In January 2020, I published Facebook Advertising Decoded in 15 Minutes. It shared principles and examples I learned working at Facebook from 2012 to 2019, investing over $1 billion on FB and Instagram ads.
2020年1月,我发表了《 15分钟内解码的Facebook广告》 。 它分享了我在2012年至2019年期间在Facebook工作的学习原则和示例,在FB和Instagram广告上投资了超过10亿美元。
I originally wrote the essay to avoid talking about ads, because I was swamped with requests for advice from various friends and founders. But instead, I found myself having more conversations than ever about Facebook direct response (DR) ads in particular, with a whole new group of VCs, Direct-To-Consumer (DTC) brands, e-commerce and agency founders.
我最初写这篇文章是为了避免谈论广告,因为我被各种各样的朋友和创始人的咨询请求所淹没。 但是,相反,我发现自己与Facebook直接响应(DR)广告的对话比以往任何时候都多,与一群全新的VC,直接面向消费者(DTC)的品牌,电子商务和代理商创始人进行了对话。
The thrust of that first essay was simple:
第一篇文章的重点很简单:
Use Facebook the way it was intended to be used, which means bringing real value to users with fresh, native content, and running liquid campaigns that allow the new Machine Learning capabilities to work their magic.
以原本打算的方式使用Facebook,这意味着以新鲜的本地内容为用户带来真正的价值,并开展流动性活动,使新的机器学习功能发挥其神奇作用。
But my conversations kept coming back to one pain-point: how to generate and test a variety of high-quality Facebook and Instagram DR ads, fast and affordably.
但是我的谈话一直回到一个痛点: 如何快速,经济地生成和测试各种高质量的Facebook和Instagram DR广告。
Recent meta-analysis data from Facebook’s data team across 163 campaigns proves that testing and learning works.
来自Facebook数据团队的163个活动的最新荟萃分析数据证明,测试和学习是有效的。
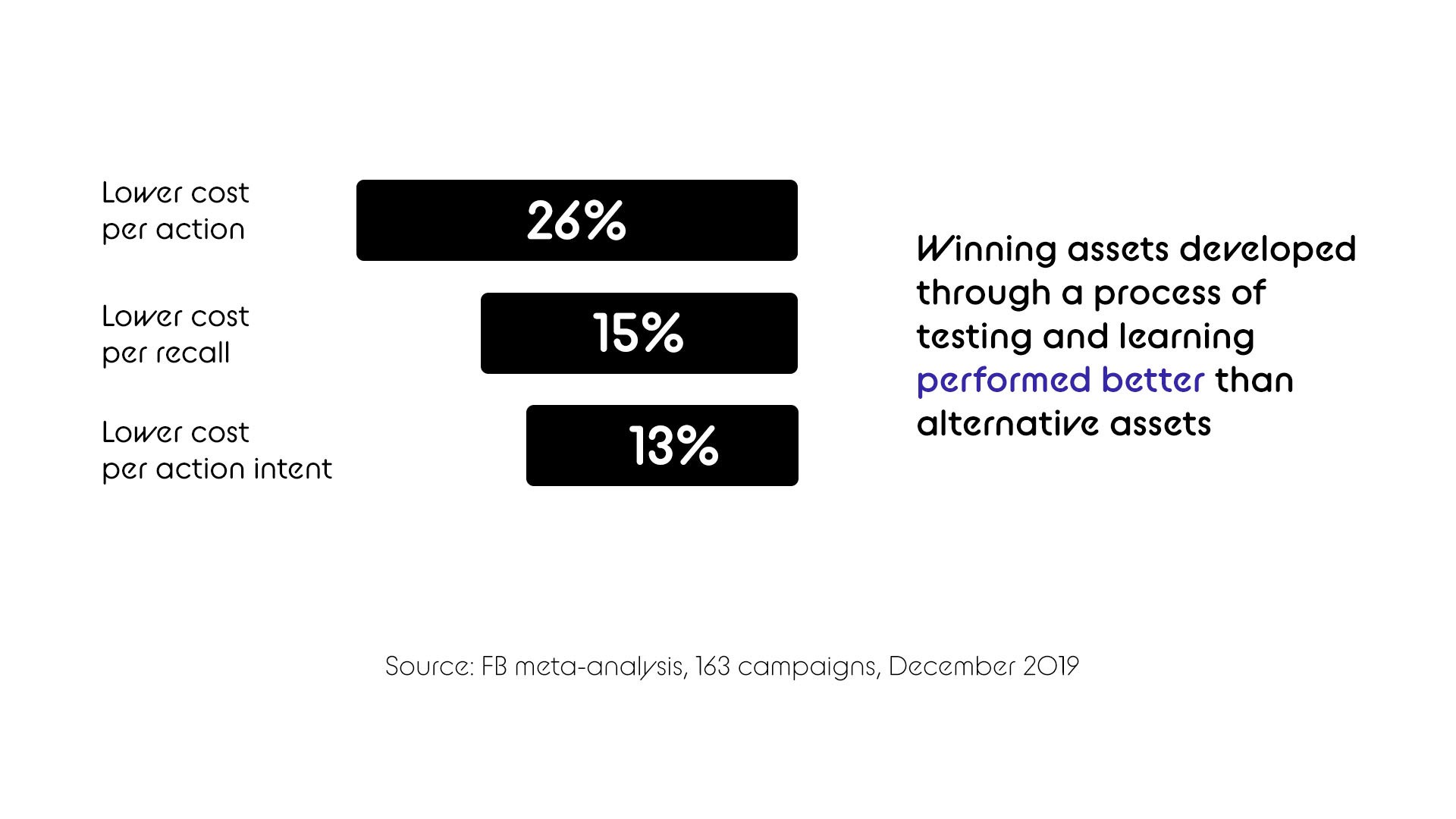
Listening to advertiser’s challenges, I realized that only Machine Generated Content (MGC) could solve this asset bottleneck, so I reached out to a Sequoia-backed Singapore startup called Pencil.
听到广告商的挑战后,我意识到只有机器生成的内容(MGC)才能解决这一资产瓶颈,因此我接触了红杉资本支持的新加坡初创公司Pencil。
Why the name Pencil? Co-founders Will Hanschell and Sumukh Avadhani believe AI will be as fundamental to creativity in the future as the pencil was in the past.
为什么叫铅笔? 联合创始人Will Hanschell和Sumukh Avadhani相信, 人工智能将像过去的铅笔一样,成为未来创造力的基础。
They patiently explained the following ML thesis to me.
他们耐心地向我解释了以下机器学习论文。
To design a learning-system to beat ROAS targets, Pencil would need to build everything into a single MGC self-serve platform, and it would need to
为了设计一个学习系统以实现ROAS目标,Pencil需要将所有内容构建到单个MGC自助服务平台中,并且需要
- generate strong headlines and product descriptions using advanced language models 使用高级语言模型生成强大的标题和产品描述
- breakdown brand films and influencer videos into short atomized clips 将品牌电影和有影响力的视频分解为短片段
- use these to generate new Facebook ads in dozens of formats using region-of-interest technology 使用这些来使用感兴趣区域技术以多种格式生成新的Facebook广告
- understand what’s “inside” generated ads across hundreds of learnable features 了解数百种可学习功能中“内部”生成的广告是什么
- rank ads by predicted outcome 按预测结果对广告进行排名
- push batches of new ads into Facebook Ads Manager 将一批新广告推送到Facebook Ads Manager中
- gather high-fidelity signal about campaign performance, relative to objective and audience 收集有关广告活动效果的高保真信号,相对于目标和受众
- use this signal to keep training the AI to constantly iterate better ads 使用此信号来持续训练AI以不断迭代更好的广告
Because Pencil was generating the ads from labeled, atomic parts, and getting a strong, fast, signal back, only then might it generate and predict outcomes accurately.
因为Pencil是从带有标签的原子部分生成广告的,并获得强烈,快速的信号回馈,所以只有这样,它才能准确地生成和预测结果。
The first MGC campaigns they showed me were already strong, and improving weekly. Creative AI had clearly arrived, and I wanted a front-row seat, so within a week, I dropped what I was doing to help build and test Pencil.
他们向我展示的第一批MGC运动已经很强大,并且每周都有改进。 Creative AI显然已经到了,我想要一个前排的座位,所以在一周之内,我放弃了自己的工作来帮助构建和测试Pencil。
Within a month, we had some of the world’s top performance marketers onboard, with 15 US DTC brands testing ads like these on live campaigns:
一个月之内,我们就吸引了一些全球顶级的绩效营销人员,有15个美国DTC品牌在实时广告系列中测试了以下广告:
To explore testing ads like these for your brand, join the Pencil waitlist or contact me on LinkedIn and tell me about your brand or agency.
