开始的话:
从基础做起,不断学习,坚持不懈,加油。
一位爱生活爱技术来自火星的程序汪
讲完 w o r d 2 v e c word2vec word2vec ,接下来我们就要讲讲 E L M o ELMo ELMo了,来自论文:Deep contextualized word representations,而 E L M o ELMo ELMo是表示 E m b e d d i n g s Embeddings Embeddings f r o m from from L a n g u a g e Language Language M o d e l s Models Models的意思。
S t e p Step Step 1. 1. 1. t r a i n train train
如下图所示:
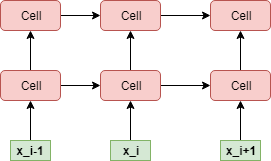
在训练过程中,
E
L
M
o
ELMo
ELMo有两个输入,一个正向的
i
d
s
ids
ids,以及一个反向的
i
d
s
ids
ids_
r
e
v
e
r
s
e
reverse
reverse。需要特别注意的是:这两个
i
d
id
id是 没有任何关系 的,也就是说不是同一个输入文本的正向
i
d
id
id和反向
i
d
id
id。
训练阶段也比较简单:
- 对于 i d s ids ids,扔进一个如上图所示的一个 m u l t i R N N C e l l multiRNNCell multiRNNCell,如果对这个结构不是很了解,请看我之前的 b l o g blog blog。
- 对于 i d s ids ids_ r e v e r s e reverse reverse,同样扔进一个 m u l t i R N N C e l l multiRNNCell multiRNNCell。
- 拿到这两个 m u l t i R N N C e l l multiRNNCell multiRNNCell的输出,分别进行 l o s s loss loss计算( s a m p l e d sampled sampled_ s o f t m a x softmax softmax_ l o s s loss loss ,然后求和求平均得到最终的loss。
当训练结束后,会得到几个很重要的文件,这些文件就是我们在 d o w n down down s t r e a m stream stream t a s k task task中需要用的:
- o p t i o n s . j s o n options.json options.json 训练的参数文件
- v o c a b vocab vocab_ e m b e d d i n g . h d f 5 embedding.hdf5 embedding.hdf5 训练完语言模型之后的 E m b e d d i n g s Embeddings Embeddings
- w e i g h t s . h d f 5 weights.hdf5 weights.hdf5 训练完之后各层的参数
- v o c a b . t x t vocab.txt vocab.txt 词表文件
S t e p Step Step 2. 2. 2. p r e d i c t predict predict
在预测阶段,对于一个输入的 i d s ids ids会进行如下操作:
- 在 f o r w a r d forward forward中输入 i d s ids ids,有两层 L S T M C e l l LSTMCell LSTMCell,保存两个结果;
- 在 b a c k w a r d backward backward中输入 i d s ids ids_ r e v e r s e reverse reverse,有两层 L S T M C e l l LSTMCell LSTMCell,保存两个结果;
- 这样我们就能拿到三个结果:
- 由 e m b e d d i n g s embeddings embeddings文件得到当前输入的 e m b e d d i n g s embeddings embeddings 第一层
- 由 f o r w a r d forward forward和 b a c k w a r d backward backward中的第一个输出的 c o n c a t concat concat结果 第二层
- 由 f o r w a r d forward forward和 b a c k w a r d backward backward中的第二个输出的 c o n c a t concat concat结果 第三层
- 对上述每一层,设置了可训练的权重参数 W W W和统一 s c a l e scale scale参数 g a m m a gamma gamma。
这样在下游的不同任务中,不同层的权重则可训练为不同的值,从而适应性更强,这也就是相对于 w o r d 2 v e c word2vec word2vec固定的 v e c t o r vector vector的优势所在了。
在预测阶段的各层的参数是直接用 w e i g h t s . h d f 5 weights.hdf5 weights.hdf5中的参数,而这些参数在下游任务中,是不参与训练过程的,也就是不会 f i n e fine fine- t u n e tune tune的,作为词向量参与训练的只有 W W W和 g a m m a gamma gamma。
更多的细节和代码注释请查看我的
g
i
t
h
u
b
github
github,有很详细的代码注释说明。
这里只是对于一些重要的地方做一个学习笔记。
另外在
g
i
t
h
u
b
github
github中还有
N
E
R
NER
NER中加入
E
L
M
o
ELMo
ELMo的实践,以及更多个人的一些项目。
谢谢
更多代码请移步我的个人 g i t h u b github github,会不定期更新。
欢迎关注