论文链接:NDDR-CNN
论文摘要:
In this paper, we propose a novel Convolutional Neural Network (CNN) structure for general-purpose multi-task learning (MTL), which enables automatic feature fusing at every layer from different tasks. This is in contrast with the most widely used MTL CNN structures which empirically or heuristically share features on some specific layers (e.g., share all the features except the last convolutional layer). The proposed layerwise feature fusing scheme is formulated by combining existing CNN components in a novel way, with clear mathematical interpretability as discriminative dimensionality reduction, which is referred to as Neural Discriminative Dimensionality Reduction (NDDR).Specifically, we first concatenate features with the same spatial resolution from different tasks according to their channel dimension. Then, we show that the discriminative dimensionality reduction can be fulfilled by 1 × 1 Convolution,Batch Normalization, and Weight Decay in one CNN. The use of existing CNN components ensures the end-to-end training and the extensibility of the proposed NDDR layer to various state-of-the-art CNN architectures in a “plug-and-play” manner. The detailed ablation analysis shows that the proposed NDDR layer is easy to train and also robust to different hyperparameters. Experiments on different task sets with various base network architectures demonstrate the promising performance and desirable generalizability of our proposed method. The code of our paper is available at https://github.com/ethanygao/NDDR-CNN.
总体来说,本文提出了一个通用的多任务CNN学习框架,这个框架能够使用NDDR模块自动融合不同任务不同层的feature,这样就无需进行人为的硬性设计,最终达到即插即用的效果。
- NDDR Layer
NDDR是网络的主要组成部分,用来进行多任务特征的融合,以及特征的降维。当多个任务的不同层的feature进入NDDR层后,NDDR首先会将所有传入的feature在最后一维上进行拼接,如公式(1)所示: - Shortcuts
在网络训练过程中,为了防止低层的梯度消失,使用Shortcuts模块将梯度从最后一层直接传入到低层,跟残差网络有点类似。
根据对NDDR的分析可知,每个主线任务会多次接收来自NDDR层的feature,如果没有Shortcuts,那么每个任务只需使用最后一个NDDR层传出的feature进行计算即可。但是每个任务的Shortcuts层将此任务接收到的多个nddr-feature根据最后一个nddr-feature进行resize后进行拼接。然后使用(1,1)的卷积核卷积为最后一个nddr-feature的shape,进行计算。如下图所示:
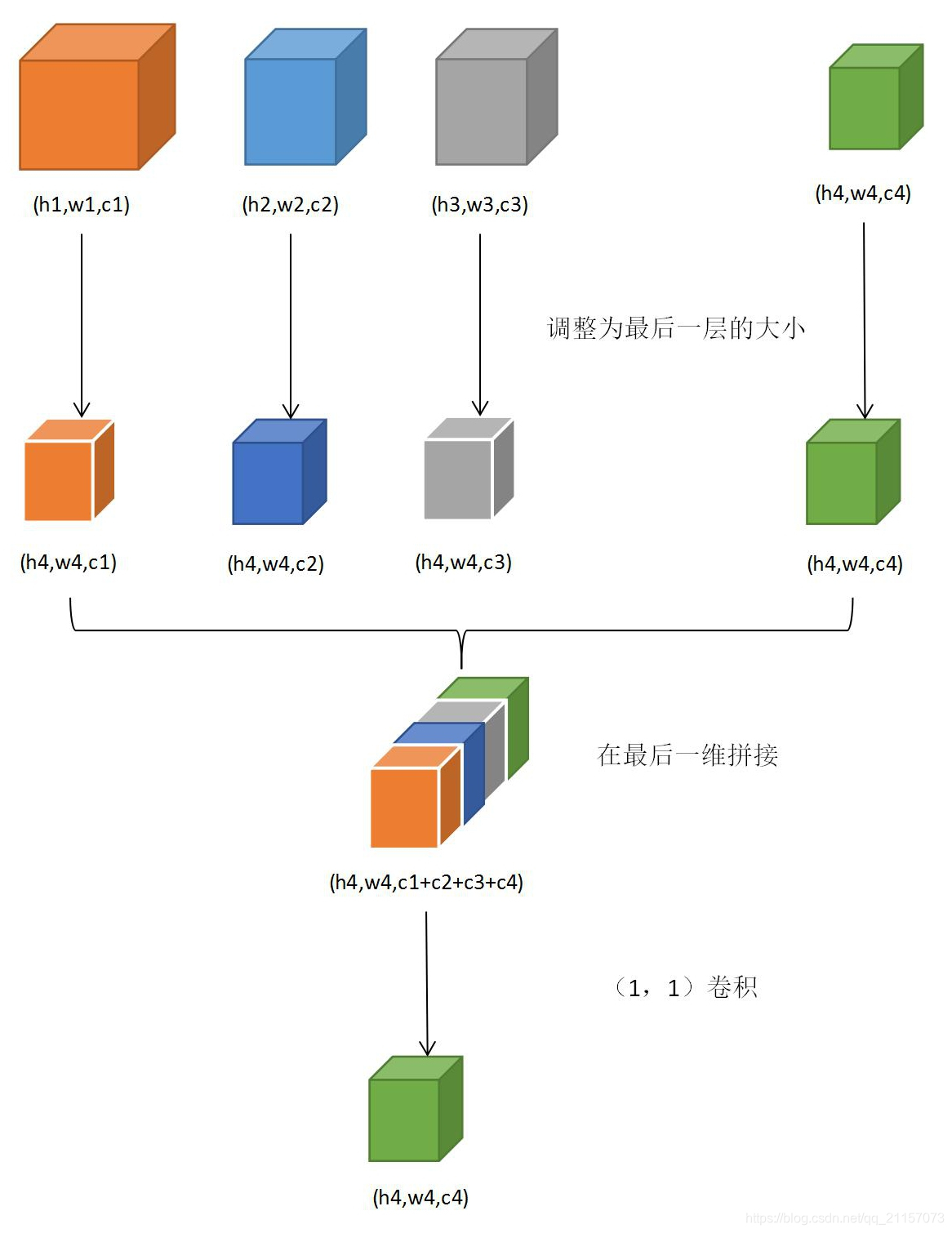
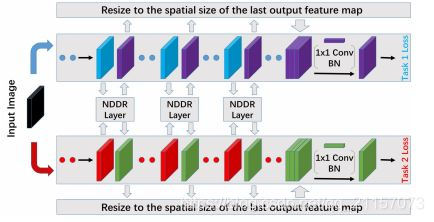
- 结果对比
论文分别采用了 VGG-16 和 ResNet-101作为基础网络。
训练了针对单个任务的网路:single task baseline;针对多多任务的启发式网络:multi-task baseline;并且论文还训练了与文章密切相关的两个网络:cross-stitch network和sluice network作为对比。
同时文章分别在Semantic Seg任务与Surface Normal Prediction任务中做了对比。
论文中还专门设计了实验,验证Shortcut模块的有效性。
此外,任务还进行了许多细节的处理。包括NDDR层的权重初始化以及学习率的选择等,都是可以借鉴的方式。