要探索针对您的品牌的此类测试广告,请加入“铅笔”等待列表, 或 在LinkedIn上 与我 联系, 并向我介绍您的品牌或代理商。
This essay covers what I’ve learned so far working with User Acquisition teams, AI engineers, and Facebook in-house teams.
本文涵盖了迄今为止与用户获取团队,AI工程师和Facebook内部团队合作所学到的知识。
It tells a story in three chapters:
它通过三个章节讲述了一个故事:
Why humans fail at generating Facebook ads
人类为何无法生成Facebook广告
Why machines do it better
为什么机器做得更好
How advertisers can start now
广告商如何立即开始
But first, a few observations:
但是首先,一些观察:
The COVID pandemic has accelerated many inevitable business trends by a decade. Creative development is now almost all remote and increasingly automated.
十年来,COVID大流行加速了许多不可避免的商业趋势。 现在,创意开发几乎全部是远程的并且越来越自动化。
There’s a lot of well-deserved hype around AI, especially after July 2020, when expert reviewers got access to OpenAI’s GPT-3, a powerful language-generation tool capable of producing human-like text on demand. Hype imagines possible futures, but this essay will focus on the very real MGC commercial opportunities today.
围绕AI有很多当之无愧的炒作 ,尤其是在2020年7月之后,当时专家评审获得了OpenAI的GPT-3的支持,GPT-3是一种强大的语言生成工具,能够按需生成类似人的文本。 Hype设想了可能的未来,但是本文将重点关注当今非常真实的MGC商业机会。
DR is eating brand advertising. Direct Response (DR) ads continue to outweigh brand ads on Facebook, by about four times. The best ads live or die by daily DR metrics, ROAS, CPA, CTRs, and strengthen the brand at the same time. According to one theory, Trump beat Hilary in 2016 because his campaign team ran DR campaigns to raise money while Clinton’s tried to run brand issue campaigns. Even Instagram, fueled by a hunger for inspiration, allows one-touch shopping now.
DR正在吃品牌广告。 直接响应(DR)广告继续超过Facebook上的品牌广告,大约四倍。 最好的广告会根据每日的灾难恢复指标,广告支出回报率,每次转化费用,点击率确定有效或无效标准,并同时增强品牌知名度。 根据一种理论,特朗普在2016年击败希拉里是因为他的竞选团队进行了DR竞选以筹集资金,而克林顿则试图开展品牌发行竞选。 甚至在渴望灵感的刺激下,Instagram现在也允许一键式购物。
MGC will impact all creative fields, but it will be sharpened first on Facebook and Instagram. The ad auction is nearing a $100 billion marketplace, powered by testing tools and fast, granular signal. By incorporating most of the data/ML into its own native tools, creative is now one of the few levers Facebook still wants businesses to pull.
MGC将影响所有创意领域,但首先将在Facebook和Instagram上得到加强。 由测试工具和快速,细粒度的信号驱动的广告拍卖市场接近1000亿美元。 通过将大多数数据/ ML集成到其自身的本地工具中,创意现已成为Facebook仍希望企业借鉴的少数杠杆之一。
This essay won’t cover all the political and ethical angles to FB’s business model, or the potential for AI to replace human jobs. Those areas interest me but would distract from my story. Suffice to say, I believe that Humans + Machines = Superhumans, and I tell my own kids to learn to write code if they want jobs in the future. (Of course, they don’t listen to me.)
本文不会涵盖FB商业模式的所有政治和道德角度,也不会涵盖AI取代人类工作的潜力。 这些领域使我感兴趣,但会分散我的故事注意力。 可以说,我相信人类+机器=超人类,而且我告诉自己的孩子,如果他们将来想要工作,他们会学习编写代码。 (当然,他们不听我的话。)
According to Nielsen, 50%+ of ad performance is driven by creative choices, and yet founders and CEOs typically spend a fraction of their time improving output. Here’s the math:
根据尼尔森(Nielsen)的说法,广告效果的50%以上是由创造性的选择决定的 ,但创始人和首席执行官通常会花费一小部分时间来提高产出。 这是数学:
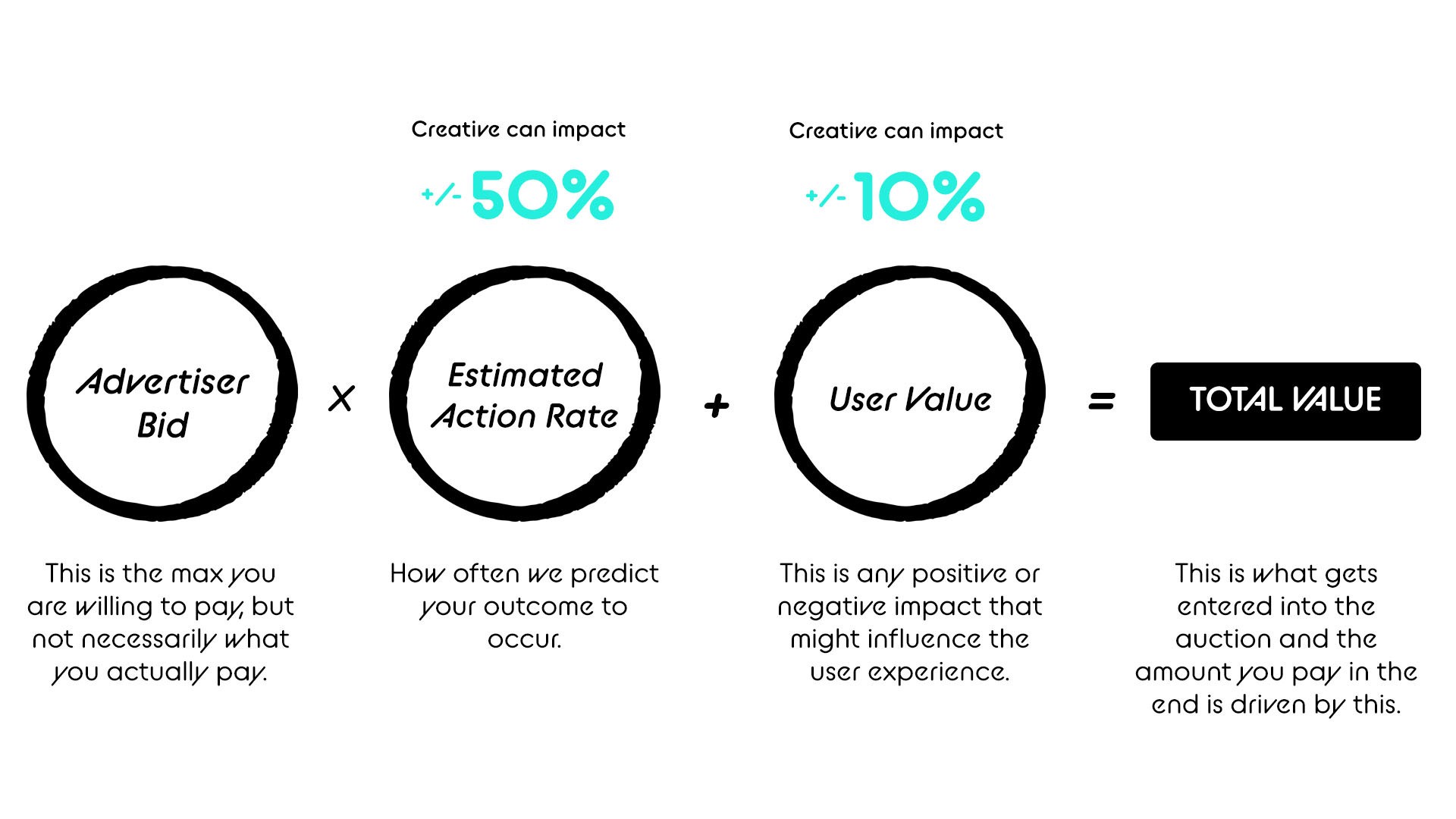
第1章:人类为何无法生成FB广告 (Chapter 1: Why Humans Fail at Generating FB Ads)
On one of my first customer calls I was asked “How long before I can take all our people fully out of the loop?” I was surprised because I’d expected more sensitivity about machines replacing humans.
在我的第一个客户电话中,有人问我:“我要花多长时间才能使所有员工完全摆脱困境?” 我很惊讶,因为我期望对替代人类的机器更加敏感。
“We’re still 6 months or so away,” I guessed, “But why do you ask?”
“我距离我们还有6个月左右的时间,但是你为什么要问?”
“Because humans make horrible Facebook ads, and we usually just get in the way,” she said.
她说:“因为人类制作了可怕的Facebook广告,而我们通常会挡在路上。”
I thought about the dire ads in my own Newsfeed and FB Ads Library, and I realized she was right: humans usually suck at Facebook ads. I decided to study why, and identified ten design faults with human brains and organizations that get in the way, drawing heavily on the work of Dan Kahneman and Shane Parrish at Farnam Street.
