ai初创企业商业化落地
重点 (Top highlight)
Billion-dollar investments in AI are booming. What does this mean for startups looking to AI for their innovative and competitive edge?
对人工智能的数十亿美元投资正在蓬勃发展 。 对于希望AI发挥创新和竞争优势的初创公司意味着什么?
The strategy seems simple: take one of humanity’s perennial problems and fix it with machine learning. Google, Facebook, Netflix, and Uber did it. It can often seem like the obvious question is why not use AI? At the very least, your new strategy is guaranteed to have the requisite buzzwords a startup seems to need in order to succeed.
该策略看起来很简单:解决人类常年遇到的问题之一,并通过机器学习加以解决。 Google,Facebook,Netflix和Uber做到了。 通常似乎很明显的问题是为什么不使用AI? 至少,您的新策略可以保证获得启动似乎需要的流行语才能成功。
There are countless examples of user experiences that are meaningfully enhanced by AI. But, there are also problems that don’t benefit from AI at all and might even be worse off if AI is applied.
AI带来了无数有意义的用户体验示例。 但是,有些问题根本无法从人工智能中受益,如果应用人工智能,情况甚至可能变得更糟。
We’ll take you through a decision making process that can help you assess if AI is the right approach for your business, including: identifying the need for AI, how to give users control over outcomes, and why AI is not really magic.
我们将指导您完成一项决策过程,该过程可以帮助您评估AI是否适合您的业务,包括:确定对AI的需求,如何使用户对结果进行控制以及AI并不是真正的魔术。
确定需求:您对AI的投资会增加价值吗? (Identify the need: Will your investment in AI add value?)
Entrepreneurs these days often start their journey by asking “How can we use AI to solve X?” While this can be a good starting point, even the best AI system will simply be a drain on resources if it doesn’t provide a unique value to users or customers. Your first priority should be to evaluate where AI could add unique value.
如今,企业家通常会通过问“我们如何使用AI解决X?”来开始自己的旅程。 尽管这可能是一个很好的起点,但是即使最好的AI系统也无法为用户或客户提供独特的价值,也只会浪费资源。 您的首要任务应该是评估AI可以在何处增加独特的价值。
Yes, AI can power a pizza recommendation platform, an age-guessing app and even a fake cat photo generator…but the critical question to ask is if AI is solving a problem in a meaningful or unique way.
是的,人工智能可以为披萨推荐平台,年龄猜测应用程序甚至是伪造的猫照片生成器提供动力……但是,关键要问的是人工智能是否以有意义或独特的方式解决了问题。
AI solutionism, or using AI for the sake of itself, is a problem illustrated in the law famously attributed to both Maslow and Kaplan: if you “give a small boy a hammer…he will find that everything he encounters needs pounding.’’
人工智能解决方案 ,或为自身而使用人工智能,是马斯洛和卡普兰都著名的法律中阐明的一个问题:如果“给小男孩敲击……他会发现他遇到的一切都需要重击”。
So how will you know if AI is right for the problem at hand?
那么您如何知道AI是否适合当前的问题呢?
Has the user need been identified as something requiring an AI solution? Just like the idea of product-market fit, there’s an underlying need to think about AI-user fit.
是否已将用户识别为需要AI解决方案的用户? 就像产品与市场契合的想法一样,也有潜在的需求来考虑AI用户的契合度 。
Conducting user research, reviewing survey data, and observing users’ lives can shift your product philosophy from technology-first to people-first, which means moving from AI “just because you can” to AI “because it uniquely addresses a core user need.”
进行用户研究,查看调查数据并观察用户的生活,可以将您的产品理念从技术至上转变为以人为本,这意味着从AI “仅因为您能”就转向AI, “因为它可以唯一地满足核心用户的需求。 ”
There are no right or wrong motivations to use AI. But it’s one thing to launch an AI-supported customer support chat-bot to help make transactions easier on customers, and it’s another thing to launch that chat-bot just because it’s currently en vogue.
使用AI没有正确或错误的动机。 但是启动AI支持的客户支持聊天机器人以帮助简化客户交易是一回事,而启动该聊天机器人则是另一回事,因为它目前正在流行 。
IDEO’s Design Kit and Google’s People+AI Guidebook can help you identify the user problems where AI can add unique value.
