In our previous tutorial, we learned about Python CSV Example. In this tutorial we are going to learn Python Multiprocessing with examples.
在上一教程中,我们了解了Python CSV Example 。 在本教程中,我们将通过示例学习Python多重处理。
Python多处理 (Python Multiprocessing)
Parallel processing is getting more attention nowadays. If you still don’t know about the parallel processing, learn from wikipedia.
如今,并行处理越来越受到关注。 如果您仍然不了解并行处理,请向Wikipedia学习。
As CPU manufacturers start adding more and more cores to their processors, creating parallel code is a great way to improve performance. Python introduced multiprocessing module to let us write parallel code.
随着CPU制造商开始向其处理器中添加越来越多的内核,创建并行代码是提高性能的好方法。 Python引入了多处理模块,让我们可以编写并行代码。
To understand the main motivation of this module, we have to know some basics about parallel programming. After reading this article, we hope that, you would be able to gather some knowledge on this topic.
要了解此模块的主要动机,我们必须了解有关并行编程的一些基础知识。 阅读本文后,我们希望您能够收集有关此主题的一些知识。
Python多处理进程,队列和锁 (Python Multiprocessing Process, Queue and Locks)
There are plenty of classes in python multiprocessing module for building a parallel program. Among them, three basic classes are Process
, Queue
and Lock
. These classes will help you to build a parallel program.
python多重处理模块中有许多类可用于构建并行程序。 其中三个基本类是Process
, Queue
和Lock
。 这些类将帮助您构建并行程序。
But before describing about those, let us initiate this topic with simple code. To make a parallel program useful, you have to know how many cores are there in you pc. Python Multiprocessing module enables you to know that. The following simple code will print the number of cores in your pc.
但是在描述这些内容之前,让我们用简单的代码启动该主题。 为了使并行程序有用,您必须知道您的PC中有多少个内核。 Python Multiprocessing模块使您知道这一点。 以下简单代码将打印您PC中的内核数。
import multiprocessing
print("Number of cpu : ", multiprocessing.cpu_count())
The following output may vary for your pc. For me, number of cores is 8.
以下输出可能因您的电脑而异。 对我来说,核心数是8。
Python多处理Process类 (Python multiprocessing Process class)
Python multiprocessing Process
class is an abstraction that sets up another Python process, provides it to run code and a way for the parent application to control execution.
Python多重处理Process
类是一种抽象,它建立了另一个Python进程,为它提供了运行代码的方式,并为父应用程序控制执行提供了一种方法。
There are two important functions that belongs to the Process class – start()
and join()
function.
有两个重要的函数属于Process类start()
和join()
函数。
At first, we need to write a function, that will be run by the process. Then, we need to instantiate a process object.
首先,我们需要编写一个将由进程运行的函数。 然后,我们需要实例化一个流程对象。
If we create a process object, nothing will happen until we tell it to start processing via start()
function. Then, the process will run and return its result. After that we tell the process to complete via join()
function.
如果我们创建一个流程对象,那么直到我们告诉它通过start()
函数开始处理之前,什么都不会发生。 然后,该过程将运行并返回其结果。 之后,我们通过join()
函数告诉该过程完成。
Without join()
function call, process will remain idle and won’t terminate.
没有join()
函数调用,进程将保持空闲状态并且不会终止。
So if you create many processes and don’t terminate them, you may face scarcity of resources. Then you may need to kill them manually.
因此,如果您创建许多进程而没有终止它们,则可能会面临资源短缺。 然后,您可能需要手动杀死它们。
One important thing is, if you want to pass any argument through the process you need to use args
keyword argument. The following code will be helpful to understand the usage of Process class.
