gym-0.26.1
Pendulum-v1
环境详细信息
double DQN
实验环境 是为了体现
double DQN
对高估的缓解, 因为Pendulum-v1
reward
最大是为0
,可以有明显的对比。
相关论文 Deep Reinforcement Learning with Double Q-Learning
对动手深度强化学习里的代码做了一些修改。
代码如下:
import gym
import torch
from torch import nn
from torch.nn import functional as F
import numpy as np
import random
import collections
from tqdm import tqdm
import matplotlib.pyplot as plt
from d2l import torch as d2l
import rl_utils
class ReplayBuffer:
"""经验回放池"""
def __init__(self, capacity):
self.buffer = collections.deque(maxlen=capacity) # 队列,先进先出
def add(self, state, action, reward, next_state, done): # 将数据加入buffer
self.buffer.append((state, action, reward, next_state, done))
def sample(self, batch_size): # 从buffer中采样数据,数量为batch_size
transition = random.sample(self.buffer, batch_size)
state, action, reward, next_state, done = zip(*transition)
return np.array(state), action, reward, np.array(next_state), done
def size(self): # 目前buffer中数据的数量
return len(self.buffer)
class Q(nn.Module):
"""只有一层隐藏层的Q网络"""
def __init__(self, state_dim, hidden_dim, action_dim):
super().__init__()
self.fc1 = nn.Linear(state_dim, hidden_dim)
self.fc2 = nn.Linear(hidden_dim, action_dim)
def forward(self, X):
X = F.relu(self.fc1(X)) # 隐藏层之后使用ReLU激活函数
return self.fc2(X)
class DQN:
"""DQN算法,包括double DQN"""
def __init__(self, state_dim, hidden_dim, action_dim, lr, gamma, epsilon, target_update, device, dqn_type='DQN'):
self.action_dim = action_dim
self.q = Q(state_dim, hidden_dim, action_dim).to(device) # Q网络
self.target_q = Q(state_dim, hidden_dim, action_dim).to(device) # 目标网络
self.target_q.load_state_dict(self.q.state_dict()) # 加载参数
