作者 | Latte拿铁 编辑 | 自动驾驶之心
原文链接:https://zhuanlan.zhihu.com/p/29066299772
点击下方卡片,关注“自动驾驶之心”公众号
>>点击进入→自动驾驶之心『大模型』技术交流群
本文只做学术分享,如有侵权,联系删文
本文提出了一种新颖的高斯变换器(GaussTR),通过与大模型对齐,推动自监督的三维空间理解。该方法促进了多功能三维表示的学习,并实现了无需显式标注的开放词汇占据预测。
GaussTR: Foundation Model-Aligned Gaussian Transformer for Self-Supervised 3D Spatial Understanding
Haoyi Jiang, Liu Liu, Tianheng Cheng, Xinjie Wang, Tianwei Lin, Zhizhong Su, Wenyu Liu, Xinggang Wang
3D Semantic Occupancy Prediction is fundamental for spatial understanding as it provides a comprehensive semantic cognition of surrounding environments. However, prevalent approaches primarily rely on extensive labeled data and computationally intensive voxel-based modeling, restricting the scalability and generalizability of 3D representation learning. In this paper, we introduce GaussTR, a novel Gaussian Transformer that leverages alignment with foundation models to advance self-supervised 3D spatial understanding. GaussTR adopts a Transformer architecture to predict sparse sets of 3D Gaussians that represent scenes in a feed-forward manner. Through aligning rendered Gaussian features with diverse knowledge from pre-trained foundation models, GaussTR facilitates the learning of versatile 3D representations and enables open-vocabulary occupancy prediction without explicit annotations. Empirical evaluations on the Occ3D-nuScenes dataset showcase GaussTR's state-of-the-art zero-shot performance, achieving 11.70 mIoU while reducing training duration by approximately 50%. These experimental results highlight the significant potential of GaussTR for scalable and holistic 3D spatial understanding, with promising implications for autonomous driving and embodied agents. Code is available at this https URL.
三维语义占据预测是空间理解的基础,因为它提供了对周围环境的全面语义认知。然而,现有方法主要依赖于大量标注数据和计算密集型的体素建模,限制了三维表示学习的可扩展性和泛化能力。本文提出了一种新颖的高斯变换器(GaussTR),通过与大模型对齐,推动自监督的三维空间理解。GaussTR采用变换器架构,以前馈方式预测表示场景的稀疏三维高斯集合。