我想到了自己的Newsfeed和FB Ads库中最可怕的广告,但我意识到她是对的:人们通常会在Facebook广告上吮吸。 我决定研究原因,并找出了十个人脑和组织受阻的设计缺陷,并在很大程度上借鉴了Farnam街的Dan Kahneman和Shane Parrish的工作。
“Fail” is a strong word, so we should define it. The majority of Facebook ads fail to hit their ROAS or CPA targets. But even when they succeed, if a team spent five weeks, six video calls, and spent $20k to create an ad, that’s still a failure in my book.
“失败”是一个很强的词,因此我们应该对其进行定义。 大多数Facebook广告均未达到广告支出回报率或CPA目标。 但是,即使他们成功了,如果一个团队花了五个星期,进行了六个视频通话,并花了2万美元制作一个广告,那对我来说仍然是一个失败。
What does success look like? Effectiveness + Efficiency + Cost.
成功是什么样的? 效率+效率+成本。
Or in plainer language: it must be good, fast and cheap, and I’ll unpack this in Chapter 2.
或用通俗易懂的语言:它一定是好的,快速且便宜的,我将在第2章中解压缩。
Here are the 10 design faults:
这是10个设计错误:
- Humans don’t scale 人类无法扩展
- Humans develop biases 人类会产生偏见
- Humans resist change 人类抵抗变化
- Humans hate criticism 人类讨厌批评
- Humans develop blind spots 人类发展盲点
- Humans chase incentives 人类追逐激励
- Humans build hierarchies 人类建立等级制度
- Humans get math wrong 人类弄错了数学
- Humans follow the herd 人类跟随牛群
- Humans panic 人类恐慌
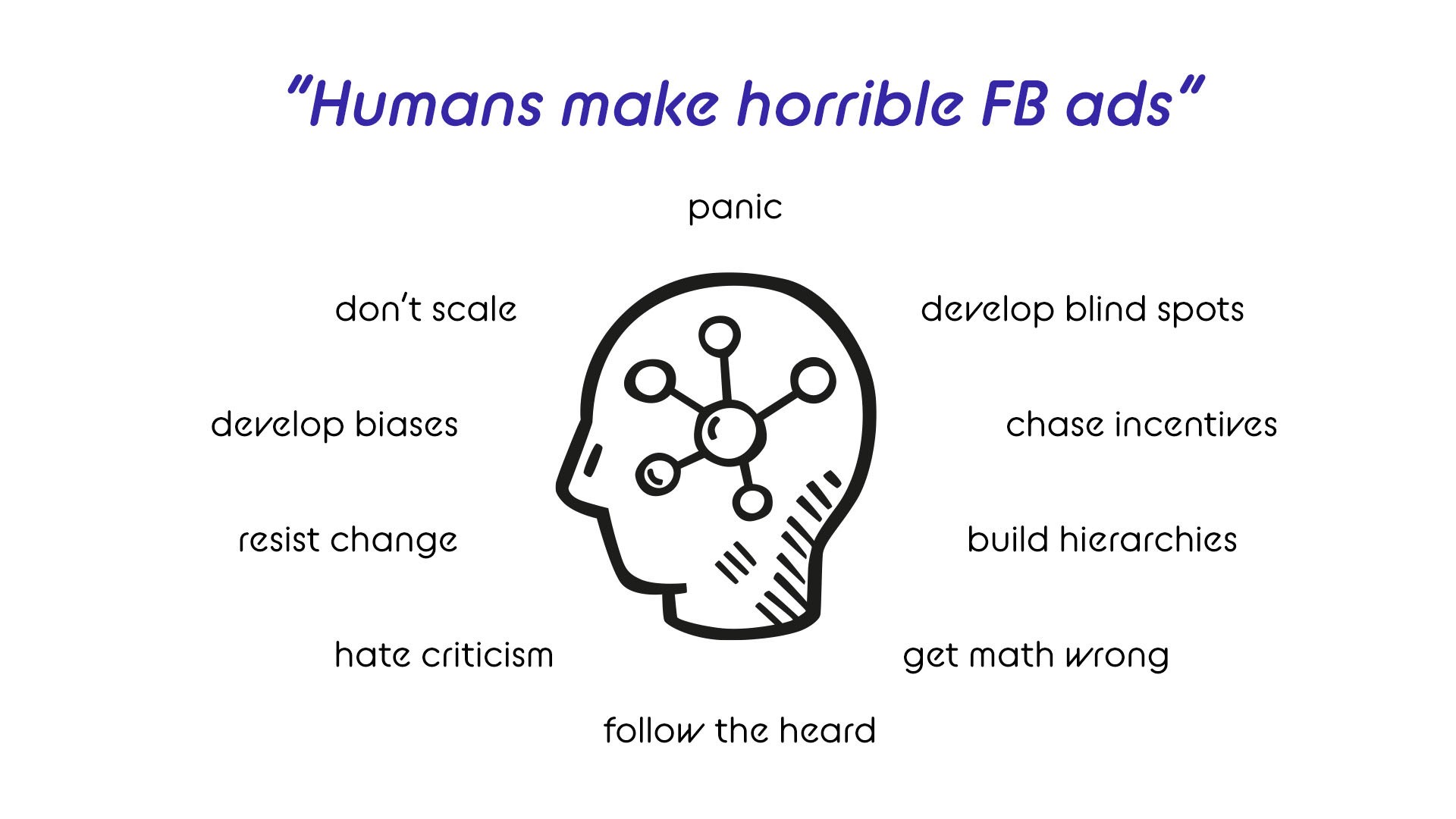
Humans don’t scale. In general, teams scale linearly, so as workload ramps up, so does hiring and cost. When a creative team tries to unlock a new Facebook ad account, they usually struggle.
人类无法扩展。 通常,团队会线性扩展,因此随着工作量的增加,招聘和成本也会增加。 当创意团队尝试解锁新的Facebook广告帐户时,他们通常会遇到困难。
- First, there are an infinite number of ads they could generate, and no clear data showing where to start. 首先,它们可以生成无限数量的广告,也没有清晰的数据显示从何开始。
- Second, if they were only to test three headlines, three opening video frames, three format sizes, three durations, three CTA buttons, that’s already 3*3*3*3*3=243 potential versions. 其次,如果他们仅测试三个标题,三个打开的视频帧,三个格式大小,三个持续时间,三个CTA按钮,则它们已经是3 * 3 * 3 * 3 * 3 = 243个潜在版本。
- Third, I’m yet to meet a designer who can generate ten different approaches, day after day, without imploding. 第三,我还没有遇到可以每天产生十种不同方法而不会崩溃的设计师。
Humans develop biases. Designers cope by repeating familiar techniques and habits. They often lock into a template that worked well for one brand before, but by now it’s overused, or unsuitable for a new brand. Humans tend to overrate things we like and overlook things we dislike, even when we know we’re not the target audience.
人类会产生偏见。 设计师通过重复熟悉的技术和习惯来应对。 他们通常锁定在一个模板上,该模板以前曾对一个品牌有效,但到现在为止,它已被过度使用或不适用于新品牌。 即使我们知道自己不是目标受众,人们也会高估我们喜欢的事物而忽略我们不喜欢的事物。
Humans resist change. To paraphrase Newton’s Law of Inertia, an object doesn’t move, or continues on its path, unless acted upon. Humans are no different. We like to keep prior commitments and stay consistent. We fall prey to first-conclusion bias and settle for our first idea to save mental energy, when optimally, thousands of different ideas should be given equal consideration.
人类抵制变化。 用牛顿惯性定律来解释,除非作用,否则物体不会移动或在其路径上继续前进。 人类没有什么不同。 我们希望保持事先的承诺并保持一致。 我们会陷入第一个结论的偏见,并为节省精神能量而决定了我们的第一个主意,在最佳状态下,应平等考虑数千种不同的主意。
Humans hate criticism. Despite what most people claim, humans don’t enjoy feedback, and there are only so many rounds of “constructive edits” we can take before our creative juices dry up. Especially if our creations never see the light of day.
人类讨厌批评。 尽管大多数人都宣称,人类无法享受反馈,但是在我们的创意汁液干dry之前,我们只能进行几轮的“建设性编辑”。 尤其是如果我们的创作从未出现过。
Humans develop blind spots. When we only look at a problem one way, we develop a blind spot, which in advertising, as in driving, can kill. The human brain misses critical patterns that machines easily find, but our egos convince us that “gut instinct” is better. Denying reality can be a survival mechanism, a deliberate tactic, or both.
人类会发展盲点。 当我们仅以一种方式看待问题时,我们就会形成一个盲点,在广告中和开车中都会造成盲点。 人脑错过了机器容易找到的关键模式,但我们的自负使我们相信“直觉”更好。 否认现实可以是一种生存机制,一种故意的策略,或两者兼而有之。
Humans chase incentives. We repeat what earns rewards, but for humans this gets tricky fast. Recently I saw an agency kill an adset that was working because they wanted to pitch their own creative team. I’ve seen a VP Marketing shut down a valuable test for fear of making his previous KPI performance look soft. Humans are conflicted by overlapping financial and status incentives.
