Based on Alternating Least Square
Alternating Least Square is a method to find the matrices X,Y given R The idea is to find the parameters which minimizes the L^2 cost function,
while regularization factor controls the speed of converge
Step:
1.fix X, optimize Y
2.fix Y, optimizr X
3.repeat until converge or reach the iteration number
Some algorithms about ALS
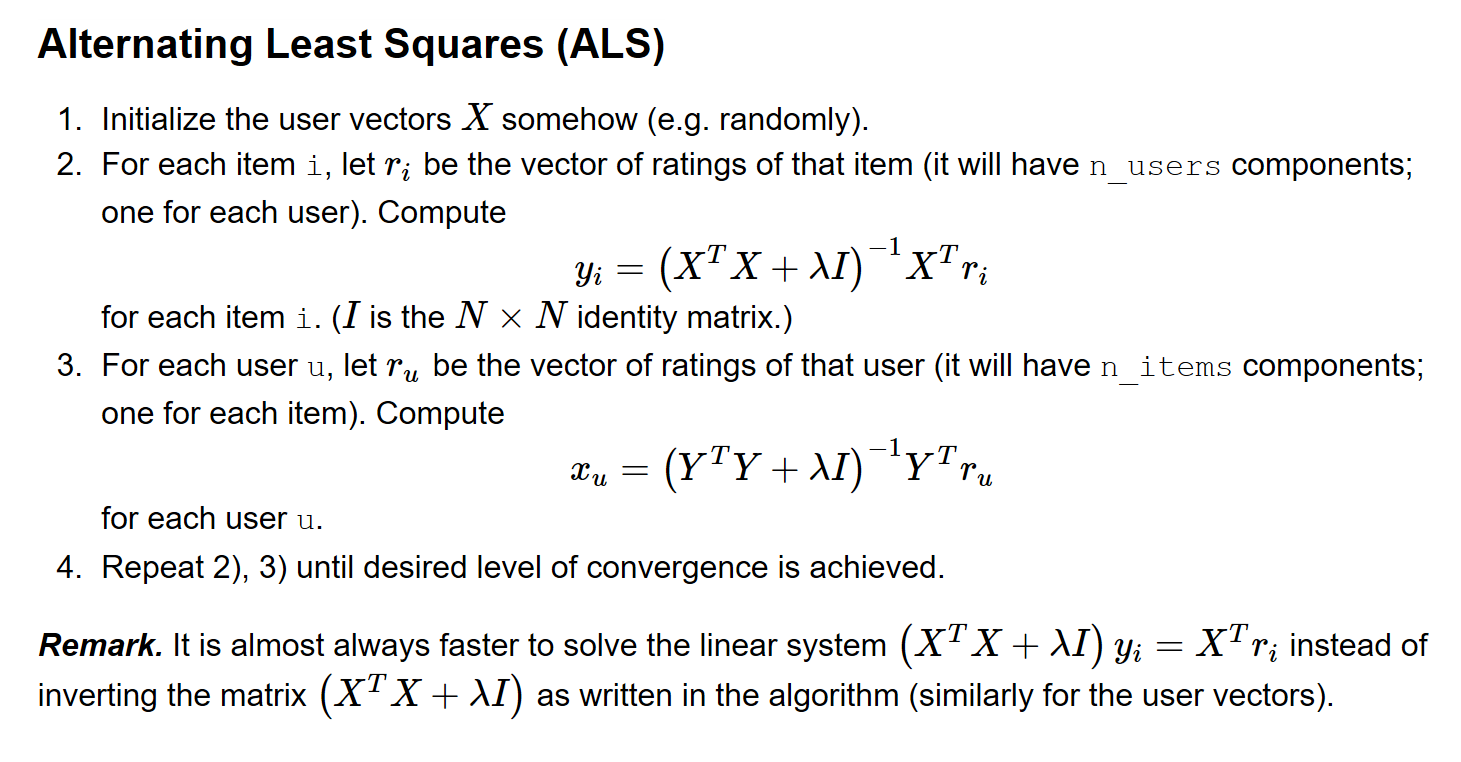
Implicit Feedback:Link
The basic approach is to forget about modeling the implicit feedback directly. Rather, we want to understand whether user
u
has a preference or not for item
i
using a simple boolean variable which we denote by
pui.pui. The number of clicks, listens, views, etc, will be interpreted as our confidence in our model.
While for the implicit feedback, the formula changes:

where C
ui
is our confidence in Pui. That is, the more a user has interacted with an item, the more we penalize our model for incorrectly predicting pui
LightFM use Stochastic Gradient Descent
DIfference between ALS and gradient descent
Need fewer iterations to reach the convergence,because every step is actually minimize the cost function