在这个实例中,我将会向大家介绍如何使用Python 为 Hadoop编写一个简单的MapReduce程序。
尽管Hadoop 框架是使用Java编写的但是我们仍然需要使用像C++、Python等语言来实现 Hadoop程序。尽管Hadoop官方网站给的示例程序是使用Jython编写并打包成Jar文件,这样显然造成了不便,其实,不一定非要这样来实现,我们可以使用Python与Hadoop 关联进行编程,看看位于/src/examples/python/WordCount.py 的例子,你将了解到我在说什么。
我们想要做什么?
我们将编写一个简单的 MapReduce 程序,使用的是C-Python,而不是Jython编写后打包成jar包的程序。
我们的这个例子将模仿 WordCount 并使用Python来实现,例子通过读取文本文件来统计出单词的出现次数。结果也以文本形式输出,每一行包含一个单词和单词出现的次数,两者中间使用制表符来想间隔。
先决条件
编写这个程序之前,你学要架设好Hadoop 集群,这样才能不会在后期工作抓瞎。如果你没有架设好,那么在后面有个简明教程来教你在Ubuntu Linux 上搭建(同样适用于其他发行版linux、unix)
如何在Ubuntu Linux 上搭建hadoop的单节点模式和伪分布模式,请参阅博文 Ubuntu上搭建Hadoop环境(单机模式+伪分布模式)
Python的MapReduce代码
使用Python编写MapReduce代码的技巧就在于我们使用了 HadoopStreaming 来帮助我们在Map 和 Reduce间传递数据通过STDIN (标准输入)和STDOUT (标准输出).我们仅仅使用Python的sys.stdin来输入数据,使用sys.stdout输出数据,这样做是因为HadoopStreaming会帮我们办好其他事。这是真的,别不相信!
Map: mapper.py
将下列的代码保存在/usr/local/hadoop/mapper.py中,他将从STDIN读取数据并将单词成行分隔开,生成一个列表映射单词与发生次数的关系:
注意:要确保这个脚本有足够权限(chmod +x mapper.py)。
#!/usr/bin/env python
import sys
# input comes from STDIN (standard input)
for line in sys.stdin:
# remove leading and trailing whitespace
line = line.strip()
# split the line into words
words = line.split()
# increase counters
for word in words:
# write the results to STDOUT (standard output);
# what we output here will be the input for the
# Reduce step, i.e. the input for reducer.py
#
# tab-delimited; the trivial word count is 1
print '%s\t%s' % (word, 1)
在这个脚本中,并不计算出单词出现的总数,它将输出 " 1" 迅速地,尽管可能会在输入中出现多次,计算是留给后来的Reduce步骤(或叫做程序)来实现。当然你可以改变下编码风格,完全尊重你的习惯。Reduce: reducer.py
将代码存储在/usr/local/hadoop/reducer.py 中,这个脚本的作用是从mapper.py 的STDIN中读取结果,然后计算每个单词出现次数的总和,并输出结果到STDOUT。
同样,要注意脚本权限:chmod +x reducer.py
#!/usr/bin/env python
from operator import itemgetter
import sys
current_word = None
current_count = 0
word = None
# input comes from STDIN
for line in sys.stdin:
# remove leading and trailing whitespace
line = line.strip()
# parse the input we got from mapper.py
word, count = line.split('\t', 1)
# convert count (currently a string) to int
try:
count = int(count)
except ValueError:
# count was not a number, so silently
# ignore/discard this line
continue
# this IF-switch only works because Hadoop sorts map output
# by key (here: word) before it is passed to the reducer
if current_word == word:
current_count += count
else:
if current_word:
# write result to STDOUT
print '%s\t%s' % (current_word, current_count)
current_count = count
current_word = word
# do not forget to output the last word if needed!
if current_word == word:
print '%s\t%s' % (current_word, current_count)
测试你的代码(cat data | map | sort | reduce)
我建议你在运行MapReduce job测试前尝试手工测试你的mapper.py 和 reducer.py脚本,以免得不到任何返回结果
这里有一些建议,关于如何测试你的Map和Reduce的功能:
hadoop@derekUbun:/usr/local/hadoop$ echo "foo foo quux labs foo bar quux" | ./mapper.py
foo 1
foo 1
quux 1
labs 1
foo 1
bar 1
quux 1
hadoop@derekUbun:/usr/local/hadoop$ echo "foo foo quux labs foo bar quux" |./mapper.py | sort |./reducer.py
bar 1
foo 3
labs 1
quux 2
using one of the ebooks as example input
(see below on where to get the ebooks)
hadoop@derekUbun:/usr/local/hadoop$ cat book/book.txt |./mapper.pysubscribe 1
to 1
our 1
email 1
newsletter 1
to 1
hear 1
about 1
new 1
eBooks. 1
在Hadoop平台上运行Python脚本
为了这个例子,我们将需要一本电子书,把它放在/usr/local/hadpoop/book/book.txt之下
hadoop@derekUbun:/usr/local/hadoop$ ls -l book
总用量 636
-rw-rw-r-- 1 derek derek 649669 3月 12 12:22 book.txt
复制本地数据到HDFS
在我们运行MapReduce job 前,我们需要将本地的文件复制到HDFS中:
hadoop@derekUbun:/usr/local/hadoop$ hadoop dfs -copyFromLocal /usr/local/hadoop/book book
hadoop@derekUbun:/usr/local/hadoop$ hadoop dfs -ls
Found 3 items
drwxr-xr-x - hadoop supergroup 0 2013-03-12 15:56 /user/hadoop/book
执行 MapReduce job现在,一切准备就绪,我们将在运行Python MapReduce job 在Hadoop集群上。像我上面所说的,我们使用的是HadoopStreaming 帮助我们传递数据在Map和Reduce间并通过STDIN和STDOUT,进行标准化输入输出。
hadoop@derekUbun:/usr/local/hadoop$hadoop jar contrib/streaming/hadoop-streaming-1.1.2.jar
-mapper /usr/local/hadoop/mapper.py
-reducer /usr/local/hadoop/reducer.py
-input book/\*
-output book-output
在运行中,如果你想更改Hadoop的一些设置,如增加Reduce任务的数量,你可以使用“-jobconf”选项:
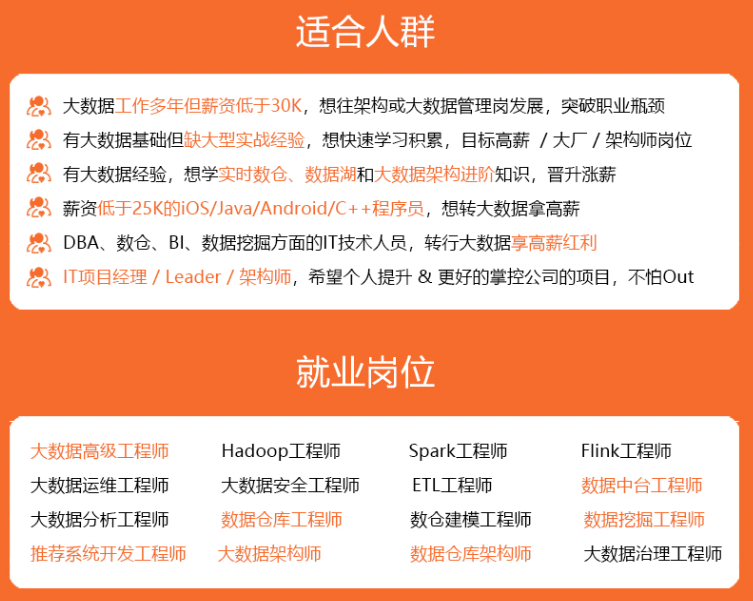
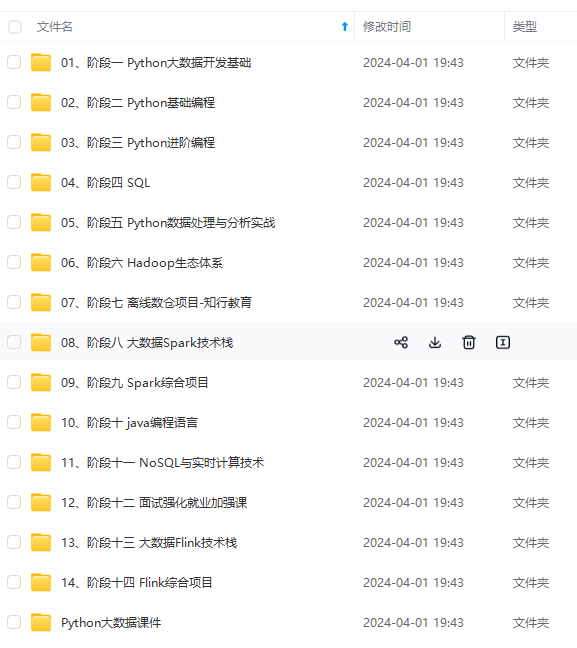
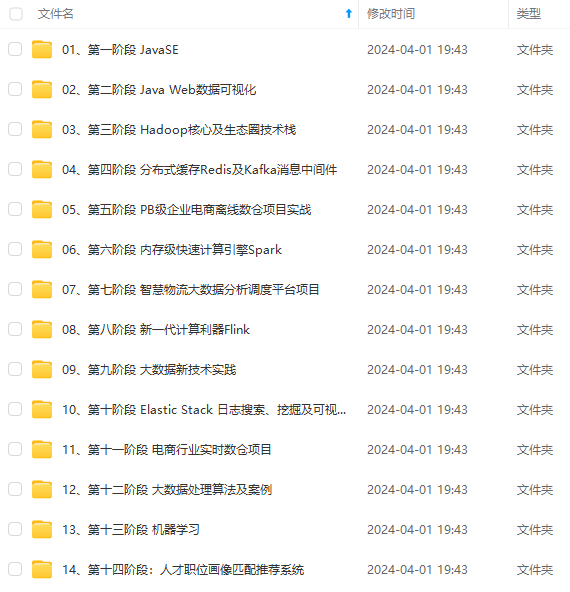
**既有适合小白学习的零基础资料,也有适合3年以上经验的小伙伴深入学习提升的进阶课程,涵盖了95%以上大数据知识点,真正体系化!**
**由于文件比较多,这里只是将部分目录截图出来,全套包含大厂面经、学习笔记、源码讲义、实战项目、大纲路线、讲解视频,并且后续会持续更新**
**[需要这份系统化资料的朋友,可以戳这里获取](https://bbs.csdn.net/topics/618545628)**
-1714747079175)]
[外链图片转存中...(img-QAGffpNO-1714747079175)]
**既有适合小白学习的零基础资料,也有适合3年以上经验的小伙伴深入学习提升的进阶课程,涵盖了95%以上大数据知识点,真正体系化!**
**由于文件比较多,这里只是将部分目录截图出来,全套包含大厂面经、学习笔记、源码讲义、实战项目、大纲路线、讲解视频,并且后续会持续更新**
**[需要这份系统化资料的朋友,可以戳这里获取](https://bbs.csdn.net/topics/618545628)**