self.optimizer = torch.optim.Adam(self.q.parameters(), lr=lr)
self.gamma = gamma
self.epsilon = epsilon
self.target_update = target_update # 目标网络更新频率
self.count = 0 # 计数器,记录更新次数
self.device = device
self.dqn_type = dqn_type
def take_action(self, state): # epsilon-贪婪策略
if np.random.random() < self.epsilon:
action = np.random.randint(self.action_dim)
else:
state = torch.tensor(np.array([state]), dtype=torch.float).to(self.device)
action = self.q(state).argmax().item()
return action
def max_q_value(self, state):
state = torch.tensor(np.array([state]), dtype=torch.float).to(self.device)
return self.q(state).max().item()
def update(self, transition_dict):
states = torch.tensor(transition_dict['states'], dtype=torch.float).to(self.device)
actions = torch.tensor(transition_dict['actions']).reshape(-1,1).to(self.device)
rewards = torch.tensor(transition_dict['rewards'], dtype=torch.float).reshape(-1,1).to(self.device)
next_states = torch.tensor(transition_dict['next_states'], dtype=torch.float).to(self.device)
dones = torch.tensor(transition_dict['dones'], dtype=torch.float).reshape(-1,1).to(self.device)
q_values = self.q(states).gather(1, actions) # Q值
if self.dqn_type == "Double DQN":
max_action = self.q(next_states).max(1)[1].reshape(-1, 1)
max_next_q_values = self.target_q(next_states).gather(1, max_action)
else:
max_next_q_values = self.target_q(next_states).max(1)[0].reshape(-1,1)
q_targets = rewards + self.gamma * max_next_q_values * (1- dones) # TD误差
loss = F.mse_loss(q_values, q_targets) # 均方误差
self.optimizer.zero_grad() # 梯度清零,因为默认会梯度累加
loss.mean().backward() # 反向传播
self.optimizer.step() # 更新梯度
