Mapreduce的相关概念
分布式并行离线计算框架MapReduce
即
如果文件里有三句话
hadoop is nice
hadoop good
hadoop is better
那么Map做的工作是
hadoop 1
is 1
nice 1
hadoop 1
good 1
hadoop 1
is 1
better
然后Reduce做的是合并
hadoop 3
is 2
nice 1
good 1
better 1
(我没排序 好像输出时是排过序的 貌似是按首字母排序)
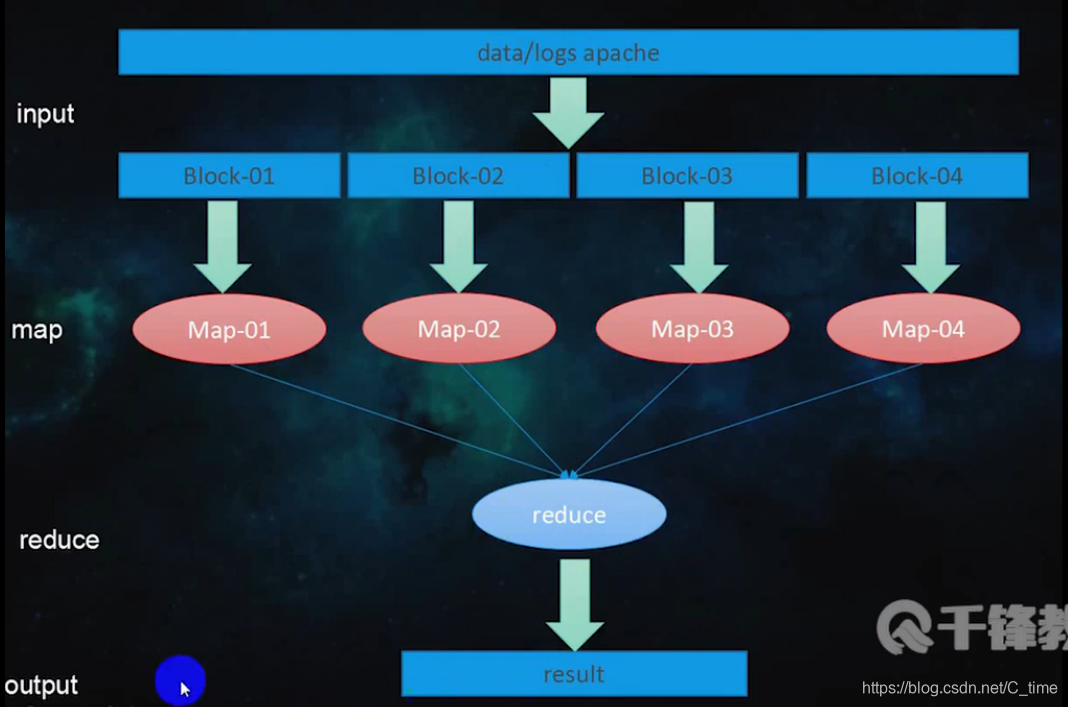
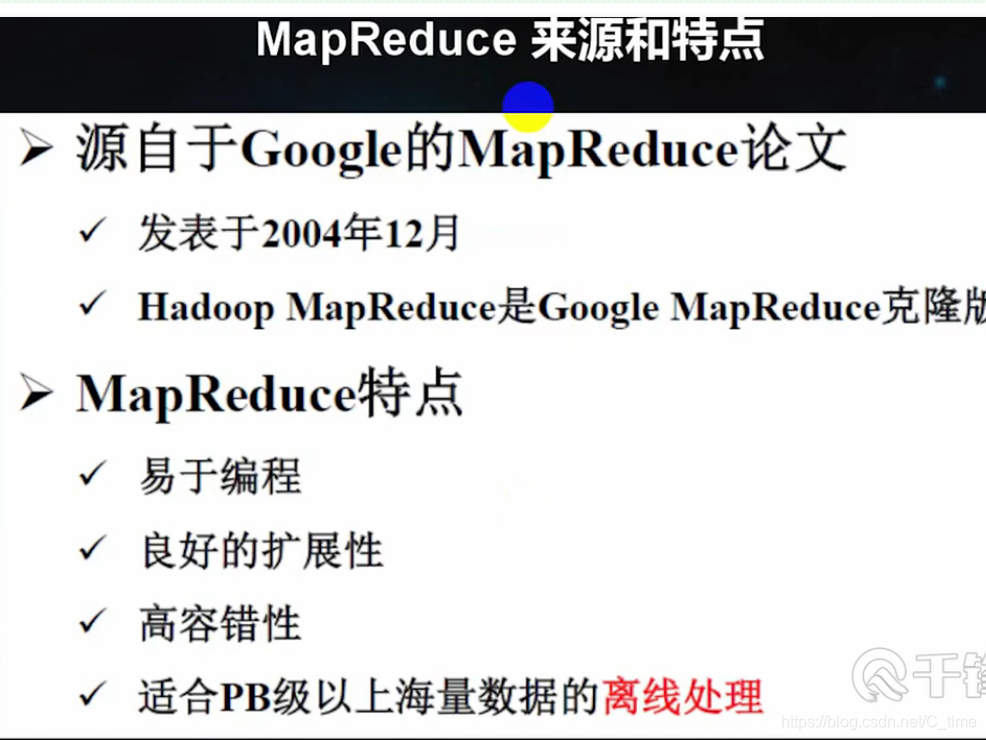
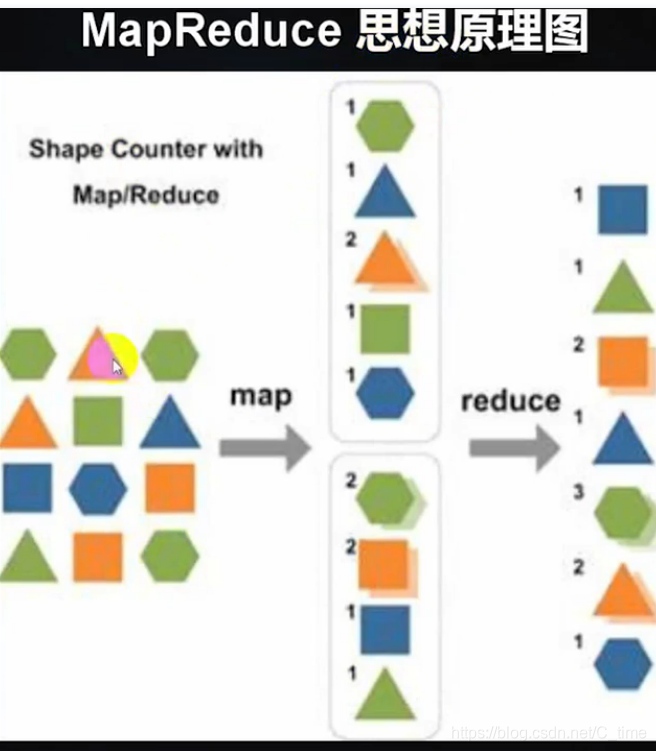
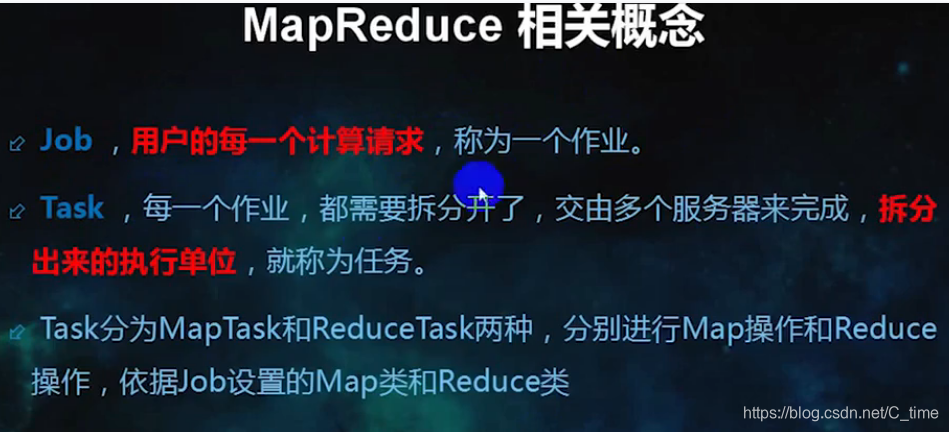
实例
前边输入输出都做过
然后调用代码的话现在我们就不用示例的了 用我们自己编写的代码来实现
按住shift alt s 选择 override / Implements Methods 重写map方法
注意使用第一个不要使用第二个
用第二个包
第一个短的包是hadoop 1.x的
右键 点击Export 导出普通的jar包
然后就下一步下一步就可以了
这个地方选主类 要选main
将文件通过shell拖到home目录后
再上传到hdfs文件系统
不过需要启动服务 start-all.sh start-dfs.sh 我也不知道对不对. 不过没启动前输入命令是报错的
hdfs dfs -put /home/words /mywords
注意输出目录时提前看看out下有啥 别重名了
hdfs dfs -ls /out
jar包位置 包名加类名写清楚 数据地址 输出结果地址
yarn jar /home/wc.jar qf.com.mr.MyWordCount /my /tmp/10
hdfs dfs -cat /tmp/10/part-r-00000
出了好多错误
搞了好长时间
最后重启了一下服务
竟然好了
我也没太搞明白哪里错了 可能是我的服务有问题 说是连不上rm2 重启好了
package qf.com.mr;
import java.io.IOException;
import org.apache.hadoop.conf.Configuration;
import org.apache.hadoop.fs.Path;
import org.apache.hadoop.io.IntWritable;
import org.apache.hadoop.io.LongWritable;
import org.apache.hadoop.io.Text;
import org.apache.hadoop.mapreduce.Job;
import org.apache.hadoop.mapreduce.Mapper;
import org.apache.hadoop.mapreduce.Reducer;
import org.apache.hadoop.mapreduce.lib.input.FileInputFormat;
import org.apache.hadoop.mapreduce.lib.output.FileOutputFormat;
/*
*@author Shishuai E-mail:1198319583@qq.com
*@version Create time : 2019年5月27日下午3:33:00
*类说明:自定义wordcount(词频统计)
*
*input
*
*words
hello qianfeng hello word
hi qianfeng hi world
best best best
*
*map阶段
*行偏移量:每一行的第一个字母距离该文件的首位置的距离
*
*