通过将渲染的高斯特征与预训练大模型的多样化知识对齐,GaussTR促进了多功能三维表示的学习,并实现了无需显式标注的开放词汇占据预测。在Occ3D-nuScenes数据集上的实验评估表明,GaussTR在零样本性能上达到了领先水平,取得了11.70的mIoU,同时将训练时间缩短了约50%。这些实验结果凸显了GaussTR在可扩展和整体三维空间理解方面的巨大潜力,对自动驾驶和具身智能体具有重要的应用前景。代码可在https://github.com/hustvl/GaussTR获取。
Subjects: | Computer Vision and Pattern Recognition (cs.CV) |
---|---|
Cite as: | arXiv:2412.13193 [cs.CV] |
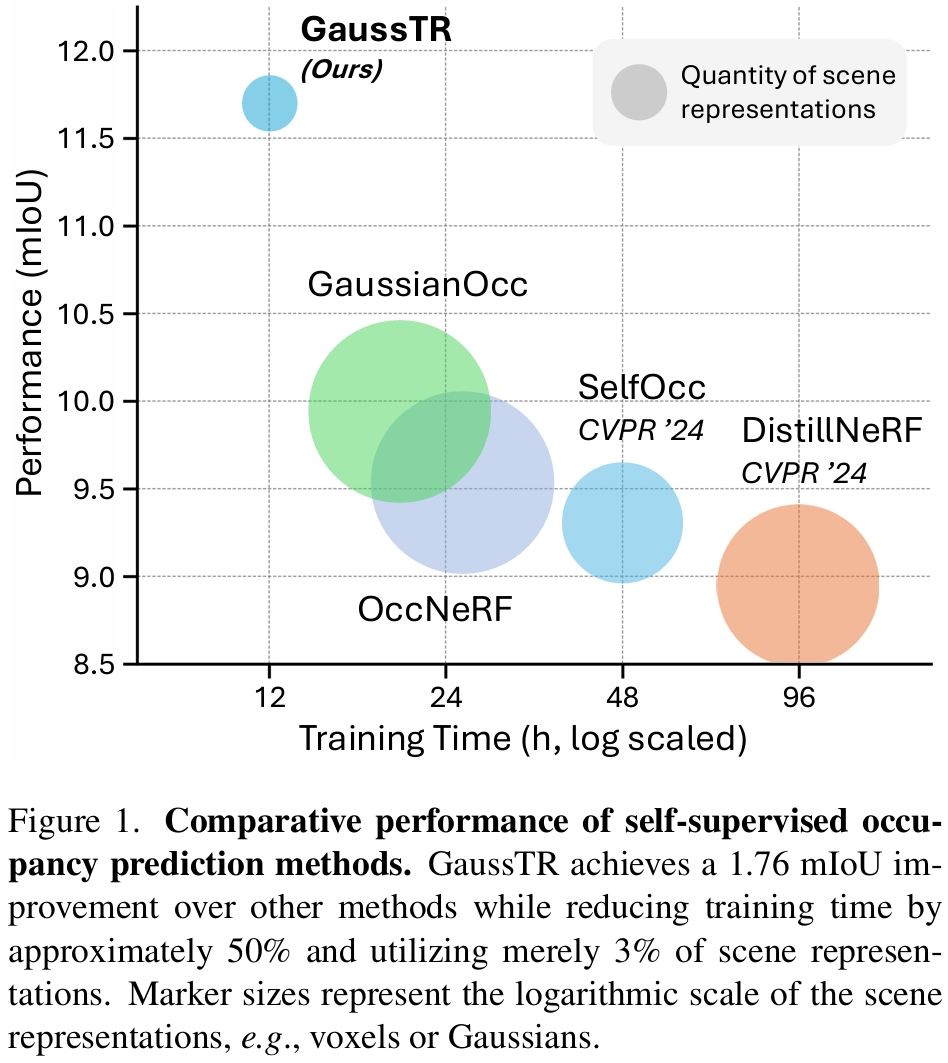
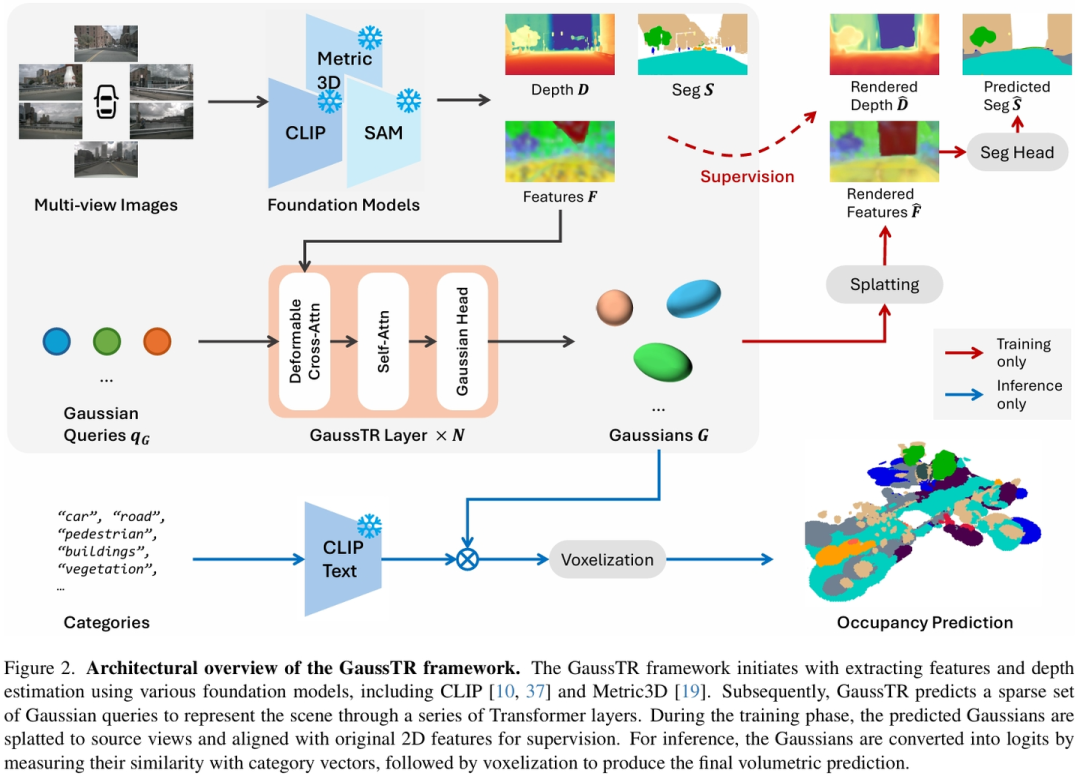
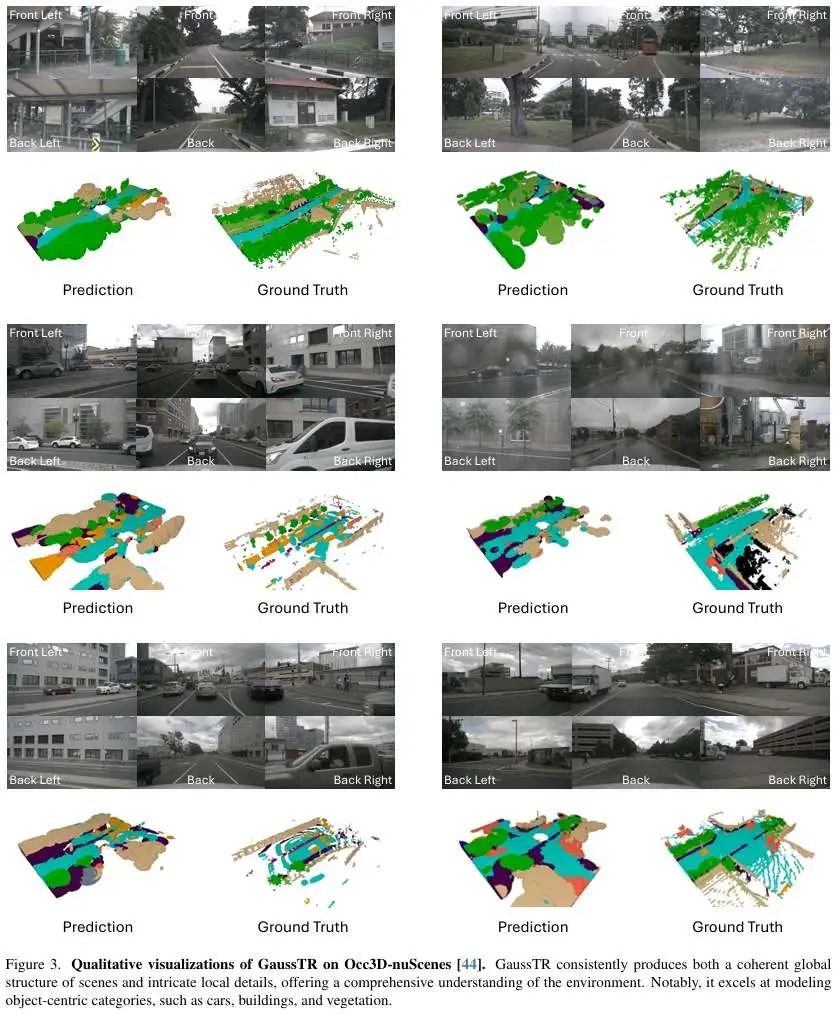
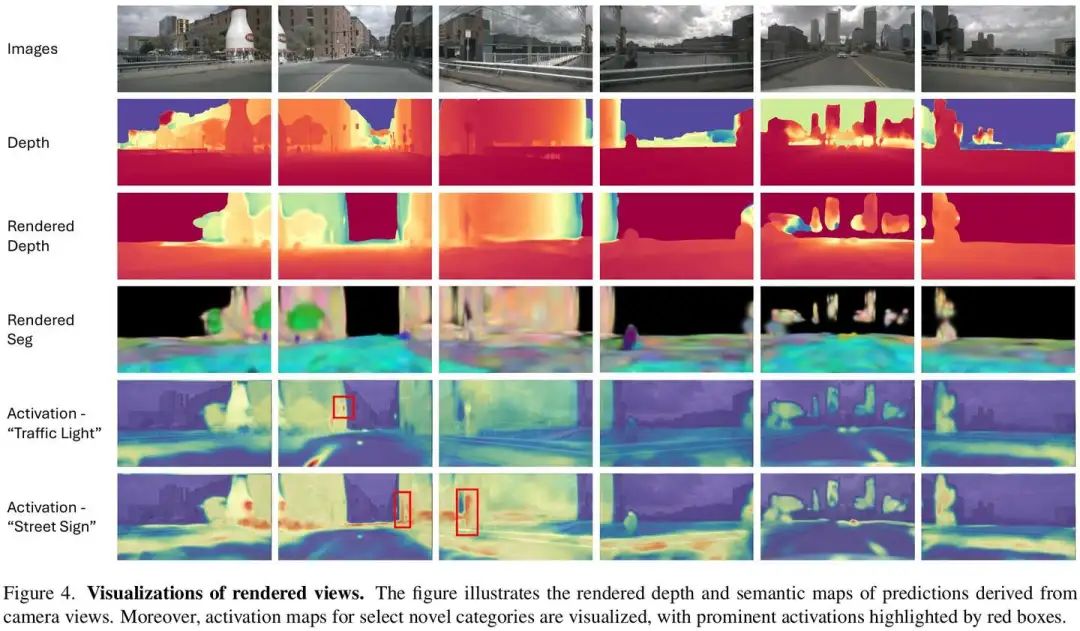
① 自动驾驶论文辅导来啦
② 国内首个自动驾驶学习社区
『自动驾驶之心知识星球』近4000人的交流社区,已得到大多数自动驾驶公司的认可!涉及30+自动驾驶技术栈学习路线,从0到一带你入门自动驾驶感知(端到端自动驾驶、世界模型、仿真闭环、2D/3D检测、语义分割、车道线、BEV感知、Occupancy、多传感器融合、多传感器标定、目标跟踪)、自动驾驶定位建图(SLAM、高精地图、局部在线地图)、自动驾驶规划控制/轨迹预测等领域技术方案、大模型,更有行业动态和岗位发布!欢迎扫描加入

③全网独家视频课程
端到端自动驾驶、仿真测试、自动驾驶C++、BEV感知、BEV模型部署、BEV目标跟踪、毫米波雷达视觉融合、多传感器标定、多传感器融合、多模态3D目标检测、车道线检测、轨迹预测、在线高精地图、世界模型、点云3D目标检测、目标跟踪、Occupancy、CUDA与TensorRT模型部署、大模型与自动驾驶、NeRF、语义分割、自动驾驶仿真、传感器部署、决策规划、轨迹预测等多个方向学习视频(扫码即可学习)
④【自动驾驶之心】全平台矩阵