so it makes sense to zoom in on that part of the graph,and to transform the data to emphasize differences
| 所以放大图的那一部分并转换数据以强调差异是有意义的
|
Cumulative distribution functions The class size paradox(悖论)
|
|
Suppose that a college offers 65 classes in a given semester(学期), with the following distribution of sizes
|
|
Unbias estimation 无偏估计检测 unbias estimator无偏估计
|
|
Scales(缩放) the freq/prob associated with the value x
|
|
It might not be obvious why this works, but since it is easier to implement than to explain, let’s try it out. | 它可能并不是显而易见的,但是因为它更容易实现比解释,让我们试试看。
|
The distributions we have used so far are called empirical distributions because they are based on empirical observations, which are necessarily finite samples.
The alternative is a continuous distribution, which is characterized by a CDF that is a continuous function (as opposed to a step function). Many real world phenomena can be approximated by continuous distributions.
| 替代方案是连续分布,其特点是CDF是一个连续函数(与阶梯函数相反)。许多现实世界现象可以通过连续分布近似。
|
In the real world, exponential distributions come up when we look at a series of events and measure the times between events, which are called interarrival times. If the events are equally likely to occur at any time, the distribution of interarrival times tends to look like an exponential distribution.
| |
For small values of n, we don’t expect an empirical distribution to fit a continuous distribution exactly. One way to evaluate the quality of fit is to generate a sample from a continuous distribution and see how well it matches the data. For the exponential, Pareto and Weibull distributions, there are simple transformations we can use to test whether a continuous distribution is a good model of a dataset.
| 对于n的小值,我们并不期望经验分布恰好适合连续分布。评估拟合质量的一种方法是从连续分布中生成一个样本,并查看它与数据的匹配程度。
|
For the normal distribution there is no such transformation, but there is an alternative called a normal probability plot. It is based on rankits: if you generate n values from a normal distribution and sort them, the kth rankit is the mean of the distribution for the kth value.
| 对于正态分布,没有这种转换,但有一个替代方案称为正常概率图。它基于rankits:如果你从正态分布生成n个值并对它们进行排序,即第k个等级 是第k个值的分布的均值。
|
Plot the sorted values from your dataset versus the random values
| 绘制您的数据集与随机值的排序值
|
If you assume that each attempt is independent of previous attempts, you will see occasional long strings of successes or failures. These apparent streaks are not sufficient evidence that there is any relationship between successive attempts.
| 如果你认为每次尝试独立于以前的尝试,你会偶尔看到长串的成功或失败。 这些明显的条纹是不够的证明连续尝试之间有任何关系。
|
A related phenomenon is the clustering illusion, which is the tendency to see clusters in spatial patterns that are actually random
| 一个相关的现象是聚类错觉,这就是倾向于看到空间模式中的聚类实际上是随机的
|
To test whether an apparent cluster is likely to be meaningful, we can simulate the behavior of a random system to see whether it is likely to produce a similar cluster. This process is called Monte Carlo simulation because generating random numbers is reminiscent of casino games (and Monte Carlo is famous for its casino).
| 为了测试一个明显的聚类是否可能是有意义的,我们可以模拟一个随机系统的行为,看它是否有可能产生一个类似的集群。这个过程被称为蒙特卡洛模拟,因为生成随机数字让人想起赌场游戏(和蒙特卡罗以赌场而闻名)。
|
The number of values you need before you see convergence depends on the skewness of the distribution. Sums from an exponential distribution converge for small sample sizes. Sums from a lognormal distribution do not.
| 在你看到收敛之前你需要的数值取决于分布的偏度。对于小样本量,指数分布的和收敛。来自对数正态分布的总和不。
|
More specifically, if the distribution of the values has mean and standard deviation m and s, the distribution of the sum is approximately N(nm, ns2). | 更具体地说,如果这些值的分布具有平均值和标准偏差m和s,总和的分布近似为N(nm,ns2)。
|
This is called the Central Limit Theorem. It is one of the most useful tools for statistical analysis, but it comes with caveats: • The values have to be drawn independently. • The values have to come from the same distribution (although this requirement can be relaxed). • The values have to be drawn from a distribution with finite mean and variance, so most Pareto distributions are out. • The number of values you need before you see convergence depends on the skewness of the distribution. Sums from an exponential distribution converge for small sample sizes. Sums from a lognormal distribution do not.
| |
|
|
|
|
统计—thinkstat英语学习
最新推荐文章于 2018-12-02 02:03:03 发布
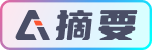