*Mapper<KEYIN, VALUEIN, KEYOUT, VALUEOUT>
*
* KEYIN 行偏移量(map阶段输入key类型)
* VALUEIN map阶段输入value类型
* KEYOUT map阶段输出key的类型
* VALUEOUT map阶段输出value的类型
*map阶段的输入数据:
*0 hello qianfeng hello word
*27 hi qianfeng hi world
*47 best best best
*
*map阶段的输出数据:
*best 1
*best 1
*best 1
*hello 1
*hello 1
*hi 1
*hi 1
*qianfeng 1
*qianfeng 1
*world 1
*world 1
*
*
*reduce阶段的输入:
*
*Reducer<KEYIN, VALUEIN, KEYOUT, VALUEOUT>
*
*KEYIN reduce阶段输入key类型(必须和map阶段的输出key类型相同)
*VALUEIN reduce阶段输入value类型(必须和map阶段的输出value类型相同)
*KEYOUT reduce阶段最终输出的key类型
*VALUEOUT reduce阶段最终输出的value类型
*
*reduce阶段的输入数据:
*best list<1, 1, 1>
*hello list<1, 1>
*hi list<1, 1>
*qianfeng list<1, 1>
*world list<1, 1>
*
*reduce阶段的输出:
*best 3
*hello 2
*hi 2
*qianfeng 2
*world 2
*/
public class MyWordCount {
public static class MyMapper extends Mapper<LongWritable, Text, Text, IntWritable>{
public static Text k = new Text();
public static IntWritable v = new IntWritable();
@Override
protected void map(LongWritable key, Text value, Mapper<LongWritable, Text, Text, IntWritable>.Context context)
throws IOException, InterruptedException {
//1.从输入数据中获取每一个文件中的每一行的值
String line = value.toString();
//2.对每一行的数据进行切分(有的不用)
String [] words = line.split(" ");
//3.循环处理
for (String word : words) {
k.set(word);
v.set(1);
//map阶段的输出 context上下文环境变量
context.write(k, v);//这个输出在循环里面 有一个输出一个
}
}
}
public static class MyReducer extends Reducer<Text, IntWritable, Text, IntWritable>{
@Override
protected void reduce(Text key, Iterable<IntWritable> values,
Context context) throws IOException, InterruptedException {
//1.自定义一个计数器
int counter = 0;
for (IntWritable i : values) {
counter += i.get();
}
//2.reduce阶段的最终输出
context.write(key, new IntWritable(counter));
