ai人工智能收入
介绍 (Introduction)
Pricing optimization is a powerful lever for revenue growth, yet it’s too often put in the too-hard basket by too many companies.
定价优化是实现收入增长的强大杠杆,但太多公司经常将定价置于过于艰难的境地。
This is because traditional pricing optimization methods can be both complex to implement and limited in their ability to accurately capture the full range of factors that can impact pricing.
这是因为传统的定价优化方法实施起来可能既复杂,又无法准确地捕获可能影响定价的所有因素。
Machine learning (ML) is well-suited to pricing optimization problems — both for its ability to handle complex features, as well as its ability to generalize to new situations. Moreover, recent advances in managed services has put these ML solutions within reach of virtually any organization.
机器学习(ML)非常适合定价优化问题-既具有处理复杂功能的能力,又具有将其推广到新情况的能力。 此外,托管服务的最新进展使这些ML解决方案几乎可以在任何组织中获得。
In this anonymized example we explore how a company with no data science expertise was able to use managed ML services to implement an ML-powered pricing strategy that performed 2x above traditional approaches and resulted in estimated revenue growth of 11%.
在这个匿名的示例中,我们探索了一家没有数据科学专业知识的公司如何能够使用托管的机器学习服务来实施以机器学习为动力的定价策略,该策略的执行效率是传统方法的2倍,并且估计收入增长了11%。
情况 (Situation)
FitCo is a premium fitness brand, based in Los Angeles, that operates a portfolio of over 600 gym and fitness center locations across the United States.
FitCo是一家总部位于洛杉矶的高级健身品牌,在美国拥有600多个健身房和健身中心。
Having grown rapidly by acquisition over the past several years, management attention had now turned its attention to boosting organic revenue growth, which had been stubbornly flat on a per-studio basis.
在过去几年中,通过并购获得了快速增长,现在,管理层的注意力已经转移到了促进自然收入增长上,而这种增长在每个工作室的基础上都持平。
FitCo had identified FitClass — its suite of specialty fitness classes — as a prime source of organic growth. Specifically, it had identified pricing of these classes as a major potential area of improvement.
FitCo已将FitClass(其专业健身课程套件)确定为有机增长的主要来源。 具体而言,它已将这些类别的定价确定为主要的潜在改进领域。
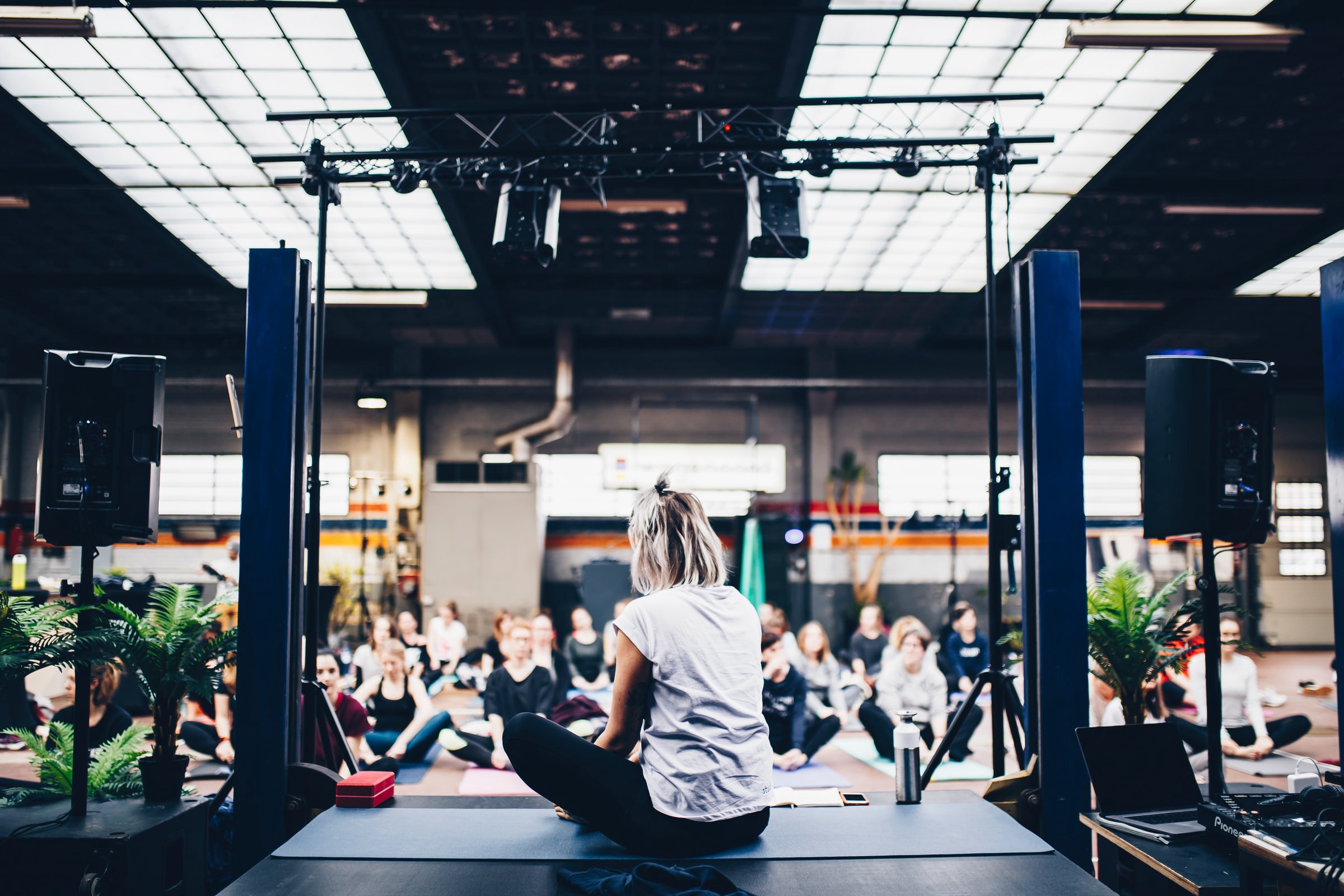
