±----------------------------±------------------------------±---------------------------------------+
Total line number = 5
It costs 0.006s
#按照自然月份的时间区间分组聚合查询
对应的 SQL 语句是:
select count(status) from root.ln.wf01.wt01 where time > 2017-11-01T01:00:00
group by([2017-11-01T00:00:00, 2019-11-07T23:00:00), 1mo, 2mo);
这条查询的含义是:
由于用户指定了滑动步长为2mo,GROUP BY 语句执行时将会每次把时间间隔往后移动 2 个自然月的步长,而不是默认的 1 个自然月。
也就意味着,我们想要取从 2017-11-01 到 2019-11-07 每 2 个自然月的第一个月的数据。
上面这个例子的第一个参数是显示窗口参数,决定了最终的显示范围是 [2017-11-01T00:00:00, 2019-11-07T23:00:00)。
起始时间为 2017-11-01T00:00:00,滑动步长将会以起始时间作为标准按月递增,取当月的 1 号作为时间间隔的起始时间。
上面这个例子的第二个参数是划分时间轴的时间间隔参数,将1mo当作划分间隔,显示窗口参数的起始时间当作分割原点,时间轴即被划分为连续的时间间隔:[2017-11-01T00:00:00, 2017-12-01T00:00:00), [2018-02-01T00:00:00, 2018-03-01T00:00:00), [2018-05-03T00:00:00, 2018-06-01T00:00:00) 等等。
上面这个例子的第三个参数是每次时间间隔的滑动步长。
然后系统将会用 WHERE 子句中的时间和值过滤条件以及 GROUP BY 语句中的第一个参数作为数据的联合过滤条件,获得满足所有过滤条件的数据(在这个例子里是在 [2017-11-01T00:00:00, 2019-11-07T23:00:00) 这个时间范围的数据),并把这些数据映射到之前分割好的时间轴中(这个例子里是从 2017-11-01T00:00:00 到 2019-11-07T23:00:00:00 的每两个自然月的第一个月)
每个时间间隔窗口内都有数据,SQL 执行后的结果集如下所示:
±----------------------------±------------------------------+
| Time|count(root.ln.wf01.wt01.status)|
±----------------------------±------------------------------+
|2017-11-01T00:00:00.000+08:00| 259|
|2018-01-01T00:00:00.000+08:00| 250|
|2018-03-01T00:00:00.000+08:00| 259|
|2018-05-01T00:00:00.000+08:00| 251|
|2018-07-01T00:00:00.000+08:00| 242|
|2018-09-01T00:00:00.000+08:00| 225|
|2018-11-01T00:00:00.000+08:00| 216|
|2019-01-01T00:00:00.000+08:00| 207|
