扩散模型 (Diffusion models) 是「生成模型」算法领域中的一个相对较新的算法。生成模型通过学习大量训练样本(例如图像或者音频),创建一些与训练数据神似,但又不是完全相同的多样化输出。
Diffusers 库是一个操作扩散模型的工具箱,由 Hugging Face 团队发布,它可以非常方便的使用各种扩散模型生成图像、音频,也可以非常方便的使用各种噪声调度器,用于调节在模型推理中的速度和质量,同时,这个库也支持多种类型的模型。
上周,Diffusers 发布了 v0.9.0 版,正式支持了最新的 Stable Diffusion 2,Stable Diffusion 可以简要理解为是一个自然语言生成图片的模型,并于上周发布了 2.0 版本,可以生成 768x768 和 512x512 分辨率的图片,也包括了一个 Upscaler Diffusion 模型,可以将图片分辨率升级为 2048x2048 甚至更高,更多关于 Stable Diffusion 2 的更新,请阅读 机器之心的文章 了解更多。
你可以在 Hugging Face Spaces 上体验 Stable Diffusion 2:
https://huggingface.co/spaces/stabilityai/stable-diffusion
差一点忘记我们的「正事儿」——介绍 Diffusers 的更新,下面开始正文部分内容:
🎨 安装最新版 Diffusers v0.9.0 体验 Stable Diffusion 2
pip install diffusers[torch]==0.9 transformers
Diffusers 支持 Stable Diffusion 2 中的多种模型,我们将在后面的章节介绍用法以及示例代码。
基于 768x768 图像的 Stable Diffusion 2.0-V
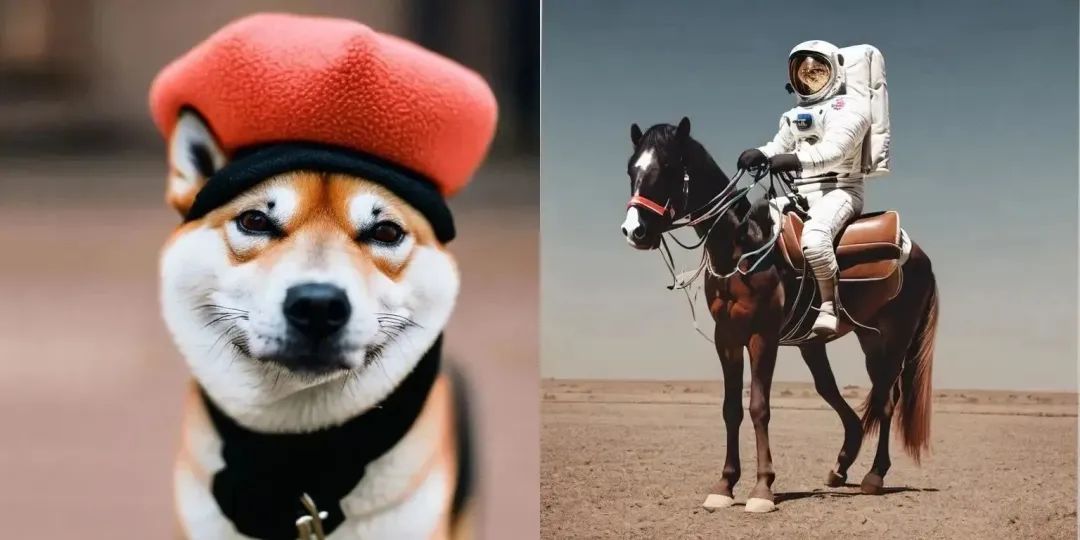
最新的基于 768x768 大小图像的稳定扩散模型:Stable Diffusion 2.0-V,它的参数数量为 U-Net 模型的 1.5 倍,但采用了 OpenCLIP-ViT/H 作为文本编码器从头开始训练,因此 2.0-V 也被称为:v-prediction 模型。
OpenCLIP-ViT/H:
https://github.com/mlfoundations/open_clipv-prediction 模型:
https://arxiv.org/abs/2202.00512
import torch
from diffusers import DiffusionPipeline, DPMSolverMultistepScheduler
repo_id = "stabilityai/stable-diffusion-2"
pipe = DiffusionPipeline.from_pretrained(repo_id, torch_dtype=torch.float16, revision="fp16")
pipe.scheduler = DPMSolverMultistepScheduler.from_config(pipe.scheduler.config)
pipe = pipe.to("cuda")
prompt = "High quality photo of an astronaut riding a horse in space"