IDEO的设计套件和Google的《 People + AI指南》可帮助您确定AI可以增加独特价值的用户问题。
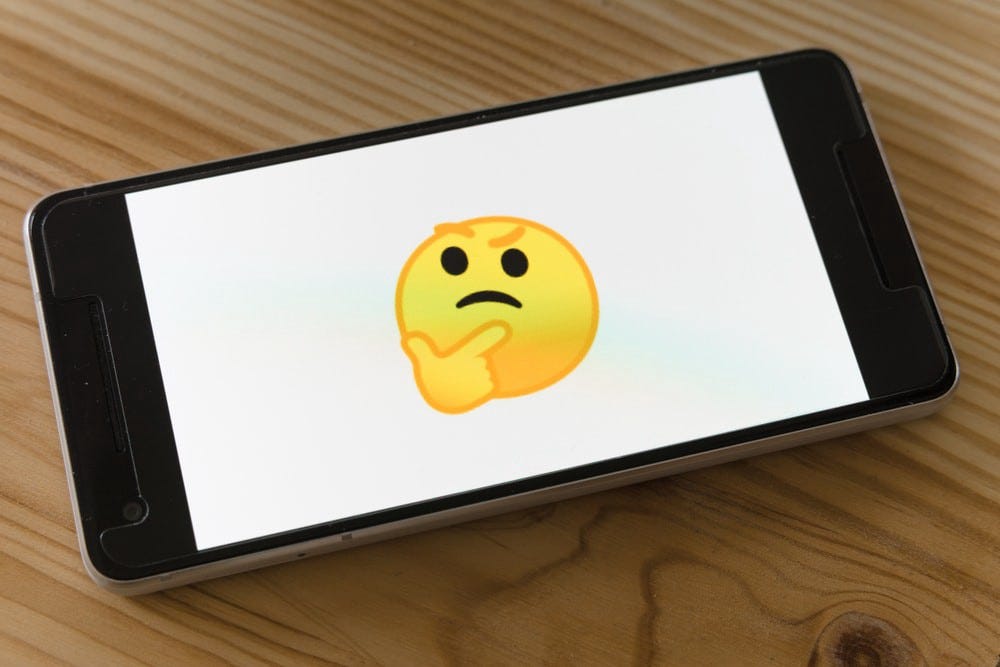
程序规则与启发式 (Programmed rules vs heuristics)
Some user problems are best addressed using heuristics and explicitly programmed rules, rather than complex AI models. For example, think about how social media feeds are organized in apps like Instagram and TikTok.
最好使用启发式和明确编程的规则而不是复杂的AI模型来解决某些用户问题。 例如,考虑一下如何在Instagram和TikTok等应用程序中组织社交媒体供稿。
One way to organize a feed is by using an ML-prediction model of what content a particular user would enjoy most. This takes into consideration the user’s inferred interests, personal information, and past interactions with the app. The ML model would rank all content based on predicted engagement and present a best guess of the most “relevant” content to an individual user.
组织提要的一种方法是使用ML预测模型 ,了解特定用户最喜欢的内容。 这考虑了用户的推断兴趣,个人信息以及与应用程序的过去交互。 ML模型将根据预测的参与度对所有内容进行排名,并向单个用户提供最“相关”内容的最佳猜测。
Alternatively, you could address the problem of ranking your social feed using heuristics without any machine learning at all. Think about feeds that show the most recently posted content first. Some studies show that users may actually prefer chronologically sorted feeds as they might lend more consistency and control to the experience.
另外,您也可以完全不用任何机器学习就可以使用启发式方法对社交Feed进行排名。 考虑提要首先显示最新发布内容的提要。 一些研究表明,用户实际上可能更喜欢按时间顺序排序的提要,因为它们可能给体验带来更多的一致性和控制力。
Another approach would be to allow users to manually upvote or downvote content themselves, not unlike voting systems on popular image boards like Imgur, and aggregators like Reddit and Digg. In this case, however, AI might be counterproductive to maintaining transparency and predictability for users. Not to mention, folk theories and distrust abound if users feel that “objective” user ratings are somehow influenced by an opaque AI layer.
另一种方法是允许用户自己手动投票或降级内容,这与流行图像板上的投票系统(如Imgur)和聚合器(如Reddit和Digg)不同。 但是,在这种情况下,AI可能对维持用户的透明度和可预测性起反作用。 更不用说,民间的理论和不信任比比皆是,如果用户感到“客观”的用户评级受到不透明的AI层的影响。
让用户控制结果 (Give users control over outcomes)
AI products come in two basic varieties: the ones that seek to automate tasks completely and the ones that seek to augment the user’s ability to do the task themselves. Automation is particularly useful when a job is repetitive or computationally complex. When human judgment is crucial for accuracy or responsibility, augmenting a task is most useful. This kind of AI-human partnership can be especially successful when people enjoy doing the job themselves or when personal responsibility around the job is expected.
