长序列检测 深度学习
by Sarah Alnegheimish
莎拉·阿尔内海姆(Sarah Alnegheimish)
In the previous post, we looked at time series data and anomalies. (If you haven’t done so already, you can read the article here.) In part 2, we will discuss time series reconstruction using generative adversarial networks (GAN)¹ and how reconstructing time series can be used for anomaly detection².
在上一篇文章中,我们研究了时间序列数据和异常。 (如果您还没有这样做,可以在这里阅读文章。)在第2部分中 ,我们将讨论使用生成对抗网络(GAN)¹重建时间序列以及如何将重建的时间序列用于异常检测²。
使用生成对抗网络的时间序列异常检测 (Time Series Anomaly Detection using Generative Adversarial Networks)
Before we introduce our approach for anomaly detection (AD), let’s discuss one of today’s most interesting and popular models for deep learning: generative adversarial networks (GAN). The idea behind a GAN is that a generator (G), usually a neural network, attempts to construct a fake image by using random noise and fooling a discriminator (D) — also a neural network. (D)’s job is to identify “fake” examples from “real” ones. They compete with each other to be best at their job. How powerful is this approach? Well, the figure below depicts some fake images generated from a GAN.
在介绍我们的异常检测方法(AD)之前,让我们讨论当今最有趣和最受欢迎的深度学习模型之一:生成对抗网络(GAN)。 GAN的想法是,通常是神经网络的生成器(G)试图通过使用随机噪声并欺骗鉴别器(D)来构造假图像-也是神经网络 。 (D)的工作是从“真实”例子中识别“假”例子。 他们彼此竞争以尽其所能。 这种方法有多强大? 好吧,下图描绘了从GAN生成的一些虚假图像。
In this project, we leverage the same approach for time series. We adopt a GAN structure to learn the patterns of signals from an observed set of data and train the generator “G”. We then use “G” to reconstruct time series data, and calculate the error by finding the discrepancies between the real and reconstructed signal. We then use this error to identify anomalies.
在此项目中,我们将相同的方法用于时间序列。 我们采用GAN结构来从观察到的数据集中学习信号模式,并训练生成器“ G”。 然后,我们使用“ G”重建时间序列数据,并通过找到真实信号与重建信号之间的差异来计算误差。 然后,我们使用此错误来识别异常。
Enough talking — let’s look at some data.
聊够了-让我们看一些数据。
讲解 (Tutorial)
In this tutorial, we will use a python library called Orion to perform anomaly detection. After following the instructions for installation available on github, we can get started and run the notebook. Alternatively, you can launch binder to directly access the notebook.
在本教程中,我们将使用一个称为Orion的python库来执行异常检测。 按照github上提供的安装说明进行操作后,我们可以开始并运行笔记本 。 或者,您可以启动活页夹以直接访问笔记本。
载入资料 (Load Data)
In this tutorial, we continue examining the NYC taxi data maintained by Numenta. Their repository, available here, is full of AD approaches and labeled data, organized as a series of timestamps and corresponding values. Each timestamp corresponds to the time of observation in Unix Time Format.
在本教程中,我们将继续检查Numenta维护的NYC出租车数据。 它们的存储库( 此处提供)充满了AD方法和标记的数据,并按一系列时间戳和相应的值进行组织。 每个时间戳对应于Unix时间格式中的观察时间 。
To load the data, simply pass the signal name into the load_signal
function. (If you are loading your own data, pass the file path.)
要加载数据,只需将信号名称传递给load_signal
函数。 (如果要加载自己的数据,请传递文件路径。)
In [1]: from orion.data import load_signal, load_anomalies
In [2]: signal = 'nyc_taxi'
# load signal
In [3]: df = load_signal(signal)
# load ground truth anomalies
In [4]: known_anomalies = load_anomalies(signal)
In [5]: df.head(5)
Out [5]:
+-------------+-----------+
| timestamp | value |
+-------------+-----------+
| 1404165600 | 10844.0 |
| 1404167400 | 8127.0 |
| 1404169200 | 6210.0 |
| 1404171000 | 4656.0 |
| 1404172800 | 3820.0 |
+-------------+-----------+
Though tables are powerful data structures, it’s hard to visualize time series through numerical values alone. So, let’s go ahead and plot the data using plot(df, known_anomalies)
.
尽管表是强大的数据结构,但仅通过数字值就很难可视化时间序列。 因此,让我们继续使用plot(df, known_anomalies)
绘制数据。
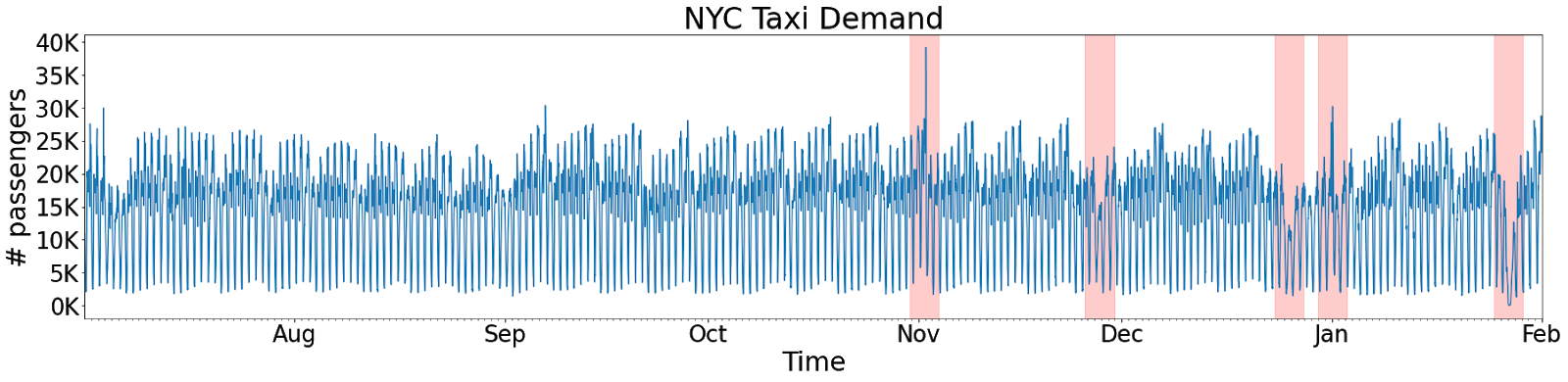
As we saw in the previous post, this data spans almost 7 months between 2014 and 2015. It contains five anomalies: NYC Marathon, Thanksgiving, Christmas, New Year’s Eve, and a major snow storm.
