"""
如何得到一个数据流中的中位数?如果从数据流中读出奇数个数值,那么中位数就是所有数值排序后位于中间的数值。
如果从数据流中读出偶数个数值,那么中位数就是所有数值排序之后中间两个数的平均值
"""
"""
构建一个最大堆和一个最小堆,分别存储比中位数小的数和大的数。当目前两堆总数为偶数
的时候,把数字存入最大堆,然后重排最大堆,如果最大堆的堆顶数字大于最小堆堆顶数字,
则把两个堆顶数字交换,重排两堆,此时两堆数字总数为奇数,直接输出最大堆堆顶数字即
为中位数;如果当前两堆总数为技术的时候,把数字存入最小堆,重排最小堆,如果最大堆
的堆顶数字大于最小堆堆顶数字,则把两个堆顶数字交换,重排两堆,此时两堆数字总数为
偶数,取两堆堆顶数字做平均即为中位数。
如果从数据流中读出偶数个数值,那么中位数就是所有数值排序之后中间两个数的平均值。
我们使用Insert()方法读取数据流,使用GetMedian()方法获取当前读取数据的中位数。
"""
class Solution:
def __init__(self):
self.left = []
self.right = []
self.count = 0
def Insert(self, num):
if self.count & 1 == 0:
self.left.append(num)
else:
self.right.append(num)
self.count += 1
def GetMedian(self, x):
if self.count == 1:
return self.left[0]
self.MaxHeap(self.left)
self.Minheap(self.right)
if self.left[0] > self.right[0]:
self.left[0], self.right[0] = self.right[0], self.left[0]
self.MaxHeap(self.left)
self.Minheap(self.right)
if self.count & 1 == 0:
return (self.left[0] + self.right[0]) / 2.0
else:
return self.left[0]
"""
用数组实现heap,从根节点开始,从上往下从左到右给每个节点编号,则根据完全二叉树的
性质,给定一个节点i, 其父亲和孩子节点的编号分别是:
parent = (i-1) // 2
left = 2 * i + 1
right = 2 * i + 2
使用数组实现堆一方面效率更高,节省树节点的内存占用,一方面还可以避免复杂的指针操作,减少
调试难度。
"""
def MaxHeap(self, alist):
length = len(alist)
if alist == None or length <= 0:
return
if length == 1:
return alist
for i in range(length // 2 -1, -1, -1):
k = i
temp = alist[k]
heap = False
while not heap and 2 * k < length-1:
index = 2*k+1 # 堆的左子树
if index < length - 1:
if alist[index] < alist[index + 1]:
index += 1 # 堆的右子树
if temp >= alist[index]:
heap = True
else:
alist[k] = alist[index]
k = index
alist[k] = temp
def Minheap(self, alist):
length = len(alist)
if alist == None or length <= 0:
return
if length == 1:
return alist
for i in range(length // 2-1, -1, -1):
k = i
temp = alist[k]
heap = False
while not heap and 2 * k < length - 1:
index = 2 * k + 1
if index < length - 1:
if alist[index] > alist[index + 1]:
index+=1
if temp <= alist[index]:
heap = True
else:
alist[k] = alist[index]
k = index
alist[k] = temp
# 基于数组的方法
class Solution2:
def __init__(self):
self.data = []
def Insert(self, x):
self.data.append(x)
self.data.sort()
def GetMedian(self):
n = len(self.data)
if n & 1 == 0:
return (self.data[n // 2-1] + self.data[n//2]) / 2.0
else:
return self.data[int(n//2)]
剑指offer python版 41.数据流中的中位数
最新推荐文章于 2023-06-16 10:45:01 发布
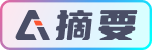