AI产品有两种基本类型 :一种寻求完全自动化任务的产品, 另一种寻求增强用户自己完成任务的能力的产品 。 当工作重复或计算复杂时,自动化特别有用。 当人工判断对于准确性或责任感至关重要时,增强任务最为有用。 当人们喜欢自己做这项工作或期望对工作承担个人责任时,这种AI人与人之间的合作特别成功。
In both cases, you’ll find that users are reluctant to rely completely on an algorithmic prediction. Research shows that people prefer to trust human experts over AI, even if these experts are more fallible.
在这两种情况下,您都会发现用户不愿完全依赖算法预测。 研究表明,人们更喜欢人类专家而不是人工智能,即使这些专家更容易出错。
Even when a technical explanation of the AI’s decision-making process can be generated, it will often be too difficult for many to understand. Therefore, building trust in your product’s AI models must be cultivated through careful communication.
即使可以生成有关AI决策过程的技术解释 ,对于许多人来说也常常很难理解。 因此, 建造 相信 产品的AI模型必须通过仔细的交流来培养。
For example, if your app uses AI to make restaurant recommendations, consider giving users the option to self-report food preferences and give feedback about visited places. The best way to provide recommendations that exactly match users’ particular tastes and preferences is simply to ask them what they like — even if that means using somewhat biased self-reported preferences. This will be considerably more effective than any ML-model prediction based on (badly) inferred tastes.
例如,如果您的应用程序使用AI提出餐厅推荐,请考虑为用户提供自我报告食物偏好的选项,并提供有关参观地点的反馈。 提供与用户的特定口味和喜好完全匹配的推荐的最佳方法就是问他们喜欢什么,即使这意味着使用偏向的自我报告的喜好。 这将比任何基于(严重)推断的味觉的ML模型预测更加有效。
让使用者坐上驾驶席 (Putting users in the driver’s seat)
If your app uses AI to suggest new movies to watch, consider giving users the option to remove or reset some of the data that’s used to produce recommendations. By contrast, if your ML predictions could have more serious repercussions, consider giving users the option to review ML predictions and potentially course-correct before any serious damage is done.
如果您的应用程序使用AI 建议观看新电影 ,请考虑为用户提供删除或重置用于产生推荐内容的某些数据的选项。 相比之下,如果您的ML预测可能有更严重的影响 ,请考虑让用户选择ML预测,并在任何严重损害发生之前纠正航向。
Build trust by putting users in the driver’s seat, allowing them to understand and manage their interactions with your AI. Not to mention, the habit of co-creation will enrich overall product value.
通过将用户放在驾驶席上来建立信任,使他们了解并管理他们与AI的交互。 更不用说,共同创造的习惯将丰富整体产品价值。
Other approaches to explainability can include articulating data sources, tying explanations to user actions, working closely with a professional UX Writer or Content Strategist, and giving users the tools to control AI outputs.
其他可解释性的方法可以包括阐明数据源 ,将解释与用户操作联系起来,与专业的UX作家或内容策略师密切合作以及为用户提供控制AI输出的工具。
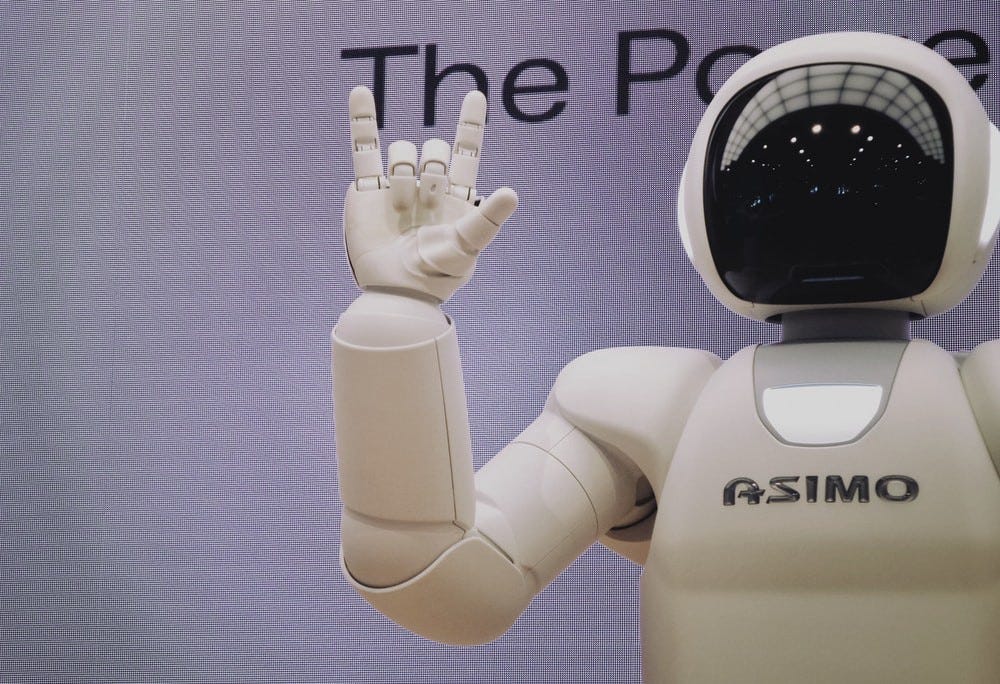
不要答应魔术 (Don’t promise magic)
When a user asks their smart home device a question about the world, the disembodied voice that replies with a chipper response can seem like magic. Voice assistants like Alexa, Siri, Alice and Google Assistant can seem to know more than any human and are always ready to help you. But what’s the best way to present personalized, hyper-helpful intelligence?
