背景
我们云平台打造新一代数据湖存储,从kafka实时读取海量数据入湖,需要经受性能,稳定性等各方面考验。同时历史影响,kafka中数据格式牵扯业务非常广,难以修改。要在这种情况下完成优化入湖操作。由于性能压测过程中发现hudi官方自带的数据入湖工具不足以支撑我们海量数据(五分钟峰值2百万)入库,针对我们业务场景,需要进行优化,使其使用最低资源进行最大限度入湖。
关键字
Hudi:数据湖存储引擎,版本为0.12.1
Avro:Avro是Hadoop的一个数据序列化系统,设计用于支持大批量数据交换的应用。
Json:一种轻量级的数据交换格式。
Scheam:数据库元数据的一个抽象集合。
官方入湖方式介绍
Hudi 在utilities中提供了一个 DeltaStreamer工具从外部数据源读取数据并写入新的Hudi表。HoodieDeltaStreamer提供了从DFS或Kafka等不同来源进行摄取的方式,并具有以下功能。
从Kafka单次摄取新事件,从Sqoop、HiveIncrementalPuller输出或DFS文件夹中的多个文件 增量导入
支持json、avro或自定义记录类型的传入数据
管理检查点,回滚和恢复
利用DFS或Confluentschema注册表的Avro模式。
支持自定义转换操作
除了上述官网说的几项,也支持读取Hive表等(历史数据)转化Hudi表,源码里还有其他的工具类,可以自行查阅源码发掘
命令行选项更详细地描述了这些功能:
[root@ha1 bin]# ./spark-submit --class org.apache.hudi.utilities.deltastreamer.HoodieDeltaStreamer --help
Options:
--master MASTER_URL spark://host:port, mesos://host:port, yarn,
k8s://https://host:port, or local (Default: local[*]).
--deploy-mode DEPLOY_MODE Whether to launch the driver program locally ("client") or
on one of the worker machines inside the cluster ("cluster")
(Default: client).
--class CLASS_NAME Your application's main class (for Java / Scala apps).
--name NAME A name of your application.
--jars JARS Comma-separated list of jars to include on the driver
and executor classpaths.
--packages Comma-separated list of maven coordinates of jars to include
on the driver and executor classpaths. Will search the local
maven repo, then maven central and any additional remote
repositories given by --repositories. The format for the
coordinates should be groupId:artifactId:version.
--exclude-packages Comma-separated list of groupId:artifactId, to exclude while
resolving the dependencies provided in --packages to avoid
dependency conflicts.
--repositories Comma-separated list of additional remote repositories to
search for the maven coordinates given with --packages.
--py-files PY_FILES Comma-separated list of .zip, .egg, or .py files to place
on the PYTHONPATH for Python apps.
--files FILES Comma-separated list of files to be placed in the working
directory of each executor. File paths of these files
in executors can be accessed via SparkFiles.get(fileName).
--archives ARCHIVES Comma-separated list of archives to be extracted into the
working directory of each executor.
--conf, -c PROP=VALUE Arbitrary Spark configuration property.
--properties-file FILE Path to a file from which to load extra properties. If not
specified, this will look for conf/spark-defaults.conf.
--driver-memory MEM Memory for driver (e.g. 1000M, 2G) (Default: 1024M).
--driver-java-options Extra Java options to pass to the driver.
--driver-library-path Extra library path entries to pass to the driver.
--driver-class-path Extra class path entries to pass to the driver. Note that
jars added with --jars are automatically included in the
classpath.
--executor-memory MEM Memory per executor (e.g. 1000M, 2G) (Default: 1G).
--proxy-user NAME User to impersonate when submitting the application.
This argument does not work with --principal / --keytab.
--help, -h Show this help message and exit.
--verbose, -v Print additional debug output.
--version, Print the version of current Spark.
Cluster deploy mode only:
--driver-cores NUM Number of cores used by the driver, only in cluster mode
(Default: 1).