正如我们在上一篇文章中看到的那样,该数据跨越2014年至2015年的近7个月。它包含五个异常:纽约马拉松,感恩节,圣诞节,除夕和一场大雪。
The central question of this post is: Can GANs be used to detect these anomalies? To answer this question, we have developed a time series anomaly detection pipeline using TadGAN, which is readily available in Orion. To use the model, pass the pipeline json
name or path to the Orion API.
这篇文章的中心问题是: 可以使用GAN来检测这些异常吗? 为了回答这个问题,我们开发了使用TadGAN的时间序列异常检测管道,该管道可在Orion中轻松获得。 要使用该模型,请将管道json
名称或路径传递给Orion API。
In [1]: from orion import Orion
In [2]: orion = Orion(
...: pipeline='tadgan.json'
...: )
# fit the pipeline on the data then detect anomalies
In [3]: anomalies = orion.fit_detect(df)
In [4]: anomalies.head(5)
Out [4]:
+-------------+-------------+------------+
| start | end | severity |
+-------------+-------------+------------+
| 1404442800 | 1404734400 | 0.521908 |
| 1408852800 | 1409050800 | 0.168267 |
| 1409378400 | 1409727600 | 0.319860 |
| 1411275600 | 1411488000 | 0.151349 |
| 1414823400 | 1415064600 | 0.158646 |
+-------------+-------------+------------+
The Orion API is a simple interface that allows you to interact with anomaly detection pipelines. To train the model on the data, we simply use the fit
method; to do anomaly detection, we use the detect
method. In our case, we wanted to fit the data and then perform detection; therefore we used the fit_detect
method. This might take some time to run. Once it’s done, we can visualize the results using plot(df, [anomalies, known_anomalies])
.
Orion API是一个简单的接口,可让您与异常检测管道进行交互。 为了在数据上训练模型,我们只需要使用fit
方法即可。 要进行异常检测,我们使用detect
方法。 在我们的例子中,我们想要拟合数据然后执行检测; 因此我们使用了fit_detect
方法。 这可能需要一些时间才能运行。 完成后,我们可以使用plot(df, [anomalies, known_anomalies])
可视化结果。
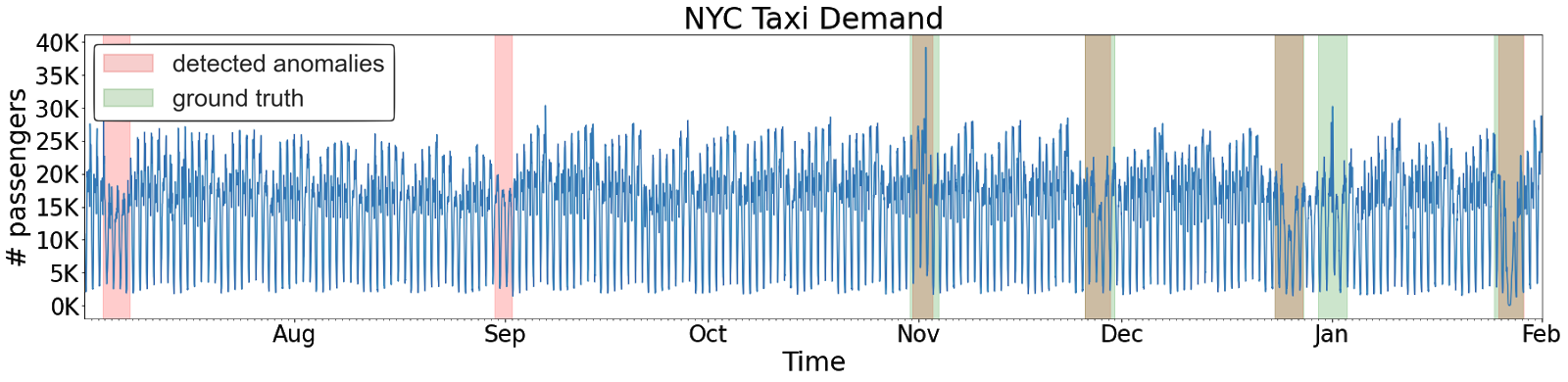
The red intervals depict detected anomalies, with green intervals showing ground truth. The model was able to detect 4 out of 5 anomalies. We also see that it detected some other intervals that were not included in the ground truth labels.
红色的间隔表示检测到的异常,绿色的间隔表示地面情况。 该模型能够检测出5个异常中的4个。 我们还看到它检测到一些其他间隔,这些间隔未包含在地面真相标签中。
Although we jumped straight to the results, let’s backtrack and look at what the pipeline actually did.