人类追求激励。 我们重复获得奖励的东西,但是对于人类来说,这变得棘手。 最近,我看到一家代理商杀死了一个正在工作的广告集,因为他们想组建自己的创意团队。 我已经看到营销副总裁关闭了一项有价值的测试,因为担心他以前的KPI表现看起来很柔和。 重叠的财务和地位激励措施给人类带来了冲突。
Humans build hierarchies. We believe we’re free-thinkers, but research shows we seek hierarchies like any other organism. The classic Stanford Prison and Milgram Experiments revealed the dangerous power of authority. In a dominance hierarchy, we take strong cues from our leaders, even if their creative judgment sucks. To overcome this at FB, when a team was struggling, we often removed members rather than adding them, to reduce hierarchy.
人类建立了等级制度 。 我们相信我们是自由思想者,但是研究表明我们在寻求像其他生物一样的等级制度。 经典的斯坦福监狱和米尔格拉姆实验揭示了权威的危险力量。 在支配地位的层次结构中,即使领导者的创造性判断令人沮丧,我们也会从他们的领导中获得强有力的暗示。 为了克服这个问题,在FB团队苦苦挣扎的时候,我们经常删除成员而不是添加成员,以减少层次结构。
Humans get math wrong. One common mistake is an obsession with CPA or ROAS targets rather than total value of sales. Performance is nothing without scale. Another issue is when teams take a small number of instances and create a general category, even if they have no statistically sound basis for the conclusion. A lesser-known type of volatility on Facebook is A/A variance, i.e. the persistent “background” randomness on the platform. Some expert estimates put this at 15–25%, so the exact same campaign that worked well today, might not work tomorrow. Data and design teams are often divided by a math gap, sometimes leading to mistrust and lost respect, but it’s important for teams to talk openly about these concepts. The best teams develop a common language, even if some members have a firmer grasp than others.
人类弄错了数学。 一个常见的错误是迷恋CPA或ROAS目标,而不是销售总价值。 没有规模,绩效就是一切。 另一个问题是,即使团队没有结论的统计依据,团队也只需要少量实例并创建一个通用类别。 Facebook上鲜为人知的波动类型是A / A方差,即平台上的持续“背景”随机性。 一些专家估计,这一比例为15%到25%,因此,今天效果很好的完全相同的广告系列可能明天就无法使用。 数据和设计团队经常会因数学差距而分开,有时会导致不信任和失去尊重,但是对于团队而言,公开讨论这些概念很重要。 即使有些成员比其他成员更坚定,最好的团队也会建立共同的语言。
Humans follow the herd. Too often, new DTC brands enter an existing category, and launch with creative that’s 95% similar to leading brands. They miss the underdog opportunity to behave like a category creator. Humans have a DNA-level instinct to seek safety in numbers and crave social approval.
人类跟随牛群。 通常,新的DTC品牌会进入现有类别,并以与领先品牌95%相似的广告素材进行发布。 他们错过了表现得像品类创造者的弱者机会。 人类具有DNA水平的本能,以寻求数量上的安全并渴望获得社会的认可。
Humans panic. When an acquisition team stops acquiring, or an agency can’t hit ROAS targets, we often see panic behavior. Structured testing loses structure. Stress causes both mental and physical responses and amplifies all the other human faults. Almost all human mental biases become worse in the face of stress as the body goes into a fight-or-flight response. Stress causes fear-based decisions and short-term optimization.
人类惊慌失措。 当收购团队停止收购,或者代理商无法实现ROAS目标时,我们经常会出现恐慌行为。 结构化测试会失去结构。 压力会引起身心React,并加剧其他所有人类过失。 随着身体进入战斗或逃跑React,几乎所有人类的心理偏见在面对压力时都会变得更加严重。 压力会导致基于恐惧的决策和短期优化。
The point of this survey of human design faults isn’t to hate on my species, but to highlight how and why things generally aren’t working well.
这项关于人为设计缺陷的调查的目的不是要讨厌我的物种,而是要强调事物通常如何以及为什么运转不佳。
As a quick test, take a moment to dive into Facebook’s Ads Library and review how many fresh, strong ads have run in the past week for your own brand or a competitor? What process and logic was used to pick these ads, out of all the infinite possibilities? And how many of our ten human design faults might have got in the way?
作为一项快速测试,花点时间进入Facebook的广告库,并回顾一下过去一周针对您自己的品牌或竞争对手投放了多少个新鲜,有力的广告? 从所有无限的可能性中,采用了什么过程和逻辑来挑选这些广告? 在我们的十个人为设计错误中,有多少可能会受到阻碍?
These are important questions because more than 50% of performance is now explained by the strength of your creative choices, and even larger brands test very few ads.
这些都是很重要的问题,因为现在您的广告素材选择力可以解释超过50%的效果,甚至更大的品牌也很少测试广告。
Creative teams are overwhelmed by the sheer volume of assets needed, and then get no feedback signal. Or worse, they feel highly frustrated that their ads don’t run. According to a Campaign Magazine article, 47% of agency folks in the US reported low or very low morale in 2016, up from a third the year before.
创意团队被所需的庞大资产所淹没,然后没有任何反馈信号。 更糟糕的是,他们对广告没有投放感到非常沮丧。 根据《 Campaign Magazine》的一篇文章,2016年美国47%的代理人报告士气低落或非常低,高于前一年的三分之一。
Enter the cavalry. Enter MGC.
输入骑兵。 输入MGC。
第2章:为什么机器做得更好 (Chapter 2: Why Machines Do It Better)
A machine will ultimately beat a human because it will never stop learning new rules.
机器最终将击败人类,因为它永远不会停止学习新规则。
In the first decade of mass mobile marketing (2010-2020), the game was all about humans learning new rules. We learned, for example, that vertical video ads ~15 seconds long, featuring social proof, with a ‘grabber’ upfront and a clear CTA button at the end, should perform well. We learned these rules, and now we use them to instruct humans or machines to follow them.
在大众移动营销的头十年(2010年至2020年)中,该游戏完全是关于人类学习新规则的。 例如,我们了解到,垂直视频广告长约15秒,具有社交证明,并且前端带有“抓取器”,并且末尾有清晰的CTA按钮,效果不错。 我们了解了这些规则,现在我们使用它们来指导人类或机器遵循这些规则。
This new decade will be about machines cracking a far more advanced “code” for each specific brand, category, or audience.
新的十年将涉及机器为每个特定品牌,类别或受众破解更高级的“代码”。
With classical programming and systems, you input rules and data, and you get answers. With ML you input data and answers, and it learns the rules.
使用经典的编程和系统,您输入规则和数据即可获得答案。 使用ML,您可以输入数据和答案,并且可以学习规则。
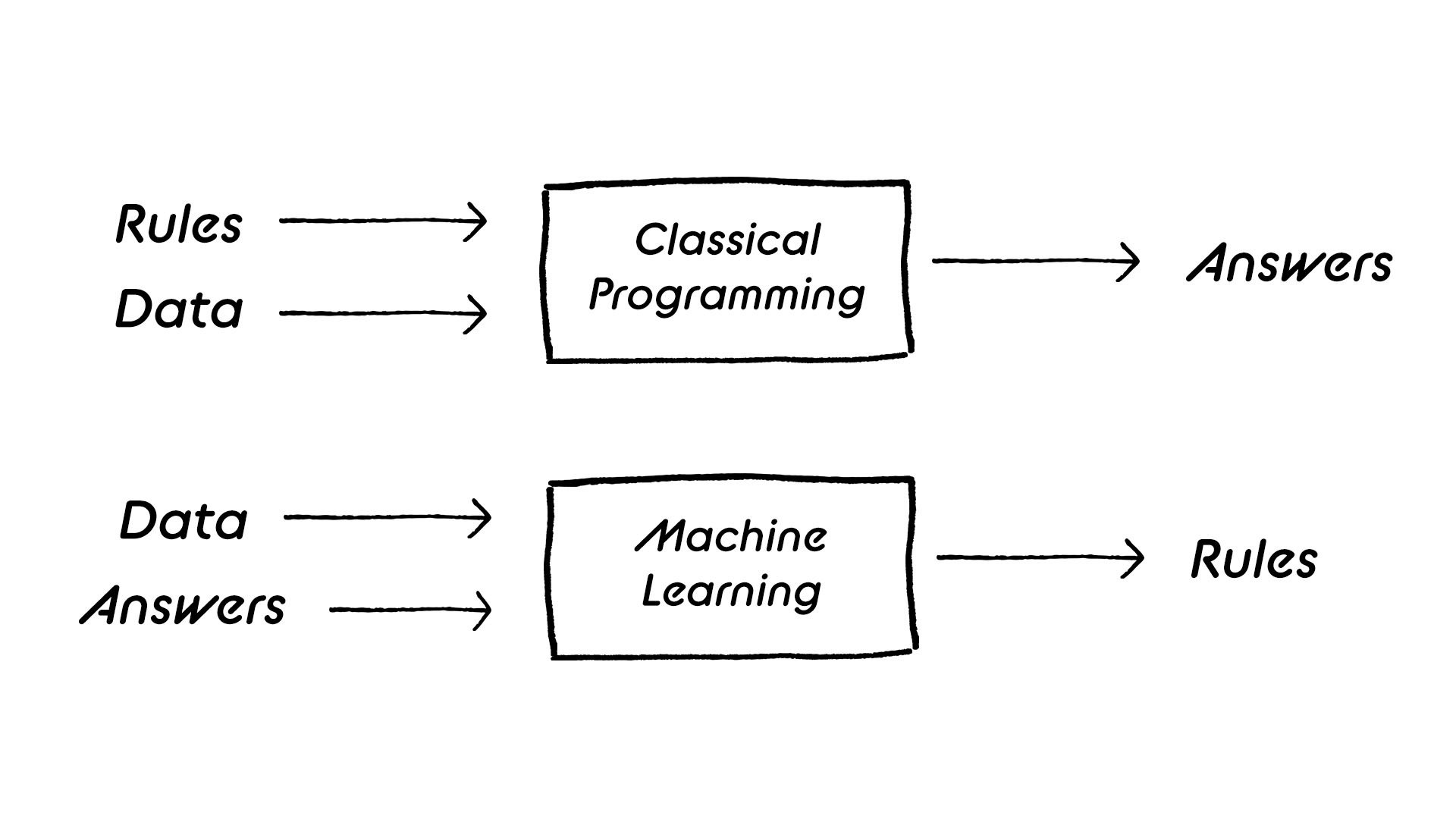
The companies or individuals who learn those new rules first will do well.
