机器学习训练集和测试集
Prerequisite:
先决条件:
Well, those who haven’t yet read my previous articles should note that for machine learning in java I am using a weka.jar file to import the required machine learning classes into my eclipse IDE. I will suggest you guys have a look at my article on data splitting using Python programming language.
好吧,那些尚未阅读我以前的文章的人应该注意,对于Java中的机器学习,我正在使用weka.jar文件将所需的机器学习类导入到我的Eclipse IDE中。 我建议你们看看我有关使用Python编程语言进行数据拆分的文章。
Let’s have a look at the basic definition of training and test sets before we proceed further.
在继续进行之前,让我们看一下训练和测试集的基本定义。
训练套 (Training Set)
The purpose of using the training set is as the name suggests is to train our model by feeding in the attributes and the corresponding target value into using the values in the training our model can identify a pattern which will be used by our model to predict the test set values.
顾名思义,使用训练集的目的是通过输入属性和相应的目标值以训练模型中的值来训练我们的模型,我们的模型可以识别出一种模式,我们的模型将使用该模式来预测测试设定值。
测试集 (Test Set)
This set is used to check the accuracy of our model and as the name suggest we use this dataset to perform the testing of our result. This data set usually contains the independent attributes using which our model predicts the dependent value or the target value. Using the predicted target values we further compare those values with the predefined set of the target values in our test set in order to determine the various evaluating parameters like RMSE,percentage accuracy, percentage error, area under the curve to determine the efficiency of our model in predicting the dependent values which in turn determines the usefulness of our model.
该集合用于检查模型的准确性,顾名思义,我们使用该数据集对结果进行测试。 该数据集通常包含独立属性,我们的模型将使用这些独立属性来预测相关值或目标值。 使用预测的目标值,我们进一步将这些值与测试集中的目标值的预定义集进行比较,以确定各种评估参数,例如RMSE,百分比精度,百分比误差,曲线下面积,以确定模型的效率预测相关值,进而确定模型的实用性。
For detailed information about training and test set, you can refer to my article about data splitting.
有关培训和测试集的详细信息,您可以参考我有关数据拆分的文章。
Another important feature that we are going to talk about is the cross-validation. Well, in order to increase the accuracy of our model we use cross-validation. Suppose if we split our data in such a way that we have 100 set of values and we split first 20 as testing sets and rest as the training sets, well since we need more data for training the splitting ratio we used here is completely fine but then there arise many uncertainties like what if the first 20 sets of data have completely opposite values from the rest of data one way to sort this issue is to use a random function which will randomly select the testing and training set values so now we have reduced chances of getting biased set of values into our training and test sets but still we have not fully sorted the problem there are still chances that maybe the randomized testing data set has the values which aren’t at all related to the training set values or it might be that the values in the test set are exactly the same as that of training set which will result in overfitting of our model ,you can refer to this article if you want to know more about overfitting and underfitting of the data.
我们将要讨论的另一个重要功能是交叉验证。 好吧,为了提高我们模型的准确性,我们使用了交叉验证。 假设如果我们以100个值集的方式拆分数据,然后将前20个值拆分为测试集,其余的拆分为训练集,那么由于我们需要更多的数据来训练拆分率,因此这里使用的方法完全可以,但是那么就会出现许多不确定性,例如如果前20组数据与其余数据具有完全相反的值,该问题排序的一种方法是使用随机函数,该函数将随机选择测试和训练集的值,因此现在我们减少了可能会在我们的训练和测试集中引入偏向的值集,但仍然没有完全解决问题,仍然有可能随机化的测试数据集具有与训练集值完全不相关的值,或者可能是测试集中的值与训练集中的值完全相同,这将导致我们的模型过度拟合,如果您想了解更多关于t的过度拟合和不足的信息 ,可以参考本文。 他数据 。
Well, then how do we solve this issue? One way is to split the data n times into training and testing sets and then find the average of those splitting datasets to create the best possible set for training and testing. But everything comes with a cost since we are repeatedly splitting out data into training and testing the process of cross-validation consumes some time. But then it is worth waiting if we can get a more accurate result.
