1. 手写数字识别简述
1.1. MNIST
- 7000 images per category
- train/test splitting: 60k vs 10k
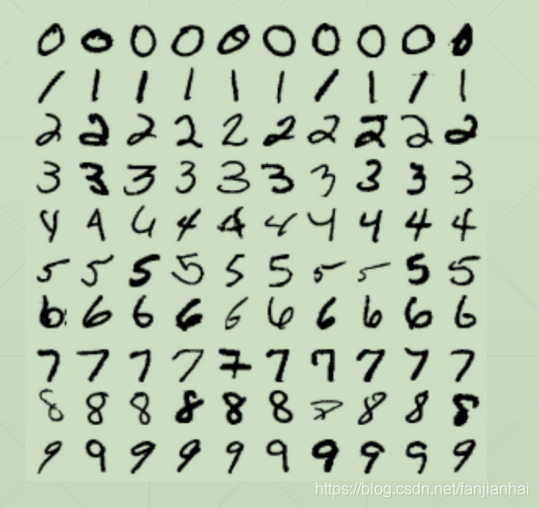
1.2. Image
- [28,28,1]
- →[784]
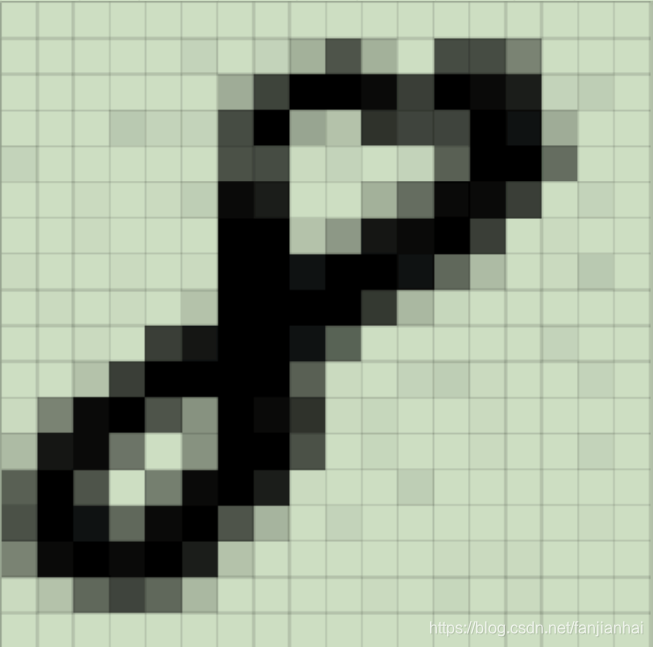
1.3. Input and Output
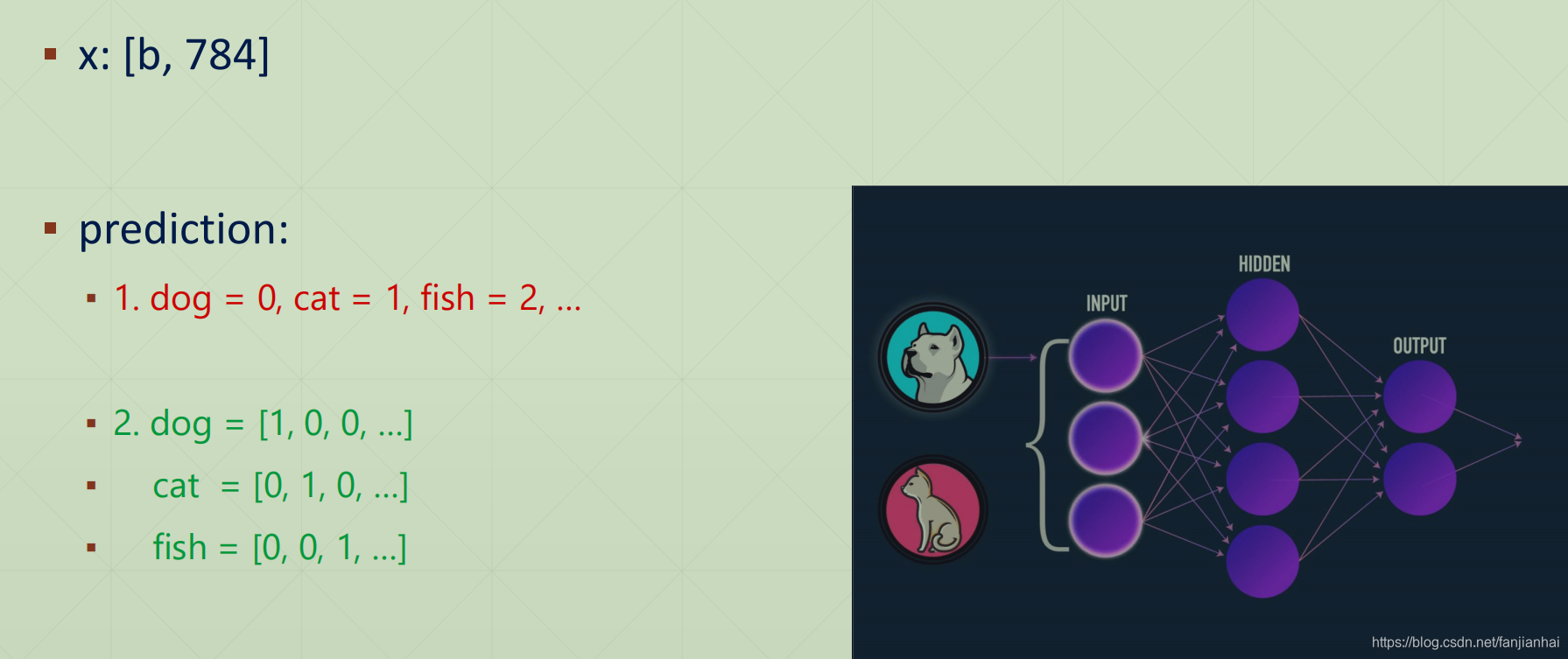
1.4. Regression VS Classification
- 𝑦 = 𝑤 ∗ 𝑥 + 𝑏
- 𝑜𝑢𝑡 = 𝑋@𝑊 + b
- out: [0.1, 0.8, 0.02, 0.08]
- 𝑝𝑟𝑒𝑑 = 𝑎𝑟𝑔𝑚𝑎𝑥(𝑜𝑢𝑡)
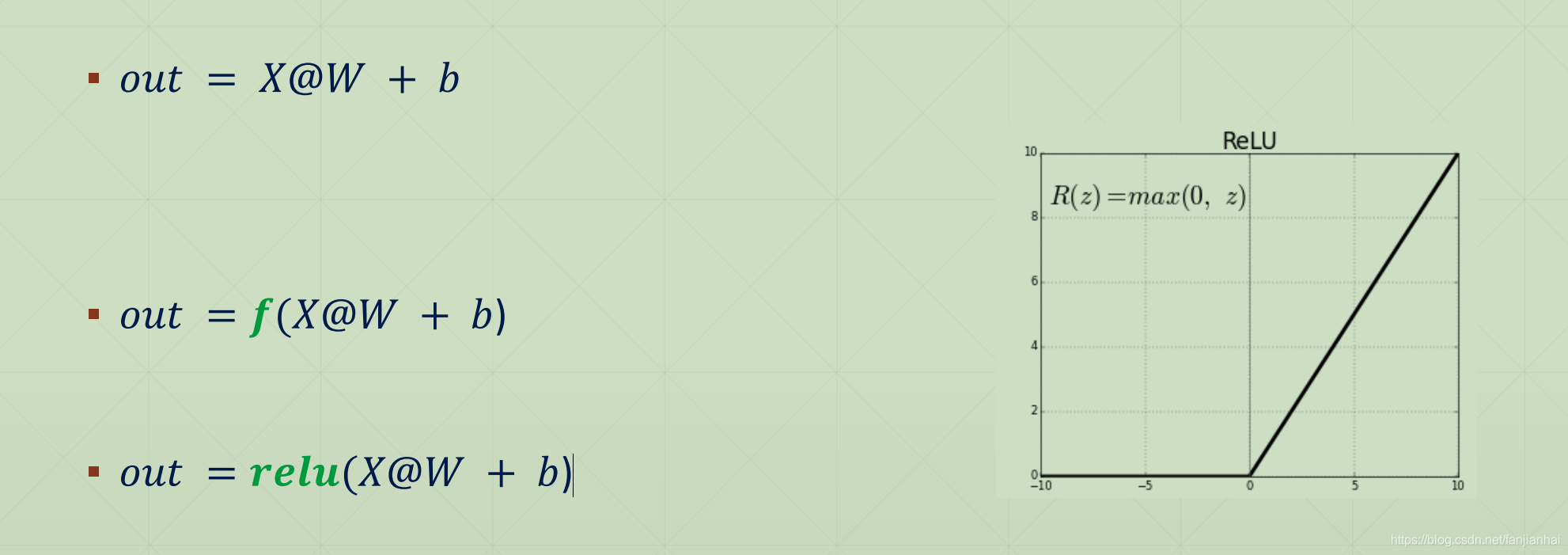
1.5. Particularly
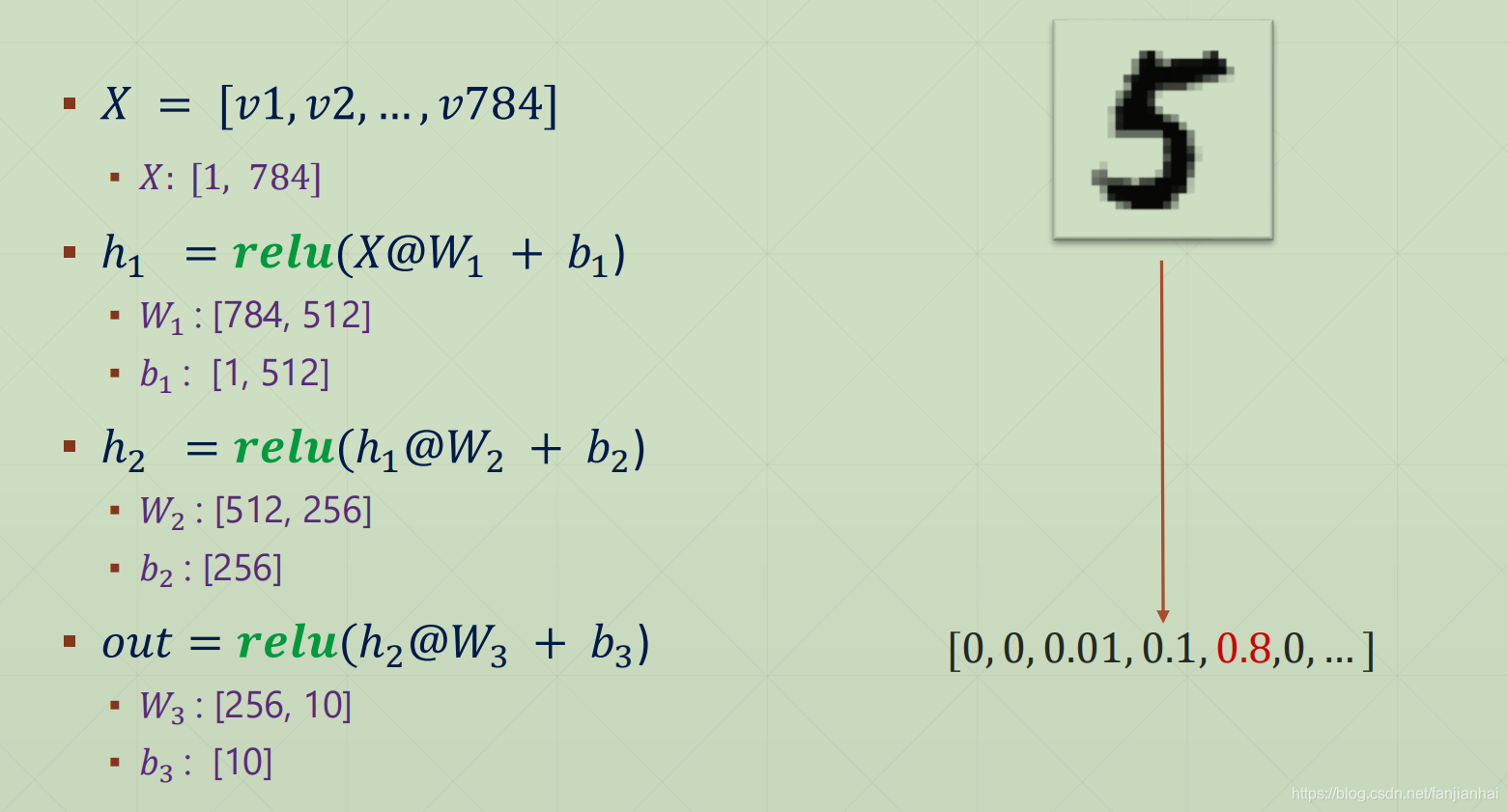
1.5. Compute Loss
- 𝑜𝑢𝑡: [1, 10]
- Y/label: 0~9
- eg.: 1 → [0, 1, 0, 0, 0, 0, 0, 0, 0, 0, 0]