Spark standalone or Mesos with cluster deploy mode only:
--supervise If given, restarts the driver on failure.
Spark standalone, Mesos or K8s with cluster deploy mode only:
--kill SUBMISSION_ID If given, kills the driver specified.
--status SUBMISSION_ID If given, requests the status of the driver specified.
Spark standalone, Mesos and Kubernetes only:
--total-executor-cores NUM Total cores for all executors.
Spark standalone, YARN and Kubernetes only:
--executor-cores NUM Number of cores used by each executor. (Default: 1 in
YARN and K8S modes, or all available cores on the worker
in standalone mode).
Spark on YARN and Kubernetes only:
--num-executors NUM Number of executors to launch (Default: 2).
If dynamic allocation is enabled, the initial number of
executors will be at least NUM.
--principal PRINCIPAL Principal to be used to login to KDC.
--keytab KEYTAB The full path to the file that contains the keytab for the
principal specified above.
Spark on YARN only:
--queue QUEUE_NAME The YARN queue to submit to (Default: "default").
入湖数据结构
我们的数据是在kafka中的,kafka中的value是json格式,格式统一但比较复杂嵌套较多。
{
"recvTime": "20221115200000",
"key": "XXX",
"dataList": [
{
"type": "7",
"chnnlId": "1",
"data": [
{
"val": "1.0",
"id": "1"
}
],
"code": "1",
"msgTime": "20221115195300"
}
],
"sysTime": "2022-11-15 19:53:11",
"id": "630217"
}
对于要入库的表字段需要将dataList中的数据拆开,单独做一行数据,key这层的则公用。具体如下
CREATE TABLE IF NOT EXISTS `hudi`.`ods`.`ods_mor_hudi_sungrow_ts`(
`topic` STRING COMMENT 'kafka topic',
`offset` BIGINT COMMENT 'kafka topic message offset in topic',
`kafka_partition` INT COMMENT 'message partition in topic',
`event_time` TIMESTAMP(3) COMMENT 'message timestamp in kafka',
`rowkey` STRING NOT NULL,
`recv_time` STRING NOT NULL ,
`key` STRING NOT NULL ,
`sys_time` STRING ,
`id` STRING NOT NULL,
`type` STRING ,
`chnnl_id` STRING,
`code` STRING COMMENT '设备编号',
`msg_time` STRING COMMENT '事件时间 times yyyyMMddHHmm00',
`data` STRING COMMENT '测点PID与PVAL map',
`pt` STRING NOT NULL COMMENT '数据分区字段,yyyyMMdd'
)COMMENT '遥测数据hudi表'
入湖实现
官方有提供一个JsonKafkaSource 的source工具,但这个工具不适合我们这种复杂的解析。所以想到用官方提供转换工具进行转换,转换工具可以使用sql,也可以使用代码,代码需要继承Transformer类。我们使用代码方式,写完后打jar包放到spark的jars目录即可。示例如下:
public class TransformerHs implements Transformer, Serializable {
private static final Logger LOG = LoggerFactory.getLogger(TransformerHs.class);
private Gson gson = new Gson();
private static List<StructField> fields;
static {
// hudi表中的字段
fields = new ArrayList<>();
fields.add(DataTypes.createStructField("key", DataTypes.StringType, false));
fields.add(DataTypes.createStructField("recvTime", DataTypes.StringType, true));
fields.add(DataTypes.createStructField("sysTime", DataTypes.StringType, true));
fields.add(DataTypes.createStructField("msgTime", DataTypes.StringType, true));
fields.add(DataTypes.createStructField("uuid", DataTypes.StringType, false));