好吧,那我们怎么解决这个问题呢? 一种方法是将数据n次分割为训练和测试集,然后找到这些分割数据集的平均值,以创建最佳的训练和测试集。 但是,一切都是有代价的,因为我们要反复将数据分成训练和测试交叉验证的过程,这会花费一些时间。 但是,如果我们可以获得更准确的结果,那就值得等待。
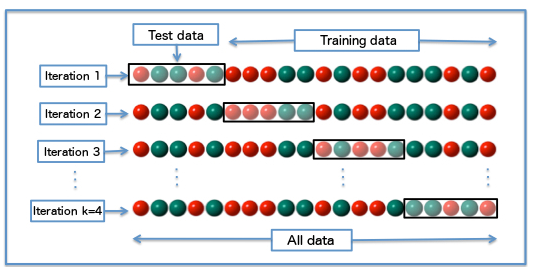
Image source: https://upload.wikimedia.org/wikipedia/commons/1/1c/K-fold_cross_validation_EN.jpg
图片来源: https : //upload.wikimedia.org/wikipedia/commons/1/1c/K-fold_cross_validation_EN.jpg
While writing the code I would be using a variable named as fold or K as shown in the above figure which signifies the no of times to perform the cross-validation.
在编写代码时,我将使用一个名为fold或K的变量,如上图所示,它表示没有时间执行交叉验证。
Below is the java code is written for generating testing and training sets in the ratio of 1:4(approx.) which is an optimal ratio of splitting the data sets.
下面是编写Java代码以生成测试和训练集的比例为1:4(大约)的比率,这是拆分数据集的最佳比率。
The data set I have used can be copied from here: File name: "headbraina.arff"
我使用的数据集可以从这里复制: 文件名:“ headbraina.arff”
@relation headbrain-weka.filters.unsupervised.attribute.Remove-R1-weka.filters.unsupervised.attribute.Remove-R1
@attribute 'Head Size(cm^3)' numeric
@attribute 'Brain Weight(grams)' numeric
@data
4512,1530
3738,1297
4261,1335
3777,1282
4177,1590
3585,1300
3785,1400
3559,1255
3613,1355
3982,1375
3443,1340
3993,1380
3640,1355
4208,1522
3832,1208
3876,1405
3497,1358
3466,1292
3095,1340
4424,1400
3878,1357
4046,1287
3804,1275
3710,1270
4747,1635
4423,1505
4036,1490
4022,1485
3454,1310
4175,1420
3787,1318
3796,1432
4103,1364
4161,1405
4158,1432
3814,1207
3527,1375
3748,1350
3334,1236
3492,1250
3962,1350
3505,1320
4315,1525
3804,1570
3863,1340
4034,1422
4308,1506
3165,1215
3641,1311
3644,1300
3891,1224
3793,1350
4270,1335
4063,1390
4012,1400
3458,1225
3890,1310
4166,1560
3935,1330
3669,1222
3866,1415
3393,1175
4442,1330
4253,1485
3727,1470
3329,1135
3415,1310
3372,1154
4430,1510
4381,1415
4008,1468
3858,1390
4121,1380
4057,1432
3824,1240
3394,1195
3558,1225
3362,1188
3930,1252
3835,1315
3830,1245
3856,1430
3249,1279
3577,1245
3933,1309
3850,1412
3309,1120
3406,1220
3506,1280
3907,1440
4160,1370
3318,1192
3662,1230
3899,1346
3700,1290
3779,1165
3473,1240
3490,1132
3654,1242
3478,1270
3495,1218
3834,1430
3876,1588
3661,1320
3618,1290
3648,1260
4032,1425
3399,1226
3916,1360
4430,1620
3695,1310
3524,1250
3571,1295
3594,1290
3383,1290
3499,1275
3589,1250
3900,1270
4114,1362
3937,1300
3399,1173
4200,1256
4488,1440
3614,1180
4051,1306
3782,1350
3391,1125
3124,1165
4053,1312
3582,1300
3666,1270
3532,1335
4046,1450
3667,1310
2857,1027
3436,1235
3791,1260
3302,1165
3104,1080
3171,1127
3572,1270
3530,1252
3175,1200
3438,1290
3903,1334
3899,1380
3401,1140
3267,1243
3451,1340
3090,1168
3413,1322
3323,1249
3680,1321
3439,1192
3853,1373
3156,1170
3279,1265
3707,1235
4006,1302
3269,1241
3071,1078
3779,1520
3548,1460
3292,1075
3497,1280
3082,1180
3248,1250
3358,1190
3803,1374
3566,1306
