1.4 TF常用函数
tf.cast(tensor,dtype=datatype)可以进行强制类型转换。
tf.reduce_min(tensor)和tf.reduce_max(tensor)将计算出张量中所有元素的最大值和最小值。
import tensorflow as tfx1 = tf.constant([1., 2., 3.], dtype=tf.float64)print("x1:", x1)x2 = tf.cast(x1, tf.int32)print("x2", x2)print("minimum of x2:", tf.reduce_min(x2))print("maxmum of x2:", tf.reduce_max(x2))
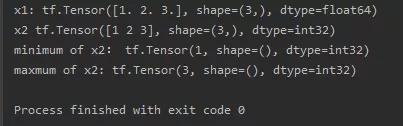
axis代表轴。以矩阵为例axis=0表示列而axis=1 表示行。tf.reduce_mean(tensor, axis=operating axis)被用来计算在axis方向上的随机数,但如果采用默认的axis,它将计算所有元素的均值。tf.reduce_sum(tensor, axis)计算合也是同理
import tensorflow as tfx = tf.constant([[1, 2, 3], [2, 2, 3]])print("x:", x)print("mean of x:", tf.reduce_mean(x))print("sum of x:", tf.reduce_sum(x, axis=1))
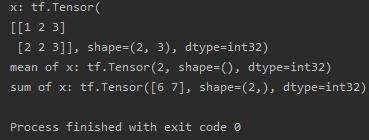
tf.Variable()可以将张量标记为可训练的。在回传环节被标记的张量可以记录梯度的信息。
import tensorflow as tfa = tf.ones([1, 3])b = tf.fill([1, 3], 3.)print("a:", a)print("b:", b)print("a+b:", tf.add(a, b))print("a-b:", tf.subtract(a, b))print("a*b:", tf.multiply(a, b))print("b/a:", tf.divide(b, a))
四则运算: tf.add, tf.subtract, tf.multiply, tf.divede(tensor1,tensor2),张量的维度必须相等
import tensorflow as tfa = tf.ones([1, 3])b = tf.fill([1, 3], 3.)print("a:", a)print("b:", b)print("a+b:", tf.add(a, b))print("a-b:", tf.subtract(a, b))print("a*b:", tf.multiply(a, b))print("b/a:", tf.divide(b, a))
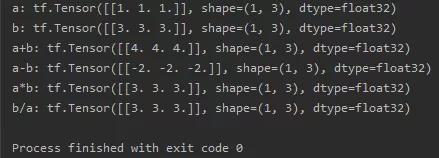
乘方运算: tf.square(tensor), tf.pow(tensor,n), tf.sqrt(tensor)
import tensorflow as tfa = tf.fill([1, 2], 3.)print("a:", a)print("a的立方:", tf.pow(a, 3))print("a的平方:", tf.square(a))print("a的开方:", tf.sqrt(a))
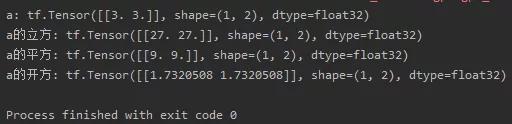
矩阵乘法: tf.matmul(tensor1,tensor2)需要符合乘法规则
import tensorflow as tfa = tf.ones([3, 2])b = tf.fill([2, 3], 3.)print("a:", a)print("b:", b)print("a*b:", tf.matmul(a, b))
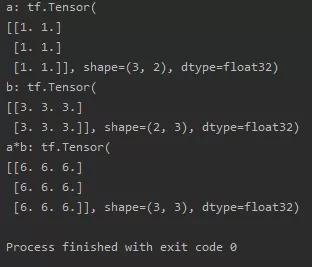
Tensorflow提供给我们一个函数把特征和标签配对。把第一位张量的第一维分隔开。常用以下语句使用
dataset. data =tf.data.Dataset.from_tensor_slices((tensor1,tensor2))
import tensorflow as tffeatures = tf.constant([12, 23, 10, 17])labels = tf.constant([0, 1, 1, 0])dataset = tf.data.Dataset.from_tensor_slices((features, labels))for element in dataset: print(element)

我们可以在with函数中使用tf.GradientTape实现对函数某个参数的求导运算
with tf.GradientTape() as tape:若干计算过程grad=tape.gradient(函数,对谁求导)
import tensorflow as tfwith tf.GradientTape() as tape: x = tf.Variable(tf.constant(3.0)) y = tf.pow(x, 2)grad = tape.gradient(y, x)print(grad)
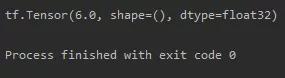
python内置函数enumerate可以枚举每一个元素并在元素前配上对应的索引号
seq = ['one', 'two', 'three']for i, element in enumerate(seq): print(i, element)
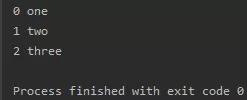
在实现分类问题时常用独热码表示标签比如标签为1,独热码表示为(0,1,0),tensorflow中提供了函数tf.one_hot(带转换的数据,depth=几分类)转换为独热码形式。
import tensorflow as tfclasses = 3labels = tf.constant([1, 0, 0, 2, 1]) output = tf.one_hot(labels, depth=classes)print("result of labels1:", output)print("")
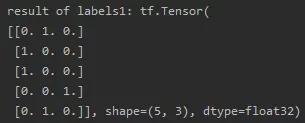

上图中我们得到的结果就是每种鸢尾花的可能性大小,但是概率不可能大于1也不可能小于0,所有上式计算出的并不是概率,所以我们使用softmax函数把输入的数据映射为0~1之间的实数并且归一化保证和为1。max理解为取最大值,是二元对立非黑即白的,而soft则是缓和了max的对立,为依照概率取值。
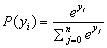
e^y0=2.75,p0=0.256
e^y1=7.46,p1=0.695
e^y2=0.52,p2=0.048
import tensorflow as tfy = tf.constant([1.01, 2.01, -0.66])y_pro = tf.nn.softmax(y)print("After softmax, y_pro is:", y_pro)print("The sum of y_pro:", tf.reduce_sum(y_pro))]

assign_sub函数可以自减参数的值并且没有返回值,在调用assign_sub前需要先定义变量为可训练的。
import tensorflow as tfx = tf.Variable(4)x.assign_sub(1)print("x:", x)

tf.argmax(张量名,axis=操作轴)返回沿指定维度最大值的索引。
import numpy as npimport tensorflow as tftest = np.array([[1, 2, 3], [2, 3, 4], [5, 4, 3], [8, 7, 2]])test = tf.convert_to_tensor(test)print("test:", test)print("每一列的最大值的索引:", tf.argmax(test, axis=0))print("每一行的最大值的索引", tf.argmax(test, axis=1))
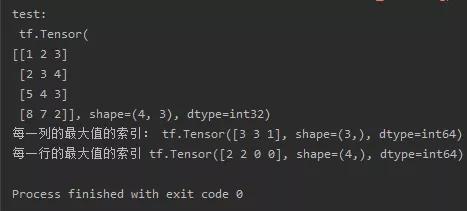
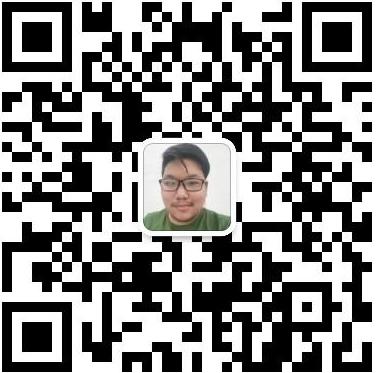
微信搜索:做梦当院士的李子哥