image = pipe(prompt, guidance_scale=9, num_inference_steps=25).images[0]
image.save("astronaut.png")
基于 512x512 图像的 Stable Diffusion 2.0-base
上面的模型是基于 SD 2.0-base 进行微调而来,SD 2.0-base 在 512x512 图像数据集上被训练为标准的噪声预测模型,当然 base 模型在我们的平台上也是支持的。
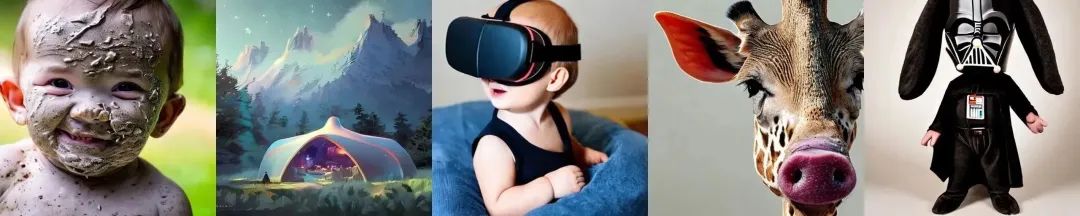
import torch
from diffusers import DiffusionPipeline, DPMSolverMultistepScheduler
repo_id = "stabilityai/stable-diffusion-2-base"
pipe = DiffusionPipeline.from_pretrained(repo_id, torch_dtype=torch.float16, revision="fp16")
pipe.scheduler = DPMSolverMultistepScheduler.from_config(pipe.scheduler.config)
pipe = pipe.to("cuda")
prompt = "High quality photo of an astronaut riding a horse in space"
image = pipe(prompt, num_inference_steps=25).images[0]
image.save("astronaut.png")
Stable Diffusion 2.0 用于图像修补 (Inpanting)
该模型用于文本引导的图像修补,它同样基于 SD 2.0-base 进行微调,遵循 LAMA 中提出的掩码生成策略 (mask-generation strategy),并结合掩码图像 (masked image) 的隐式 VAE 表示。
LAMA:
https://github.com/saic-mdal/lama
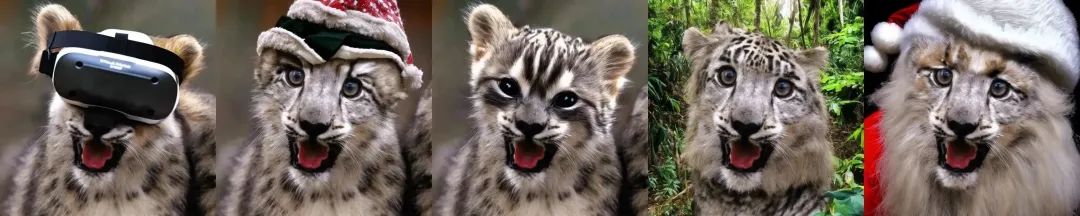
import PIL
import requests
import torch
from io import BytesIO
from diffusers import DiffusionPipeline, DPMSolverMultistepScheduler
def download_image(url):
response = requests.get(url)
return PIL.Image.open(BytesIO(response.content)).convert("RGB")
img_url = "https://raw.githubusercontent.com/CompVis/latent-diffusion/main/data/inpainting_examples/overture-creations-5sI6fQgYIuo.png"
mask_url = "https://raw.githubusercontent.com/CompVis/latent-diffusion/main/data/inpainting_examples/overture-creations-5sI6fQgYIuo_mask.png"