FitClasses are a popular offering across FitCo’s brands. They are premium experiences catering to niche fitness demand and sold on a pay-per-class basis on top of standard memberships.
FitClasses是FitCo各品牌的热门产品。 它们是满足小众健身需求的优质体验,并在按标准付费的基础上按等级收费销售。
Whilst FitCo had ensured a consistent user experience across its portfolio, local operators were still able to set schedules and prices for FitClasses in their studios with nearly total independence. As a result, prices varied widely between classes and locations.
尽管FitCo在其产品组合中确保了一致的用户体验,但本地运营商仍能够在几乎完全独立的情况下在其工作室中为FitClasses设置时间表和价格。 结果,价格在班级和地点之间差异很大。
Whilst FitCo understood that some of this variance reflected local conditions, they also suspected there was considerable room for improvement in the way prices were set across its portfolio.
FitCo理解这种差异反映了当地情况,但他们也怀疑整个投资组合的定价方式仍有很大的改进空间。
并发症 (Complication)
FitCo had undertaken a pricing exercise two years ago in which prior management had opted to centralize FitClass pricing and institute a blanket price increase of between 10% and 20% across the board.
FitCo在两年前进行了一次定价活动,先前的管理层选择集中化FitClass定价,并全面实行10%至20%的全面价格上调。
This blunt approach had not been successful. It had failed to take into account the price elasticity of demand of its customer set across the wide range of classes and locations, and the price increases actually resulted in overall revenue declines of 2% as the subsequent reduction in demand in many classes outstripped the increases in price. They were forced to unwind the price changes a couple months later.
这种直率的方法没有成功。 它没有考虑到不同类别和地区的客户需求的价格弹性,价格上涨实际上导致总收入下降了2%,因为随后许多类别的需求下降超过了涨幅价格。 几个月后,他们被迫取消价格变动。
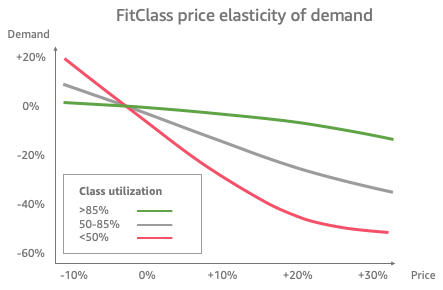
Though painful, that experience had at least been useful in giving FitCo a pretty solid dataset on price elasticity of its FitClass customer set. It could chart how class demand changed in response to price increases for each of three utilization bands — high (>85%), medium (50–85%) and low (<50%). Modeling out the various impacts of price increases on demand, FitCo estimated a revenue full potential of 15% from more efficient pricing.