尽管我们直接获得了结果,但让我们回头看看管道实际执行了什么。
引擎盖下 (Under the hood)
The pipeline performs a series of transformations on the data, including preprocessing, model training, and post-processing, to obtain the result you have just seen. These functions, which we refer to as primitives, are specified within the model’s json
file. More specifically, if we were to look at the TadGAN model, we find these primitives applied sequentially to the data:
管道对数据执行一系列转换,包括预处理 , 模型训练和后处理 ,以获得您刚刚看到的结果。 这些功能(我们称为原语)在模型的json
文件中指定。 更具体地说,如果我们看一下TadGAN模型,我们发现这些原语顺序地应用于数据:
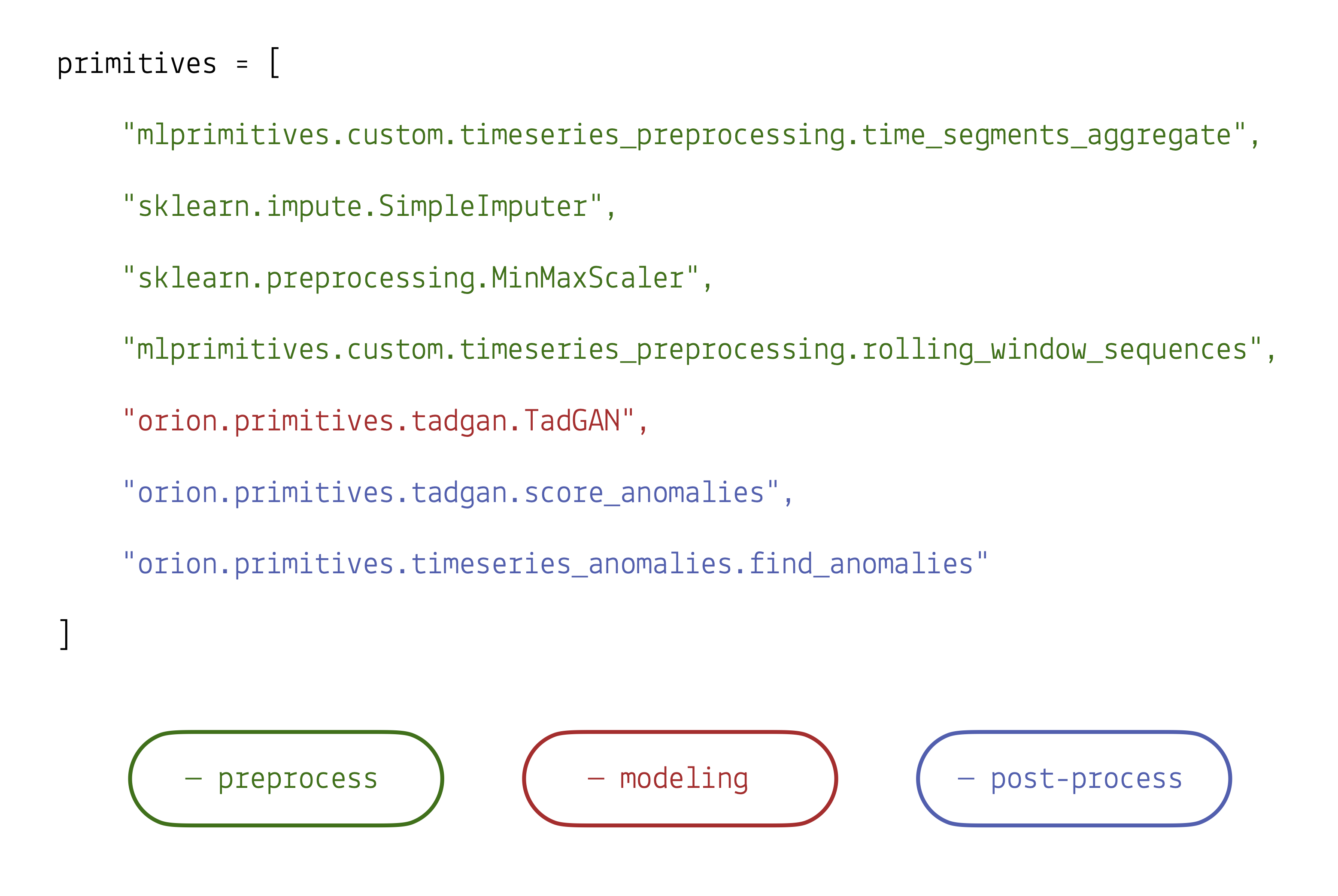
Each primitive is responsible for a single task; each procedure is described in the course of this tutorial.
每个原语负责一项任务; 本教程中将介绍每个过程。
前处理 (Preprocessing)
Before we can use the data, we need to preprocess it. Preprocessing requires us to:
在使用数据之前,我们需要对其进行预处理。 预处理要求我们:
time_segments_aggregate
divides the signal into intervals and applies an aggregation function — producing an equally spaced, aggregated version of the time series.time_segments_aggregate
将信号划分为间隔,并应用聚合函数-生成时间序列的等距聚合版本。SimpleImputer
imputes missing values with a specified value.SimpleImputer
使用指定的值估算缺少的值。MinMaxScaler
scales the values between a specified range.MinMaxScaler
在指定范围之间缩放值。rolling_window_sequences
divides the original time series into signal segments.rolling_window_sequences
将原始时间序列分为多个信号段。
Prepare Data — First, we make the signal of equal steps. Second, we impute missing values using the mean. Third, we scale the data between [-1, 1].
准备数据—首先,我们发出相等步幅的信号。 其次,我们使用均值估算缺失值。 第三,我们在[-1,1]之间缩放数据。
from sklearn.impute import SimpleImputer
from sklearn.preprocessing import MinMaxScaler
from mlprimitives.custom.timeseries_preprocessing import time_segments_aggregate
# equalize steps (decide on a frequency)
X, index = time_segments_aggregate(df,
interval=1800,
time_column='timestamp',
method=['mean'])
# impute missing values
imp = SimpleImputer()
X = imp.fit_transform(X)
# scale the data between [-1, 1]
scaler = MinMaxScaler(feature_range=(-1, 1))
X = scaler.fit_transform(X)
If we go back to the source of the NYC Taxi data, we find that it records a value every 30 minutes. Since timestamps are defined by seconds, we set the interval as 1800
. We also opt for the default aggregation method, which in this case is taking the mean
value of each interval. We also impute the data with the mean value. In this specific example, we can safely remove the time_segments_aggregate
and impute
primitives since the data is already equally spaced and does not contain missing values(of course, not all data is this pristine). Next, we scale the data between [-1, 1] such that it’s properly normalized for modeling.