|2019-03-01T00:00:00.000+08:00| 216|
|2019-05-01T00:00:00.000+08:00| 207|
|2019-07-01T00:00:00.000+08:00| 199|
|2019-09-01T00:00:00.000+08:00| 181|
|2019-11-01T00:00:00.000+08:00| 60|
±----------------------------±------------------------------+
对应的 SQL 语句是:
select count(status) from root.ln.wf01.wt01 group by([2017-10-31T00:00:00, 2019-11-07T23:00:00), 1mo, 2mo);
这条查询的含义是:
由于用户指定了滑动步长为2mo,GROUP BY 语句执行时将会每次把时间间隔往后移动 2 个自然月的步长,而不是默认的 1 个自然月。
也就意味着,我们想要取从 2017-10-31 到 2019-11-07 每 2 个自然月的第一个月的数据。
与上述示例不同的是起始时间为 2017-10-31T00:00:00,滑动步长将会以起始时间作为标准按月递增,取当月的 31 号(即最后一天)作为时间间隔的起始时间。若起始时间设置为 30 号,滑动步长会将时间间隔的起始时间设置为当月 30 号,若不存在则为最后一天。
上面这个例子的第一个参数是显示窗口参数,决定了最终的显示范围是 [2017-10-31T00:00:00, 2019-11-07T23:00:00)。
上面这个例子的第二个参数是划分时间轴的时间间隔参数,将1mo当作划分间隔,显示窗口参数的起始时间当作分割原点,时间轴即被划分为连续的时间间隔:[2017-10-31T00:00:00, 2017-11-31T00:00:00), [2018-02-31T00:00:00, 2018-03-31T00:00:00), [2018-05-31T00:00:00, 2018-06-31T00:00:00) 等等。
上面这个例子的第三个参数是每次时间间隔的滑动步长。
然后系统将会用 WHERE 子句中的时间和值过滤条件以及 GROUP BY 语句中的第一个参数作为数据的联合过滤条件,获得满足所有过滤条件的数据(在这个例子里是在 [2017-10-31T00:00:00, 2019-11-07T23:00:00) 这个时间范围的数据),并把这些数据映射到之前分割好的时间轴中(这个例子里是从 2017-10-31T00:00:00 到 2019-11-07T23:00:00:00 的每两个自然月的第一个月)
每个时间间隔窗口内都有数据,SQL 执行后的结果集如下所示:
±----------------------------±------------------------------+
| Time|count(root.ln.wf01.wt01.status)|
±----------------------------±------------------------------+
|2017-10-31T00:00:00.000+08:00| 251|
|2017-12-31T00:00:00.000+08:00| 250|
|2018-02-28T00:00:00.000+08:00| 259|
|2018-04-30T00:00:00.000+08:00| 250|
