ai人工智能对话了
对话式AI就是组装积木 (Conversational AI is about assembling building blocks)
Conversational AI is about assembling building blocks and for me, it was my first exposure to Artificial Intelligence. After a couple of years of interacting with it, I quickly fell in love with using it. What I liked most about conversational AI was how accessible it is to get started and do something with it.
对话式AI就是组装积木,对我来说,这是我第一次接触人工智能。 经过几年的互动,我很快就爱上了它。 我最喜欢会话式AI的地方是入门和使用它的便捷性。
Building conversational products today is more about putting pre-built or pre-trained pieces together than becoming a machine learning expert. When I have to describe it to anyone, it looks like this:
如今,构建对话产品更多的是将预先构建或经过培训的片段放在一起,而不是成为机器学习专家。 当我不得不向任何人描述它时,它看起来像这样:

In the example above, if you build an Alexa skill or an action on Google, you are not in charge of implementing the speech-to-text, the Google Nest Hub or the smart speaker handle it, or the actual natural language understanding (i.e., the algorithm that will take a text and match it to a predefined understanding). You can use building blocks that are already made available by Amazon, Google, Microsoft, or IBM.
在上面的示例中,如果您在Google上构建了Alexa技能或动作,则不负责实施语音转文本,Google Nest Hub或智能扬声器来处理语音转文本,也不负责实际的自然语言理解(即,该算法将采用文本并将其匹配到预定义的理解)。 您可以使用Amazon,Google,Microsoft或IBM已经提供的构建基块。
人工智能反映了其创造者及其偏见 (AI is a reflection of its creators and their biases)
In light of recent events that occurred in the United States, highlighting the problems of systemic racism, and the protests that followed around the world, we have been looking at ways to address the struggles faced by the black community.
鉴于美国最近发生的事件突出了系统种族主义的问题,以及世界各地随之而来的抗议活动,我们一直在寻找解决黑人社区所面临的斗争的方法。
In these conversations, technology has not been scrutinized as much, mostly because these systems, rooted in mathematics, are perceived to be based on logic, not human interaction. But when we reflect deeper on it, our existing technology has a single flaw that no proof or algorithm can easily solve: It is built by humans. Therefore, human failings can be built into the machine, however unaware we are of them.
在这些对话中,对技术的关注程度不高,主要是因为这些植根于数学的系统被认为基于逻辑,而不是人类交互。 但是,当我们对它进行更深入的思考时,我们现有的技术只有一个缺陷,而没有任何证据或算法可以轻松解决: 它是人为构建的 。 因此,可以将人为的缺陷内置到机器中,但是我们并不知道它们是什么。
I decided to reflect on conversational AI. As seen in the diagram above, when we build our conversational AI, we rely on that block from Google or Amazon to complete the speech to text functionality. If we look at this recent Stanford study, a lot of people may never hear from your conversational agent because when the device does not do a proper speech to text, it never goes into the next block and therefore African Americans will face difficulties accessing your services.
我决定反思一下对话式AI。 如上图所示,当我们构建对话式AI时,我们依靠Google或Amazon的阻止功能来完成语音转文本功能。 如果我们看一下斯坦福大学最近的这项研究 ,很多人可能永远都不会听到您的对话代理人的消息,因为如果该设备不能正确地发短信讲话,它就不会进入下一个障碍,因此非裔美国人将很难获得您的服务。
As I started digging deeper, I realized that the systems we rely on in these products, convenient APIs (for sentiment analysis for example) or pre-trained language models were prone to something called bias.
当我开始深入研究时,我意识到我们在这些产品中所依赖的系统,便捷的API(例如,用于情感分析)或经过预先训练的语言模型都容易产生偏差。
Bias has as many definitions as the context in which you use it, I picked these two:
偏差的定义与您使用它的上下文一样多,我选择了以下两个:
The way our past experiences distort our perception of and reaction to information, especially in the context of mistreating other humans.
我们过去的经历扭曲了我们对信息的理解和React,特别是在虐待他人的情况下。
Algorithm bias occurs when a computer system reflects the implicit values of the humans who created it.
当计算机系统反映创建它的人的隐式值时,就会发生算法偏差。
I started looking at my diagram through the lens of potential bias and it now looks like this
我开始从潜在偏见的角度看图,现在看起来像这样

If the top uses for conversational AI are entertainment and automation, what happens when the enterprise adoption turns a loan assessment into a conversational agent? If we continue to drill down, what happens if it uses a risk assessment API or model with a bias to conduct the evaluation?
如果对话式AI的主要用途是娱乐和自动化,当企业采用将贷款评估转变为对话式代理时会发生什么? 如果我们继续深入研究,如果它使用带有偏见的风险评估API或模型来进行评估会怎样?
Do the same questions apply to a conversational agent for news feed? What happens when my smart speaker or phone curates Twitter trends but filter the content as it’s considered hate or inappropriate language?