如果我们回到纽约出租车数据的来源,我们发现它每30分钟记录一次值。 由于时间戳以秒为单位,因此我们将时间间隔设置为1800
。 我们还选择默认的汇总方法,在这种情况下,该方法采用每个时间间隔的mean
。 我们还用平均值来估算数据。 在此特定示例中,我们可以安全地删除time_segments_aggregate
并impute
原始数据,因为数据已经均匀分布并且不包含缺失值(当然,并非所有数据都是原始数据)。 接下来,我们在[-1,1]之间缩放数据,以便对其进行适当规范化以进行建模。
After this, we need to prepare the input for training the TadGAN model. To obtain the training samples, we introduce a sliding window to divide the original time series into signal segments. The following illustration depicts this idea.
之后,我们需要准备用于训练TadGAN模型的输入。 为了获得训练样本,我们引入了一个滑动窗口将原始时间序列划分为信号段。 下图描述了这个想法。
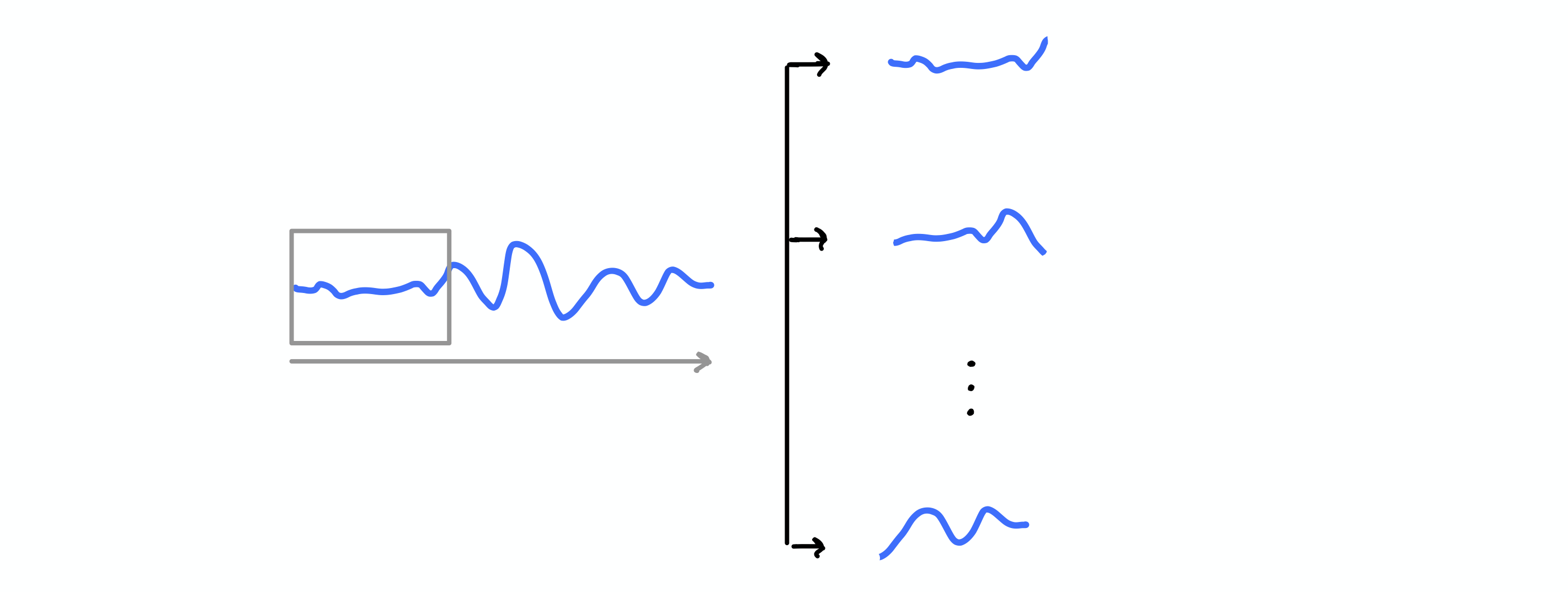
In [1]: from mlprimitives.custom.timeseries_preprocessing
...: import rolling_window_sequences
In [2]: X, X_index, y, y_index = rolling_window_sequences(X, index,
...: window_size=100,
...: target_size=1,
...: step_size=1,
...: target_column=0)
In [3]: print("Training data input shape: {}".format(X.shape))
Out [3]: Training data input shape: (10222, 100, 1)
Here, X
represents the input used to train the model. It is an np.array
of size: number of training examples by window_size
. In our case, we see X
has 10222
training examples. Notice that 100
represents the window_size
. Using plot_rws(X, k=4)
we can visualize X
.
在此, X
表示用于训练模型的输入。 它是一个np.array
的大小: window_size
的训练示例数。 在我们的案例中,我们看到X
有10222
训练示例。 请注意, 100
表示window_size
。 使用plot_rws(X, k=4)
我们可以可视化X

This makes the input ready for our machine learning model.
这使输入准备好用于我们的机器学习模型。
造型 (Modeling)
Orion provides a suite of ML models that can be used for anomaly detection; such as ARIMA, LSTM, GAN, and more.
Orion提供了一套ML模型,可用于异常检测。 例如ARIMA,LSTM,GAN等。
In this tutorial, we will focus on using GAN. In case you are not familiar with GANs, there are many tutorials that help you implement one using different packages, tensorflow, or pytorch.