尽管很痛苦,但这种经验至少在为FitCo提供有关其FitClass客户集的价格弹性的相当可靠的数据集方面很有用。 它可以绘制出三个使用频段(高(> 85%),中(50-85%)和低(<50%))中的每个使用频段随价格上涨而变化的图表。 通过模拟价格上涨对需求的各种影响,FitCo估计,更有效的定价可带来15%的全部收入潜力。
To capture that higher revenue potential, FitCo now only needed to be able to accurately predict demand for classes into the future — whether current or new — to accurately model price impacts on revenue. This would enable FitCo to determine whether and how much of a price increase each class could profitably sustain.
为了捕获更高的收入潜力,FitCo现在仅需要能够准确预测对未来类别的需求(无论是当前的还是新的),以准确地模拟价格对收入的影响。 这将使FitCo能够确定每个类别的价格上涨是否以及能够维持多少利润。
FitCo had initially attempted this using traditional rules-based methods — effectively a series of if/then statements to set bands based on certain conditions. With some extensive trial and error they had managed to write a function that was estimated to generate about 5% in additional revenue. This wasn’t bad, but this approach had two primary limitations: (1) it failed to fully account for interrelationship of the wide variety of factors relevant to each class — it incorrectly predicted too many classes in the wrong band, causing a decline in usage — and (2) it failed to generalize to new class schedules or details at any given location — it couldn’t adequately account for new combinations of factors or scenarios.
FitCo最初使用传统的基于规则的方法进行了尝试,实际上是通过一系列if / then语句来基于某些条件设置频段。 经过大量的反复试验,他们设法编写了一个函数,该函数估计可产生约5%的额外收入。 这样做还不错,但是这种方法有两个主要局限性:(1)它未能完全说明与每个班级相关的多种因素之间的相互关系–错误地预测了错误频段中的过多班级,从而导致用法-(2)无法在任何给定位置推广到新的课程表或详细信息-无法充分考虑因素或场景的新组合。
Looking for alternative approaches, FitCo turned to ML.
在寻找替代方法时,FitCo转向了ML。
机器学习解决方案 (ML solution)
ML is well-suited to these types of classification problems precisely because of its ability to process a wide range of factors and generalize to unseen or new situations.
ML非常适合这些类型的分类问题,这是因为ML能够处理各种因素并将其推广到看不见的情况或新情况。
However, like most organizations of its size and in its industry, FitCo did not have ML capabilities or a team of data scientists on hand to design, build and deploy an ML solution. This had previously been a major barrier to ML adoption. Today though, the availability of ML managed services have largely democratized access to ML capabilities.
但是,像大多数同等规模和其行业的组织一样,FitCo没有ML功能,也没有一组数据科学家来设计,构建和部署ML解决方案。 以前,这是采用机器学习的主要障碍。 但是,如今,ML管理服务的可用性已大大普及了ML功能的访问。
For their solution, FitCo chose Amazon SageMaker, which featured among other things, an AutoML capability called AutoPilot that could take a simple tabular dataset and automate the process of building an ML workload around it.