if self.count % self.target_update == 0:
self.target_q.load_state_dict(self.q.state_dict())
self.count += 1
def dis_to_con(discrete_action, env, action_dim): # 将离散动作转回连续的函数
action_lowbound= env.action_space.low[0] # 连续动作的最小值
action_upbound = env.action_space.high[0] # 连续动作的最大值
return action_lowbound + (discrete_action / (action_dim - 1) * (action_upbound - action_lowbound))
def train_DQN(agent, env, num_episodes, reply_buffer, miniaml_size, batch_size):
return_list = []
max_q_value_list = []
max_q_value = 0
for i in range(10):
with tqdm(total=int(num_episodes/10), desc=f'Iteration {i}') as pbar:
for i_episode in range(int(num_episodes/10)):
episode_return = 0
state = env.reset()[0]
done, truncated= False, False
while not done and not truncated :
action = agent.take_action(state)
max_q_value = agent.max_q_value(state) * 0.005 + max_q_value * 0.995 # 平滑处理
max_q_value_list.append(max_q_value) # 保存每个状态的最大Q值
action_continuous = dis_to_con(action, env, agent.action_dim)
next_state, reward, done, truncated, info = env.step([action_continuous])
replay_buffer.add(state, action, reward, next_state, done)
state = next_state
episode_return += reward
# 当buffer数据的数量超过一定值后,才进行Q网络训练
if replay_buffer.size() > minimal_size:
b_s, b_a, b_r, b_ns, b_d = replay_buffer.sample(batch_size)
transition_dict = {'states': b_s, 'actions': b_a, 'next_states': b_ns, 'rewards': b_r, 'dones': b_d}
agent.update(transition_dict)
return_list.append(episode_return)
if (i_episode+1) % 10 == 0:
pbar.set_postfix({'episode': '%d' % (num_episodes / 10 * i + i_episode+1),
'return': '%.3f' % np.mean(return_list[-10:])})
pbar.update(1)