相同的问题是否适用于新闻代理的对话代理? 当我的智能扬声器或电话策划Twitter趋势但过滤掉被视为仇恨或不当语言的内容时,会发生什么?
大型科技公司为什么不修复它? (Why aren’t the big tech companies fixing it?)
As I kept discovering more and more studies and articles denouncing bias and calling out for changes and improvements, my first thought was “what are Amazon, Google, IBM, or Microsoft doing?”. And the answer is they are trying (Google, IBM, Microsoft), but they have three challenges to overcome:
当我不断发现越来越多的研究和文章谴责偏见并呼吁进行更改和改进时,我的第一个念头是“亚马逊,谷歌,IBM或微软在做什么?”。 答案是他们正在尝试( Google , IBM , Microsoft ),但是他们要克服三个挑战:
Despite their best efforts, with a limited diverse workforce, these organizations will have difficulty correcting an issue that may have been caused indirectly by a lack of representation in the first place.
尽管他们尽了最大的努力,但是在多元化的员工队伍中 ,这些组织仍然很难纠正最初由于缺乏代表性而间接造成的问题。
The challenges to solve are complex: In our example earlier of the issues with the understanding of African American voices by speech to text engines, one tentative solution would be to retrain the language models with thousands of African American voice samples. It is not impossible, but it takes time, and unfortunately, sometimes the data is not available.
解决的挑战非常复杂:在我们前面的示例中,通过对文本引擎的语音理解非裔美国人语音的问题,一种尝试性的解决方案是使用数千种非裔美国人语音样本重新训练语言模型。 这不是不可能的 ,但是需要时间,但是不幸的是,有时数据不可用。
No one solution can fix all the issues: what works for mitigating the bias in facial recognition may not apply to the struggles in conversational AI.
没有一种解决方案可以解决所有问题:缓解面部识别偏差的有效方法可能不适用于对话式AI。
从业人员减轻偏见的最佳策略 (Top strategies for practitioners to mitigate bias)
I started thinking about how the product builders can help. The first thing I noticed was that even though we are using pre-built building blocks, these systems still allow us to do additional training specific to the product that we are working on.
我开始考虑产品制造商如何提供帮助。 我注意到的第一件事是,即使我们使用的是预先构建的构建基块,这些系统仍然允许我们针对正在研究的产品进行额外的培训。
Google or Alexa, for example, allows you to define language models on their platforms. One of the things you provide is called sample utterances or training phrases. This helps the system better understand when people are speaking to it.
例如,Google或Alexa允许您在其平台上定义语言模型。 您提供的一件事称为样本话语或训练短语 。 这有助于系统更好地理解人们何时与之交谈。
Coming back to our earlier examples of poor speech to text, if we take an example from https://fairspeech.stanford.edu/, I found two solutions to mitigate the issues of speech to text and natural language understanding.