首先学习这些新规则的公司或个人会做得很好。
Imagine if a Fortune 500 brand like Coca-Cola or Nike harnessed ML to learn the codes across all their markets and categories so that one day the CMO could present those data-backed insights to her Board? That CMO could also explain that she has carved out data-backed insights which belong to the company, and aren’t hostage to a black-box algorithm inside one of the Big Tech platforms. At the enterprise level, MGC can generate scaled, proprietary, and sustainable data insights.
想象一下,如果像可口可乐或耐克这样的财富500强品牌利用ML来学习其所有市场和类别的代码,以便有一天CMO可以向董事会提出这些数据支持的见解? 该CMO还可以解释说,她已经获得了属于公司的数据支持的见解,并且没有成为Big Tech平台之一内部的黑匣子算法的人质。 在企业级别,MGC可以生成规模化,专有和可持续的数据见解。
Let’s use our definition of success: “is it Fast + Good + Cheap,” to understand why machines will win.
让我们用对成功的定义:“是快速+好+便宜”,以了解机器为什么会获胜。
When I started in marketing, this trifecta was often used to force trade-offs. A marketing director would complain about her agency’s performance, and the agency head would explain “you can have it fast and cheap, or good and cheap, or fast and good, but you can’t have all three.” It became a business truth universally acknowledged.
当我开始进行营销时,通常会使用这种三连胜来进行权衡。 营销总监会抱怨她的代理机构的业绩,而代理机构负责人会解释“您可以快速而便宜地买东西,或者便宜又好又快又好,但不能同时拥有这三个。” 这已成为公认的商业真理。
But with automated ML, it’s different. Now you can have it fast + good + cheap.
但是使用自动ML则有所不同。 现在,您可以快速,优质,廉价地买到它。
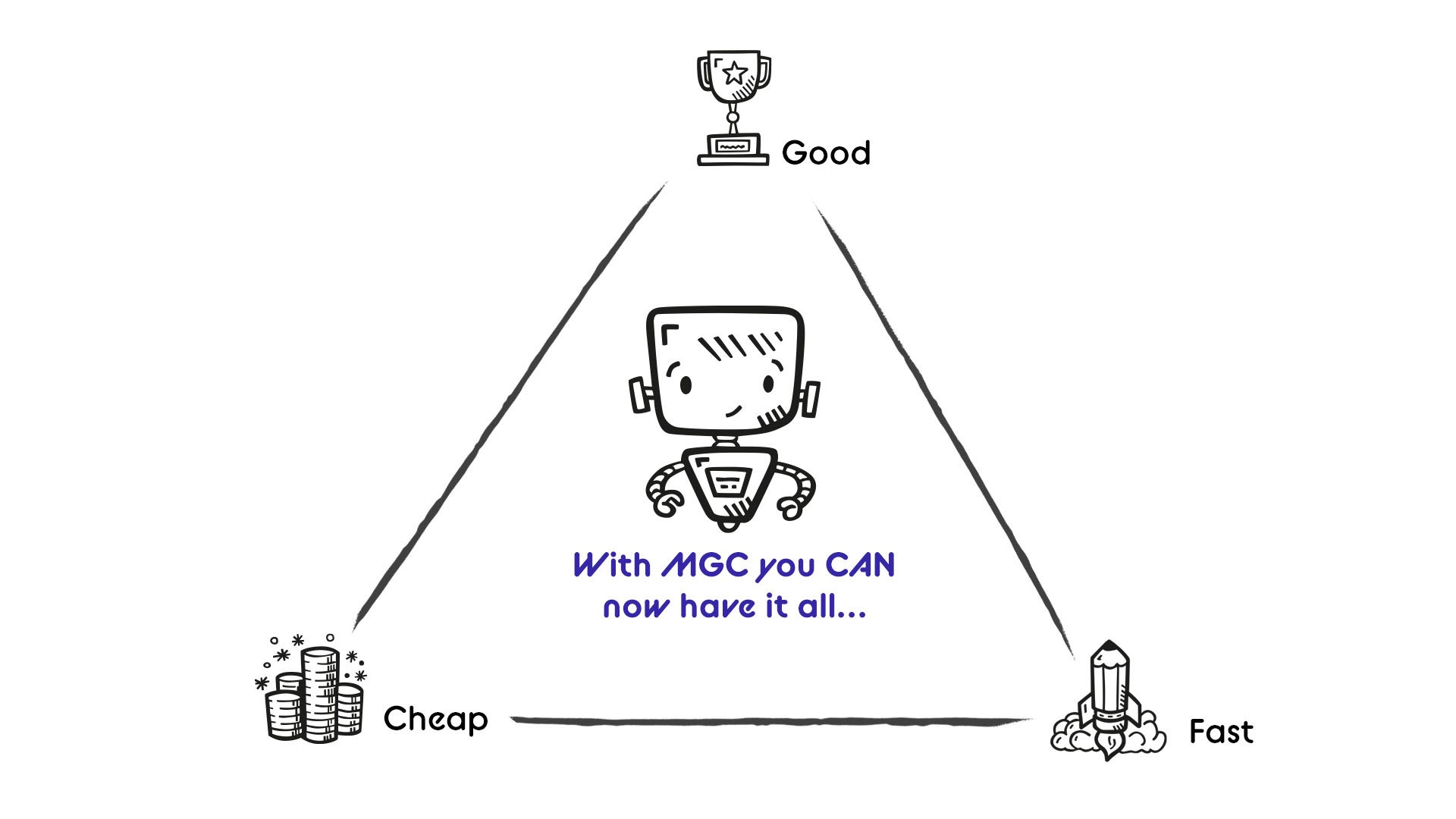
Here’s how…
这是如何做…
快速广告 (Fast ads)
Speed in marketing drives competitive advantage.
营销速度提升了竞争优势。
DTC and e-commerce have become high-velocity contact sports, and whoever adapts first to changes in taste, price, weather, availability, public mood, or even how a Facebook bug impacts the auction, usually wins.
DTC和电子商务已成为高速接触运动,无论谁首先适应口味,价格,天气,可及性,公众情绪的变化,甚至Facebook错误如何影响拍卖,通常都会获胜。
I asked an experienced performance marketer to share his strongest lever for Facebook ROAS, and he surprised me. He drives performance by identifying high-performance SKUs and then uses them to generate acquisition or remarketing ads fast enough to satisfy demand.
我请一位经验丰富的绩效营销人员分享他对Facebook广告投资报酬率的最强杠杆,他让我感到惊讶。 他通过识别高性能SKU来提高绩效,然后使用它们来生成购买或再营销广告,以足够快的速度满足需求。
Most marketers, he said, aren’t close enough to the data, and don’t know which specific red sweater or new flavor has the potential to drive top-funnel acquisition with strong advertising behind it. The holy grail is turning daily sales data into video ads within 24 hours.