在本教程中,我们将重点介绍使用GAN。 如果您对GAN不熟悉,有很多教程可以帮助您使用不同的包, tensorflow或pytorch来实现一个。
To select a model of interest, we specify its primitive within the pipeline. To use the GAN model, we will be using the primitive:
要选择感兴趣的模型,我们在管道中指定其原语。 要使用GAN模型,我们将使用原语:
TadGAN
trains a custom time series GAN model.TadGAN
训练自定义时间序列GAN模型。
Training— The core idea of a reconstruction-based anomaly detection method is to learn a model that can generate (construct) a signal with similar patterns to what it has seen previously.
训练 -基于重构的异常检测方法的核心思想是学习一个模型,该模型可以生成(构造)信号,信号模式与以前看到的模式相似。

The general training procedure of GANs is based on the idea that we want to reconstruct the signal as best as possible. To do this, we learn two mapping functions: an encoder (E) that maps the signal to the latent representation, “z”, and a generator (G) that recovers the signal from the latent variable. The discriminator (Dx) measures the realness of the signal. Additionally, we introduce a second discriminator (Dz) to distinguish between random latent samples “z” and encoded samples E(x). The intention behind Dz is to force E to encode features into a representation that is as close to white noise — as possible. This acts as a way to regularize the encoder E and avoid overfitting. The intuition behind using GANs for time series anomaly detection is that an effective model should not be able to reconstruct anomalies as well as “normal” instances.
GAN的一般训练过程是基于这样的想法,即我们希望尽可能地重建信号。 为此,我们学习了两个映射函数:将信号映射到潜在表示“ z”的编码器(E)和从潜在变量中恢复信号的生成器(G)。 鉴别器(D x )测量信号的真实性。 另外,我们引入了第二个鉴别符(D z )来区分随机潜在样本“ z”和编码样本E(x)。 D z背后的意图是迫使E将特征编码成尽可能接近白噪声的表示形式。 这用作使编码器E正规化并避免过度装配的方式。 使用GAN进行时间序列异常检测的直觉是有效的模型不应该能够重建异常以及“正常”实例。
To use the TadGAN
model, we specify a number of parameters including model layers (structure of the previously mentioned neural networks). We also specify the input dimensions, the number of epochs, the learning rate, etc. All the parameters are listed below.
为了使用TadGAN
模型,我们指定了许多参数,包括模型层(前面提到的神经网络的结构)。 我们还指定输入尺寸,时期数,学习率等。所有参数在下面列出。
from model import hyperparameters
from orion.primitives.tadgan import TadGAN
hyperparameters["epochs"] = 100
hyperparameters["shape"] = (100, 1) # based on the window size
hyperparameters["optimizer"] = "keras.optimizers.Adam"
hyperparameters["learning_rate"] = 0.0005
hyperparameters["latent_dim"] = 20
hyperparameters["batch_size"] = 64
tgan = TadGAN(**hyperparameters)
tgan.fit(X)
It might take a bit of time for the model to train.
模型可能需要一些时间来训练。
Reconstruction— After the GAN finishes training, we next attempt to reconstruct the signal. We use the trained encoder (E) and generator (G) to reconstruct the signal.
重建— GAN完成训练后,我们接下来尝试重建信号。 我们使用训练有素的编码器(E)和发生器(G)重建信号。

We pass the segment of the signal (same as the window) to the encoder and transform it into its latent representation, which then gets passed into the generator for reconstruction. We call the output of this process the reconstructed signal. We can summarize it for a segment s as: s → E(s) → G(E(s))≈ ŝ. When s is normal, s and ŝ should be close. On the other hand, if s is abnormal then s and ŝ should deviate.
我们将信号的一部分(与窗口相同)传递给编码器,并将其转换为潜在的表示形式,然后将其传递到生成器中进行重构。 我们将此过程的输出称为重构信号。 我们可以将段s总结为: s→ E (s) → G ( E (s))≈ŝ 。 当s正常时, s和ŝ应该接近。 另一方面,如果s异常,则s和ŝ应偏离。
The process above reconstructs one segment (window). We can get all the reconstructed segments by using the predict
method in our API — X_hat, critic = tgan.predict(X)
. We can use plot_rws(X_hat, k=4)
to view the result.
上面的过程重建了一个片段(窗口)。 我们可以用得到的所有重建的部分predict
-我们的API的方法X_hat, critic = tgan.predict(X)
我们可以使用plot_rws(X_hat, k=4)
查看结果。

Per figure above, we notice that a reconstructed datapoint may appear in multiple windows based on the step_size
and window_size
that we have chosen in the preprocessing step. To get the final value of a datapoint for a particular time point, we aggregate the multiple reconstructed values for that datapoint. This results in a single value for each timestamp, resulting in a fully reconstructed version of the original signal in df
.