当用户向智能家居设备询问有关世界的问题时,用削片机响应进行回复的无形语音听起来像是魔术。 语音助手,例如Alexa , Siri , Alice和Google Assistant似乎比任何人都了解更多,并且随时准备为您提供帮助。 但是,呈现个性化,超级有用的情报的最佳方法是什么?
It can be tempting to market AI as a kind of wizardry; however, there is no such thing as AI magic. Contrary to Arthur C. Clarke’s oft-quoted third law of technology — “Any sufficiently advanced technology is indistinguishable from magic,” communicating notions of magic won’t help users or impress investors.
将AI视为一种巫术可能很诱人。 但是,没有AI魔术这样的东西。 与亚瑟·克拉克 ( Arthur C. Clarke )经常引用的第三技术定律相反: “任何足够先进的技术都无法与魔术区分开”, 传达魔术概念不会帮助用户或打动投资者。
The “magic of AI” is a rhetoric that invites associations with inexplicable or omnipotent power, and tends to create unrealistic expectations about what AI can and cannot do. Such misaligned expectations ultimately lead to disappointment and disengagement.
“ AI的魔力”是一种措辞,它以无法解释的或无所不能的力量来邀请协会,并倾向于对AI可以做什么和不能做什么产生不切实际的期望。 这种错位的期望最终导致失望和脱离。
Anthropomorphizing AI assistants can often exacerbate the problem, directly or indirectly leading users to assume that their virtual assistant has broad human capabilities. Rather than presenting AI as an all-knowing virtual assistant, consider highlighting specific features of the assistant product and how this benefits the user’s goals. This can help users to gradually update their mental models around the evolving AI product capabilities.
拟人化的AI助手通常会直接或间接导致用户认为他们的虚拟助手具有广泛的人类能力,从而加剧该问题。 与其将AI展示为无所不知的虚拟助手,不如着重强调助手产品的特定功能以及这将如何使用户的目标受益。 这可以帮助用户围绕不断发展的AI产品功能逐步更新其思维模型 。
冲帐 (Strike a balance)
There’s a delicate balance to strike between the blanket statement of AI magic and deep technical explanations of the underlying technology. Too much machine learning jargon can get in the way of users when they’re trying to learn to use a product, not explore its mechanisms.
在AI魔术的全面陈述与对底层技术的深入技术解释之间要找到微妙的平衡。 当用户试图学习使用产品而不是探索其机制时,太多的机器学习术语会妨碍用户的使用。
The Google Flights price insights feature is an example of a balanced integration of complex machine learning and user needs. Nowhere in the interface is there mention of “deep learning” or “data crunching.” Instead, the price insights tool simply provides users with a helpful tip about whether flight prices are currently low, typical, or high, and what will likely happen to prices in the near future.
Google Flights价格洞察功能是复杂机器学习和用户需求均衡集成的一个示例。 界面中没有任何地方提到“深度学习”或“数据处理”。 相反, 价格洞察力工具仅向用户提供有关航班价格当前是低价,典型价还是高价以及不久的将来价格可能发生变化的有用提示。
This example also shows how multiple UX design elements can work together to explain an AI prediction and foster trust.