他说,大多数营销人员还没有足够的数据,也不知道哪种特定的红色毛衣或新口味有可能通过强大的广告来推动顶级渠道的收购。 圣杯正在24小时内将每日销售数据转换为视频广告。
FB itself has built dynamic, automated creative tools such as Dynamic Creative and Dynamic Ads to help cut friction and accelerate responsiveness, and both are worth testing.
FB本身已经建立了动态的,自动的创意工具,例如Dynamic Creative和Dynamic Ads,以帮助减少摩擦并加快响应速度,两者都值得测试。
Dynamic Creative performs basic early prototyping, and takes multiple ad formats such as images, videos, titles, descriptions, and CTAs, and can be a good way to get started. Dynamic Ads are good for an extensive product range to generate feed-based ads, or when there are daily or even hourly price fluctuations, such as hotels and airlines.
动态广告素材执行基本的早期原型制作,并采用多种广告格式,例如图像,视频,标题,说明和CTA,并且是入门的好方法。 动态广告适用于广泛的产品范围,以生成基于Feed的广告,或者在每天甚至每小时的价格波动时(例如酒店和航空公司)。
Dynamic campaigns also target prospects down the funnel who’ve browsed product pages or shown intent based on pixel events, ideal for abandoned cart promotions or cross-selling. Ads “follow” users with the products or offers they’ve expressed an interest in.
动态广告系列还会将潜在客户定位到浏览过产品页面或根据像素事件显示意图的渠道下方的潜在客户,非常适合放弃购物车促销或交叉销售。 广告将用户感兴趣的产品或报价“跟进”用户。
FB’s dynamic ad creation tools will continue to evolve and give stronger insights to advertisers. But to hit top funnel CPA targets at scale you will still need to rapidly generate a variety of high-quality, on-brand Facebook and Instagram ads. MGC is best for that, through its combination of ML pattern recognition, high-fidelity signal feedback, and sheer computing power.
FB的动态广告制作工具将继续发展,并为广告客户提供更深刻的见解。 但是,要大规模达到最高的渠道每次转化费用目标,您仍然需要快速生成各种高质量的品牌Facebook和Instagram广告。 通过将ML模式识别,高保真信号反馈和强大的计算能力相结合,MGC对此是最好的。
If we look under the hood of a language AI, there’s a lot going on. It’s built on GPT-2, but it layers on task-specific fine-tuning as a post-processing step, with multiple custom models filtering downstream stages to qualify what is good from a user perspective. Finally, there’s a custom scoring pipeline which ranks the generated text in order of quality.
如果我们看一下语言AI的幕后知识,那么将会发生很多事情。 它建立在GPT-2之上,但它作为后处理步骤在特定于任务的微调上分层,并具有多个自定义模型,这些模型过滤了下游阶段,从而从用户的角度确定了什么是好的。 最后,有一个自定义评分管道,该管道按质量顺序对生成的文本进行排名。
But for users, MGC should feel simple and magical. After setting up a brand and messaging kit, and dropping in existing film or image assets, MGC can generate dozens of high-quality ads in minutes, ranked by expected outcomes.
但是对于用户而言,MGC应该感到简单而神奇。 设置好品牌和消息传递工具包并放下现有的电影或图像资产后,MGC可以在几分钟内生成数十个高质量广告,并按预期结果进行排名。
It can review, edit, and push final ads directly into a Facebook account to start testing.
它可以查看,编辑最终广告并将其直接推送到Facebook帐户中,以开始测试。
Soon it will all take seconds, and feed-based integrations will further automate the workflow. This new speed is enabled by code, but it’s only made possible by removing humans from the process.
很快,这将花费数秒钟,基于提要的集成将进一步使工作流程自动化。 此新速度由代码启用,但只有通过从流程中删除人员才能实现。
好广告 (Good ads)
Beauty is in the eye of the beholder, so humans will naturally disagree on a “good-looking” ad. But a good DR ad very clearly means hitting CPA and ROAS targets at scale.
情人眼中的美丽,因此人们自然会不同意“好看”的广告。 但是,好的DR广告显然很明显地达到了CPA和ROAS目标。
“Good” creative can also mean unlocking a new growth audience, which then serves as a seed to iterate new batches. MGC platforms like Pencil find good ads by generating meaningful variation at scale, and then testing to find the winners.
“好的”创意还可能意味着释放新的增长受众,然后作为迭代新批次的种子。 诸如Pencil之类的MGC平台可以通过产生有意义的规模变化,然后进行测试以找到赢家来找到好的广告。
In a sense they are first tested and ranked in vitro using AI, and then they are tested in vivo when the campaigns are launched and get responses from real potential customers. It’s evolution-by-natural-selection, on steroids.
从某种意义上说,首先要使用AI对它们进行测试并在体外进行排名,然后在启动活动并获得真正的潜在客户的响应时对其进行体内测试。 它是对类固醇的自然选择进化。
Whereas human designers unconsciously “predict” and then manually create their “best ad’’, a machine optimizes for meaningful variation, generating a portfolio of different approaches.
人类设计师会在不知不觉中“预测”然后手动创建“最佳广告”的情况,而一台机器会针对有意义的变化进行优化,从而生成各种方法的组合。
Whereas human marketers give tight briefs for specific assets, and then judge which ones might work, ML learns the rules for itself, through fast, small-scale testing of diverse portfolios of ads, followed by iteration.
人工营销人员会详细介绍特定资产,然后判断哪些资产可能会起作用,而ML则通过对各种广告组合进行快速,小规模的测试,然后进行迭代,来了解自身的规则。
MGC forces users to go beyond incremental optimization (e.g. Google famously testing different shades of blue for its search ads) and encourages more substantial innovation, by proposing very different ad structures.
MGC迫使用户超越渐进式优化(例如,著名的Google测试其搜索广告的深浅不同的蓝色),并通过提出截然不同的广告结构来鼓励进行更多实质性的创新。
Be wary of anyone who claims to know “the rules,” because beyond a few, general macro rules, the important micro rules will be different for each brand and audience, and will require ML to find them quantitatively.
警惕任何声称知道“规则”的人,因为除了少数通用宏规则之外,重要的微观规则对于每个品牌和受众都会有所不同,并且需要ML进行定量查找。
Importantly, this specific AI approach only works well within an integrated platform which builds the ads from labeled, atomic parts, and gets a strong, fast, signal back. Only then can it generate and predict outcomes accurately.
重要的是,这种特定的AI方法只能在集成平台中很好地工作,该平台可以从标记的原子部分构建广告,并获得强大,快速的信号回馈。 只有这样,它才能准确地生成和预测结果。
Already after initial testing with Pencil, we can see some distinctive techniques work for specific brands, such as
在使用Pencil进行初步测试之后,我们可以看到一些针对特定品牌的独特技术,例如
- Clear product shots and descriptions in large captions in the first seconds 在头几秒钟内以大标题清除产品图片和说明
- Persistent product bottom-bars with logo and integrated CTA button 永久性产品底栏,带有徽标和集成的CTA按钮
- Juxtaposing literal and abstract headlines 文字和抽象标题并列
Understandably, marketers fear that robots will create robotic ads.
可以理解,营销人员担心机器人会创建机器人广告。
But learning machines can be made to create originally in a number of ways. They can be trained for one set of outcomes, producing unexpected results in another. They can be trained on one set of inputs, and then provided another. Noise and randomness can be introduced into their processes.
但是可以通过多种方式使学习机器最初创建。 可以对他们进行一组结果的培训,而在另一组结果中产生出乎意料的结果。 可以在一组输入上对它们进行培训,然后再提供另一组。 噪声和随机性可以引入其过程。
便宜的广告 (Cheap ads)
MGC can already beat humans on cost, and this trend will accelerate. Like most self-serve tools, as volume scales up, the cost per ad comes down.
MGC已经可以在成本上击败人类,并且这种趋势将会加速。 像大多数自助服务工具一样,随着数量的增加,每个广告的成本也会下降。
First there are the direct cost savings of designer salaries or fees.
首先,可以直接节省设计师薪金或费用。
Second, there are indirect cost savings from fewer manager briefs and reviews, and from data analysis being mostly integrated and automated.
其次, 通过减少经理摘要和评论以及主要是集成和自动化的数据分析,可以间接节省成本 。
Third, creative production costs will drop because it’s simpler to collect small batches of high-quality clips and images for MGC, than it is to storyboard, shoot and edit a scripted film.
第三, 创意制作成本将降低,因为为MGC收集小批量的高质量剪辑和图像要比情节提要,拍摄和剪辑电影更简单。
Fourth, you can sweat your existing creative assets more efficiently. Take that $200k film you shot on the Amalfi Coast three summers ago, and see how the MGC repurposes it for your next campaign.
第四,您可以更有效地利用现有的创意资产 。 拿三个夏天前在阿马尔菲海岸拍摄的那部价值20万美元的电影,看看MGC如何将其用于下一次竞选。
I believe that Humans + Machines = Superhumans, and I’m excited to see what the world’s top creative minds will create with an MGC in their hands.