根据上图,我们注意到,根据我们在预处理步骤中选择的step_size
和window_size
,重构的数据点可能会出现在多个窗口中。 为了获得特定时间点的数据点的最终值,我们汇总了该数据点的多个重构值。 这将为每个时间戳生成一个单一值,从而在df
中生成原始信号的完全重建版本。
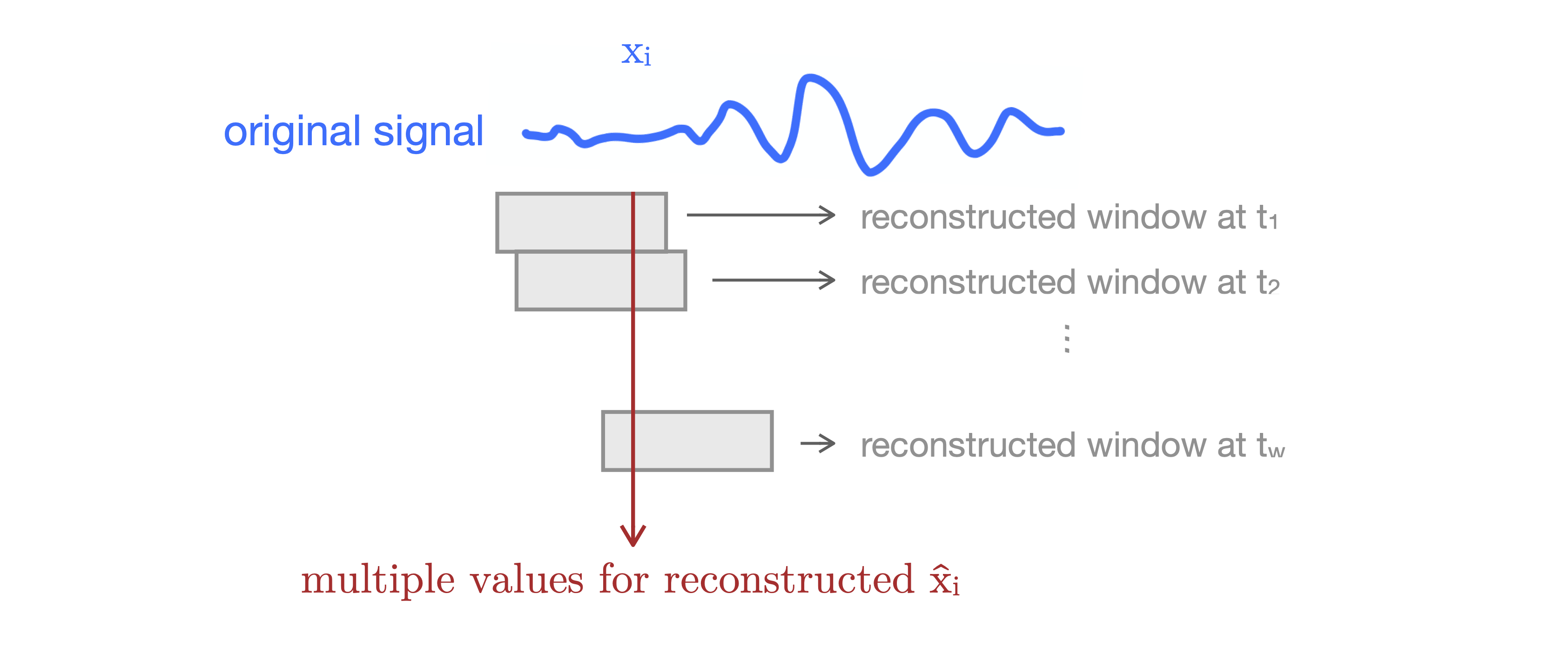
To reassemble or “unroll” the signal, we can choose different aggregation methods. In our implementation, we chose it as the median value.
要重组或“展开”信号,我们可以选择不同的汇总方法。 在我们的实现中,我们选择它作为中间值。
We can then use y_hat = unroll_ts(X_hat)
to flatten the reconstructed samples X_hat
and plot([y, y_hat], labels=['original', 'reconstructed'])
for visualization.
然后,我们可以使用y_hat = unroll_ts(X_hat)
来展平重构的样本X_hat
和plot([y, y_hat], labels=['original', 'reconstructed'])
以进行可视化。
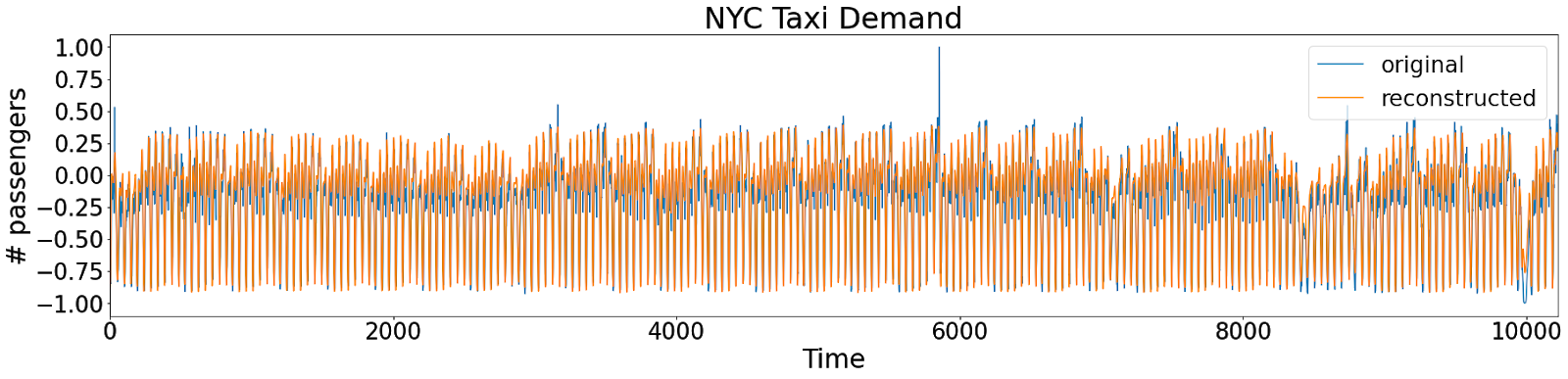
We can see that the GAN model did well in trying to reconstruct the signal. We also see how it expected the signal to be, in comparison to what it actually is.
我们可以看到,GAN模型在尝试重建信号方面做得很好。 与实际信号相比,我们还看到了信号的期望值。
后期处理 (Post-processing)
The next step in the pipeline is to perform post-processing, it includes calculating an error then using it to locate the anomalies. The primitives we will use are:
流水线的下一步是执行后处理,包括计算错误,然后使用它来定位异常。 我们将使用的原语是:
score_anomalies
calculates the error between the real and reconstructed signal, this is specific to the GAN model.score_anomalies
计算真实信号与重构信号之间的误差,这是GAN模型所特有的。find_anomalies
identifies anomalous intervals based on the error obtained.find_anomalies
根据获得的错误标识异常间隔。
Error Scores — We use the discrepancies between the original signal and the reconstructed signal as the reconstruction error score. There are many methods to calculate this error, such as point and area difference.