//这个输出在循环外面 等统计完了这一个容器再输出
}
}
//驱动
public static void main(String[] args) throws IOException, ClassNotFoundException, InterruptedException {
//1.获取配置对象信息
Configuration conf = new Configuration();
//2.对conf进行设置(没有就不用)
//3.获取job对象 (注意导入的包)
Job job = Job.getInstance(conf, "mywordcount");
//4.设置job的运行主类
job.setJarByClass(MyWordCount.class);
System.out.println("jiazai finished");
//5.对map阶段进行设置
job.setMapperClass(MyMapper.class);
job.setMapOutputKeyClass(Text.class);
job.setMapOutputValueClass(IntWritable.class);
FileInputFormat.addInputPath(job, new Path(args[0]));//具体路径从控制台输入
System.out.println("map finished");
//6.对reduce阶段进行设置
job.setReducerClass(MyReducer.class);
job.setOutputKeyClass(Text.class);
job.setOutputValueClass(IntWritable.class);
FileOutputFormat.setOutputPath(job, new Path(args[1]));
System.out.println("reduce finished");
//7.提交job并打印信息
int isok = job.waitForCompletion(true) ? 0 : 1;
//退出job
System.exit(isok);
System.out.println("all finished");
}
}
MapReduce数学运算案例
- 类说明:将汽车生产流水线的三个时间段的记录算一个平均值
package qf.com.mr;
import java.io.IOException;
import org.apache.hadoop.conf.Configuration;
import org.apache.hadoop.fs.Path;
import org.apache.hadoop.io.FloatWritable;
import org.apache.hadoop.io.LongWritable;
import org.apache.hadoop.io.Text;
import org.apache.hadoop.mapreduce.Job;
import org.apache.hadoop.mapreduce.Mapper;
import org.apache.hadoop.mapreduce.Reducer;
import org.apache.hadoop.mapreduce.lib.input.FileInputFormat;
import org.apache.hadoop.mapreduce.lib.output.FileOutputFormat;
/*
*@author Shishuai E-mail:1198319583@qq.com
*@version Create time : 2019年5月27日下午10:10:20
*类说明:将汽车生产流水线的三个时间段的记录算一个平均值
*
*l z w y
*L_1 393 430 276
*L_2 388 560 333
*L_3 450 600 312