此示例还显示了多个UX设计元素如何可以一起工作以解释AI预测并建立信任。
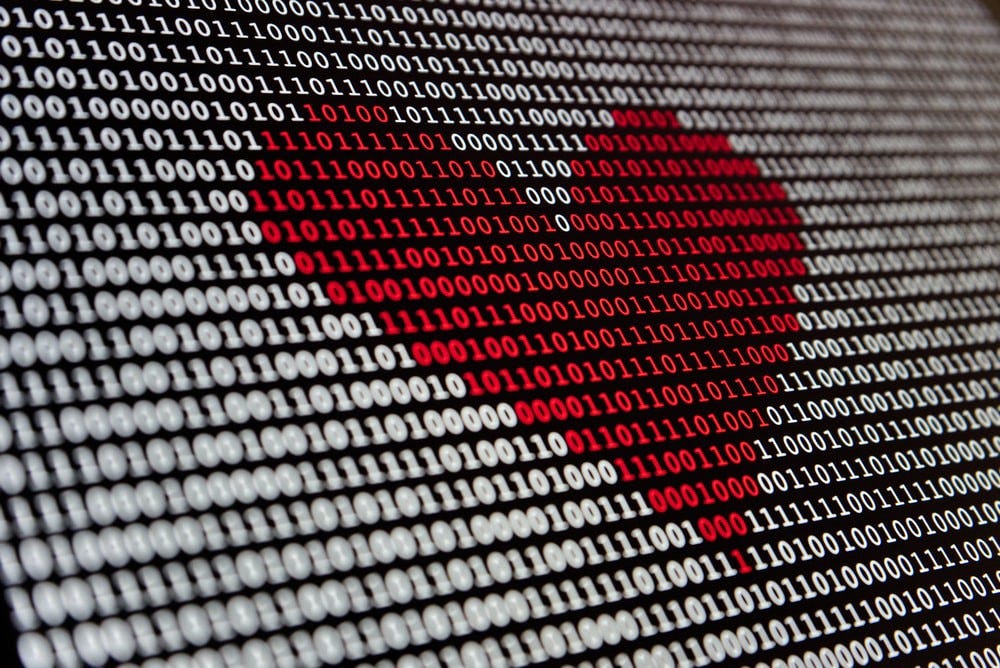
您的产品发布了! 怎么办? (Your product launched! Now what?)
The user experience of AI is different from what came before. AI products can adapt and get better over time. This means that users may need to adjust their mental models of how the product works, and product owners may need to adapt as well.
AI的用户体验与以前不同。 随着时间的流逝,人工智能产品可以适应并变得更好。 这意味着用户可能需要调整其关于产品工作方式的思维模型,并且产品所有者也可能需要适应 。
For example, if you’re using AI to curate and filter your product’s social feeds, at some point in a product life-cycle, you might realize that the AI has learned to prioritize clickbait content and cat videos over important news articles. This means that after launch, you may want to reconsider what you optimize for to ensure diversity in a user’s news feed as well as consistent quality.
例如,如果您使用AI来策划和过滤产品的社交摘要,则在产品生命周期的某个时刻,您可能会意识到AI已经学会了将点击诱饵内容和Cat视频优先于重要新闻。 这意味着启动后,您可能需要重新考虑要优化的内容,以确保用户新闻提要中的多样性以及一致的质量 。
As the ML model learns more about a given user, new features can offer more value, and product owners should adapt to new usage patterns that will follow. Track and measure the success of your product by continuously listening to users (strictly within the bounds of privacy, or course), and running evaluative studies, e.g. using Happiness Tracking Surveys, Jobs-to-be-done trackers or Top Tasks studies.
随着ML模型了解有关给定用户的更多信息,新功能可以提供更多价值,并且产品所有者应适应随之而来的新使用模式。 通过持续聆听用户(严格在隐私或课程范围内)并进行评估研究,例如使用幸福追踪调查 , 即将完成的 任务 追踪器或热门任务研究 ,来跟踪和衡量产品的成功。
寻找更多? (Looking for more?)
Behind these suggestions is a commitment to human-centered AI. Google’s People+AI Guidebook is an open, free resource for further examples and advice on how to design human-centered AI products.
这些建议的背后是对以人为本的AI的承诺。 Google的People + AI指南是一个开放的免费资源,可提供有关如何设计以人为中心的AI产品的更多示例和建议。
The guidebook offers new perspectives on the risks and opportunities around the UX of AI. Topics include user trust and mental models, each with worksheets and resources to help you build human-centered AI products.
该指南提供了有关AI UX周围的风险和机遇的新观点。 主题包括用户信任和心理模型,每个模型都包含工作表和资源,以帮助您构建以人为中心的AI产品。
Now it’s your turn–your AI-powered startup is waiting.
现在轮到您了-您的AI创业公司正在等待。
Author: Slava Polonski, UX Researcher at GoogleEditor: Alexandra Hays, UX Writer at Google
作者: Google UX研究员Slava Polonski 编辑: Google UX作家Alexandra Hays
ai初创企业商业化落地