回到前面的较差的语音到文本的示例,如果我们以https://fairspeech.stanford.edu/为例,我发现了两种解决方案来缓解语音对文本和自然语言理解的问题。
减轻语音对文本的偏见 (Mitigate the speech-to-text bias)
When doing high fidelity prototype user testing, using, for example, the Amazon Echo Show, with the team, we would review the Voice history (i.e. the transcript and the recording of what your Alexa enabled device heard).
在进行高保真度原型用户测试时,例如与团队一起使用Amazon Echo Show,我们将查看语音历史记录(即成绩单和Alexa支持设备听到的录音)。
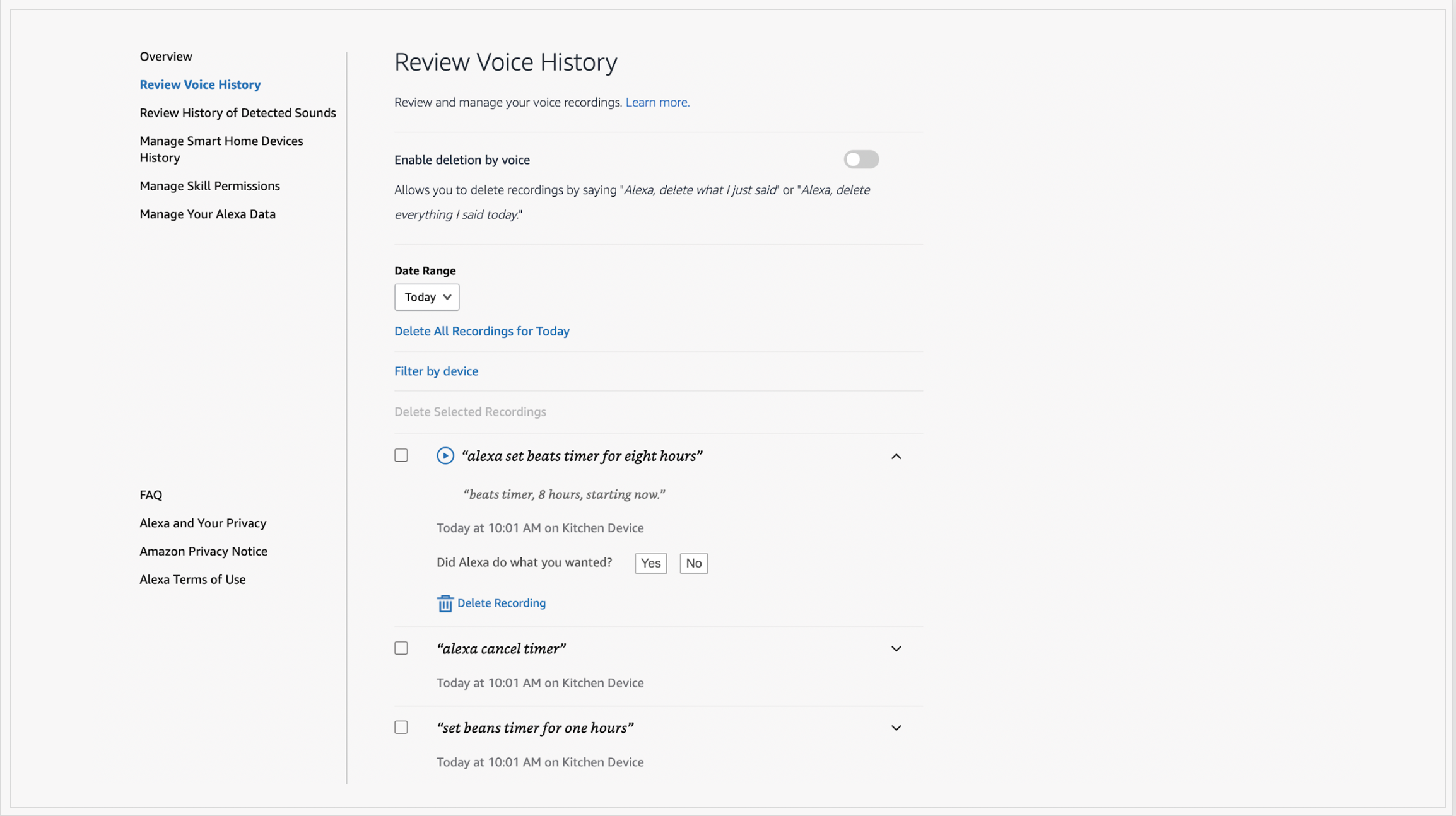
This would enable us, during the testing, to find out whether Amazon (or any other voice-enabled devices) speech to text was having issues with some accents.
这将使我们能够在测试期间找出Amazon(或任何其他启用语音的设备)对文字的语音是否在某些重音上存在问题。
In the example above I want to say “set beans timer for eight hours”. For an application, I could add all the wrong transcripts (for example “set beats timer for eight hours”) to the sample utterances. Even though that’s not solving the root of the problem, it helps mitigate the issue for the users who are victims of the biased text to speech, because Amazon will not just end the conversation or provide the wrong response.
在上面的示例中,我想说“ 将bean计时器设置为八个小时 ”。 对于一个应用程序,我可以将所有错误的成绩单(例如,“ 将节拍计时器设置为八个小时 ”)添加到示例话语中。 即使这不能解决问题的根源,它也可以帮助偏爱文字到语音的用户减轻问题,因为亚马逊不仅会结束对话或提供错误的回复。
减轻自然语言的理解偏差 (Mitigate the natural language understanding bias)
In the Amazon console, in the build section, there is a very useful tool that is a little hidden but that gives you access to a sample of Natural Language Understanding results.
在Amazon控制台的“ 构建”部分中,有一个非常有用的工具 ,该工具有点隐藏,但可让您访问“自然语言理解”结果示例。

Under the unresolved utterances are shown the utterances that users said but that could not be mapped to an intent. We usually mapped them to the closest intent. As with speech to text before, this will not entirely solve the issue but it provides a good mitigation solution.
在未解决的话语下方显示了用户说过但无法映射到意图的话语。 我们通常将它们映射到最接近的意图。 与以前的文字讲话一样,这不能完全解决问题,但可以提供一个很好的缓解方法。
This is only the beginning of the journey, join us on our July 09th event to find out more strategies for product builders to mitigate the bias in conversational AI products.
这只是旅程的开始, 我们在7月9日的活动中为我们寻找更多的策略,以帮助产品制造商减轻对话型AI产品的偏见。
致谢 (Acknowledgments)
A big THANK YOU and shoutout to Polina Cherkashyna, Aimee Reynolds, and Corin Lindsay for editing, reviewing, and ideating with me on this.
非常感谢您 ,并向Polina Cherkashyna , Aimee Reynolds和Corin Lindsay表示感谢,以帮助我进行编辑,审阅和提出想法。
ai人工智能对话了