*
*生产线 生产平均值
*L_1 *
*L_2 *
*/
public class Avg {
public static class MyMapper extends Mapper<LongWritable, Text, Text, FloatWritable>{
public static Text k = new Text();
public static FloatWritable v = new FloatWritable();
//setup 只在map阶段执行之前执行一次(用于初始化等一次性操作)
@Override
protected void setup(Mapper<LongWritable, Text, Text, FloatWritable>.Context context)
throws IOException, InterruptedException {
}
@Override
protected void map(LongWritable key, Text value, Mapper<LongWritable, Text, Text, FloatWritable>.Context context)
throws IOException, InterruptedException {
//1.从输入数据中获取每一个文件中的每一行的值
String line = value.toString();
//2.对每一行的数据进行切分(有的不用)
String [] words = line.split("\t");
//3.按照业务循环处理
String lineName = words[0];
int z = Integer.parseInt(words[1]);
int w = Integer.parseInt(words[2]);
int y = Integer.parseInt(words[3]);
k.set(lineName);
float avg = (float)((z+w+y)*1.0/(words.length-1));
v.set(avg);
context.write(k, v);
}
//在整个map执行之后 一般作用于最后的结果
@Override
protected void cleanup(Mapper<LongWritable, Text, Text, FloatWritable>.Context context)
throws IOException, InterruptedException {
}
}
public static class MyReducer extends Reducer<Text, FloatWritable, Text, Text>{
//在reduce阶段执行之前 执行 仅一次
@Override
protected void setup(Reducer<Text, FloatWritable, Text, Text>.Context context)
throws IOException, InterruptedException {
context.write(new Text("生产线 生产平均值"), new Text(""));
}
@Override
protected void reduce(Text key, Iterable<FloatWritable> values, Context context)
throws IOException, InterruptedException {
context.write(key, new Text(values.iterator().next().get()+""));
}
//在reduce执行之后执行 仅执行一次
@Override
protected void cleanup(Reducer<Text, FloatWritable, Text, Text>.Context context)
