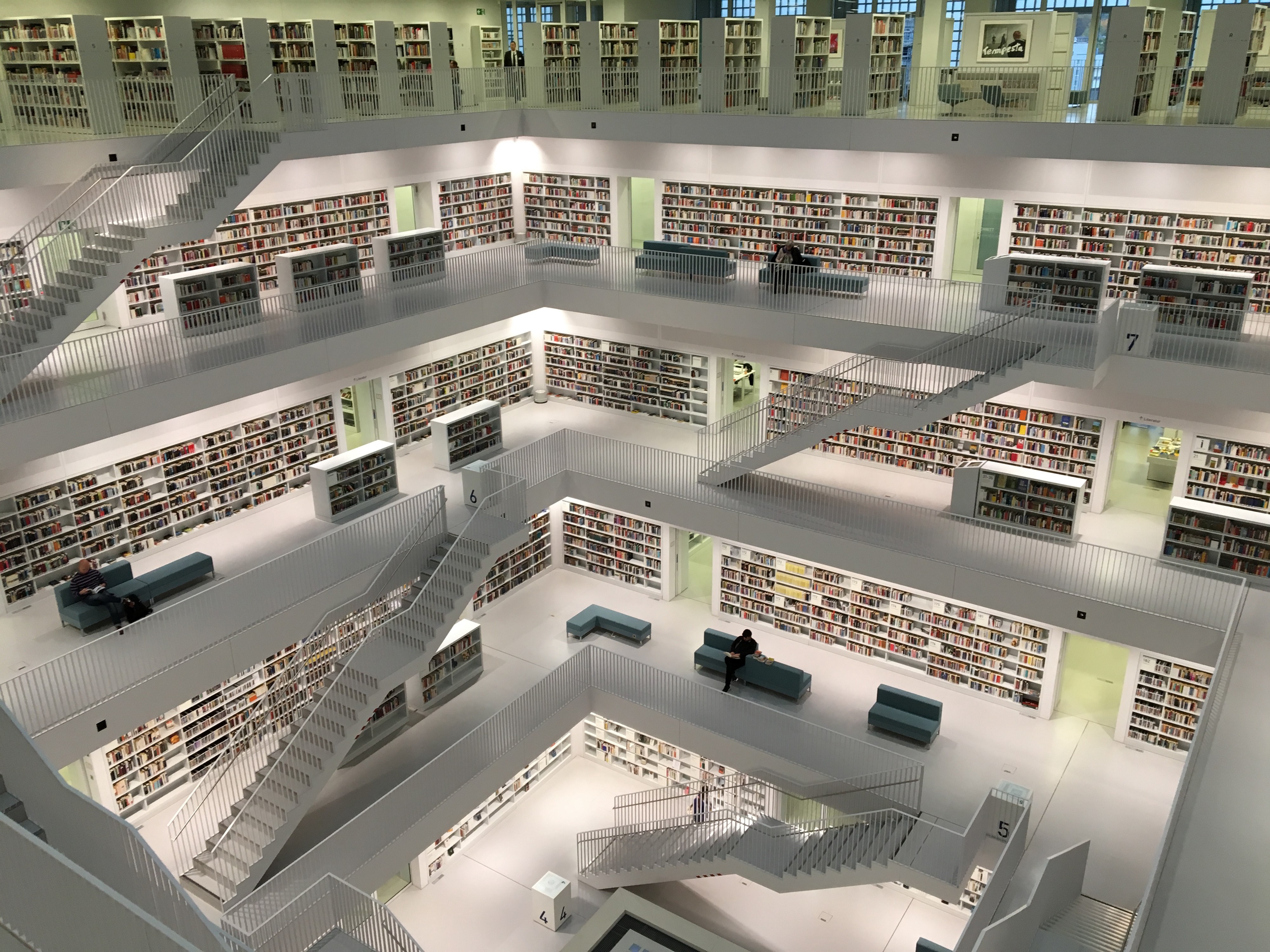
You can further check out the PyCaret website for documentation on other supervised and unsupervised experiments that can be implemented in a similar manner within SQL Server.
您可以进一步访问PyCaret网站,以获取其他可以在SQL Server中以类似方式实施的有监督和无监督实验的文档。
My future posts will be tutorials on exploring other supervised & unsupervised learning techniques using Python and PyCaret within a SQL Server.
我未来的文章将是有关在S QL服务器中使用Python和PyCaret探索其他有监督和无监督学习技术的教程。
五,重要链接 (V. Important Links)
翻译自: https://towardsdatascience.com/ship-ml-model-to-data-using-pycaret-part-ii-6a8b3f3d04d0
机器学习 数据模型