对于他们的解决方案,FitCo选择了Amazon SageMaker,该产品除其他功能外还具有一项称为AutoPilot的AutoML功能,该功能可以获取简单的表格数据集并自动围绕其构建ML工作负载的过程。
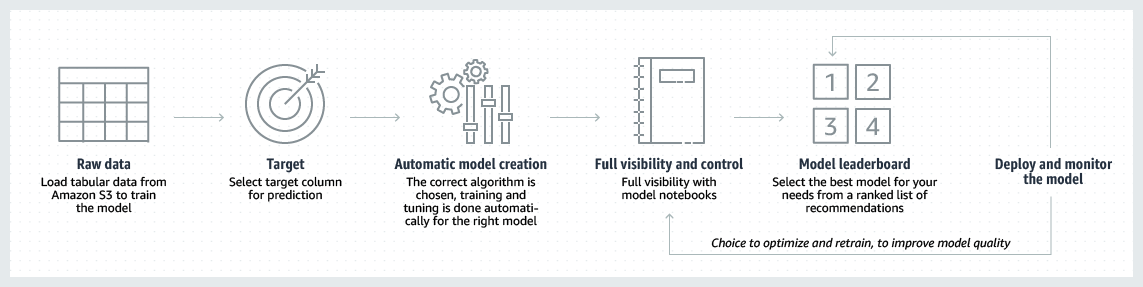
With AutoPilot, FitCo no longer needed a team of data scientists to get the benefit of ML. Instead, they were able to drive this initiative with a three-person project team consisting of the CFO as business owner, CTO as technology owner, and a single back-end developer responsible for building and integrating the solution.
有了AutoPilot ,FitCo不再需要一个数据科学家团队来获得ML的好处。 取而代之的是,他们能够由三人组成的项目团队来推动这一计划,该团队由CFO(业务所有者),CTO(技术所有者)以及负责构建和集成解决方案的单个后端开发人员组成。
训练数据 (Training data)
To build their training dataset, FitCo gathered historical utilization data for each of their classes over the past two years.
为了建立他们的训练数据集,FitCo收集了过去两年中每个班级的历史利用率数据。
Utilization for each class was expressed as a percentage of total places filled. FitCo converted the data in this column into ‘high’ , ‘medium’ and ‘low’ based on the utilization bands above and named this column ‘target’. This would be the column to be predicted by the ML model.
每个班级的利用率表示为所占总席位的百分比。 FitCo根据上面的使用范围将此列中的数据转换为“高”,“中”和“低”,并将此列命名为“目标”。 这将是ML模型要预测的列。
They then combined this data with a set of internal features they thought likely to indicate utilization. They also added a range of external data that they expected to be relevant. The result was a dataset with 800K instances and which contained the following features:
然后,他们将此数据与他们认为可能表明利用率的一组内部功能结合在一起。 他们还添加了他们期望相关的一系列外部数据。 结果是一个包含800K实例的数据集,其中包含以下功能:
- type of class (categorical) 类的类型(分类)
- location (categorical) 位置(类别)
- day of week (categorical) 星期几(分类)
- time of day (numerical) 一天中的时间(数字)
- instructor (categorical) 指导老师(分类)
- studio brand (categorical) 工作室品牌(分类)
- is a public holiday (binary) 是一个公共假期(二进制)
- is a school holiday (binary) 是学校假期(二进制)
- external temperature (numerical) 外部温度(数值)
- target (categorical) 目标(绝对)
FitCo did some basic feature engineering to better organize and format this data set, converted it to csv format and saved in an S3 bucket. They now had a dataset with which they could train their ML model.
FitCo进行了一些基本的功能工程设计,以更好地组织和格式化此数据集,将其转换为csv格式并保存在S3存储桶中。 他们现在有了一个可以训练其ML模型的数据集。
亚马逊SageMaker AutoPilot (Amazon SageMaker AutoPilot)
FitCo chose Autopilot for its ability to simplify and streamline the core components of the machine learning process. AutoPilot automates the process of exploring data, engineering features, testing different algorithms, and selecting the best model. All it requires is that you provide a tabular dataset.
FitCo之所以选择自动驾驶仪,是因为它能够简化和简化机器学习过程的核心组件。 AutoPilot自动执行探索数据,工程特征,测试不同算法以及选择最佳模型的过程。 它所需要的只是提供表格数据集。
In addition, it automatically surfaces the code base it used, adding visibility and reproducibility into the process. This was an important differentiator for FitCo’s CTO because it gave FitCo the opportunity to explore, and learn from, the steps that had been taken to generate the model, as well as giving it a code base that it could modify and optimize it into the future.