|2018-06-30T00:00:00.000+08:00| 242|
|2018-08-31T00:00:00.000+08:00| 225|
|2018-10-31T00:00:00.000+08:00| 216|
|2018-12-31T00:00:00.000+08:00| 208|
|2019-02-28T00:00:00.000+08:00| 216|
|2019-04-30T00:00:00.000+08:00| 208|
|2019-06-30T00:00:00.000+08:00| 199|
|2019-08-31T00:00:00.000+08:00| 181|
|2019-10-31T00:00:00.000+08:00| 69|
±----------------------------±------------------------------+
#左开右闭区间
每个区间的结果时间戳为区间右端点,对应的 SQL 语句是:
select count(status) from root.ln.wf01.wt01 group by ((2017-11-01T00:00:00, 2017-11-07T23:00:00],1d);
这条查询语句的时间区间是左开右闭的,结果中不会包含时间点 2017-11-01 的数据,但是会包含时间点 2017-11-07 的数据。
SQL 执行后的结果集如下所示:
±----------------------------±------------------------------+
| Time|count(root.ln.wf01.wt01.status)|
±----------------------------±------------------------------+
|2017-11-02T00:00:00.000+08:00| 1440|
|2017-11-03T00:00:00.000+08:00| 1440|
|2017-11-04T00:00:00.000+08:00| 1440|
|2017-11-05T00:00:00.000+08:00| 1440|
|2017-11-06T00:00:00.000+08:00| 1440|
|2017-11-07T00:00:00.000+08:00| 1440|
|2017-11-07T23:00:00.000+08:00| 1380|
±----------------------------±------------------------------+
Total line number = 7
It costs 0.004s
#与分组聚合混合使用
通过定义 LEVEL 来统计指定层级下的数据点个数。
例如:
统计降采样后的数据点个数
select count(status) from root.ln.wf01.wt01 group by ((2017-11-01T00:00:00, 2017-11-07T23:00:00],1d), level=1;
结果:
±----------------------------±------------------------+
| Time|COUNT(root.ln.*.*.status)|
±----------------------------±------------------------+
|2017-11-02T00:00:00.000+08:00| 1440|
