一、上传数据至OBS及授权给ModelArts使用 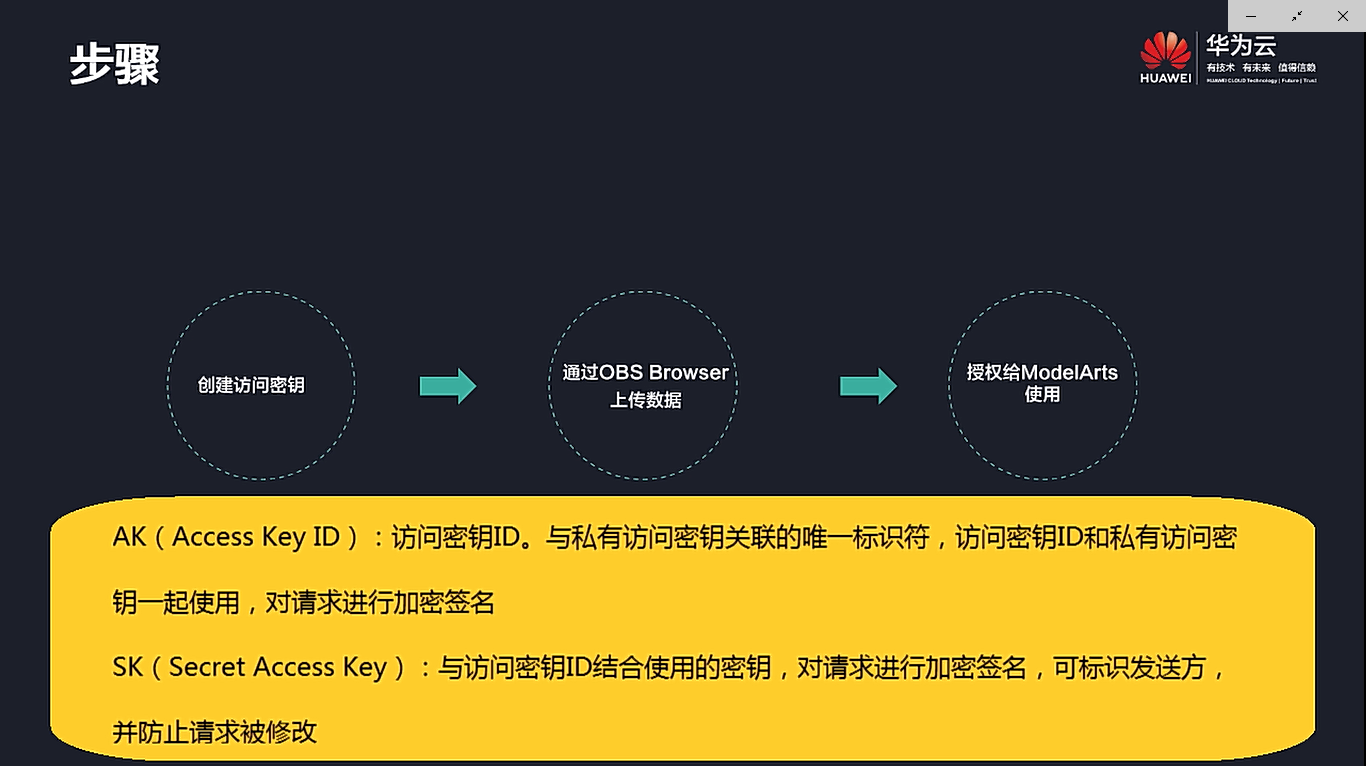
二、ModelArts-Notebook介绍 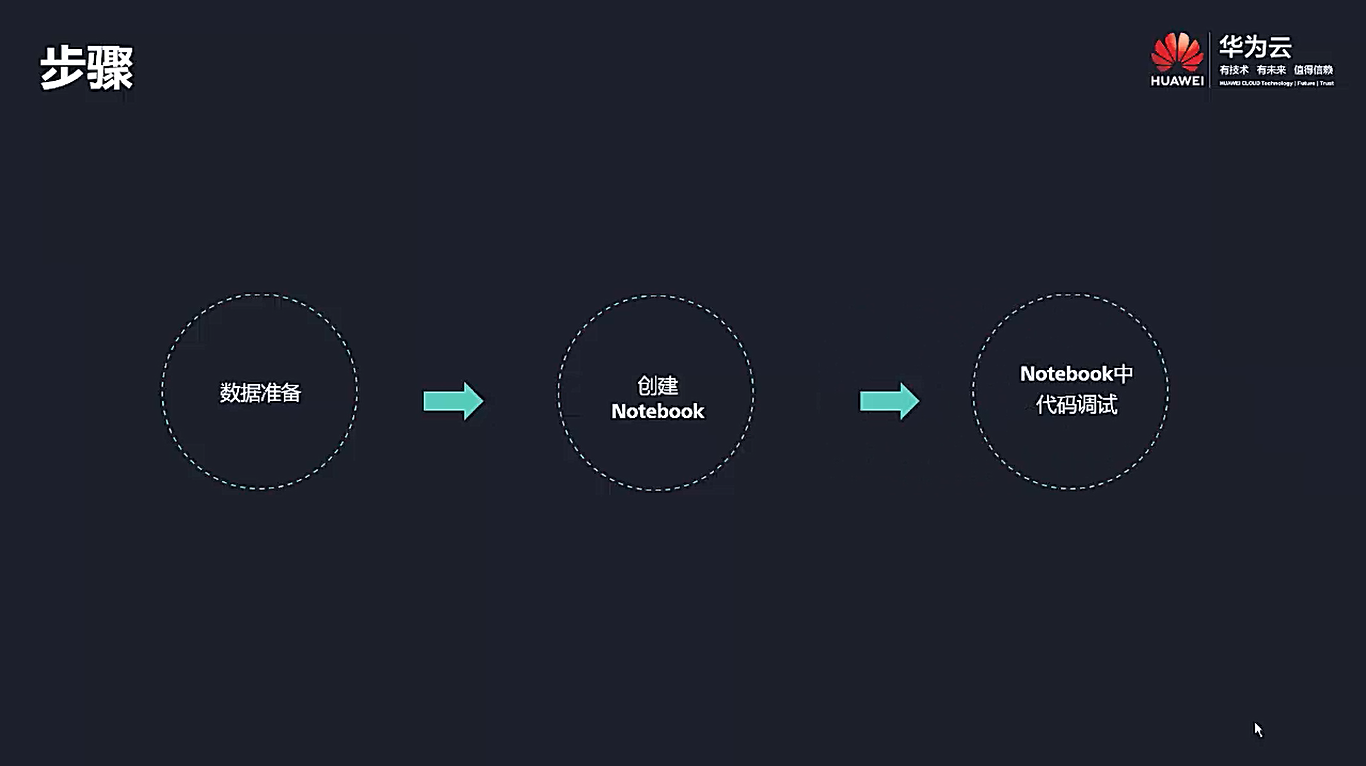
三、ModelArts-训练作业介绍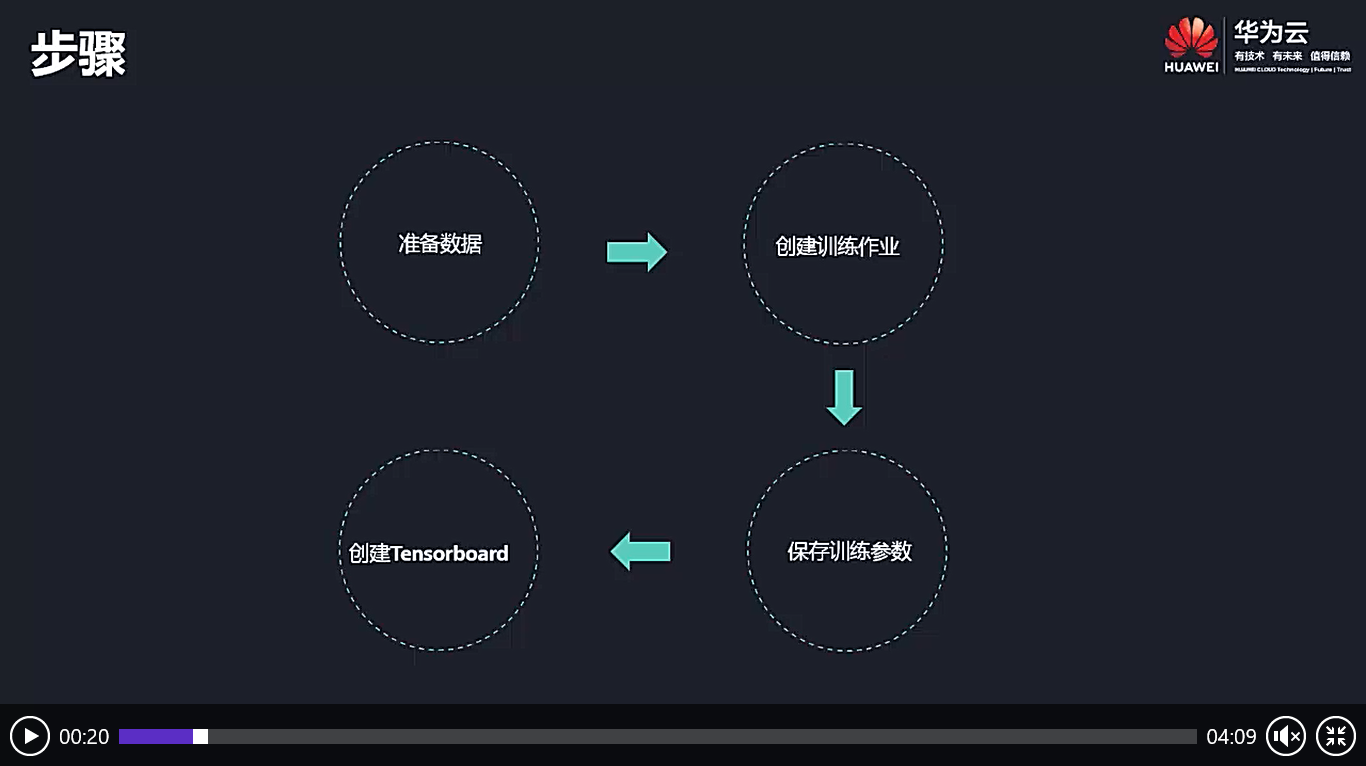
搬几个pytorch教程的例子
Before introducing Pytorch, we will first implement the network using numpy.
在介绍 PyTorch 之前, 我们先使用 numpy 实现网络.
Numpy provides an n-dimensional array object, and many functions for manipulating these arrays. Numpy is a generic framework for scientific computing;it does not know anything about anything about computation graphs,or deep learning, or gradients.However we can