一、Python所有方向的学习路线
Python所有方向的技术点做的整理,形成各个领域的知识点汇总,它的用处就在于,你可以按照下面的知识点去找对应的学习资源,保证自己学得较为全面。
二、Python必备开发工具
工具都帮大家整理好了,安装就可直接上手!
三、最新Python学习笔记
当我学到一定基础,有自己的理解能力的时候,会去阅读一些前辈整理的书籍或者手写的笔记资料,这些笔记详细记载了他们对一些技术点的理解,这些理解是比较独到,可以学到不一样的思路。
四、Python视频合集
观看全面零基础学习视频,看视频学习是最快捷也是最有效果的方式,跟着视频中老师的思路,从基础到深入,还是很容易入门的。
五、实战案例
纸上得来终觉浅,要学会跟着视频一起敲,要动手实操,才能将自己的所学运用到实际当中去,这时候可以搞点实战案例来学习。
六、面试宝典
简历模板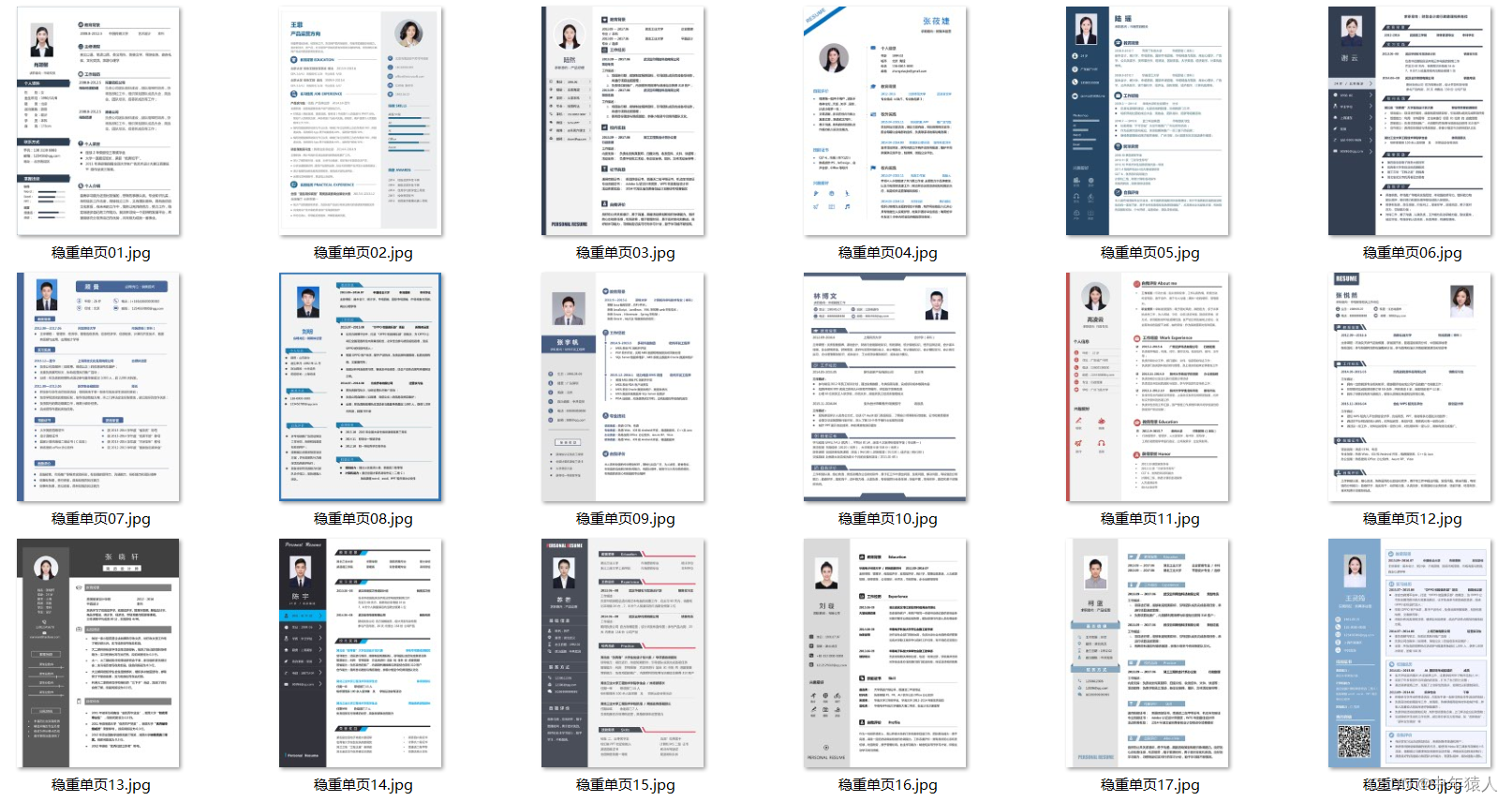
网上学习资料一大堆,但如果学到的知识不成体系,遇到问题时只是浅尝辄止,不再深入研究,那么很难做到真正的技术提升。
一个人可以走的很快,但一群人才能走的更远!不论你是正从事IT行业的老鸟或是对IT行业感兴趣的新人,都欢迎加入我们的的圈子(技术交流、学习资源、职场吐槽、大厂内推、面试辅导),让我们一起学习成长!