|2017-11-03T00:00:00.000+08:00| 1440|
|2017-11-04T00:00:00.000+08:00| 1440|
|2017-11-05T00:00:00.000+08:00| 1440|
|2017-11-06T00:00:00.000+08:00| 1440|
|2017-11-07T00:00:00.000+08:00| 1440|
|2017-11-07T23:00:00.000+08:00| 1380|
±----------------------------±------------------------+
Total line number = 7
It costs 0.006s
加上滑动 Step 的降采样后的结果也可以汇总
select count(status) from root.ln.wf01.wt01 group by ([2017-11-01 00:00:00, 2017-11-07 23:00:00), 3h, 1d), level=1;
±----------------------------±------------------------+
| Time|COUNT(root.ln.*.*.status)|
±----------------------------±------------------------+
|2017-11-01T00:00:00.000+08:00| 180|
|2017-11-02T00:00:00.000+08:00| 180|
|2017-11-03T00:00:00.000+08:00| 180|
|2017-11-04T00:00:00.000+08:00| 180|
|2017-11-05T00:00:00.000+08:00| 180|
|2017-11-06T00:00:00.000+08:00| 180|
|2017-11-07T00:00:00.000+08:00| 180|
±----------------------------±------------------------+
Total line number = 7
It costs 0.004s
#路径层级分组聚合
在时间序列层级结构中,分层聚合查询用于对某一层级下同名的序列进行聚合查询。
使用 GROUP BY LEVEL = INT 来指定需要聚合的层级,并约定 ROOT 为第 0 层。若统计 “root.ln” 下所有序列则需指定 level 为 1。
分层聚合查询支持使用所有内置聚合函数。对于 sum,avg,min\_value, max\_value, extreme 五种聚合函数,需保证所有聚合的时间序列数据类型相同。其他聚合函数没有此限制。
示例1: 不同 database 下均存在名为 status 的序列, 如 “root.ln.wf01.wt01.status”, “root.ln.wf02.wt02.status”, 以及 “root.sgcc.wf03.wt01.status”, 如果需要统计不同 database 下 status 序列的数据点个数,使用以下查询:
select count(status) from root.\*\* group by level = 1
运行结果为:
±------------------------±--------------------------+
|count(root.ln.*.*.status)|count(root.sgcc.*.*.status)|