throws IOException, InterruptedException {
}
}
//驱动
public static void main(String[] args) throws IOException, ClassNotFoundException, InterruptedException {
//1.获取配置对象信息
Configuration conf = new Configuration();
//2.对conf进行设置(没有就不用)
//3.获取job对象 (注意导入的包)
Job job = Job.getInstance(conf, "myAvg");
//4.设置job的运行主类
job.setJarByClass(Avg.class);
//System.out.println("jiazai finished");
//5.对map阶段进行设置
job.setMapperClass(MyMapper.class);
job.setMapOutputKeyClass(Text.class);
job.setMapOutputValueClass(FloatWritable.class);
FileInputFormat.addInputPath(job, new Path(args[0]));//具体路径从控制台输入
//System.out.println("map finished");
//6.对reduce阶段进行设置
job.setReducerClass(MyReducer.class);
job.setOutputKeyClass(Text.class);
job.setOutputValueClass(Text.class);
FileOutputFormat.setOutputPath(job, new Path(args[1]));
//System.out.println("reduce finished");
//7.提交job并打印信息
int isok = job.waitForCompletion(true) ? 0 : 1;
//退出job
System.exit(isok);
System.out.println("all finished");
}
}
上述代码 打包出来 拖到home下
然后在01下写个名为avg文件 间隔必须是tab键 打出来的 因为代码分隔使用 \t
vi /home/avg
然后hdfs dfs -put /avg 上传到hdfs上使用
yarn jar /home/wc.jar qf.com.mr.Avg /avg /out/avg1
hdfs dfs -cat /out/avg1/part-r-00000
Awk和MapReduce的处理方式比较
awk是shell下一种命令
awk使用格式
例子 找etc下的passwd的内容大于500的
我们先看看这个目录下有啥
就找每行第一组数字大于等于500的
1.用cat和管道的
cat /etc/passwd | awk -F ':' 'BEGIN{print "名字\t用户ID" } {if($3 >= 500) print $1, $3}'
2.不用cat和管道 在后面直接写路径
awk -F ':' 'BEGIN{print "名字\t用户ID" } {if($3 >= 500) print $1, $3}' /etc/passwd
然后我们再做一些刚刚MapReduce做的事情
每一条生产线的平均值
NF是每一行的列数 这avg这个文件中 每一行的列数都是4
awk -F '\t' 'BEGIN{print "生产线\t生产平均值" } {print $1, ($2+$3+$4)/(NF-1)}' /home/avg
挺快的
所以说
少量数据或者做数据验证的时候
用awk的这个shell工具脚本比较方便
但awk不能处理海量的数据