Import helper functions
from mlfromscratch.utils import divide_on_feature, train_test_split, get_random_subsets, normalize
from mlfromscratch.utils import accuracy_score, calculate_entropy
from mlfromscratch.unsupervised_learning import PCA
from mlfromscratch.supervised_learning import ClassificationTree
from mlfromscratch.utils.misc import bar_widgets
from mlfromscratch.utils import Plot
class RandomForest():
“”"Random Forest classifier. Uses a collection of classification trees that
trains on random subsets of the data using a random subsets of the features.
Parameters:
n_estimators: int
The number of classification trees that are used.
max_features: int
The maximum number of features that the classification trees are allowed to
use.
min_samples_split: int
The minimum number of samples needed to make a split when building a tree.
min_gain: float
The minimum impurity required to split the tree further.
max_depth: int
The maximum depth of a tree.
“”"
def init(self, n_estimators=100, max_features=None, min_samples_split=2,
min_gain=0, max_depth=float(“inf”)):
self.n_estimators = n_estimators # Number of trees
self.max_features = max_features # Maxmimum number of features per tree
self.min_samples_split = min_samples_split
self.min_gain = min_gain # Minimum information gain req. to continue
self.max_depth = max_depth # Maximum depth for tree
self.progressbar = progressbar.ProgressBar(widgets=bar_widgets)
Initialize decision trees
self.trees = []
for _ in range(n_estimators):
self.trees.append(
ClassificationTree(
min_samples_split=self.min_samples_split,
min_impurity=min_gain,
max_depth=self.max_depth))
def fit(self, X, y):
n_features = np.shape(X)[1]
If max_features have not been defined => select it as
sqrt(n_features)
if not self.max_features:
self.max_features = int(math.sqrt(n_features))
Choose one random subset of the data for each tree
subsets = get_random_subsets(X, y, self.n_estimators)
for i in self.progressbar(range(self.n_estimators)):
X_subset, y_subset = subsets[i]
Feature bagging (select random subsets of the features)
idx = np.random.choice(range(n_features), size=self.max_features, replace=True)
Save the indices of the features for prediction
self.trees[i].feature_indices = idx
最后
不知道你们用的什么环境,我一般都是用的Python3.6环境和pycharm解释器,没有软件,或者没有资料,没人解答问题,都可以免费领取(包括今天的代码),过几天我还会做个视频教程出来,有需要也可以领取~
给大家准备的学习资料包括但不限于:
Python 环境、pycharm编辑器/永久激活/翻译插件
python 零基础视频教程
Python 界面开发实战教程
Python 爬虫实战教程
Python 数据分析实战教程
python 游戏开发实战教程
Python 电子书100本
Python 学习路线规划
网上学习资料一大堆,但如果学到的知识不成体系,遇到问题时只是浅尝辄止,不再深入研究,那么很难做到真正的技术提升。
一个人可以走的很快,但一群人才能走的更远!不论你是正从事IT行业的老鸟或是对IT行业感兴趣的新人,都欢迎加入我们的的圈子(技术交流、学习资源、职场吐槽、大厂内推、面试辅导),让我们一起学习成长!