fields.add(DataTypes.createStructField("data", DataTypes.StringType, true));
}
// 实现的转换方式
@Override
public Dataset<Row> apply(JavaSparkContext jsc, SparkSession sparkSession, Dataset<Row> rowDataset, TypedProperties typedProperties) {
JavaRDD<Row> rowJavaRdd = rowDataset.toJavaRDD();
LOG.info("lwy apply ");
List<Row> rowList = new ArrayList<>();
Row tmp = null;
for (Row row : rowJavaRdd.collect()) {
// 每条数据调用转换函数,进行转换
tmp = buildRow(row);
if (null != tmp) {
rowList.add(tmp);
}
}
JavaRDD<Row> stringJavaRdd = jsc.parallelize(rowList);
List<StructField> fields = new ArrayList<>();
if (CollectionUtils.isEmpty(fields)) {
builFields(fields);
}
StructType schema = DataTypes.createStructType(fields);
// 根据类型构建schema,将数据转换成DataSet
Dataset<Row> dataFrame = sparkSession.createDataFrame(stringJavaRdd, schema);
return dataFrame;
}
// 实现转换方式
private Row buildRow(Row row) {
Row returnRow = null;
try {
String key = row.getString(0);
String recv_time = row.getString(1);
String sys_time = row.getString(2);
String dataList = row.getString(4);
List<Map<String, Object>> list = gson.fromJson(dataList, List.class);
String msg_time = (String) list.get(0).get("msgTime");
if (StringUtils.isBlank(key)) {
return returnRow;
}
List<Map<String, String>> datas = (List<Map<String, String>>) list.get(0).get("data");
Map<String, String> data = new HashMap<>();
for (Map<String, String> tmp : datas) {
data.put(tmp.get("id"), tmp.get("val"));
}
data.put("recvTime", recv_time);
returnRow = RowFactory.create(key, recv_time, sys_time,msg_time, gson.toJson(data));
} catch (Exception e) {
LOG.warn("此行数据,报错了={}", row);
}
return returnRow;
}
}
除了实现此方式,还需要自定义输入输出的格式:
public class DataSchemaProviderHs extends SchemaProvider {
private static Schema sourceSchema;
private static Schema targetSchema;
public DataSchemaProviderHs(TypedProperties props, JavaSparkContext jssc) {
super(props, jssc);
}
@Override
public Schema getSourceSchema() {
if (null == sourceSchema) {
return new Schema.Parser().parse("{\"type\":\"record\",\"name\":\"test_name\",\"fields\":[{\"name\":\"key\",\"type\":[\"string\",\"null\"]},{\"name\":\"recvTime\",\"type\":[\"string\",\"null\"]},{\"name\":\"sysTime\",\"type\":[\"string\",\"null\"]},{\"name\":\"dataList\",\"type\":[\"string\",\"null\"]}]}");
} else {
return sourceSchema;
}
}
@Override
public Schema getTargetSchema() {
if (null == targetSchema) {
return new Schema.Parser().parse("{\"type\":\"record\",\"name\":\"test_name\",\"fields\":[{\"name\":\"key\",\"type\":[\"string\",\"null\"]},{\"name\":\"recvTime\",\"type\":[\"string\",\"null\"]},{\"name\":\"sysTime\",\"type\":[\"string\",\"null\"]},{\"name\":\"msgTime\",\"type\":[\"string\",\"null\"]},{\"name\":\"uuid\",\"type\":[\"string\",\"null\"]},{\"name\":\"data\",\"type\":[\"string\",\"null\"]}]}");
} else {
return targetSchema;