此外,它会自动显示所使用的代码库,从而在过程中增加可见性和可重复性。 这是FitCo CTO的重要区别因素,因为它使FitCo有机会探索和学习生成模型所采取的步骤,并为其提供了可以在未来进行修改和优化的代码库。 。
To start the AutoPilot process, FitCo used the no-code interface available within Amazon SageMaker Studio. This required three key steps:
为了启动AutoPilot流程,FitCo使用了Amazon SageMaker Studio中可用的无代码界面。 这需要三个关键步骤:
- Name their experiment 为实验命名
- Point SageMaker to the s3 bucket where their training file was located, and 将SageMaker指向其训练文件所在的s3存储桶,然后
- Define the variable to be predicted 定义要预测的变量
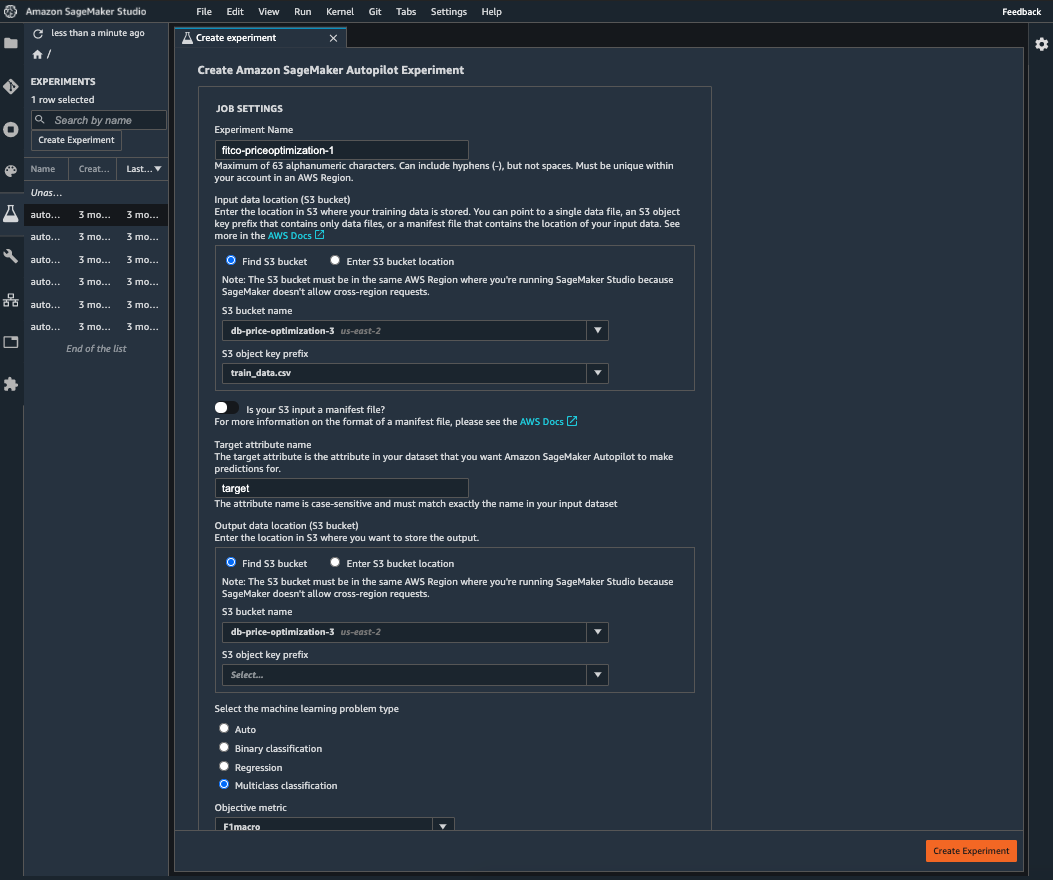
Once these details were entered, they simply hit Create Experiment and FitCo’s ML model build was underway, running a range of trials to determine the best performing ML approach.