±------------------------±--------------------------+
| 20160| 10080|
±------------------------±--------------------------+
Total line number = 1
It costs 0.003s
示例2: 统计不同设备下 status 序列的数据点个数,可以规定 level = 3,
select count(status) from root.\*\* group by level = 3
运行结果为:
±--------------------------±--------------------------+
|count(root.*.*.wt01.status)|count(root.*.*.wt02.status)|
±--------------------------±--------------------------+
| 20160| 10080|
±--------------------------±--------------------------+
Total line number = 1
It costs 0.003s
注意,这时会将 database ln 和 sgcc 下名为 wt01 的设备视为同名设备聚合在一起。
示例3: 统计不同 database 下的不同设备中 status 序列的数据点个数,可以使用以下查询:
select count(status) from root.\*\* group by level = 1, 3
运行结果为:
±---------------------------±---------------------------±-----------------------------+
|count(root.ln.*.wt01.status)|count(root.ln.*.wt02.status)|count(root.sgcc.\*.wt01.status)|
±---------------------------±---------------------------±-----------------------------+
| 10080| 10080| 10080|
±---------------------------±---------------------------±-----------------------------+
Total line number = 1
It costs 0.003s
示例4: 查询所有序列下温度传感器 temperature 的最大值,可以使用下列查询语句:
select max\_value(temperature) from root.\*\* group by level = 0
运行结果:
±--------------------------------+
|max\_value(root.*.*.\*.temperature)|
±--------------------------------+
| 26.0|
±--------------------------------+
Total line number = 1
It costs 0.013s
示例5: 上面的查询都是针对某一个传感器,特别地,如果想要查询某一层级下所有传感器拥有的总数据点数,则需要显式规定测点为 \*