easily use numpy to fit a two-layer network to random data by manully implement the forward and backward pass through the network using numpy operations:
Numpy 提供了一个n维的数组对象, 并提供了许多操纵这个数组对象的函数. Numpy 是科学计算的通用框架; Numpy 数组没有计算图, 也没有深度学习, 也没有梯度下降等方法实现的接口. 但是我们仍然可以很容易地使用 numpy 生成随机数据 并将产生的数据传入双层的神经网络, 并使用 numpy 来实现这个网络的正向传播和反向传播:
# -*- coding: utf-8 -*-
import numpy as np
# N 是一个batch的样本数量; D_in是输入维度;
# H 是隐藏层向量的维度; D_out是输出维度.
N, D_in, H, D_out = 64, 1000, 100, 10
# 创建随机的输入输出数据
x = np.random.randn(N, D_in)
y = np.random.randn(N, D_out)
# 随机初始化权重参数
print("输入维度D_in:", D_in)
print("隐藏层向量维度H:", H)
w1 = np.random.randn(D_in, H)
# print("w1:", w1)
w2 = np.random.randn(H, D_out)
# print("w2:", w2)
learning_rate = 1e-6
for t in range(500):
# 前向计算, 算出y的预测值
h = x.dot(w1)
h_relu = np.maximum(h, 0)
y_pred = h_relu.dot(w2)
# 计算并打印误差值
loss = np.square(y_pred - y).sum()
# print(t, loss)
# print("t:",t)
# 在反向传播中, 计算出误差关于w1和w2的导数
grad_y_pred = 2.0 * (y_pred - y)
grad_w2 = h_relu.T.dot(grad_y_pred)
grad_h_relu = grad_y_pred.dot(w2.T)
grad_h = grad_h_relu.copy()
grad_h[h < 0] = 0
grad_w1 = x.T.dot(grad_h)
# 更新权重
w1 -= learning_rate * grad_w1
w2 -= learning_rate * grad_w2