错误分数—我们将原始信号和重建信号之间的差异用作重建误差分数。 有许多计算此误差的方法,例如点和面积差。
# pair-wise error calculation
error = np.zeros(shape=y.shape)
length = y.shape[0]
for i in range(length):
error[i] = abs(y_hat[i] - y[i])
# visualize the error curve
fig = plt.figure(figsize=(30, 3))
plt.plot(error)
plt.show()

Analyzing the data, we noticed a large deviation between the two signals, present in some regions more than others. For a more robust measure, we use dynamic time warping (DTW) to account for signal delays and noise. This is the default approach for error calculation in the score_anomaly
method but can be overriden using the rec_error_type
parameter.
分析数据后,我们注意到两个信号之间存在较大偏差,在某些区域中存在的偏差要大于其他区域。 为了采取更可靠的措施,我们使用动态时间规整(DTW)来考虑信号延迟和噪声。 这是score_anomaly
方法中错误计算的默认方法,但可以使用rec_error_type
参数覆盖。
During the training process, the discriminator has to distinguish between real input sequences and constructed ones; thus, we refer to it as the critic score. To think of it, this score is also of relevance to distinguish anomalous sequences from normal ones, since we assume that anomalies will not be reconstructed. score_anomaly
leverages this critic score by first smoothing the score through kernel density estimation (KDE) on the collection of critics and then taking the maximum value as the smoothed value. The end error score combines the reconstruction error and the critic score.
在训练过程中,鉴别器必须区分真实的输入序列和构造的输入序列。 因此,我们将其称为评论者分数。 考虑到这一点,该分数也与区分异常序列与正常序列有关,因为我们假设将不会重建异常。 score_anomaly
通过首先在评论者集合上通过核密度估计(KDE)对分数进行平滑,然后将最大值作为平滑值score_anomaly
利用此批评者分数。 最终错误分数结合了重构错误和评论者分数。
from orion.primitives.tadgan import score_anomalies
error, true_index, true, pred = score_anomalies(X, X_hat,
critic,
X_index,
rec_error_type="dtw",
comb="mult")
pred = np.array(pred).mean(axis=2)
# visualize the error curve
plot_error([[true, pred], error])

Now we can visually see where the error reaches a substantially high value. But how should we decide if the error value determines a potential anomaly? We could use a fixed threshold that says if error > 10
, then the datapoint should be classified as anomalous.
现在,我们可以直观地看到错误在何处达到很高的值。 但是,我们应该如何确定误差值是否确定潜在的异常呢? 我们可以使用一个固定的阈值,即如果error > 10
,则该数据点应归类为异常。
thresh = 10
intervals = list()
i = 0
max_start = len(error)
while i < max_start:
j = i
start = index[i]
while error[i] > thresh:
i += 1
end = index[i]
if start != end:
intervals.append((start, end, np.mean(error[j: i+1])))
i += 1
anomalies = pd.DataFrame(intervals, columns=['start', 'end', 'score'])
plot(df, [anomalies, known_anomalies])
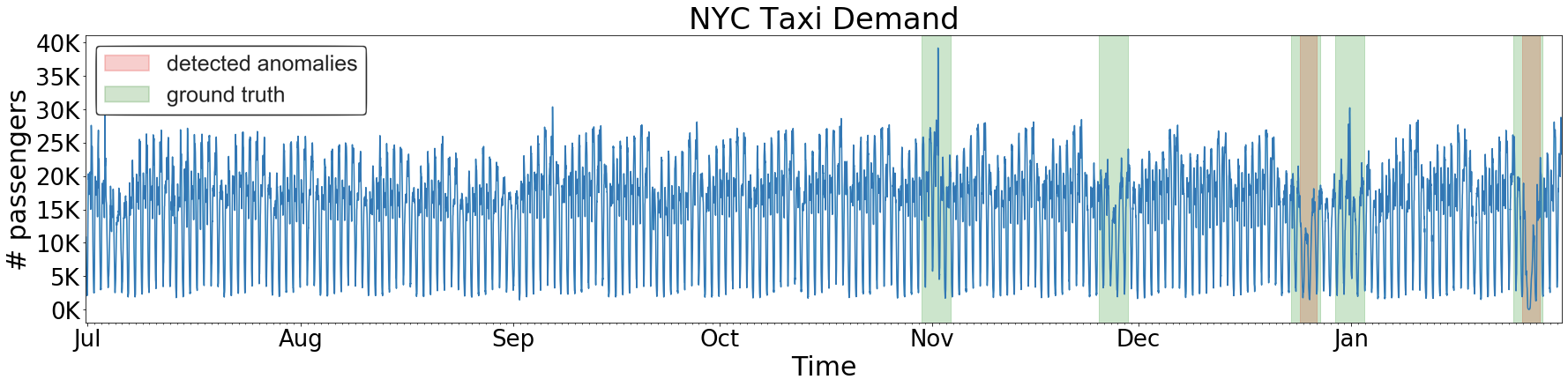
While a fixed threshold raised two correct anomalies, it missed out on the other three. If we were to look back at the error plot, we notice that some deviations are abnormal within its local region. So, how can we incorporate this information in our thresholding technique? We can use window-based methods to detect anomalies in context.
虽然固定的阈值提出了两个正确的异常,但它错过了其他三个。 如果我们回顾一下误差图,我们会注意到在其局部区域内有些偏差是异常的。 那么,如何将这些信息纳入我们的阈值技术中? 我们可以使用基于窗口的方法来检测上下文中的异常。
We first define the window of errors that we want to analyze. We then find the anomalous sequences in that window by looking at the mean and standard deviation of the errors. For errors that fall far from the mean (such as four standard deviations away), we classify its index as anomalous. We store the start/stop index pairs that correspond to each anomalous sequence, along with its score. We then move the window and repeat the procedure.