init_image = download_image(img_url).resize((512, 512))
mask_image = download_image(mask_url).resize((512, 512))
repo_id = "stabilityai/stable-diffusion-2-inpainting"
pipe = DiffusionPipeline.from_pretrained(repo_id, torch_dtype=torch.float16, revision="fp16")
pipe.scheduler = DPMSolverMultistepScheduler.from_config(pipe.scheduler.config)
pipe = pipe.to("cuda")
prompt = "Face of a yellow cat, high resolution, sitting on a park bench"
image = pipe(prompt=prompt, image=init_image, mask_image=mask_image, num_inference_steps=25).images[0]
image.save("yellow_cat.png")
Stable Diffusion X4 超分辨率图像 (Upscaler)
这款模型在 512x512 的数据集上训练,并且是基于文本指导 (text-guided) 的隐式分辨率提高扩散器模型 (latent upscaling diffusion model)。
除了文本输入之外,它还接收一个 noise_level
作为输入参数,可用于根据预定义的扩散计划 (predefined diffusion schedule) 向输入的低分辨率图像添加噪声。
latent upscaling diffusion model:
https://arxiv.org/abs/2112.10752predefined diffusion schedule:
https://hf.co/stabilityai/stable-diffusion-x4-upscaler/blob/main/low_res_scheduler/scheduler_config.json
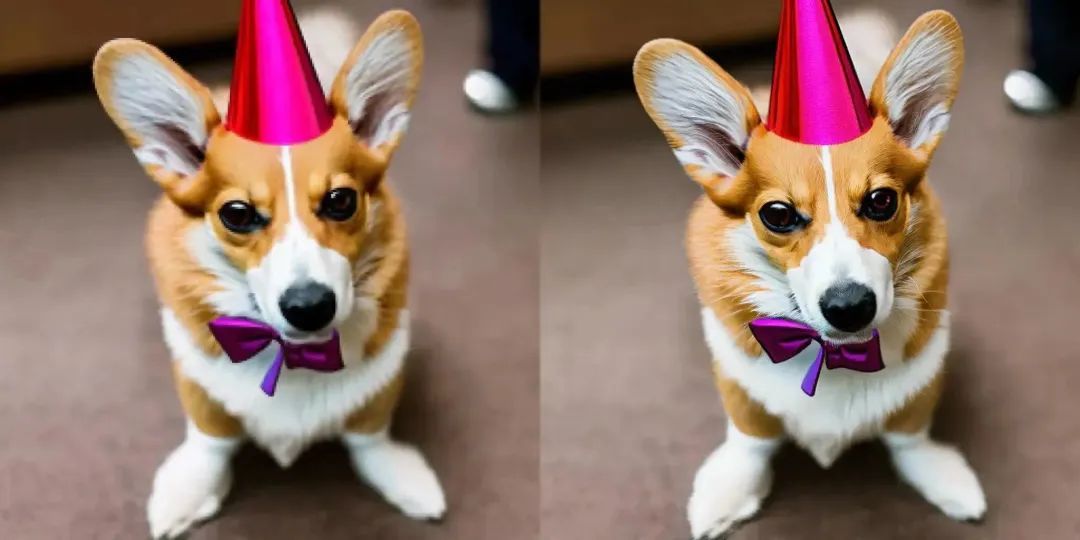
保存并加载多功能扩散器 (Versatile Diffusion) 的 bug 已经被修复
我们修复了之前在保存并加载多功能扩散器时出现的 bug ,以便保证大家更高效地工作。
以上就是本次 Diffusers v0.9.0 更新的内容,更详细的更新内容,欢迎点击阅读原文在 GitHub 上查阅,如果有任何发现的 Bug 和建议,欢迎你在 GitHub Issue 里向我们提出:
https://github.com/huggingface/diffusers/issues
正文部分译者:
丁继峥 Johnson,微信号:ZJUer_0817 拾象DAO成员,浙江大学机器人工程专业,主要关注 AI 模型与交互的前沿进展,专用机器人的产业落地,通用机器人的无限可能。
文章头图: Lynn
我们正在招募更多翻译志愿者帮助我们扩充官方公众号内容,如果你感兴趣,欢迎通过文章下方的留言功能介绍自己,并留下联系方式。谢谢!