episodes_list = list(range(len(return_list)))
mv_return = rl_utils.moving_average(return_list, 5)
plt.plot(episodes_list, mv_return)
plt.xlabel('Episodes')
plt.ylabel('Returns')
plt.title(f'{agent.dqn_type} on {env_name}')
plt.show()
frames_list = list(range(len(max_q_value_list)))
plt.plot(frames_list, max_q_value_list)
plt.axhline(0, c='orange', ls='--')
plt.axhline(10, c='red', ls='--')
plt.xlabel('Frames')
plt.ylabel('Q value')
plt.title(f'{agent.dqn_type} on {env_name}')
plt.show()
lr = 1e-2
num_episodes = 200
hidden_dim = 128
gamma = 0.98
epsilon = 0.01
target_update = 50
buffer_size = 5000
minimal_size = 1000
batch_size = 64
device = d2l.try_gpu()
print(device)
env_name = "Pendulum-v1"
env = gym.make(env_name)
state_dim = env.observation_space.shape[0]
action_dim = 11 # 把连续动作分解成11个离散动作
random.seed(0)
np.random.seed(0)
torch.manual_seed(0)
replay_buffer = ReplayBuffer(buffer_size)
agent = DQN(state_dim, hidden_dim, action_dim, lr, gamma, epsilon, target_update, device)
train_DQN(agent, env, num_episodes, replay_buffer, minimal_size, batch_size)
random.seed(0)
np.random.seed(0)
torch.manual_seed(0)
replay_buffer = ReplayBuffer(buffer_size)
agent = DQN(state_dim, hidden_dim, action_dim, lr, gamma, epsilon, target_update, device,"Double DQN")
train_DQN(agent, env, num_episodes, replay_buffer, minimal_size, batch_size)
在jupyter
中运行,结果如下:
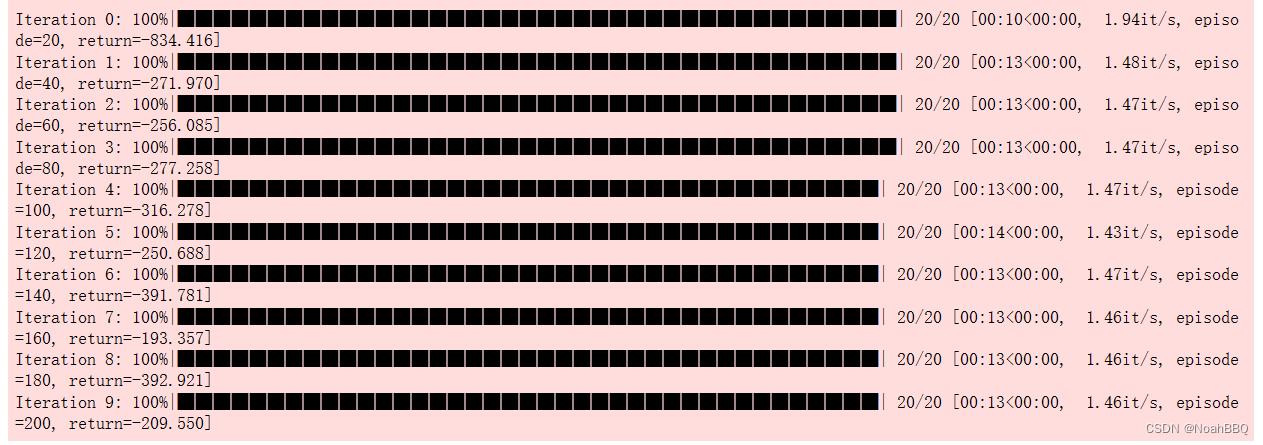
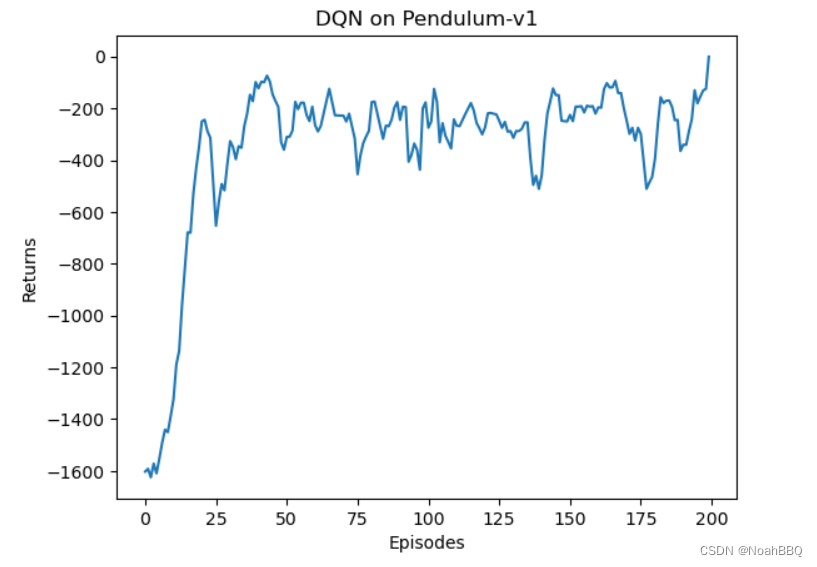
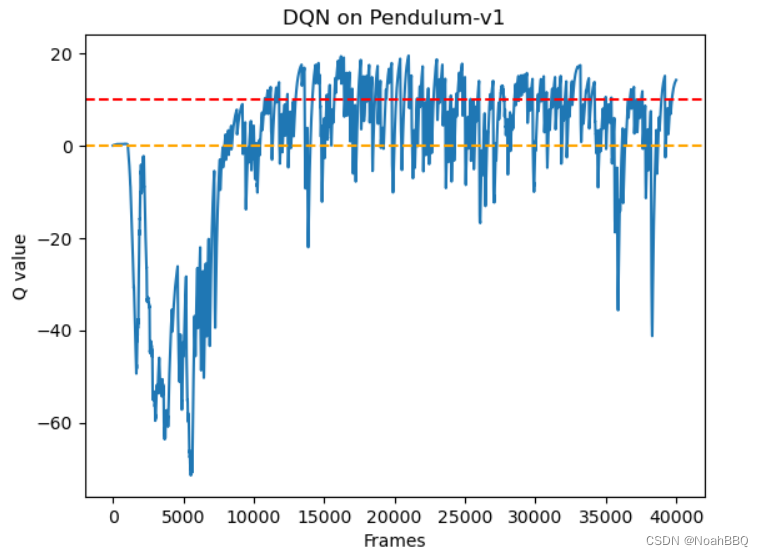
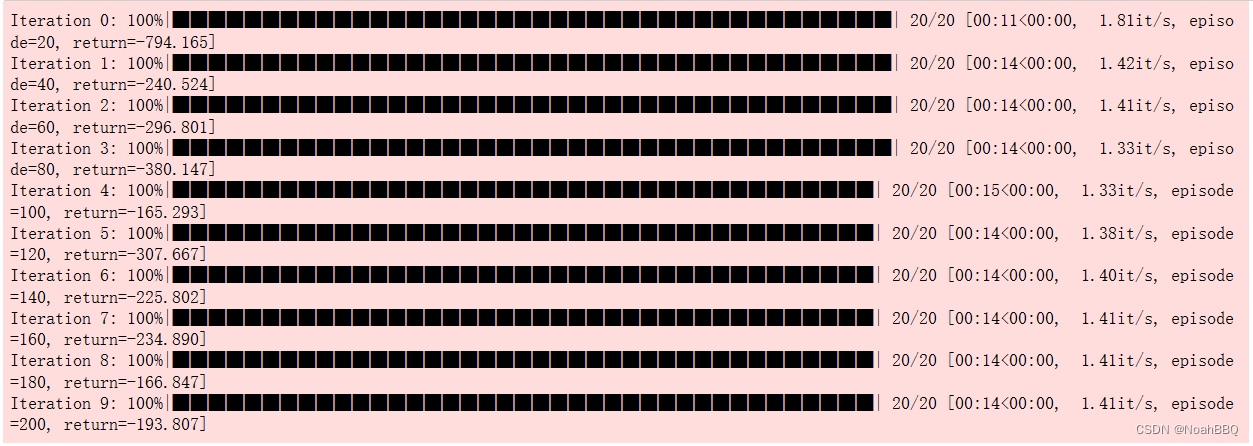
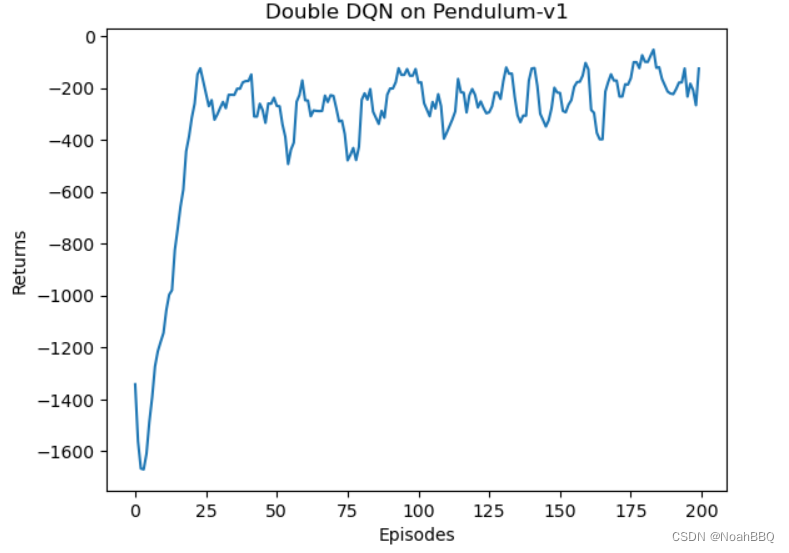
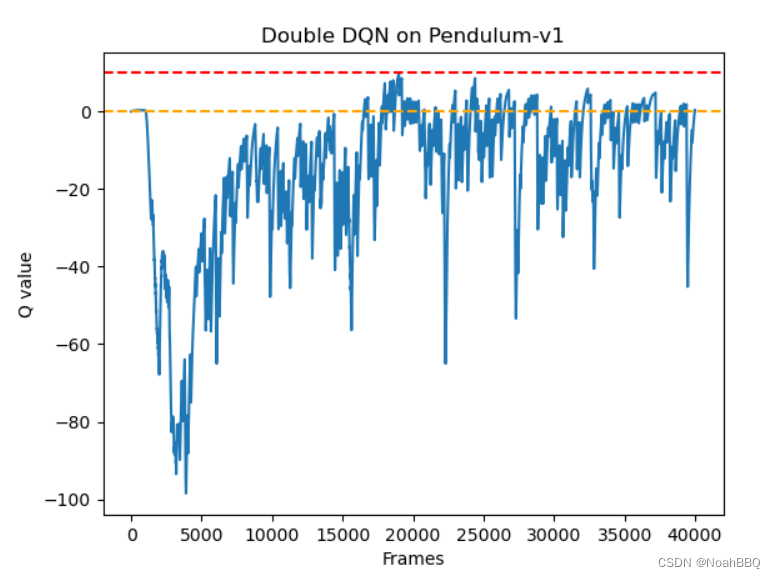
可以看到从double DQN
确实缓解了高估的问题,q-value
整体下移了。