}
}
}
启动脚本
/soft/spark3.2.3/bin/spark-submit \
--master yarn \
--deploy-mode cluster \
--name test_to_hudi \
--queue test \
--conf 'spark.serializer=org.apache.spark.serializer.KryoSerializer' \
--conf 'spark.sql.extensions=org.apache.spark.sql.hudi.HoodieSparkSessionExtension' \
--conf 'spark.sql.catalog.spark_catalog=org.apache.spark.sql.hudi.catalog.HoodieCatalog' \
--conf 'spark.dynamicAllocation.enabled=false' \
--conf 'spark.executor.heartbeatInterval=4000' \
--conf 'spark.local.dir=/data1/hadoop/tmp/nm-local-dir,/data2/hadoop/tmp/nm-local-dir,/data3/hadoop/tmp/nm-local-dir' \
--conf 'spark.default.parallelism=256' \
--conf 'spark.sql.shuffle.partitions=256' \
--conf 'spark.driver.maxResultSize=16g' \
--conf 'spark.rpc.message.maxSize=2047' \
--driver-memory 32g --executor-memory 8g --executor-cores 2 --num-executors 16 \
--conf spark.kryoserializer.buffer=1024m \
--class org.apache.hudi.utilities.deltastreamer.HoodieDeltaStreamer spark-internal \
--props hdfs://mycluster/user/spark/conf/test_table.properties \
--target-base-path hdfs://mycluster/user/hive/warehouse/ods.db/test_table \
--table-type MERGE_ON_READ --target-table test_table \
--source-ordering-field key \
--source-class org.apache.hudi.utilities.sources.JsonKafkaSource \
--schemaprovider-class XXXX.DataSchemaProviderTs \
--op UPSERT \
--continuous \
--enable-hive-sync \
--source-limit 10000000
缺点
数据量大时,driver端出现瓶颈,因为所有数据要返回到driver端进行转换。
Json转换无法拿到kafka中相关信息,例如offset等
监控上查看,--source-limit配置到200w就是极限了。
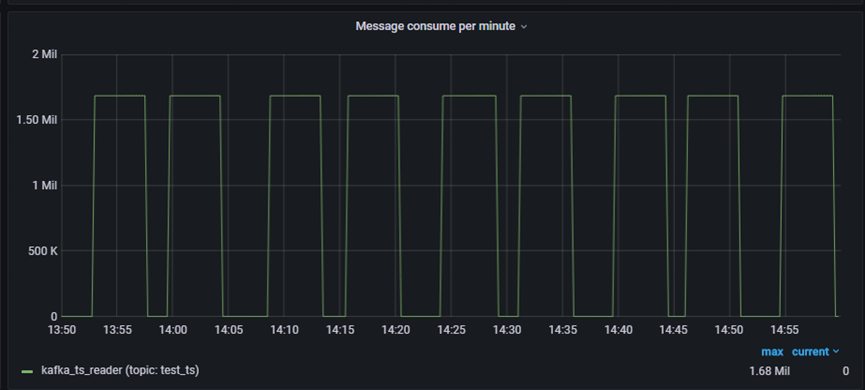
优化
源码分析
分析hudi入库源码,是批量获取数据的方法,根据不同数据源实现不同的获取数据接口。入库时通过源类型,获取数据,然后根据再进行转换。
我们可以看到case AVRO: 这个分支,是最优的,从获取数据后直接就是GenericRecord格式,不需要像json那样还需要单独拉回来转一遍。把所有的转换放到extcutor中转换,分散转换压力。
理论有了,咱开干。
public InputBatch<JavaRDD<GenericRecord>> fetchNewDataInAvroFormat(Option<String> lastCkptStr, long sourceLimit) {
switch (source.getSourceType()) {
case AVRO:
// 这里返回的直接是avro格式
return ((AvroSource) source).fetchNext(lastCkptStr, sourceLimit);
case JSON: {
// 这里返回的rdd里面还是json字符串
InputBatch<JavaRDD<String>> r = ((JsonSource) source).fetchNext(lastCkptStr, sourceLimit);
// 获取avro格式器
AvroConvertor convertor = new AvroConvertor(r.getSchemaProvider().getSourceSchema());
// 将json字符串转换成source的avro格式
return new InputBatch<>(Option.ofNullable(r.getBatch().map(rdd -> rdd.map(convertor::fromJson)).orElse(null)),
r.getCheckpointForNextBatch(), r.getSchemaProvider());
}
case ROW: {
InputBatch<Dataset<Row>> r = ((RowSource) source).fetchNext(lastCkptStr, sourceLimit);
return new InputBatch<>(Option.ofNullable(r.getBatch().map(
rdd -> {
SchemaProvider originalProvider = UtilHelpers.getOriginalSchemaProvider(r.getSchemaProvider());
return (originalProvider instanceof FilebasedSchemaProvider)
? HoodieSparkUtils.createRdd(rdd, HOODIE_RECORD_STRUCT_NAME, HOODIE_RECORD_NAMESPACE, true,
