随着社交网络的普及,越来越多的用户通过facebook等社交平台联系到了一起。本文将基于facebook的好友关系数据,研究用户分布规律,并提供简单的好友推荐算法。
数据来源
KONECT http://konect.uni-koblenz.de/networks/facebook-wosn-links
数据原始格式
. txt文本格式,空格分隔
. 注释信息以%开头
. 每行一组数据,共四个字段
第一字段:用户ID1
第二字段:用户ID2
第三字段:用途不明
第四字段:好友关系建立时间,多数为0,数据缺失
% sym unweighted
% 817035 63731 63731
1 2 1 0
1 3 1 0
1 4 1 0
2 3 1 1183325626
2 7 1 0
2 9 1 1187651286
...
- 1
- 2
- 3
- 4
- 5
- 6
- 7
- 8
- 9
- 10
- 11
- 12
- 13
- 14
- 15
- 16
- 17
- 1
- 2
- 3
- 4
- 5
- 6
- 7
- 8
- 9
- 10
- 11
- 12
- 13
- 14
- 15
- 16
- 17
Note:好友信息以无向图的形式存储,不存在重复链接,保证第一字段用户ID始终小于第二字段用户ID;从实例数据中我们可以看到用户1和用户2是好友,但只存在于用户1的好友记录里,而不会在用户2的好友记录里重复出现
数据清洗
需要从原始数据中清除这些:
- 以%开头的注释行
- 不明用途的第三字段
- 数据缺失的第四字段
cat facebook-wosn-links.txt | sed '/%/d' |
awk '{print $1" "$2}' > facebook-wosn-links-clean.txt
数据读入
利用R语言read.table函数以table格式存储数据
friends.whole <- read.table("Your File Address",
header=FALSE, sep=" ", col.names=c("from","to"))
数据选取
数据中包含非常多的用户,数据间相互影响,为了使结果更清晰,我们选定某一用户,分析其好友的分布特点
library(igraph)
# 将所有用户按照好友数量倒序排序
sort(table(c(friends.whole$from, friends.whole$to)), dec=T)
# 选定拥有合适好友数量的用户
uid <- 979
# 好友ID
friends.connected <- unique(c(friends.whole$to[friends.whole$from == uid],
friends.whole$from[friends.whole$to == uid]))
# 选取该用户所有好友
friends.sample <- friends.whole[
((friends.whole$from %in% friends.connected) &
(friends.whole$to %in% friends.connected)), c(1,2)]
# 创建graph对象,并去除循环
friends.graph <- graph.data.frame(d = friends.sample,
directed = F, vertices = unique(c(friends.sample$from,
friends.sample$to)))
friends.graph <- simplify(friends.graph)
is.simple(friends.graph)
# 去除孤立的点,其实本例中并不存在孤立点,但作为标准化操作保留
dg <- degree(friends.graph)
friends.graph <- induced.subgraph(friends.graph,
which(dg > 0))
- 1
- 2
- 3
- 4
- 5
- 6
- 7
- 8
- 9
- 10
- 11
- 12
- 13
- 14
- 15
- 16
- 17
- 18
- 19
- 20
- 21
- 22
- 23
- 24
- 25
- 26
- 27
- 28
- 29
- 1
- 2
- 3
- 4
- 5
- 6
- 7
- 8
- 9
- 10
- 11
- 12
- 13
- 14
- 15
- 16
- 17
- 18
- 19
- 20
- 21
- 22
- 23
- 24
- 25
- 26
- 27
- 28
- 29
好友分布图
plot(friends.graph, layout = layout.fruchterman.reingold,
vertex.size = 2.5, vertex.label = NA, edge.color =
grey(0.5), edge.arrow.mode = "-")
从下图可以看出,好友的分布具有一定的聚集性
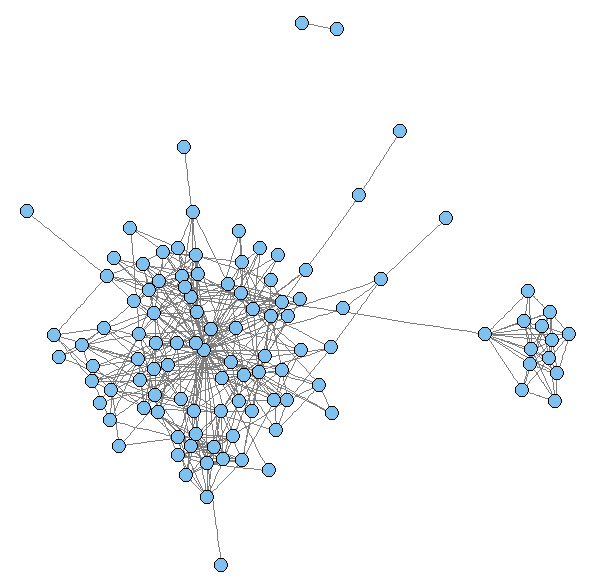