select count(\*) from root.ln.\*\* group by level = 2
运行结果:
±---------------------±---------------------+
|count(root.*.wf01.*.*)|count(root.*.wf02.*.*)|
±---------------------±---------------------+
| 20160| 20160|
±---------------------±---------------------+
Total line number = 1
It costs 0.013s
#标签分组聚合
IoTDB 支持通过 GROUP BY TAGS 语句根据时间序列中定义的标签的键值做聚合查询。
我们先在 IoTDB 中写入如下示例数据,稍后会以这些数据为例介绍标签聚合查询。
这些是某工厂 factory1 在多个城市的多个车间的设备温度数据, 时间范围为 [1000, 10000)。
时间序列路径中的设备一级是设备唯一标识。城市信息 city 和车间信息 workshop 则被建模在该设备时间序列的标签中。
其中,设备 d1、d2 在 Beijing 的 w1 车间, d3、d4 在 Beijing 的 w2 车间,d5、d6 在 Shanghai 的 w1 车间,d7 在 Shanghai 的 w2 车间。
d8 和 d9 设备目前处于调试阶段,还未被分配到具体的城市和车间,所以其相应的标签值为空值。
create database root.factory1;
create timeseries root.factory1.d1.temperature with datatype=FLOAT tags(city=Beijing, workshop=w1);
create timeseries root.factory1.d2.temperature with datatype=FLOAT tags(city=Beijing, workshop=w1);
create timeseries root.factory1.d3.temperature with datatype=FLOAT tags(city=Beijing, workshop=w2);
create timeseries root.factory1.d4.temperature with datatype=FLOAT tags(city=Beijing, workshop=w2);
create timeseries root.factory1.d5.temperature with datatype=FLOAT tags(city=Shanghai, workshop=w1);
create timeseries root.factory1.d6.temperature with datatype=FLOAT tags(city=Shanghai, workshop=w1);
create timeseries root.factory1.d7.temperature with datatype=FLOAT tags(city=Shanghai, workshop=w2);
create timeseries root.factory1.d8.temperature with datatype=FLOAT;
create timeseries root.factory1.d9.temperature with datatype=FLOAT;