输入这些详细信息后,他们只需点击“创建实验”,FitCo的ML模型构建便开始了,并进行了一系列试验,以确定性能最佳的ML方法。
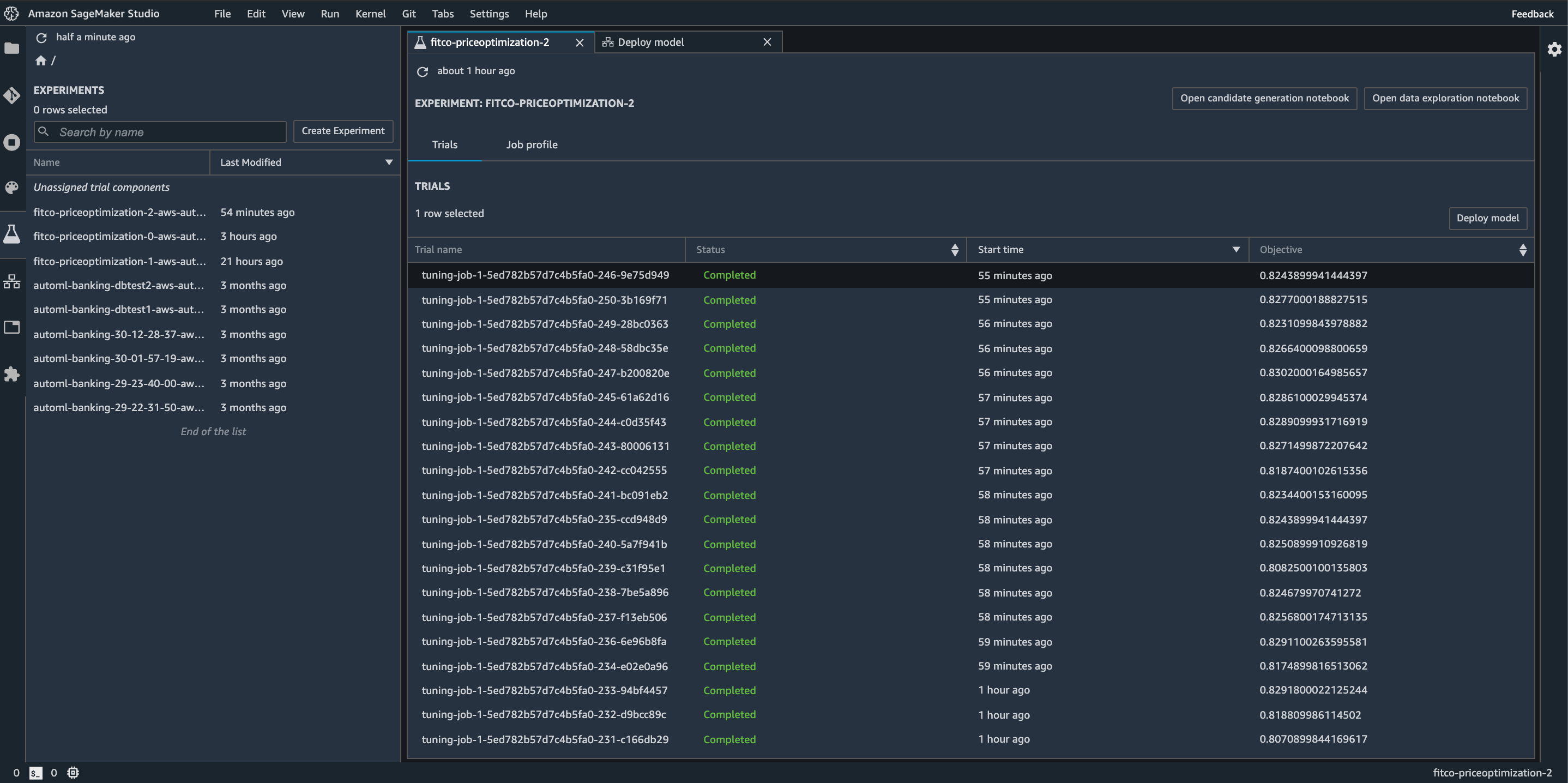
This process took about an hour to complete. Once it had concluded, FitCo could then simply sort the list of trials to find the best performing model. They were able to generate the notebook that contained the code of this model, and they were also able to deploy the model to a SageMaker endpoint that enabled them to further test the inferences (predictions) generated by the model on new data, or even put it into production.
此过程耗时约一个小时。 一旦得出结论,FitCo就可以简单地对试验清单进行排序,以找到性能最佳的模型。 他们能够生成包含该模型代码的笔记本,还能够将模型部署到SageMaker端点,这使他们能够进一步测试该模型对新数据生成的推论(预测),甚至可以将其放入它进入生产。
结果 (Results)
When modeled against FitCo’s test dataset, the ML model outperformed their rules-based approach by 2x, increasing revenue by an estimated 11% overall.
