def compute_pca(X, n_components=2):
"""
Input:
X: of dimension (m,n) where each row corresponds to a word vector
n_components: Number of components you want to keep.
Output:
X_reduced: data transformed in 2 dims/columns + regenerated original data
"""
### START CODE HERE (REPLACE INSTANCES OF 'None' with your code) ###
# mean center the data
X_demeaned = X - np.mean(X,axis=0)
# calculate the covariance matrix
covariance_matrix = np.cov(X_demeaned,rowvar=0)
# calculate eigenvectors & eigenvalues of the covariance matrix
eigen_vals, eigen_vecs = np.linalg.eigh(covariance_matrix)
# sort eigenvalue in increasing order (get the indices from the sort)
idx_sorted = np.argsort(eigen_vals)
# reverse the order so that it's from highest to lowest.
idx_sorted_decreasing = idx_sorted[::-1]
# sort the eigen
PCA降维
最新推荐文章于 2024-06-17 10:56:02 发布
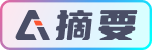