org.apache.hudi.common.util.Option.ofNullable(r.getSchemaProvider().getSourceSchema())
).toJavaRDD() : HoodieSparkUtils.createRdd(rdd,
HOODIE_RECORD_STRUCT_NAME, HOODIE_RECORD_NAMESPACE, false, Option.empty()).toJavaRDD();
})
.orElse(null)), r.getCheckpointForNextBatch(), r.getSchemaProvider());
}
default:
throw new IllegalArgumentException("Unknown source type (" + source.getSourceType() + ")");
}
}
自定义Source
自定义AvroSource格式数据,实现从kafka读取数据。实现方式与JsonSource类似,不同点在于返回格式不一样,一个是返回。
自定义SungrowKafkaSource类,继承AvroSource,因为我们最终是要他返回AVRO类型
fetchNewData中实现从kafka获取数据,
toRDD方法,将数据转换成spark RDD,在rdd中加入map操作,实现数据由json转换成avro,同时将脏数据filter。
public class SungrowKafkaSource extends AvroSource {
private static final Logger LOG = LogManager.getLogger(TsSungrowKafkaSource.class);
private final KafkaOffsetGen offsetGen;
private final HoodieDeltaStreamerMetrics metrics;
private final SchemaProvider schemaProvider;
public SungrowKafkaSource(TypedProperties properties, JavaSparkContext sparkContext, SparkSession sparkSession,
SchemaProvider schemaProvider, HoodieDeltaStreamerMetrics metrics) {
// 构造方法,初始化
super(properties, sparkContext, sparkSession, schemaProvider);
this.metrics = metrics;
properties.put("key.deserializer", StringDeserializer.class.getName());
properties.put("value.deserializer", StringDeserializer.class.getName());
offsetGen = new KafkaOffsetGen(properties);
this.schemaProvider = schemaProvider;
}
@Override
protected InputBatch<JavaRDD<GenericRecord>> fetchNewData(Option<String> lastCheckpointStr, long sourceLimit) {
try {
OffsetRange[] offsetRanges = offsetGen.getNextOffsetRanges(lastCheckpointStr, sourceLimit, metrics);
long totalNewMsgs = KafkaOffsetGen.CheckpointUtils.totalNewMessages(offsetRanges);
LOG.info("About to read " + totalNewMsgs + " from Kafka for topic :" + offsetGen.getTopicName());
if (totalNewMsgs <= 0) {
return new InputBatch<>(Option.empty(), KafkaOffsetGen.CheckpointUtils.offsetsToStr(offsetRanges));
}
// offserrange转换成rdd
JavaRDD<GenericRecord> newDataRDD = toRDD(offsetRanges);
return new InputBatch<>(Option.of(newDataRDD), KafkaOffsetGen.CheckpointUtils.offsetsToStr(offsetRanges));
} catch (org.apache.kafka.common.errors.TimeoutException e) {
throw new HoodieSourceTimeoutException("Kafka Source timed out " + e.getMessage());
}
}
private JavaRDD<GenericRecord> toRDD(OffsetRange[] offsetRanges) {
// 获取avro格式类
SungrowAvroConvertor convertor = new SungrowAvroConvertor(this.schemaProvider.getTargetSchema());
JavaRDD<GenericRecord> jsonStringRDD = KafkaUtils.createRDD(sparkContext,
offsetGen.getKafkaParams(),
offsetRanges,
LocationStrategies.PreferConsistent())
.filter(x -> !StringUtils.isNullOrEmpty((String) x.value()))
.map(obj -> {
try {
// 构建json转换工具
Gson gson = new Gson();
Map<String, Object> map = gson.fromJson((String) obj.value(), Map.class);
// 涉及自定义数据转换
.....