insert into root.factory1.d1(time, temperature) values(1000, 104.0);
insert into root.factory1.d1(time, temperature) values(3000, 104.2);
insert into root.factory1.d1(time, temperature) values(5000, 103.3);
insert into root.factory1.d1(time, temperature) values(7000, 104.1);
insert into root.factory1.d2(time, temperature) values(1000, 104.4);
insert into root.factory1.d2(time, temperature) values(3000, 103.7);
insert into root.factory1.d2(time, temperature) values(5000, 103.3);
insert into root.factory1.d2(time, temperature) values(7000, 102.9);
insert into root.factory1.d3(time, temperature) values(1000, 103.9);
insert into root.factory1.d3(time, temperature) values(3000, 103.8);
insert into root.factory1.d3(time, temperature) values(5000, 102.7);
insert into root.factory1.d3(time, temperature) values(7000, 106.9);
insert into root.factory1.d4(time, temperature) values(1000, 103.9);
insert into root.factory1.d4(time, temperature) values(5000, 102.7);
insert into root.factory1.d4(time, temperature) values(7000, 106.9);
insert into root.factory1.d5(time, temperature) values(1000, 112.9);
insert into root.factory1.d5(time, temperature) values(7000, 113.0);
insert into root.factory1.d6(time, temperature) values(1000, 113.9);
insert into root.factory1.d6(time, temperature) values(3000, 113.3);
insert into root.factory1.d6(time, temperature) values(5000, 112.7);
insert into root.factory1.d6(time, temperature) values(7000, 112.3);
insert into root.factory1.d7(time, temperature) values(1000, 101.2);
