This article is the notes when I learn the class "Neural Networks & Deep Learning" by teacher Andrew Ng. It shows info of Logistic Regression which is the basics of Neural Network Programming. Shares it with you and hope it helps.
Logistic regression is a learning algorithm used in a supervised learning problem when the output 𝑦 are all either zero or one, so for binary classification problems.
Given an input feature vector x which maybe corresponding to an image that you want to recognize as either a cat picture or not a cat picture, the algorithm will evaluate the probability of a cat being in that image. Mathematically:
, where
It means, given x, we want to know which is the chance of y=1
The parameters used in Logistic regression are:
• The input features vector: , where
is the number of features
• The training label:
• The weights: , where
is the number of features
• The threshold:
• The output:
• Sigmoid function:
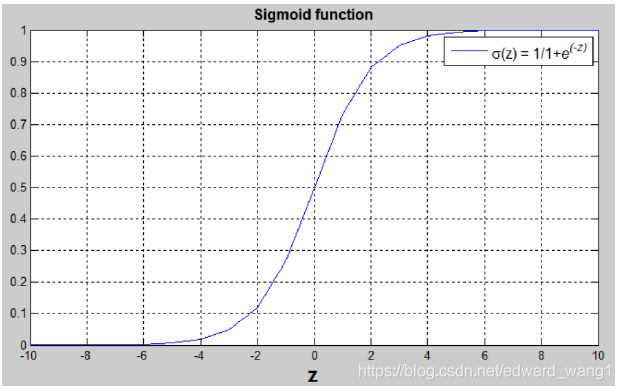
is a linear function
, but since we are looking for a probability constraint between [0,1], the sigmoid function is used. The function is bounded between [0,1] as shown in Figure-1.
Some observations from the graph:
• If 𝑧 is a large positive number, then
• If 𝑧 is small or large negative number, then
• If 𝑧=0, then
<end>