我相信人类+机器=超人类,我很高兴看到世界顶级创意人才将掌握MGC,将创造出什么。
I’m also hoping to see strong creatives earn their true value.
我也希望看到有实力的广告人能获得真正的价值。
Today it’s rare to find designers who deeply understand DR creative and can generate high-quality ads on their own. They are in hot demand, and can earn six figures, at least in the US. Using MGC, the best designers will soon be leveraged and be able to 10X their creative output.
如今,很难找到能够深刻理解DR创意并能够自行生成高质量广告的设计师。 他们需求旺盛,至少在美国可以赚到六位数。 使用MGC,最好的设计师将很快得到利用,并能够将其创意输出提高10倍。
Many years ago I sat in an office in Singapore with the legendary copywriter and adman Neil French, while he negotiated a seven-figure salary with Sir Martin Sorrell. I was in awe at the amount at the time.
许多年前,我和传奇的撰稿人,广告人尼尔·法文(Neil French)坐在新加坡的办公室里,而他与马丁·索雷尔爵士(Sir Martin Sorrell)商定了七位数的薪水。 当时我对这种数量感到敬畏。
But I predict that the best designers will earn even more in the future, by leveraging their experience and talent using AI.
但是我预测,最好的设计师将通过利用AI的经验和才华,在未来获得更多收益。
“Give me a lever and a place to stand, and I will move the earth,” said Archimedes, over two thousand years ago.
两千多年前的阿基米德说:“给我一个杠杆和一个站立的地方,我将移动地球。”
第3章:广告商如何开始 (Chapter 3: How Advertisers Can Start Now)
There will be a short window to use MGC to crack the Creative AI code first for each category. It’s simple to get started, and there will be immediate performance gains, but the real prize will be for the brands that give it sufficient iteration waves for the ML to start cracking their rules.
将有一个短暂的窗口,使用MGC首先为每个类别破解Creative AI代码。 这很容易上手,并且会立即获得性能提升,但是真正的奖励将是那些给予ML足够反复迭代以使ML开始破解其规则的品牌。
On the surface, it won’t look like much has changed at first.
从表面上看,起初看起来并没有太大变化。
Your existing human ads delivered 1.8X ROAS. The new MGC ads also hit 1.8X ROAS. Faster and cheaper maybe, but no better, right?
您现有的人工广告带来了1.8倍的广告支出回报率。 新的MGC广告也达到了1.8倍的广告支出回报率。 也许更快更便宜,但没有更好,对吧?
But beneath the surface, a lot has changed. For every batch of ads the AI has generated, it has gathered deep, granular data and received high-fidelity FB signal back via the API connection.
但是在表面之下,发生了很多变化。 对于AI生成的每一批广告,它都收集了深入的细粒度数据,并通过API连接接收了高保真FB信号。
The ML will be closer to cracking the code and finding a key to unlocking sustainable performance future gains.
机器学习将更接近破解代码并找到释放可持续性能未来收益的关键。
Here’s how brands can start adapting now for an MGC future.
以下是品牌如何开始适应MGC未来的方式。
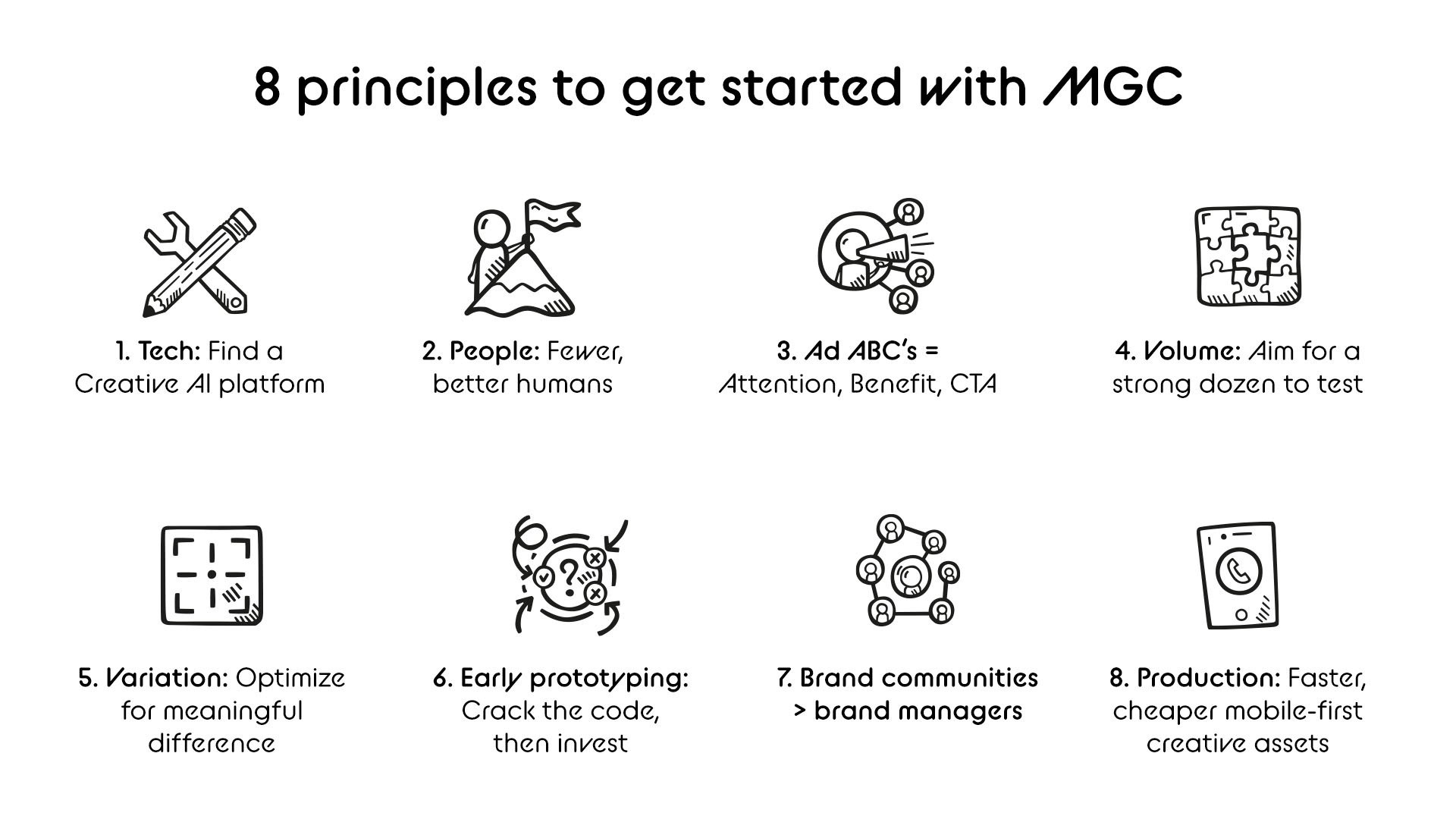
Tech: Find a Creative AI platform. There are a few already, but many more will emerge. Find one with a long roadmap runway, to go deep with.
技术:查找创意AI平台。 已经有一些,但还会出现更多。 找到一条路线图很长的路要走。
- Is it fast, good and cheap? 它快,好又便宜吗?
- Can you see the output ads? 您可以看到输出广告吗?
- Is it optimized for the platform where you invest the most? 是否针对您投资最多的平台进行了优化?
- Does the team have a proven track-record building AI and ads that deliver results? 团队是否拥有久经考验的建立记录的AI和可带来效果的广告?
Ask tough technical questions and focus on where the roadmap is heading, not just what it can do today.
提出棘手的技术问题,并关注路线图的发展方向,而不仅仅是今天的功能。
2. People: Fewer, better humans. Try to keep humans out of the loop. Don’t create a review committee to slow everything down. Empower somebody directly responsible for campaign performance with a self-serve tool. Consolidate decision making. Some of the best growth teams I’ve seen work in triads: a marketer, a designer, and an analyst. Perhaps this will collapse further to just two or even one superhuman.
2.人:更少,更好的人。 尽量避免人类陷入困境。 不要建立审查委员会来减慢一切。 使用自助服务工具授权直接负责竞选活动的人员。 巩固决策。 我见过的一些最好的成长团队在三合会上工作:营销人员,设计师和分析师。 也许这会进一步崩溃,只有两个甚至一个超人。
3. Ad ABC’s = Attention, Benefit, CTA. Most top-performing ads broadly follow this ABC formula. And so should at least some of your MGC output ads, so that you can pack your first tests with strong contenders.