insert into root.factory1.d7(time, temperature) values(3000, 99.3);
insert into root.factory1.d7(time, temperature) values(5000, 100.1);
insert into root.factory1.d7(time, temperature) values(7000, 99.8);
insert into root.factory1.d8(time, temperature) values(1000, 50.0);
insert into root.factory1.d8(time, temperature) values(3000, 52.1);
insert into root.factory1.d8(time, temperature) values(5000, 50.1);
insert into root.factory1.d8(time, temperature) values(7000, 50.5);
## 最后
**自我介绍一下,小编13年上海交大毕业,曾经在小公司待过,也去过华为、OPPO等大厂,18年进入阿里一直到现在。**
**深知大多数Java工程师,想要提升技能,往往是自己摸索成长,自己不成体系的自学效果低效漫长且无助。**
**因此收集整理了一份《2024年嵌入式&物联网开发全套学习资料》,初衷也很简单,就是希望能够帮助到想自学提升又不知道该从何学起的朋友,同时减轻大家的负担。**
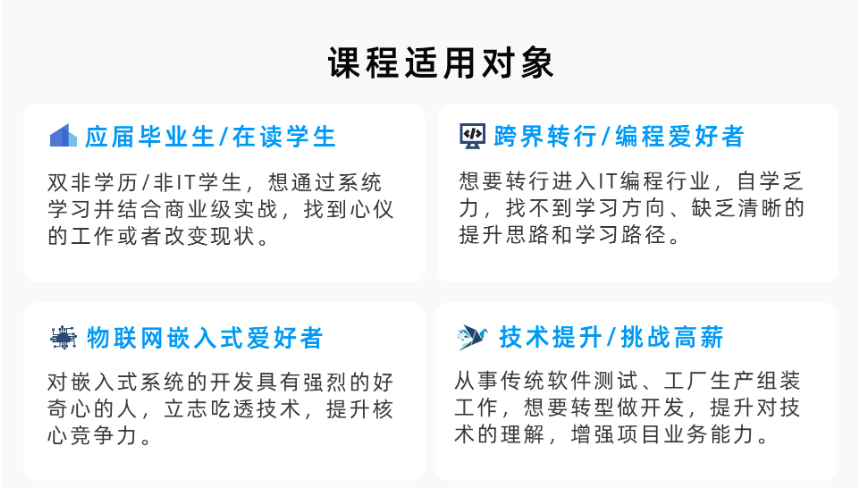
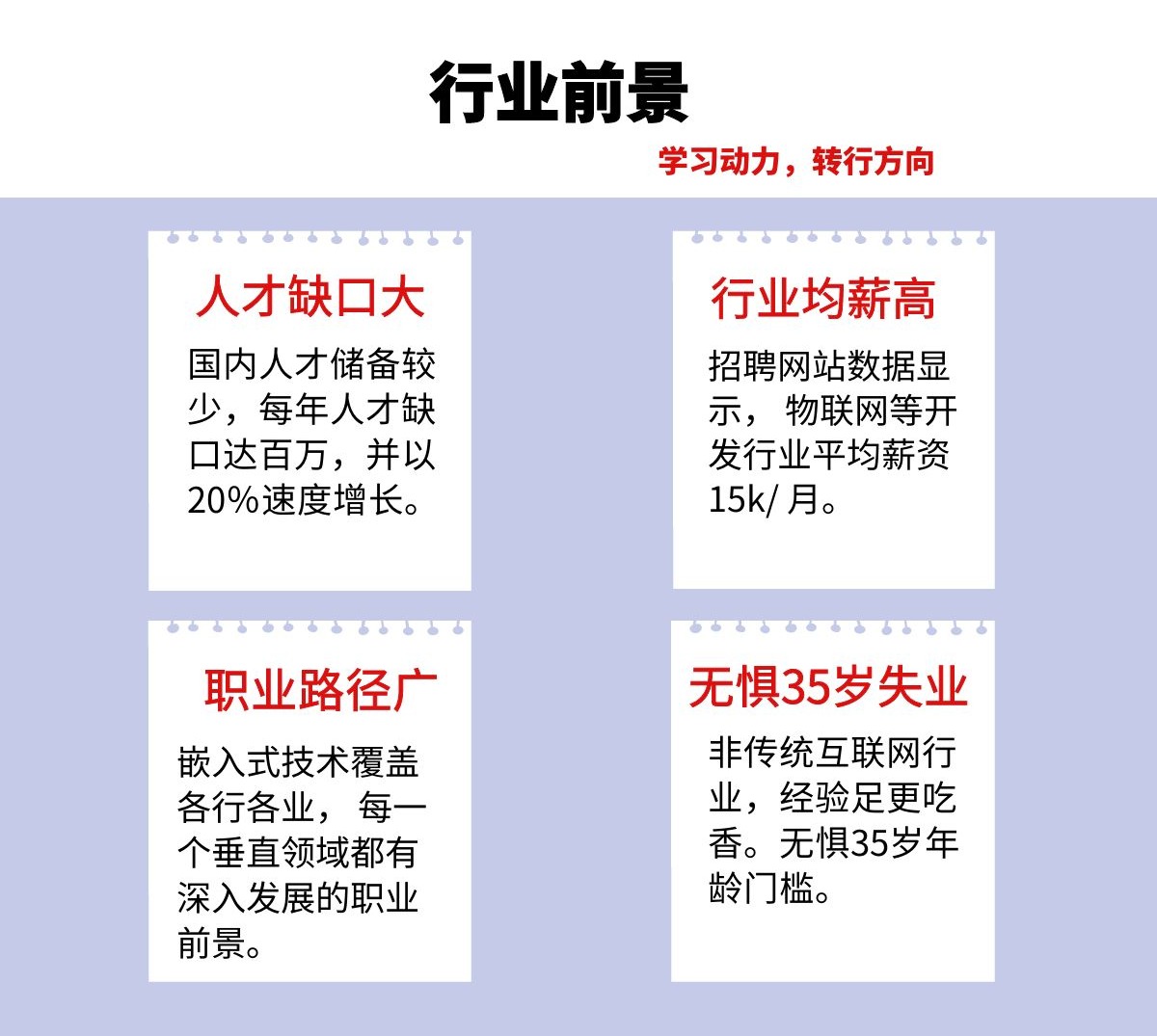
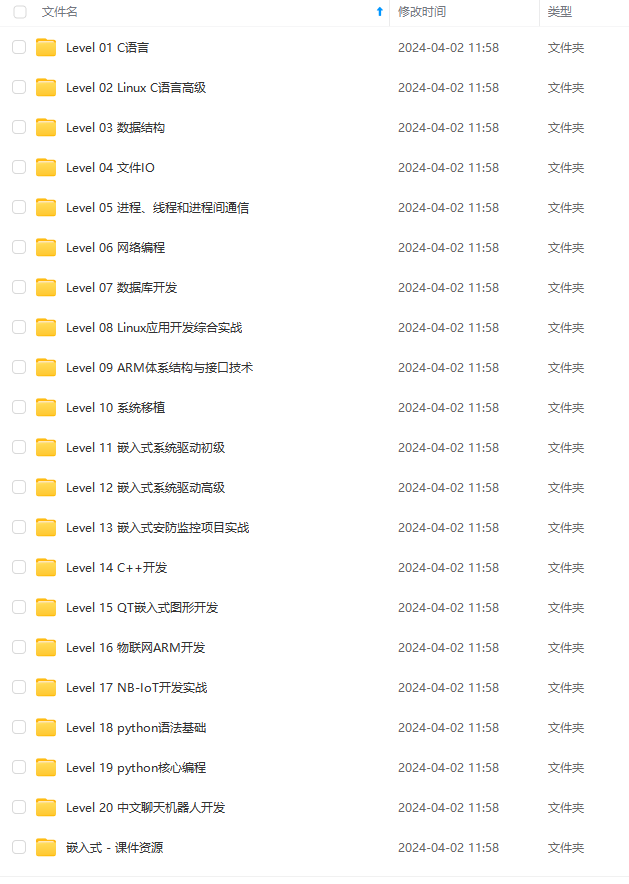
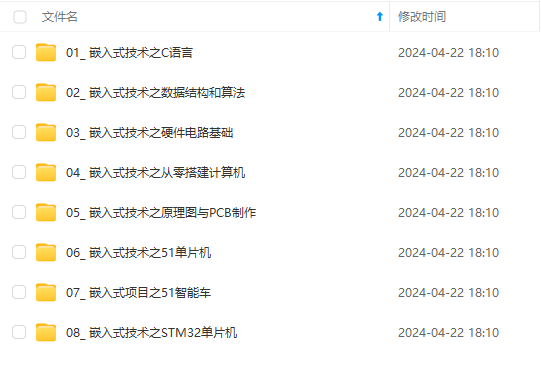
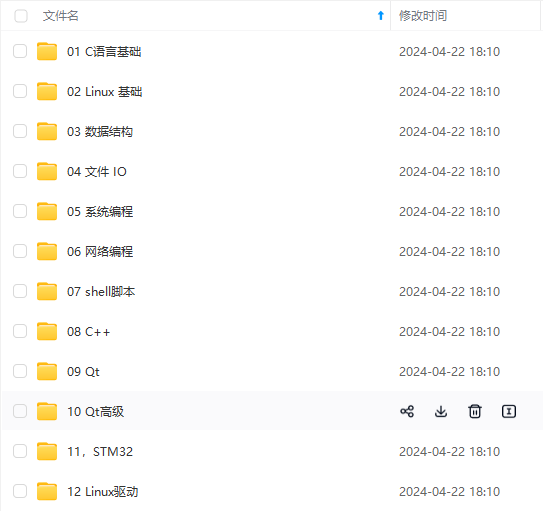
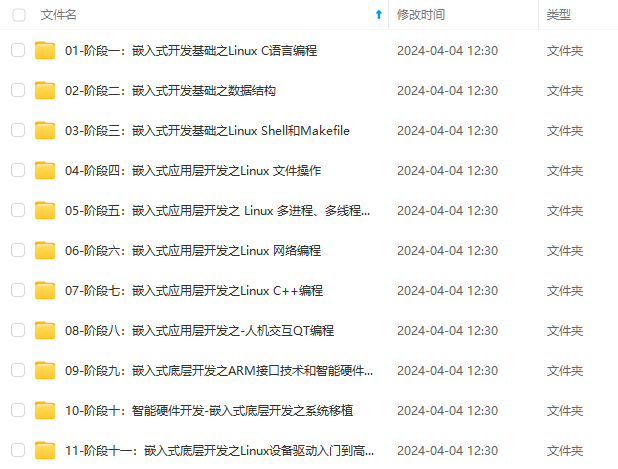
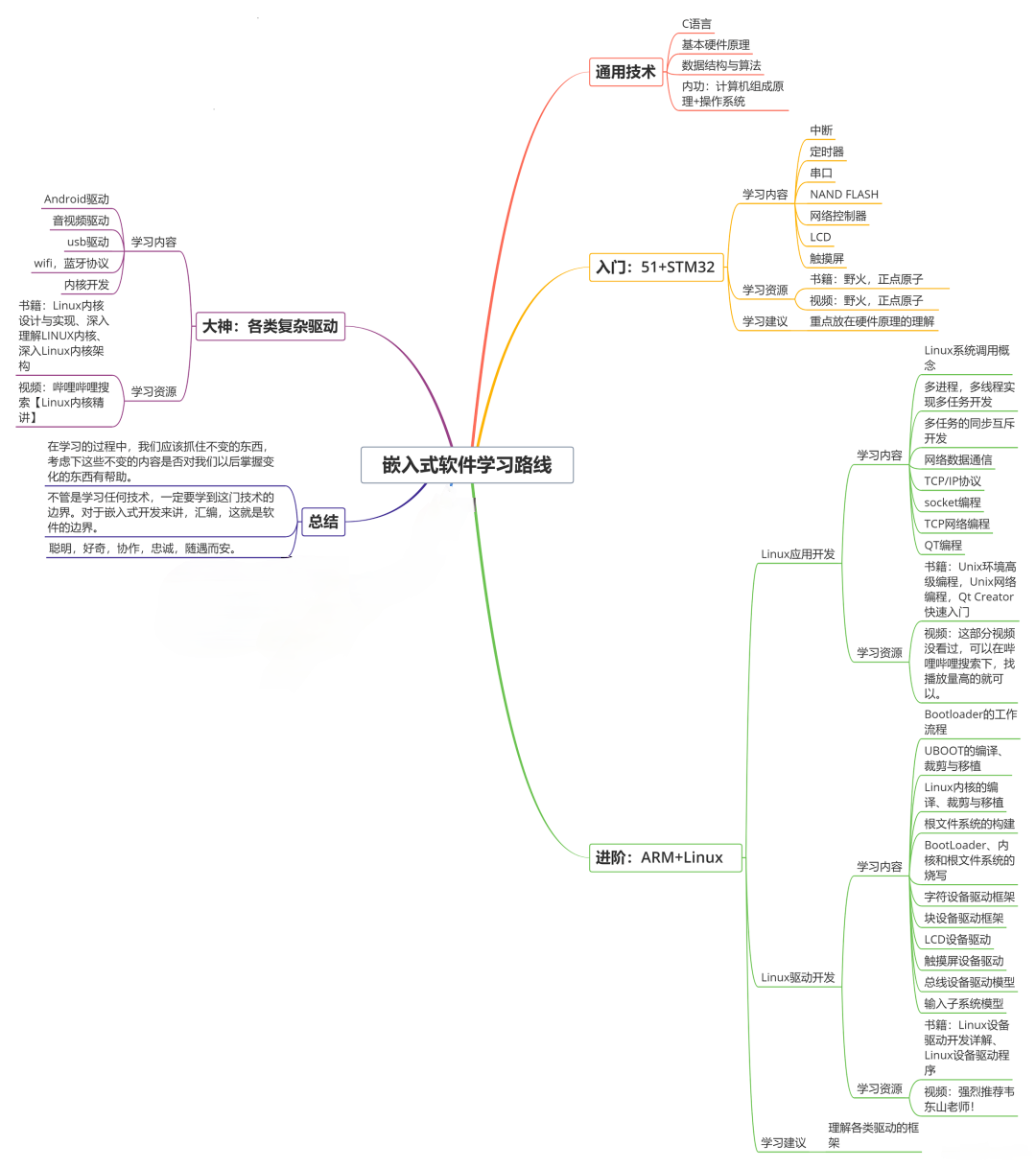
**既有适合小白学习的零基础资料,也有适合3年以上经验的小伙伴深入学习提升的进阶课程,基本涵盖了95%以上嵌入式&物联网开发知识点,真正体系化!**
[**如果你觉得这些内容对你有帮助,需要这份全套学习资料的朋友可以戳我获取!!**](https://bbs.csdn.net/topics/618654289)
**由于文件比较大,这里只是将部分目录大纲截图出来,每个节点里面都包含大厂面经、学习笔记、源码讲义、实战项目、讲解视频,并且后续会持续更新**!!
I0-1715583993688)]
[外链图片转存中...(img-t0Q4hrO9-1715583993689)]
[外链图片转存中...(img-8I2UY0ne-1715583993689)]
**既有适合小白学习的零基础资料,也有适合3年以上经验的小伙伴深入学习提升的进阶课程,基本涵盖了95%以上嵌入式&物联网开发知识点,真正体系化!**
[**如果你觉得这些内容对你有帮助,需要这份全套学习资料的朋友可以戳我获取!!**](https://bbs.csdn.net/topics/618654289)
**由于文件比较大,这里只是将部分目录大纲截图出来,每个节点里面都包含大厂面经、学习笔记、源码讲义、实战项目、讲解视频,并且后续会持续更新**!!