如果以FitCo的测试数据集为模型进行建模,则ML模型的性能要比其基于规则的方法高出2倍,从而使整体收入增长约11%。
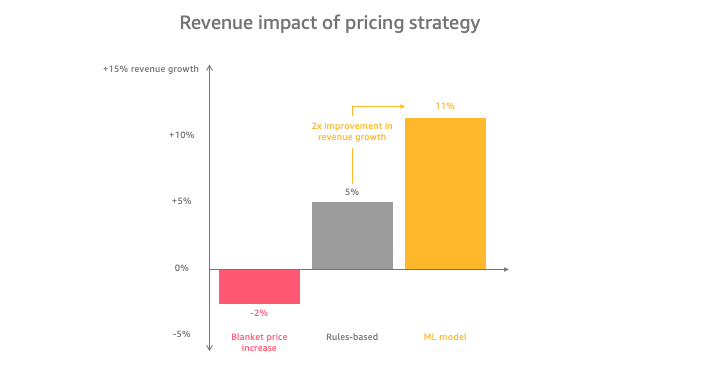
This performance improvement stemmed primarily from the higher precision of the ML predictions vs the rules-based approach. In a multi-class classification problem, the challenge is not only to predict the right class, but to minimize the cost of inaccuracies. For example, it is less costly to inaccurately predict a low demand class as ‘medium’ than as ‘high’. Specifically with FitCo’s price elasticity profile, the cost of that particular error was approximately 4x greater in the form of lower demand.
相对于基于规则的方法,这种性能改进主要来自ML预测的更高精确度。 在多类别分类问题中,挑战不仅在于预测正确的类别,而且在于最大程度地减少不准确性的成本。 例如,准确地将低需求类别预测为“中”比“高”类别的成本更低。 特别是在FitCo的价格弹性曲线中,以较低的需求形式出现的特定错误的成本大约高出4倍。
A comparison of this performance between traditional and ML approaches can be seen below, and illustrates that the traditional approach actually outperformed ML in accurately predicting high demand classes. The issue is that it was unable to do so while also accurately predicting medium and low demand classes. Moreover, it made costly errors in inaccurately predicting low classes as high demand classes. The ML model was better able to more holistically map the shape of the data to account for both situations.
传统方法和机器学习方法之间的性能比较如下所示,它说明了传统方法在准确预测高需求类别方面实际上胜过机器学习。 问题在于,它无法做到这一点,同时也无法准确预测中,低需求类别。 此外,它在错误地将低等级预测为高需求等级时造成了代价高昂的错误。 ML模型能够更好地从整体上映射数据的形状以解决这两种情况。
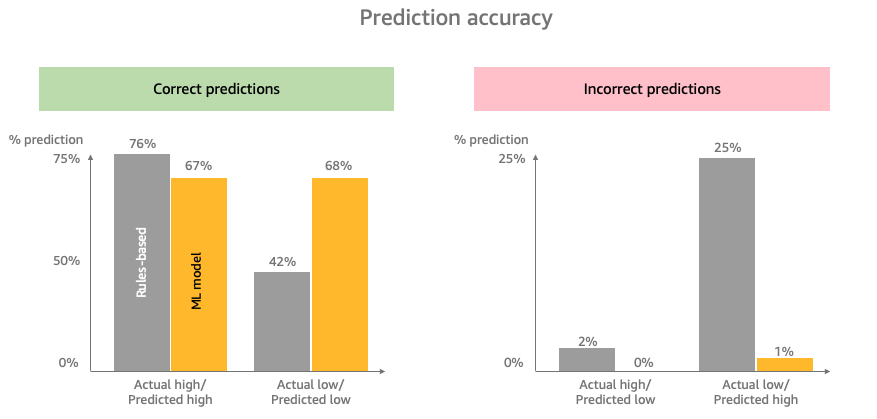
These errors came at a considerable cost to revenue for the rules-based approach that the ML model could avoid. For example, inaccurately predicting a low demand class as high demand resulted in a demand decline of nearly 50%, far offsetting the 30% higher price paid by the remaining members.