我们首先定义要分析的错误窗口。 然后,我们通过查看误差的均值和标准偏差,在该窗口中找到异常序列。 对于与平均值相差甚远的误差(例如相距四个标准偏差),我们将其索引归类为异常。 我们存储与每个异常序列相对应的开始/停止索引对及其分数。 然后,我们移动窗口并重复该过程。
from orion.primitives.timeseries_anomalies import find_anomalies
# find anomalies
intervals = find_anomalies(error, index,
window_size_portion=0.33,
window_step_size_portion=0.1,
fixed_threshold=True)
anomalies = pd.DataFrame(intervals, columns=['start', 'end', 'score'])
plot(df, [anomalies, known_anomalies])
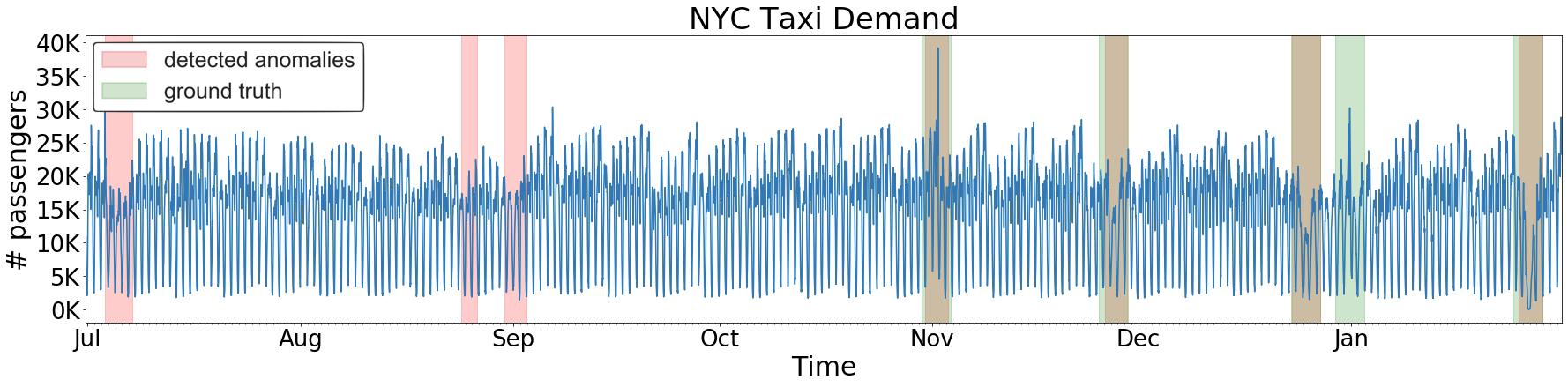
We now have similar results as we saw previously. The red intervals depict the detected anomalies, the green intervals show the ground truth. 4 out of 5 anomalies were detected. We also see that it detected some other intervals that were not included in the ground truth labels.
现在,我们得到的结果与之前看到的类似。 红色的间隔表示检测到的异常,绿色的间隔表示地面情况。 5个异常中有4个被检测到。 我们还看到它检测到一些其他间隔,这些间隔未包含在地面真相标签中。
Orion API (Orion API)
Using the Orion API and pipelines, we simplified this process yet allowed flexibility for pipeline configuration.
使用Orion API和管道,我们简化了此过程,但允许灵活配置管道。
How to configure a pipeline?
如何配置管道?
Once primitives are stitched together, we can identify anomalous intervals in a seamless manner. This serial process is easy to configure in Orion.
将图元缝合在一起后,我们可以无缝地识别异常间隔。 这个串行过程很容易在Orion中配置。
To configure a pipeline, we adjust the parameters of the primitive of interest within the pipeline.json
file or directly by passing the dictionary to the API.
要配置管道,我们可以在pipeline.json
文件中调整感兴趣的原语的参数,或者直接将字典传递给API。
In the following example, I changed the aggregation level as well as the number of epochs
for training. These changes will override the parameters specified in the json
file. To know more about the API usage and primitive designs, please refer to the documentation. How we set the model and change the values of the hyperparameters is explained in the mlprimitives
library. You can refer to its documentation here.
在以下示例中,我更改了聚合级别以及用于训练的epochs
数。 这些更改将覆盖json
文件中指定的参数。 要了解有关API用法和原始设计的更多信息,请参阅文档 。 mlprimitives
库中说明了如何设置模型和更改超参数的值。 您可以在此处参考其文档。
from orion import Orion
parameters = {
'mlprimitives.custom.timeseries_preprocessing.time_segments_aggregate#1': {
"interval": 3600 # hour level
},
'orion.primitives.tadgan.TadGAN#1': {
“epochs”: 25,
}
}
orion = Orion(
'tadgan.json',
parameters
)
anomalies = orion.fit_detect(df)
Now anomalies
holds the detected anomalies.
现在, anomalies
保留检测到的异常。
In this tutorial, we looked at using time series reconstruction to detect anomalies. In the next post, we will explore more about evaluating pipelines and how we measure the performance of a pipeline against the ground truth. We will also look at comparing multiple anomaly detection pipelines from an end-to-end perspective.
在本教程中,我们研究了使用时间序列重建来检测异常。 在下一篇文章中,我们将探索有关评估管道的更多信息,以及如何根据实际情况评估管道的性能。 我们还将从端到端的角度比较多个异常检测管道。
- In addition to the vanilla GAN, we also introduce other neural networks including: an encoding network to reduce the feature space, as well as a secondary discriminator. 除香草GAN之外,我们还引入了其他神经网络,包括:减少特征空间的编码网络以及辅助鉴别器。
This tutorial walks through the different steps taken to perform anomaly detection using the TadGAN model. The particulars of TadGAN and how it was architected will be detailed in another post.
本教程分步介绍了使用TadGAN模型执行异常检测所采取的不同步骤。 TadGAN的详细信息及其构建方式将在另一篇文章中详细介绍。
长序列检测 深度学习