机器学习 生成
AI Composers present ideas to their human partners. People can then take certain elements and work them into their own, uniquely human, compositions. These AI-assisted compositions are then used to further train AI Composers, creating a cycle that renews itself symbiotically. Progress is inexorable.
AI作曲家向其人类合作伙伴展示想法。 然后,人们可以采用某些要素并将其运用到自己独特的人类作品中。 然后将这些AI辅助的合成物用于进一步训练AI作曲者,从而创建一个可以共生更新的循环。 进步是不可阻挡的。
Motivation I wanted to sample tracks of my choosing and layer them using a machine learning framework. I sought to use machine learning to ‘mashup’ songs. Moreover, I wanted to output sheet music that musicians could fool around with.
中号otivation我想我选择的样本轨道,并使用机器学习框架层他们。 我试图使用机器学习来“混搭”歌曲。 此外,我想输出乐手可以混搭的活页乐谱。
If you’re looking for instant gratification: proceed directly to Go, don’t collect $200, and check out the corresponding Python Notebook, where you can play around and bring your very own AI Composer to life.
如果您正在寻找即时的满足感:请直接转到Go,不要花200美元,而是查看相应的Python笔记本,在那里您可以玩耍并使自己的AI Composer栩栩如生。
However, if you’re intrigued by the notion of an AI composer and are curious as to the project’s inspiration and intent, indulge yourself by reading forth!
但是,如果您对AI作曲家的概念感兴趣,并且对项目的灵感和意图感到好奇,请阅读本书,尽情享受!
By the end of this article, you will be able to channel your inner (AI) composer.
到本文结尾,您将能够引导自己的内部(AI)作曲家。
AI作曲家? (The AI Composer?)
Some humans are living, breathing groove machines, musicians. I am by no means of this sort, however I am a devout supporter of live music. There is profound pleasure in observing groove machines in their element, becoming entranced by their beats, and humbled by their harmonies. Music is a transcendent art. Not only does it move us, it moves with us.
一些人正在生活,呼吸开槽机,音乐家。 我绝不是这种人,但是我是现场音乐的虔诚支持者。 观察开槽机的元素,被其拍子迷住并因其和谐而谦卑,这是一种极大的乐趣。 音乐是一种超然的艺术。 它不仅使我们感动,也与我们一起感动。
Music has evolved alongside humanity, it is inexorably linked to our world view and the technology we use to generate it. Advances in technology have dramatically restructured our musical landscape. Electronic gadgets have afforded musicians an ability to shape their sound with mind-boggling variety.
音乐随着人类的发展而发展,它与我们的世界观以及我们用来创造音乐的技术紧密相关。 科技的进步极大地改变了我们的音乐格局。 电子产品使音乐家能够以令人难以置信的多样性来塑造自己的声音。
Musicians are sculptors of sound. Some do it spontaneously, like a jazz guitarist plucking an improvised solo with intoxicating brio. Others do it more methodically, such as a symphony orchestra, the sublime synchronicity with which the performers become united. Expressed as formalized sheet music or intricately engrained in mental structure, all music is in a sense, composed.
音乐家是声音的雕刻家。 有些人是自然而然地做到这一点的,例如爵士吉他手用醉人的布里欧弹奏一个即兴的独奏。 其他人则更系统地做到这一点,例如交响乐团,表演者团结一致的崇高同步性。 表现为形式化的乐谱或根深蒂固的心理结构,所有音乐在某种意义上都是组成的。
The electronic analogue of the performer has been around since the advent of the electromagnetic speaker. The electronic analogue of the composer, however, is less defined. Such a notion has only recently been given credence now that AI techniques have achieved nominal success: We are thus entering the age of the AI composer.
自从电磁扬声器问世以来,表演者的电子模拟就已经存在。 但是,作曲家的电子模拟定义较少。 既然AI技术已经取得了巨大的成功,这种想法直到最近才得到信任:我们正步入AI作曲家的时代。
In the traditional sense a composer stitches musical ideas together into song. Drawing upon their prior auditory and emotional experience, talented composers seize our senses with their moving passages. Can a machine be trained to compose songs, too? And if so, why would we want it to write music, anyhow??
在传统意义上,作曲家将音乐思想拼合成歌曲。 有才华的作曲家利用他们先前的听觉和情感经验,通过其感人的段落抓住我们的感官。 机器也可以训练作曲吗? 如果是这样,为什么我们要它写音乐呢?
Machines can enrich our auditory experience. AI in particular can be leveraged to detect musical nuances that have hitherto existed beyond the bounds of human creativity. What is more, AI can be employed such that these machine-detected musical idioms are uniquely combined. This is the essence of the AI Composer: Machine learning tools are, at present, capable of detecting musical idioms and stitching them together in neat ways.