- eg.: 3 → [0, 0, 0, 1, 0, 0, 0, 0, 0, 0, 0]
- Euclidean Distance(欧式距离): 𝑜𝑢𝑡 → Label
1.6. In a nutshell
- 𝑜𝑢𝑡 = 𝑟𝑒𝑙𝑢{𝑟𝑒𝑙𝑢 𝑟𝑒𝑙𝑢 𝑋@𝑊1 + 𝑏1 @𝑊2 + 𝑏2 @𝑊3 + 𝑏3}
- 𝑝𝑟𝑒𝑑 = 𝑎𝑟𝑔𝑚𝑎𝑥(𝑜𝑢𝑡)
- 𝑙𝑜𝑠𝑠 = 𝑀𝑆𝐸(𝑜𝑢𝑡, 𝑙𝑎𝑏𝑒𝑙)
- minimize 𝑙𝑜𝑠𝑠
- [𝑊1, 𝑏1′, 𝑊2′, 𝑏2′, 𝑊3′ , 𝑏3′ ]
2. We need TensorFlow
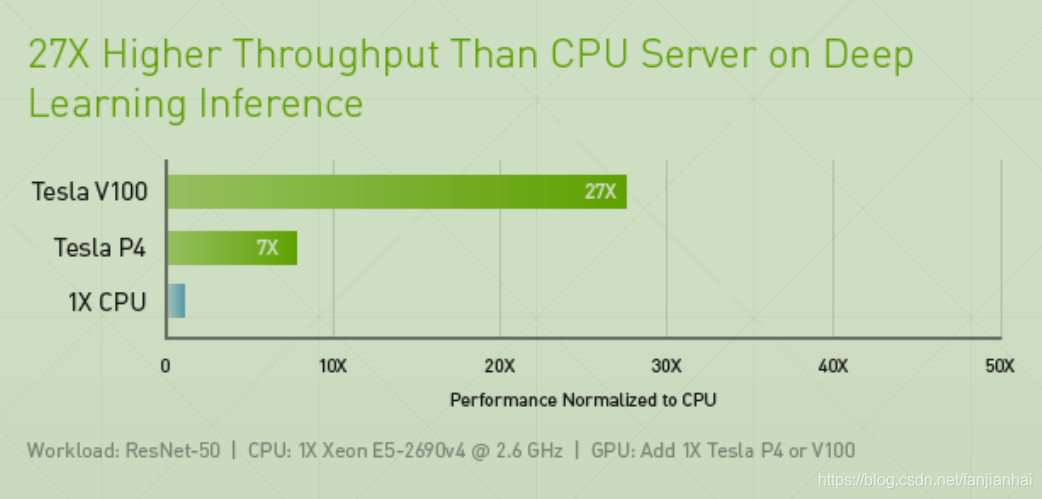
3. Code
- X and Y
- 𝑜𝑢𝑡 = 𝑟𝑒𝑙𝑢{𝑟𝑒𝑙𝑢 𝑟𝑒𝑙𝑢 𝑋@𝑊1 + 𝑏1 @𝑊2 + 𝑏2 @𝑊3 + 𝑏3}
- Compute out&loss
- Compute gradient and optimize
- Loop
import os
import tensorflow as tf
from tensorflow import keras
from tensorflow.keras import layers, optimizers, datasets