3.广告ABC =注意,收益,CTA。 大多数效果最好的广告大致都遵循此ABC公式。 因此,至少您的某些MGC输出广告也应该如此,以便您可以将首批测试与强大的竞争者捆绑在一起。
- Attention — ABC ads grab attention in the first second, through a thumb-stopping hook message and/or visual. The more limbic the better: fear, sex, food, money triggers all stop thumb-scrollers in their feed. 注意力-ABC广告在第一秒通过引人注目的钩子消息和/或视觉效果吸引了注意力。 边缘性越强越好:恐惧,性别,食物,金钱触发所有停止拇指滚动的饲料。
- Benefit — Then they communicate the benefit, functional and/or emotional, often with social proof testimonials or reviews. Adding an RTB (Reason to buy/believe) often helps. 收益-然后,他们通常会通过社会证明书或评论来传达功能和/或情感上的收益。 添加实时出价(购买/相信原因)通常会有所帮助。
- CTA — Finally they close with a call-to-action, often time-bound (“40% off before Memorial Day”) and language that matches the button selected for each ad. (“Shop now,” “Sign up”) CTA-最后,它们以号召性用语结束,号召性用语通常是有时间限制的(“阵亡将士纪念日前折扣40%”),并且语言与为每个广告选择的按钮匹配。 (“立即购买”,“注册”)
4. Volume: Aim for a strong dozen to test. Stop hunting for one sure-thing great ad, you’ll never predict it accurately. Aim for a strong dozen to run in a test account, and keep the audience targeting as broad as possible, to let FB do the hard work getting each ad in front of the right prospects. Campaign Budget Optimization (CBO) might help here, especially for popular categories where a lot of previous buyer-signal exists, but it doesn’t work in all cases. Many experts prefer to guarantee minimum delivery against each test ad, so CBO doesn’t kill a “golden goose” ad too early. When ads are working in test accounts, study the buyer profile and comments to find new language and insights, and promote winners into scaled accounts until they fatigue. By then, you should have iterated new strong ads to replace fading stars, possibly from a similar DNA.
4.数量:力争测试。 停止搜寻一个肯定的好广告,您将永远无法准确预测它。 力争在测试帐户中投放大量广告,并尽可能扩大受众群体的定位范围,以使FB尽力而为,使每个广告都定位在正确的潜在客户面前。 广告系列预算优化(CBO)在这里可能会有所帮助,特别是对于存在很多先前购买者信号的热门类别而言,但并非在所有情况下都有效。 许多专家更喜欢保证每个测试广告的最低投放量,因此CBO不会过早杀死“金鹅”广告。 广告在测试帐户中运作时,请研究买方资料和评论,以找到新的语言和见解,并将获胜者推广到规模较大的帐户中,直到疲倦为止。 到那时,您应该已经迭代了新的强广告来替换可能来自相似DNA的衰落恒星。
5. Variation: Optimize for meaningful difference. Being irrelevant on Facebook can be very expensive, so keep trying to use assets and language likely to be meaningful for your target audience. Use the power of variety to find and unlock new audiences. Don’t assume videos or “stills” (single image ads) will work better; it varies ~50/50 by brand, by level of the funnel, and by how the Facebook algorithm is behaving that week. Keep an open mind. Or more precisely, use Creative AI programmed to keep an open mind!
5.差异:针对有意义的差异进行优化。 在Facebook上不相关可能会非常昂贵,因此请继续尝试使用可能对您的目标受众有意义的资产和语言。 利用多样化的力量找到并释放新的受众群体。 不要以为视频或“静态图片”(单个图片广告)会更好。 根据品牌,渠道的级别以及该周Facebook算法的运行情况,它的变化约为50/50。 保持开放的心态。 更准确地说,使用编程的Creative AI保持开放的态度!
6. Early prototyping: Crack the code, then invest. Some marketers plan to use Creative AI to prototype ads early in the creative process. Use existing assets and AI to find the language and visual hooks that connect with audiences, and then let design teams translate these into longer, more polished final films, perhaps with higher production values. For instance, incubate ads on Facebook, and then invest behind a more expensive 30-second TV Ad to hit broader reach and frequency targets. How long before we see a $5m Superbowl spot born in MGC?
6.早期原型制作:破解代码,然后进行投资。 一些营销人员计划在创意过程的早期使用Creative AI来制作广告原型。 使用现有资产和AI查找与观众连接的语言和视觉挂钩,然后让设计团队将它们翻译成更长,更精美的最终电影,也许具有更高的生产价值。 例如,在Facebook上孵化广告,然后投资更昂贵的30秒电视广告来达到更广泛的覆盖范围和频率目标。 我们要等多久才能看到在MGC诞生500万美元的超级碗?
7. Brand communities > brand managers. A good Creative AI tool lets you input all your logos, fonts and colors, and drop in your own assets. But brand managers are still often concerned about giving up style control to a machine. They don’t need to worry, as long as a human review happens before campaigns are launched, and “off-brand” ads can be rejected or edited. The evidence suggests that consumers are now more attracted by brand communities, where they also have a voice. In truth, unless you are managing a brand like Apple, brands today are a lot less consistent than their brand ‘managers’ think they are and must adapt natively, especially on social platforms. Brand teams are often overly fixated on storytelling, but I haven’t seen that work well on short-form platforms like Facebook.
7.品牌社区>品牌经理。 出色的Creative AI工具可让您输入所有徽标,字体和颜色,并放入自己的资产。 但是品牌经理仍然经常担心放弃对机器的样式控制。 他们无需担心,只要在启动广告系列之前进行人工审核即可,并且可以拒绝或修改“非品牌”广告。 有证据表明,消费者现在更受品牌社区的吸引,他们在那里也有发言权。 实际上,除非您管理的是苹果这样的品牌,否则今天的品牌要比其品牌“经理”所认为的一致程度要差很多,而且必须在本地进行适应,尤其是在社交平台上。 品牌团队通常过于讲故事,但我还没有看到它在Facebook等简短平台上能很好地发挥作用。
8. Production: Faster, cheaper mobile-first creative assets. Creative production costs will drop overall because it’s far simpler to collect small batches of high-quality clips and images for MGC, than to storyboard, shoot and edit scripted films. Creative AI users will rethink production, likely shooting a wider variety of shorter, higher-quality assets, and a mix of UGC, film, stop-motion, and even animation. In short, shoot it all, throw it into the sausage-maker, then review and edit.
8.生产: 更快,更便宜的移动优先创意资产。 创意制作成本将总体下降,因为为MGC收集小批量的高质量剪辑和图像要比情节提要,拍摄和编辑脚本电影容易得多。 富有创造力的AI用户将重新考虑生产,可能会拍摄更多种类的较短,更高质量的资产,并将UGC,电影,定格动画甚至动画混合在一起。 简而言之,将其全部拍摄,放入香肠机中,然后进行检查和编辑。
摘要:使用MGC达成目标 (Summary: Use MGC To Hit Your Goals)
Machines can already beat humans at Chess and Go. Soon, generating Facebook ads without MGC will be like turning up to a gunfight with a knife: brave, but somewhat suicidal.
在国际象棋和围棋中,机器已经可以击败人类。 很快,在没有MGC的情况下生成Facebook广告就好比用刀进行枪战:勇敢,但有些自杀。
Once you get started, playing with AI is magical.
一旦开始,玩AI就是神奇的事情。
For example, Pencil’s language AI is designed so the user simply inputs two words or phrases, and effortlessly generates dozens of fresh headlines.
例如,Pencil的语言AI的设计使用户只需输入两个单词或短语,就可以毫不费力地生成许多新标题。
In one test, the French beauty retailer Sephora input “makeup” and “food,” and Pencil’s AI generated “Eye candy,” “Hungry for looks,” and “A feast on the eyes.” All these lines generated CTRs above the human control copy-line.
在一项测试中,法国美容零售商丝芙兰(Sephora)输入了“化妆品”和“食物”,而Pencil的AI生成了“眼睛糖果”,“渴望外表”和“大饱眼福”。 所有这些行都在人工复制行上方生成了点击率。
In this essay, we’ve explored why humans often fail at generating Facebook ads, why machines will do it better, and how advertisers can start using MGC now.
在本文中,我们探讨了为什么人们经常无法生成Facebook广告,为什么机器会做得更好,以及广告商现在如何开始使用MGC。
To explore running an MGC test for your brand on a live campaign, join the Pencil waitlist or contact me on LinkedIn and tell me about your brand or agency.
要探索在实时广告系列中为您的品牌进行MGC测试,请加入Pencil候补清单 或 在LinkedIn上 与我 联系, 并向我介绍您的品牌或代理商。
To dive deeper into MGC, start with this great resource, Algorithm-Driven Design: How Artificial Intelligence is Changing Design, compiled by Yury Vetrov.
要更深入地研究MGC,请从Yury Vetrov编译的大量资源“ 算法驱动设计:人工智能如何改变设计”开始 。
翻译自: https://medium.com/better-marketing/how-to-create-facebook-ads-with-ai-in-8-steps-6aa83d9887ea