机器可以丰富我们的听觉体验。 尤其是可以利用AI来检测迄今为止人类创造力范围之外存在的音乐细微差别。 而且,可以采用AI,以便将这些机器检测的音乐习语独特地组合在一起。 这是AI Composer的本质:机器学习工具目前能够检测音乐成语并将其以整齐的方式拼接在一起。
Accordingly, I envision a symbiotic relationship between AI Composers and their human counterparts. AI Composers present ideas to their human partners. People can then take certain elements and work them into their own, uniquely human, compositions. These AI-assisted compositions are then used to further train AI Composers, creating a cycle that renews itself symbiotically.
因此,我设想了AI作曲家与人类同行之间的共生关系。 AI作曲家向其人类合作伙伴展示想法。 然后,人们可以采用某些元素并将其运用到自己独特的人类作品中。 然后将这些AI辅助的合成物用于进一步训练AI作曲者,从而创建一个可以共生更新的循环。
实验:制作AI作曲家 (The Experiment: Making an AI Composer)
Forays into the intersection spanned by AI and music are numerous, and have, in a sense, reached mainstream status. Google’s Magenta project being an example of this.
在AI和音乐跨越的交汇点上的尝试很多,并且在某种意义上已经达到了主流地位。 Google的Magenta项目就是一个例子。
Magenta is a fun little library that uses TensorFlow as its machine learning workhorse. Examples using Magenta to toy around with music (and art) exist. However, I sought something more. I wanted to sample tracks of my choosing and layer them using a machine learning framework. I sought to use machine learning to ‘mashup’ songs. Moreover, I wanted to output sheet music that musicians could fool around with.
Magenta是一个有趣的小图书馆,它使用TensorFlow作为机器学习的主力。 存在使用洋红色在音乐(和艺术)周围嬉戏的示例。 但是,我寻求更多。 我想对自己选择的轨迹进行采样,并使用机器学习框架对其进行分层。 我试图使用机器学习来“混搭”歌曲。 此外,我想输出乐手可以混搭的活页乐谱。
洋红色的音乐和机器学习。 (Music and Machine Learning with Magenta.)
Magenta can be used to manipulate music in a multitude of ways. The inclined reader could perhaps be stimulated by the additional Python Notebooks supplied by the Magenta team. (All resources mentioned here are collated into a list at the conclusion of this article)
洋红色可用于以多种方式操纵音乐。 洋红色团队提供的其他Python笔记本可能会刺激倾斜的读者。 (本文提到的所有资源都在本文结尾处整理成一个列表)
Of the Colab notebooks made available by the Magenta team, the MusicVAE notebook fit the bill as being something that I could modify to serve as an AI conductor in the capacity desired. The MusicVAE project is described in vivid technical detail via the corresponding research paper [1].
的Colab笔记本电脑通过品红团队提供的MusicVAE笔记本适合该法案为的东西,我可以修改以用作所需容量的AI导体。 MusicVAE项目通过相应的研究论文[1]生动地描述了技术细节。
Upon opening the MusicVAE notebook, one is greeted with the following summary: “MusicVAE learns a latent space of musical scores. This Colab notebook provides functionality for you to randomly sample from the prior distribution and interpolate between existing sequences for several pre-trained MusicVAE models.”
打开MusicVAE笔记本后,会看到以下摘要:“ MusicVAE学习了乐谱的潜在空间。 这款Colab笔记本为您提供了从先前的分布中随机采样并在多个经过预训练的MusicVAE模型的现有序列之间进行插值的功能。”
Via Magenta’s MusicVAE notebook, it is straightforward to feed MIDI files into select pre-trained models. (So as not to lose you in a sea of acronyms: MIDI is a format that facilitates communication between electronic instruments and computers) A boon for the music production industry, and likewise an asset to our AI Composer, MIDI files can house multi-instrument scores. Furthermore, owing to today’s open-source community, sizable collections of MIDI files are freely downloadable; for example check out this Resource [2].
通过Magenta的MusicVAE笔记本,可以很容易地将MIDI文件输入选定的预训练模型。 (为避免使您迷失首字母缩略词:MIDI是一种促进电子乐器与计算机之间进行通信的格式)音乐制作行业的福音,也是我们AI Composer的资产,MIDI文件可以容纳多种乐器分数。 此外,由于当今的开源社区,可免费下载大量MIDI文件。 例如,查看此资源[2]。
With musical scores (via MIDI files) and an AI tool (Magenta) to sample existing works, I was able to sample the scores and create unique, ‘mashed up’ compositions. The resulting, mashed up compositions can be downloaded (in MIDI format) for use in an music editor of your choosing (e.g. GarageBand on a Mac). Despite the joys of mixing tracks live on a PC, there’s something about a printed, sheet music score that I’m a fan of. See the music, play the music, mix the music; I find joy in this particular order.
借助乐谱(通过MIDI文件)和AI工具(洋红色)对现有作品进行采样,我能够对乐谱进行采样并创建独特的“混搭”乐曲。 可以将生成的混音作品下载(以MIDI格式),以供您选择的音乐编辑器(例如Mac上的GarageBand)使用。 尽管可以在PC上实时混音,但我还是喜欢印刷的乐谱。 看音乐,播放音乐,混音乐; 我以这种特殊的顺序感到高兴。
To shore up this last detail, the conversion of MIDI files to sheet music, I found a drag-and-drop web resource that was up to the task. Mission accomplished. I was able to complete the experiment. I used machine learning to create a unique, mashed-up musical score.