3145,1202
3503,1240
3571,1316
3724,1280
3615,1350
3203,1180
3609,1210
3561,1127
3979,1324
3533,1210
3689,1290
3158,1100
4005,1280
3181,1175
3479,1160
3642,1205
3632,1163
3069,1022
3394,1243
3703,1350
3165,1237
3354,1204
3000,1090
3687,1355
3556,1250
2773,1076
3058,1120
3344,1220
3493,1240
3297,1220
3360,1095
3228,1235
3277,1105
3851,1405
3067,1150
3692,1305
3402,1220
3995,1296
3318,1175
2720,955
2937,1070
3580,1320
2939,1060
2989,1130
3586,1250
3156,1225
3246,1180
3170,1178
3268,1142
3389,1130
3381,1185
2864,1012
3740,1280
3479,1103
3647,1408
3716,1300
3284,1246
4204,1380
3735,1350
3218,1060
3685,1350
3704,1220
3214,1110
3394,1215
3233,1104
3352,1170
3391,1120
Code:
码:
import weka.core.Instances;
import java.io.File;
import java.util.Random;
import weka.core.converters.ArffSaver;
import weka.core.converters.ConverterUtils.DataSource;
import weka.classifiers.Evaluation;
import weka.classifiers.bayes.NaiveBayes;
public class testtrainjaava{
public static void main(String args[]) throws Exception{
//load dataset
DataSource source = new DataSource("headbraina.arff");
Instances dataset = source.getDataSet();
//set class index to the last attribute
dataset.setClassIndex(dataset.numAttributes()-1);
int seed = 1;
int folds = 15;
//randomize data
Random rand = new Random(seed);
//create random dataset
Instances randData = new Instances(dataset);
randData.randomize(rand);
//stratify
if (randData.classAttribute().isNominal())
randData.stratify(folds);
// perform cross-validation
for (int n = 0; n < folds; n++) {
//Evaluation eval = new Evaluation(randData);
//get the folds
Instances train = randData.trainCV(folds, n);
Instances test = randData.testCV(folds, n);
ArffSaver saver = new ArffSaver();
saver.setInstances(train);
System.out.println("No of folds done = " + (n+1));
saver.setFile(new File("trainheadbraina.arff"));
saver.writeBatch();
//if(n==9)
//{System.out.println("Training set generated after the final fold is");
//System.out.println(train);}
ArffSaver saver1 = new ArffSaver();
saver1.setInstances(test);
saver1.setFile(new File("testheadbraina1.arff"));
saver1.writeBatch();
}
}
}
Output
输出量
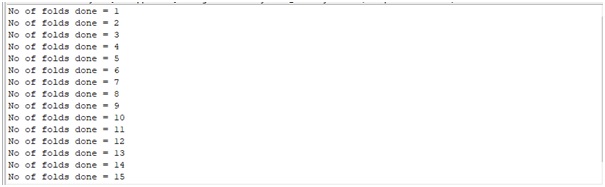
After getting this output just go to the destination folder in which you have to save the training and testing data sets and you should see the following results.
获得此输出后,只需转到目标文件夹,您必须在其中保存训练和测试数据集,并且应该看到以下结果。
Dataset generated for training the model
生成用于训练模型的数据集
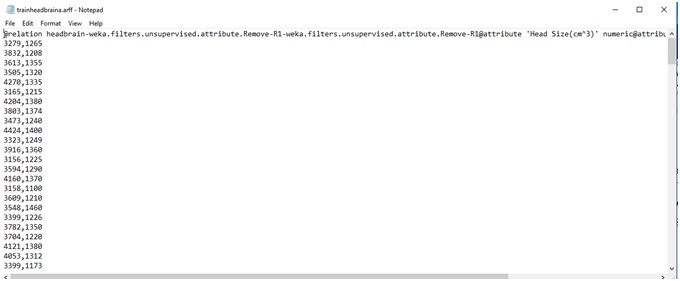
Dataset generated for testing the model
生成用于测试模型的数据集
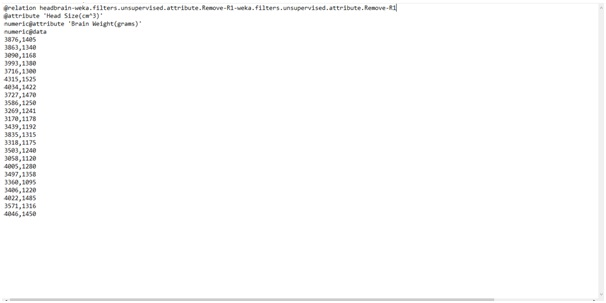
This was all for today guys hope you liked this, feel free to ask your queries and have a great day ahead.
今天,这就是所有这些家伙希望您喜欢的东西,随时询问您的问题,并祝您有美好的一天。
翻译自: https://www.includehelp.com/ml-ai/training-and-testing-sets-in-java.aspx
机器学习训练集和测试集