接下来,我们希望将不同群体的好友用不同的颜色标明出来,提供类似于好友自动分组的功能;使用的是igraph包提供的walktrap.community函数
friends.com = walktrap.community(friends.graph, steps=10)
# 返回每个节点的分组结果
V(friends.graph)$sg = friends.com$membership
# 按照分组结果赋予节点不同的颜色
V(friends.graph)$color = NA
V(friends.graph)$color = rainbow(max(V(friends.graph)$sg))[V(friends.graph)$sg]
plot(friends.graph, layout = layout.fruchterman.reingold,
vertex.size = 5, vertex.color = V(friends.graph)$color,
vertex.label = NA, edge.color = grey(0.5), edge.arrow.mode = "-")
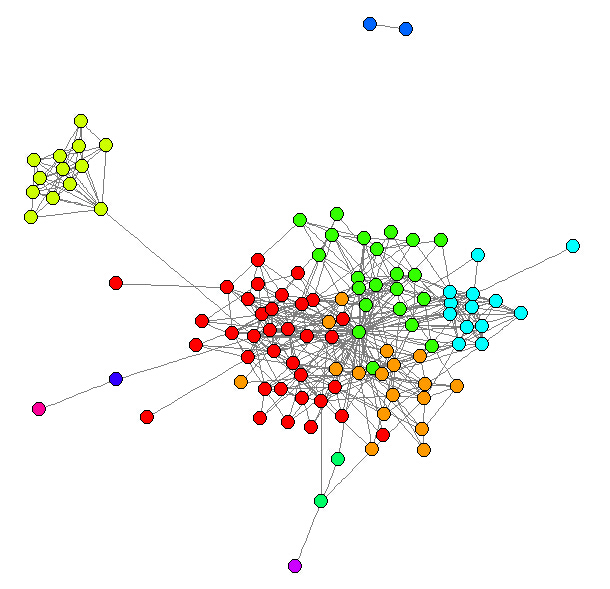
在图中,有些用户是中间人的角色,连接了两个聚集,我们可以利用igraph包提供的betweenness函数找出他们
V(friends.graph)$btn = betweenness(friends.graph, directed = F)
plot(V(friends.graph)$btn, xlab="Vertex", ylab="Betweenness")
betweenness函数统计的是通过每个节点的最短路径的数量,该值越高,则表明该节点作为中间节点的作用越强
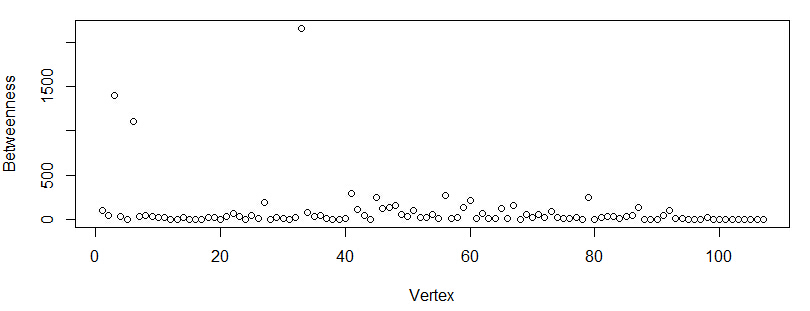
从图上看出,有3个节点的betweenness值明显高于其他节点,因此我们可以选取betweenness值500为阙值将这3个节点在图上标明出来
V(friends.graph)$size = 5
V(friends.graph)[btn>=500]$size = 15
V(friends.graph)$label = NA
V(friends.graph)[btn>=500]$label = V(friends.graph)[btn>=500]$name
plot(friends.graph, layout = layout.fruchterman.reingold,
vertex.size = V(friends.graph)$size,
vertex.color = V(friends.graph)$color,
vertex.label = V(friends.graph)$label,
edge.color = grey(0.5),
edge.arrow.mode = "-")
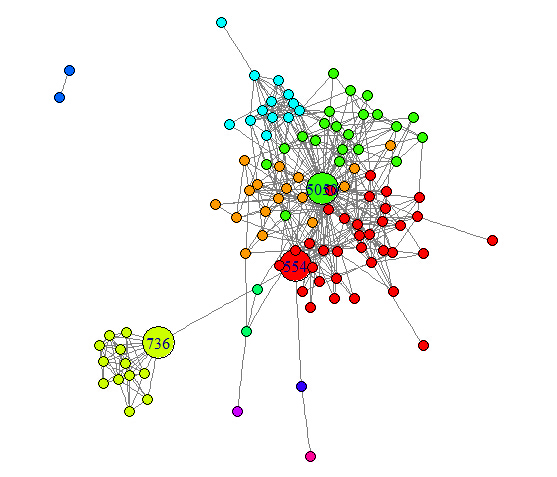
最后,我们希望从二级好友中找出一些用户来进行推荐,可以根据共同好友数量来选取
# 所有二级好友
friends.2nd <- c(friends.whole$from[
(friends.whole$to %in% friends.connected)],
friends.whole$to[
(friends.whole$from %in% friends.connected)])
# 该用户本身也会被包含到二级好友中,需要剔除
friends.2nd <- friends.2nd[friends.2nd!=uid]
# 按共同好友的数量进行倒序排序
friends.recommand <- sort(table(friends.2nd), dec = T)
# 取出共同好友最多的10个用户来推荐
friends.recommand <- friends.recommand[1:10]
> friends.recommand[1:10]
friends.2nd
用户ID 5050 5559 6896 5587 7659 6255 14521 554 5586 6062
共同好友数量 63 22 20 19 18 17 16 15 15 15
- 1
- 2
- 3
- 4
- 5
- 6
- 7
- 8
- 9
- 10
- 11
- 12
- 13
- 14
- 15
- 16
- 17
- 18
- 19
- 1
- 2
- 3
- 4
- 5
- 6
- 7
- 8
- 9
- 10
- 11
- 12
- 13
- 14
- 15
- 16
- 17
- 18
- 19
根据共同好友数量的推荐算法虽然较为简单,但只要网络本身包含较多的真实线下好友关系,推荐的结果还是非常有价值的。此外,还有许多推荐算法,如根据用户的属性(兴趣爱好,地理位置等)来做聚类推荐,我们将在以后的文章中进行探讨。
参考资料:http://cos.name/2011/04/exploring-renren-social-network/