一件重要的事情是,如果要在过程中传递任何参数,则需要使用args
关键字参数。 以下代码将有助于理解Process类的用法。
from multiprocessing import Process
def print_func(continent='Asia'):
print('The name of continent is : ', continent)
if __name__ == "__main__": # confirms that the code is under main function
names = ['America', 'Europe', 'Africa']
procs = []
proc = Process(target=print_func) # instantiating without any argument
procs.append(proc)
proc.start()
# instantiating process with arguments
for name in names:
# print(name)
proc = Process(target=print_func, args=(name,))
procs.append(proc)
proc.start()
# complete the processes
for proc in procs:
proc.join()
The output of the following code will be:
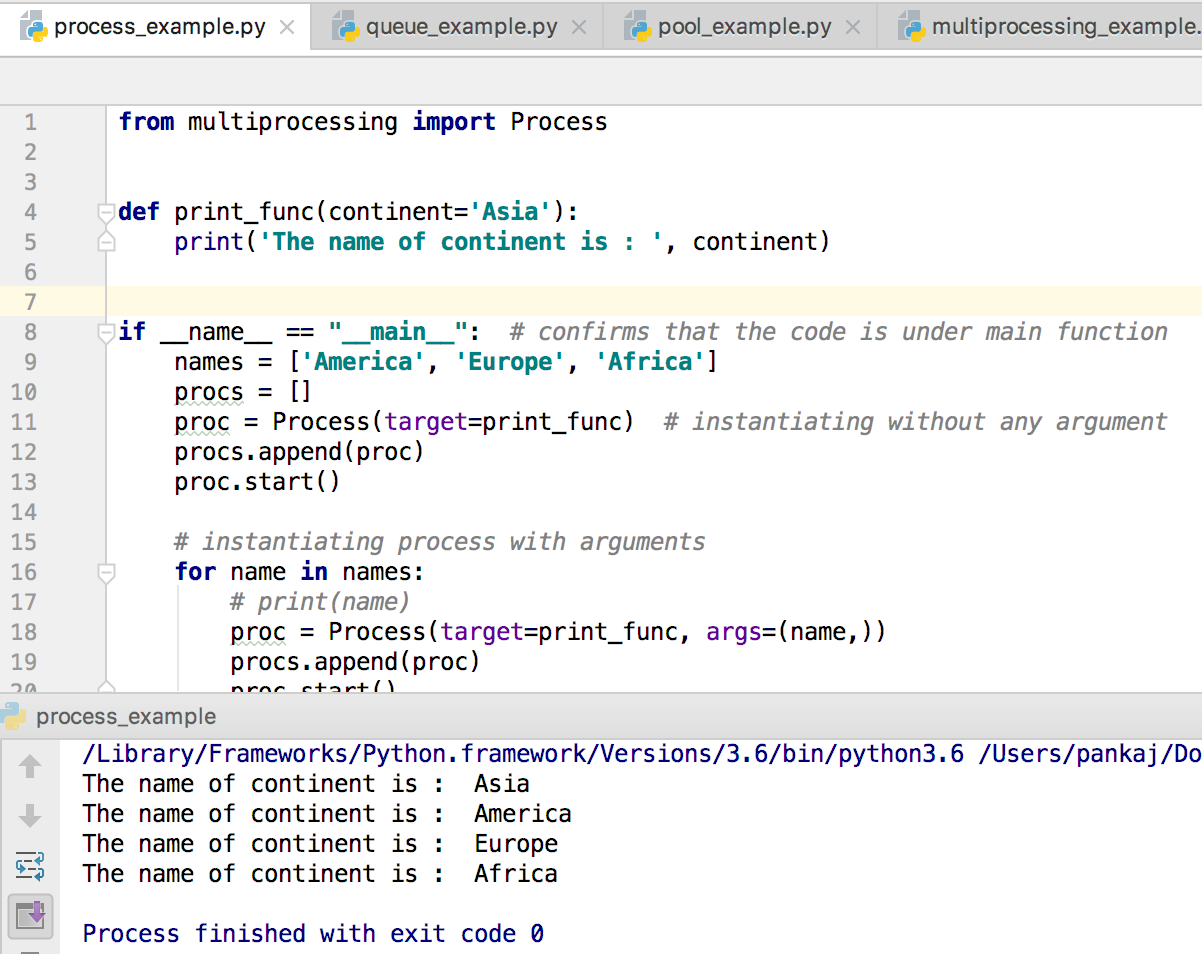
以下代码的输出将是:
Python多处理Queue类 (Python multiprocessing Queue class)
You have basic knowledge about computer data-structure, you probably know about Queue.
您具有有关计算机数据结构的基本知识,您可能也了解Queue。
Python Multiprocessing modules provides Queue
class that is exactly a First-In-First-Out data structure. They can store any pickle Python object (though simple ones are best) and are extremely useful for sharing data between processes.
Python多重处理模块提供的Queue
类完全是先进先出数据结构。 它们可以存储任何pickle的Python对象(虽然最好是简单的对象),并且对于在进程之间共享数据非常有用。
Queues are specially useful when passed as a parameter to a Process’ target function to enable the Process to consume data. By using put()
function we can insert data to then queue and using get()
we can get items from queues. See the following code for a quick example.
当队列作为参数传递给流程的目标函数以使流程能够使用数据时,队列特别有用。 通过使用put()
函数,我们可以将数据插入然后排队,而使用get()
我们可以从队列中获取项目。 请参见以下代码以获取快速示例。
from multiprocessing import Queue
colors = ['red', 'green', 'blue', 'black']
cnt = 1
# instantiating a queue object
queue = Queue()
print('pushing items to queue:')
for color in colors:
print('item no: ', cnt, ' ', color)
queue.put(color)
cnt += 1
print('\npopping items from queue:')
cnt = 0
while not queue.empty():
print('item no: ', cnt, ' ', queue.get())
cnt += 1
Python多处理锁类 (Python multiprocessing Lock Class)
The task of Lock class is quite simple. It allows code to claim lock so that no other process can execute the similar code until the lock has be released. So the task of Lock class is mainly two. One is to claim lock and other is to release the lock. To claim lock the, acquire()
function is used and to release lock release()
function is used.
