解决中文乱码问题
双击 shift 搜索 matplotlibrc
194行 font.family : sans-serif
206行 font.sans-serif : SimHei, Microsoft YaHei, 系统文件自带
繪圖
from pandas import read_csv
import matplotlib.pyplot as plt
df = read_csv('./gapminder.tsv', sep='\t')
global_year1 = df.groupby('year')['lifeExp'].mean()
global_year2 = df.groupby('year')['gdpPercap'].mean()
fig, (ax1, ax2) = plt.subplots(1, 2, figsize=(8, 4))
ax1.plot(global_year1)
ax2.plot(global_year2)
ax1.set_title('全球按年平均年龄')
ax2.set_title('全球按年平均GDP')
ax1.legend(['平均年龄'])
ax2.legend(['平均GDP'])
plt.show()
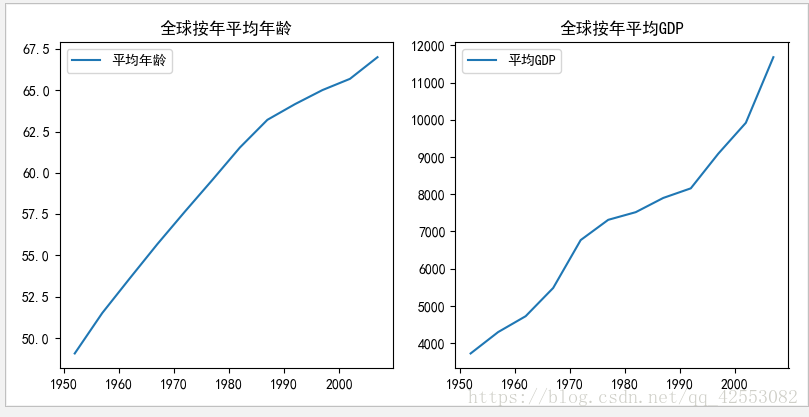
import pandas
import matplotlib.pyplot as plot
df = pandas.read_csv("./gapminder.tsv", sep='\t')
# 全球年平均寿命
global_yearly_life_expectancy = df.groupby("year")["lifeExp"].mean()
print(global_yearly_life_expectancy)
# year
# 1952 49.057620
# 1957 51.507401
# ................
# 2007 67.007423
# Name: lifeExp, dtype: float64
# 使用matplotlib可视化显示--一维表数据
global_yearly_life_expectancy.plot()
# 显示示例 或 plot.legend() 不放列表时,用默认的lifeExp
plot.legend(["平均寿命"])
# plot.legend()
# 显示标题
plot.title("全球年平均寿命")
# 显示
plot.show()
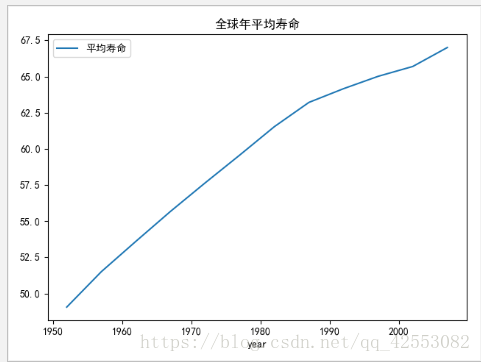
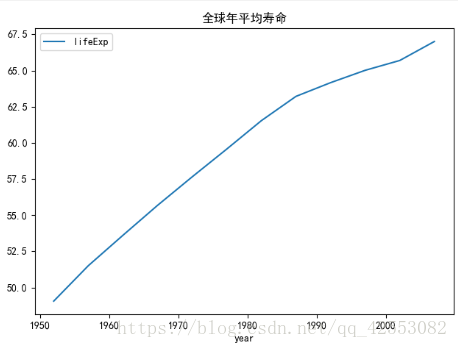
df = pandas.read_csv('./gapminder.tsv', sep='\t')
for item in zip(df.columns, df.dtypes):
print(item)
#output:
# ('country', dtype('O'))
# ('continent', dtype('O'))
# ('year', dtype('int64'))
# ('lifeExp', dtype('float64'))
# ('pop', dtype('int64'))
# ('gdpPercap', dtype('float64'))
res = dict(zip(df.columns, df.dtypes))
print(res)
#output:
# {'country': dtype('O'), 'continent': dtype('O'),
# 'year': dtype('int64'), 'lifeExp': dtype('float64'),
# 'pop': dtype('int64'), 'gdpPercap': dtype('float64')}
country = res.get('country')
print(country)
#output:
# object
平均值,出現次數?
global_year_lifeExp = df.groupby('year')['lifeExp'].mean()
# print(global_year_lifeExp)
#按year, continent分组后的 lifeExp,gdpPercap的平均值, 即每个年份下,每个大洲的 lifeExp,gdpPercap的平均值
num_global_year_lifeExp = df.groupby(['year','continent'])[['lifeExp','gdpPercap']].mean()
# print(num_global_year_lifeExp)
# print(num_global_year_lifeExp.reset_index()) 数据还原到原来格式
# 按continent分组后, 每个country下出现的次数统计
print(df.groupby('continent')['country'].nunique())
一,二维数据
print(df.loc[0:3, ['year', 'gdpPercap']])
print(df.iloc[0:3, [2, 5]])
print(df.iloc[0:6,3:6])
print(df.loc[:,['country','year']])
print(df.iloc[:,[2,6]].head())
print(df.loc[0,['year']])
print(type(df.loc[0,['year']]))
haha = df['country']
print(haha)
print(df.shape)
print(df.tail(3))
print(df.tail(100))
print(df.head())
print(df.head(18))
print(df.loc[0])
# country Afghanistan
# continent Asia
# year 1952
# lifeExp 28.801
# pop 8425333
# gdpPercap 779.445
# Name: 0, dtype: object
print(df.loc[3])
# country Afghanistan
# continent Asia
# year 1967
# lifeExp 34.02
# pop 11537966
# gdpPercap 836.197
# Name: 3, dtype: object
print(df.iloc[-1]) # last = df.shape[0]-1, print(df.loc[last])
# country Zimbabwe
# continent Africa
# year 2007
# lifeExp 43.487
# pop 12311143
# gdpPercap 469.709
# Name: 1703, dtype: object
print(df.iloc[[0,-2,-1]]) ## last = df.shape[0]-1, print(df.iloc[[0,2,last]])
# country continent year lifeExp pop gdpPercap
# 0 Afghanistan Asia 1952 28.801 8425333 779.445314
# 1702 Zimbabwe Africa 2002 39.989 11926563 672.038623
# 1703 Zimbabwe Africa 2007 43.487 12311143 469.709298
#得到year,pop 的....n行
subset = df.loc[0:3,["year","pop"]]
# subset = df.loc[:,["year","pop"]]
#得到2,4和最后一列数据
subset2 = df.iloc[:,[2,4,-1]]
# print(subset2)
# #得到下标是3到6的列
subset3 = df.iloc[:,3:6]
print(subset3.head())
# lifeExp pop gdpPercap
# 0 28.801 8425333 779.445314
# 1 30.332 9240934 820.853030
# 2 31.997 10267083 853.100710
# 3 34.020 11537966 836.197138
# 4 36.088 13079460 739.981106