// 转换成avro
return convertor.fromJson(map);
} catch (Exception e) {
return null;
}
}).filter(Objects::nonNull);
return jsonStringRDD;
}
@Override
public void onCommit(String lastCkptStr) {
if (this.props.getBoolean(KafkaOffsetGen.Config.ENABLE_KAFKA_COMMIT_OFFSET.key(),
KafkaOffsetGen.Config.ENABLE_KAFKA_COMMIT_OFFSET.defaultValue())) {
offsetGen.commitOffsetToKafka(lastCkptStr);
}
}
}
提交脚本
/soft/spark3.2.3/bin/spark-submit \
--master yarn \
--deploy-mode cluster \
--name test_ts_to_hudi \
--queue test \
--conf 'spark.serializer=org.apache.spark.serializer.KryoSerializer' \
--conf 'spark.sql.extensions=org.apache.spark.sql.hudi.HoodieSparkSessionExtension' \
--conf 'spark.sql.catalog.spark_catalog=org.apache.spark.sql.hudi.catalog.HoodieCatalog' \
--conf 'spark.dynamicAllocation.enabled=false' \
--conf 'spark.executor.heartbeatInterval=4000' \
--conf 'spark.local.dir=/data1/hadoop/tmp/nm-local-dir,/data2/hadoop/tmp/nm-local-dir,/data3/hadoop/tmp/nm-local-dir' \
--conf 'spark.default.parallelism=256' \
--conf 'spark.sql.shuffle.partitions=2048' \
--conf 'spark.driver.maxResultSize=4g' \
--conf 'spark.rpc.message.maxSize=2047' \
--driver-memory 4g --executor-memory 8g --executor-cores 2 --num-executors 16 \
--props hdfs://mycluster/user/spark/conf/test_table.properties \
--target-base-path hdfs://mycluster/user/hive/warehouse/ods.db/test_table \
--table-type MERGE_ON_READ --target-table test_table \
--source-ordering-field key \
--source-class XXX.SungrowKafkaSource \
--schemaprovider-class XXXX.DataSchemaProviderTs \
--op UPSERT \
--continuous \
--enable-hive-sync \
--source-limit 20000000
测试结果
相比于之前,driver端资源减少了8倍,由原来的32G降到4G,executor资源不变。单次入库量由原来的100w提升到2000w,扩大20倍。
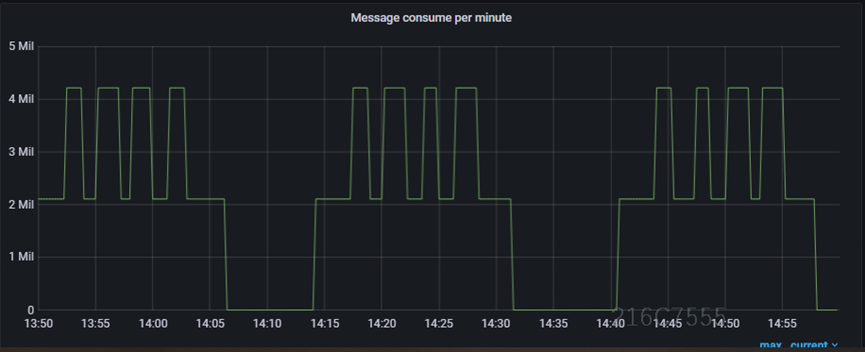
总结
通过自定义source的优化,我们5分钟遥测数据在800w体量下,能够稳定入库,单次入库量由原来的100w提升到2000w,扩大近20倍。为后面的压缩争取了足够时间。