#!/usr/bin/python3 # -*-coding:utf-8 -*- # @Time :2018/3/14 # @Author :machuanbin """ tensorflow :1.3.0 pandas: 0.19.2 """ import tensorflow as tf import os from tensorflow.examples.tutorials.mnist import input_data from tensorflow.contrib import rnn import numpy as np config=tf.ConfigProto() # config.gup_options.allow_growth=True sess=tf.Session(config=config) current_dir = os.path.abspath('.\MNIST_data') mnist=input_data.read_data_sets(current_dir,one_hot=True) # print(mnist.train.images.shape) lr=1e-3 #在训练和测试的时候,我们想用不同的batch_size所以采用占位符 batch_size=tf.placeholder(tf.int32,[]) keep_prob=tf.placeholder(tf.float32,[]) # 每个时刻的输入特征是28维的,就是每个时刻输入一行,一行有 28 个像素 input_size=28 # 时序持续长度为28,即每做一次预测,需要先输入28<
MultiLSTM预测Mnist
最新推荐文章于 2018-03-17 13:51:48 发布
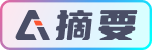