Lock类的任务非常简单。 它允许代码声明锁,以便在释放锁之前没有其他进程可以执行类似的代码。 因此,Lock类的任务主要是两个。 一种是要求锁定,另一种是释放锁定。 要声明锁定,使用acquire()
函数,并释放锁定release()
函数。
Python多处理示例 (Python multiprocessing example)
In this Python multiprocessing example, we will merge all our knowledge together.
在这个Python多处理示例中,我们将所有知识融合在一起。
Suppose we have some tasks to accomplish. To get that task done, we will use several processes. So, we will maintain two queue. One will contain the tasks and the other will contain the log of completed task.
假设我们要完成一些任务。 为了完成该任务,我们将使用几个过程。 因此,我们将维持两个队列。 一个将包含任务,另一个将包含已完成任务的日志。
Then we instantiate the processes to complete the task. Note that the python Queue class is already synchronized. That means, we don’t need to use the Lock class to block multiple process to access the same queue object. That’s why, we don’t need to use Lock class in this case.
然后,我们实例化流程以完成任务。 请注意,python Queue类已经同步。 这意味着,我们不需要使用Lock类来阻止多个进程访问同一队列对象。 这就是为什么在这种情况下我们不需要使用Lock类。
Below is the implementation where we are adding tasks to the queue, then creating processes and starting them, then using join()
to complete the processes. Finally we are printing the log from the second queue.
下面是将任务添加到队列中,然后创建进程并启动它们,然后使用join()
完成过程的实现。 最后,我们从第二个队列中打印日志。
from multiprocessing import Lock, Process, Queue, current_process
import time
import queue # imported for using queue.Empty exception
def do_job(tasks_to_accomplish, tasks_that_are_done):
while True:
try:
'''
try to get task from the queue. get_nowait() function will
raise queue.Empty exception if the queue is empty.
queue(False) function would do the same task also.
'''
task = tasks_to_accomplish.get_nowait()
except queue.Empty:
break
else:
'''
if no exception has been raised, add the task completion
message to task_that_are_done queue
'''
print(task)
tasks_that_are_done.put(task + ' is done by ' + current_process().name)
time.sleep(.5)
return True
def main():
number_of_task = 10
number_of_processes = 4
tasks_to_accomplish = Queue()
tasks_that_are_done = Queue()
processes = []
for i in range(number_of_task):
tasks_to_accomplish.put("Task no " + str(i))
# creating processes
for w in range(number_of_processes):
p = Process(target=do_job, args=(tasks_to_accomplish, tasks_that_are_done))
processes.append(p)
p.start()
# completing process
for p in processes:
p.join()
# print the output
while not tasks_that_are_done.empty():
print(tasks_that_are_done.get())
return True
if __name__ == '__main__':
main()
Depending on the number of task, the code will take some time to show you the output. The output of the following code will vary from time to time.
根据任务的数量,代码将需要一些时间来显示输出。 以下代码的输出将不时变化。
Python多处理池 (Python multiprocessing Pool)
Python multiprocessing Pool can be used for parallel execution of a function across multiple input values, distributing the input data across processes (data parallelism). Below is a simple Python multiprocessing Pool example.
Python多处理池可用于跨多个输入值并行执行功能,从而跨进程分配输入数据(数据并行性)。 下面是一个简单的Python多处理池示例。
from multiprocessing import Pool
import time
work = (["A", 5], ["B", 2], ["C", 1], ["D", 3])
def work_log(work_data):
print(" Process %s waiting %s seconds" % (work_data[0], work_data[1]))
time.sleep(int(work_data[1]))
print(" Process %s Finished." % work_data[0])
def pool_handler():
p = Pool(2)
p.map(work_log, work)
if __name__ == '__main__':
pool_handler()
Below image shows the output of the above program. Notice that pool size is 2, so two executions of work_log
function is happening in parallel. When one of the function processing finishes, it picks the next argument and so on.
下图显示了以上程序的输出。 请注意,池大小为2,因此work_log
函数的两个执行并行发生。 当函数处理之一完成时,它将选择下一个参数,依此类推。
So, that’s all for python multiprocessing module.
因此,这就是python多处理模块的全部内容。
Reference: Official Documentation
参考: 官方文档
翻译自: https://www.journaldev.com/15631/python-multiprocessing-example