os.environ['TF_CPP_MIN_LOG_LEVEL'] = '2'
# X and Y
(x, y), (X_val, y_val) = datasets.mnist.load_data()
x = tf.convert_to_tensor(x, dtype=tf.float32) / 255
y = tf.convert_to_tensor(y, dtype=tf.int32)
y = tf.one_hot(y, depth=10)
print(x.shape, y.shape)
train_dataset = tf.data.Dataset.from_tensor_slices((x, y))
train_dataset = train_dataset.batch(200)
# for step, (x, y) in enumerate(train_dataset):
# print(step, x.shape, y, y.shape)
# 𝑜𝑢𝑡 = 𝑟𝑒𝑙𝑢{𝑟𝑒𝑙𝑢 𝑟𝑒𝑙𝑢 𝑋@𝑊1 + 𝑏1 @𝑊2 + 𝑏2 @𝑊3 + 𝑏3}
model = keras.Sequential([
layers.Dense(512, activation='relu'),
layers.Dense(256, activation='relu'),
layers.Dense(10)
])
optimizer = optimizers.SGD(learning_rate=0.001)
def train_epoch(epoch):
# loop
for step, (x, y) in enumerate(train_dataset):
with tf.GradientTape() as tape:
# [b, 28, 28] => [b, 784]
x = tf.reshape(x, (-1, 28 * 28))
# compute output
# [b, 784] => [b, 10]
out = model(x)
# compute loss
loss = tf.reduce_sum(tf.square(out - y)) / x.shape[0]
# optimize and update w1, w2, w3, b1, b2, b3
grads = tape.gradient(loss, model.trainable_variables)
# w' = w - lr * grad
optimizer.apply_gradients((zip(grads, model.trainable_variables)))
if step % 100 == 0:
print(epoch, step, 'loss:', loss.numpy())
def train():
for epoch in range(300):
train_epoch(epoch)
if __name__ == '__main__':
train()
4. Pycharm无法对TensorFlow2.0中的Keras模块进行自动补全的解决办法
- TensorFlow2.0之后,Keras作为其主要模块被官方推荐使用。但是在pycharm中使用tensorflow.keras总是无法弹出自动补全,导致编码效率过慢。其实原因很简单,这就是Pycharm的一个bug。下载使用Pycharm2019.3之后的版本就能解决。
- 当前(2019年11月21日)2019.3版本还没有正式发布,可以使用Pycharm EAP版本,也就是测试版,链接在此:点我下载
5. 需要全套课程视频+PPT+代码资源可以私聊我
- 方式1:CSDN私信我!
- 方式2:
QQ邮箱
:594042358@qq.com
或者直接加我QQ
: 594042358
!