Notes of Neural Networks & Deep Learning by Andrew Ng, Introduction to Deep Learning, what is neural network.
The term deep learning refers to training neural networks, sometimes very large neural networks. So what is exactly a neural network?
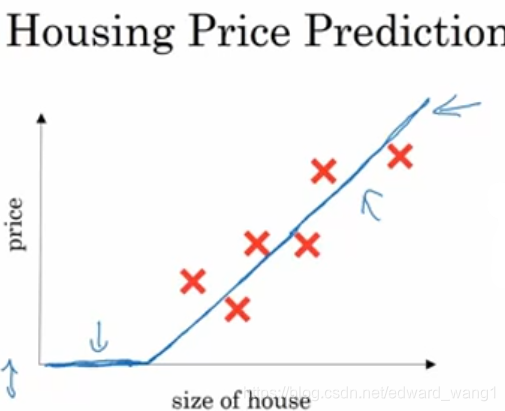
Let's start with a housing price prediction example. Say you have a data set with six houses. You know the size of the houses in square feet or square meters, and you know the price of the houses. You want to fit a function to predict the price of the house as a function of the size. Well, we can fit the data with linear regression. But we know the prices cannot be negative, so to to be a bit fancier, let's bend the curve here so it ends up zero here. So this thick blue line ends up being your function for predicting the price of a house as a function of its size. You can think of this function that you've just fit to housing prices as a very simple neural network as figure-2.
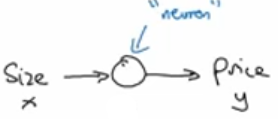
We have, as the input to the neural network, the size of the house called x. It goes into this node/circle. And then it outputs the price we call y. This little circle is called a neuron. Then your neural network implements this function we draw in figure-1. And what the neuron does is it inputs the size, computes the linear function, and then outputs the estimated price. By the way, in the neural network literature, you see this function a lot. This function is called a ReLU function which stands for Rectified Linear Unit. And rectify just means take a max of zero. This is a tiny neural network and a large neural network is formed by taking many of these single neurons and stack them together.
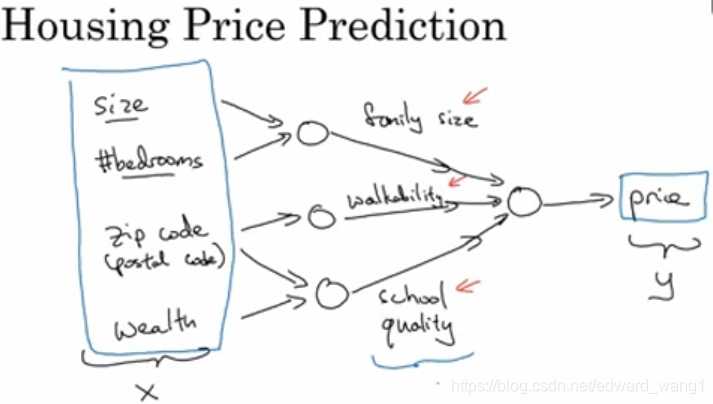
For example, instead of predicting the price of the house from its size, you now have other features about the house:
- We may know the number of bedrooms (#bedrooms) besides the size. One of the things that really affects the price of a house is family size that the house can support. And actually the size of the house and #bedrooms determine whether or not a house can fit your family size.
- Maybe you know the zip code / postal code. It maybe as a feature tells you walkability. It means whether its neighborhood highly walkable? Can you just walk to the grocery store? Do you need drive?
- And the wealth, plus the zip code tell you how good is the school quality.
Each of those little circles in figure-3 can be one of those ReLU or some other slightly nonlinear function. So based on the size and #bedrooms, you can estimate the family size that can be supported. With zip code, you can estimate walkability. Based on zip code and wealth you can estimate the school quality. Finally, the way people decide how much they're willing to pay for a house is they look at the things that really matter to them. In this case, family size, walkability and school quality. And these help you predict the price. In this example, x is the four inputs and y is the price tying to predict. So by stacking together a few of the single neurons or the simple predictors, we now have a slightly larger neuro network. Part of the magic of a neural network is that when you implement it, you need to give it just the input x, and the output y. All of these things in the middle (hidden layer), it will figure out by itself.
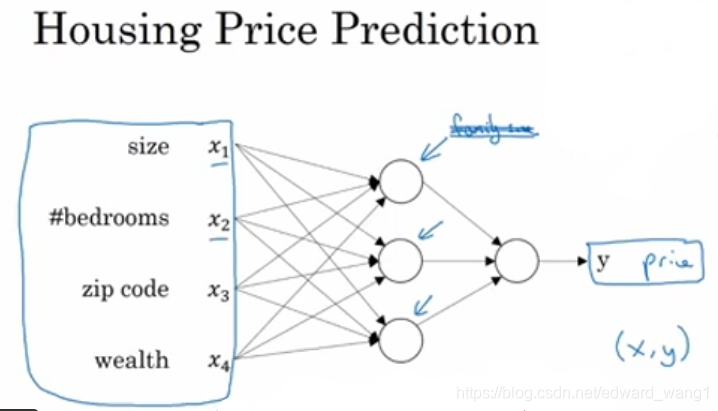
So, what you actually implemented is as figure-4. It's a neural network with four inputs and one output. And it has one hiddle layer with three hidden units. Note that each of the hidden unit takes its inputs of all four features. So, rather than saying this first hidden unit represents 'family size' which depends only on the features of features and
. Instead, we'll say, well, neural network, you decide whatever you want this first hiddenn unit to be and we'll give you all four features to compute whatever you want. The remarkable thing about neural networks is that given enough training examples
, neural networks are remarkably good at figuring out functions that accurately map from x to y.
So, that's a basic neural network. It turns out that as you build out your own neural networks, you probably find them to be most useful, most powerful in supervised learning settings. Meaning that you're trying to take an input x and map it to some output y.
<end>