对于ML模型可以避免的基于规则的方法,这些错误要付出可观的收入成本。 例如,由于高需求导致需求下降了近50%,因此不准确地预测了低需求类别,从而远远抵消了其余成员支付的高出30%的价格。
The matrix below shows the revenue growth impact of each type of prediction, expressed as the difference in performance between traditional and ML methods.
下面的矩阵显示了每种预测类型对收入增长的影响,表示为传统方法与机器学习方法之间的性能差异。
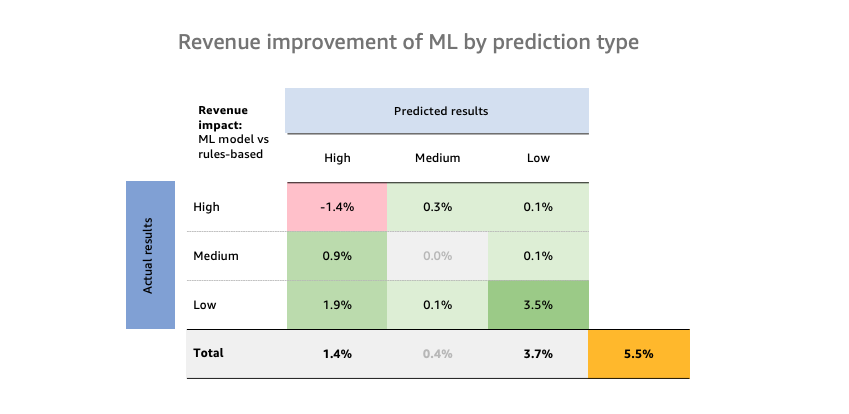
Though traditional methods managed to beat the ML model in correctly labeling a higher proportion of high classes as high (a metric known as ‘recall’), it did so by also inaccurately labeling many more ‘medium’ and ‘low’ demand classes as high too (known as ‘precision’).
尽管传统方法在正确地将较高比例的高等级标记为高(一种称为“召回”的指标)上成功击败了ML模型,但这样做的方法是不正确地将更多“中”和“低”需求等级标记为高也称为“精度”。
As a result, although its accurate high predictions generated 1.4% higher revenue, that came at a cost of losing 2.8% of revenue from the demand declines of incorrectly charging higher prices to higher elasticity medium and low classes. A similar pattern emerged in low predictions; the ML model’s greater accuracy meant that it only reduced the price of classes for which one would expect to see higher demand.
结果,尽管其准确的高预测产生了1.4%的收入增长,但其结果是由于需求下降而导致收入损失了2.8%,这是由于不正确地向较高弹性的中,低阶层收取了较高的价格。 低预测中也出现了类似的模式。 ML模型的准确性更高,这意味着它只降低了人们期望获得更高需求的类的价格。
As a result of this higher precision —the more accurate prediction of both high and low classes — the ML model was able to generate 5.5% higher revenue overall, more than double that of rules-based approaches.
由于具有更高的精确度(对高低阶层的预测都更加准确),因此ML模型总体上可产生5.5%的收入,是基于规则的方法的两倍多。
结论 (Conclusion)
Pricing optimization is a powerful lever for revenue growth, and the application of ML provides a powerful solution, which can often outperform traditional approaches.
定价优化是收入增长的强大杠杆,而ML的应用提供了强大的解决方案,通常可以胜过传统方法。
In FitCo’s case, the application of ML to their challenge generated a 2x uplift in revenue growth vs their best performing rules-based alternative, and produced an estimated 11% uplift in revenue.
在FitCo的案例中,将ML应用于他们的挑战使收入增长增长了2倍,而其表现最佳的基于规则的替代方法则使收入增长了11%。
FitCo’s example helps demonstrate both how ML can be applied to optimize pricing, as well as the way managed services like SageMaker AutoPilot are able to put these powerful ML solutions within reach of virtually any organization.
FitCo的示例有助于说明如何应用ML来优化定价,以及诸如SageMaker AutoPilot之类的托管服务将这些强大的ML解决方案置于几乎任何组织都可以使用的方式。
ai人工智能收入