为了支持最后一个细节,即将MIDI文件转换为乐谱,我找到了一个可以完成任务的拖放式Web资源。 任务完成。 我能够完成实验。 我使用机器学习来创建独特的混搭乐谱。
未来方向:朝着艺术家友好的,人工智能辅助的乐曲创作工具发展? (Future Directions: Towards an artist friendly, AI-assisted Song Composition Tool?)
With community support and collaborative insight, I’m hoping that the project described at present might spur something larger. I envision a tool that can be of utility to a large cross-section of musicians and songwriters who are seeking a creative spark.
有了社区的支持和协作的见解,我希望目前描述的项目可能会激发更大的收获。 我设想了一种工具,该工具可用于寻求创造性火花的众多音乐人和词曲作者。
Does the notion of composing and mixing music within extended reality environments (e.g. AR/VR environments) generate excitement?
在扩展现实环境(例如AR / VR环境)中创作和混合音乐的想法是否会引起兴奋?
Can streamlined models be employed to run an AI composer directly within someone’s browser or within an app?
可以使用简化的模型直接在某人的浏览器或应用程序内运行AI作曲家吗?
Can a web UI be constructed such that AI composer tools can run seamlessly on a back-end server?
是否可以构建Web UI,使AI Composer工具可以在后端服务器上无缝运行?
Would it be beneficial if we were to provide an environment in which users can use AI to compose a track and then, without leaving the environment, mix the track to their liking?
如果我们要提供一个环境,使用户可以使用AI编写曲目,然后在不离开环境的情况下,按自己的喜好混合曲目,这将是有益的吗?
For starters, however, I would be delighted if this project were to pique the interest of a high school student, an excited young human who cannot wait to fool around with the tool at hand. The enthusiastic student might then use it to create a score of their own, print out a bunch of copies, bring them to their Jazz Band class, and subsequently surprise their unsuspecting classmates with a AI-assisted composition!
但是,对于初学者来说,如果这个项目能够激起一个高中生的兴趣,我将很高兴,他是一个激动的年轻人,迫不及待地想使用手头的工具。 然后,热情的学生可能会用它来创建自己的乐谱,打印出一堆副本,将它们带到爵士乐队的班级,然后用AI辅助的作曲给毫无戒心的同学们惊喜!
深入研究细节:如何亲自执行实验! (Dive Into the Details: How to execute the experiment yourself!)
This project is based upon the Magenta team’s Music VAE project and the corresponding publication [1]. My work expanding upon theirs lives my this Github repo.
该项目基于Magenta团队的Music VAE项目和相应的出版物[1]。 我的工作扩展到他们的生活,这个Github回购。
0a. Start with a good attitude. A knack for transforming frustration into determination is a prized asset in this industry.
0a。 从一个好的态度开始。 将挫折感转化为决心的诀窍是该行业的一项宝贵资产。
0b. You can run my notebook ‘as-is’ in Google Colab. However, you might find it fun to have some songs that you actually want to hear mashed up. If you don’t have MIDI files of these particular tracks, this resource is a good place to begin your search.
0b。 您可以按原样在Google Colab中运行我的笔记本。 但是,您可能会发现一些真正想听的歌曲混在一起很有趣。 如果您没有这些特定音轨的MIDI文件,则此资源是开始搜索的好地方。
Subsequent steps are written with reference to the Ipython notebook displayed below.
后续步骤是参考下面显示的Ipython笔记本编写的。
Open my Github hosted Ipython Notebook. (I’m partial to using Google Colab; simply click the blue button atop the rendering provided below!)
打开我的Github托管的Ipython Notebook 。 (我偏爱使用Google Colab;只需单击下面提供的渲染上方的蓝色按钮!)
2. Locate the cell below “Setting up the Environment” and run it.
2.在“设置环境”下找到单元格,然后运行它。
3. Load the 16 bar trio models by running the cell beneath the “Loading the models.” heading.
3.通过运行“加载模型”下方的单元来加载16 bar三重奏模型。 标题。
N.B 1: My decision to use the 16 bar trio models is arbitrary, and is based upon my intended output, a musical score. The Magenta team’s notebook includes examples based upon other models. These are fun, too! However for the sake of brevity, I shall leave these as areas to be charted by the enthused reader.
注意1:我决定使用16巴三重奏模型是任意的,并且基于我的预期输出,即乐谱。 洋红色团队的笔记本中包含基于其他型号的示例。 这些也很有趣! 但是,为了简洁起见,我将把这些留给有热情的读者绘制。
N.B. 2: It’s okay to be confused by some of these abbreviations and phrases! One can adroitly create an AI composer without being privy to their meanings.
注意2:可以将其中一些缩写词和短语弄混! 一个人可以熟练地创建一个AI作曲家,而无需对其含义有所了解。
However, in the event that there’s some legitimate intrigue surrounding the acronyms VAE and LSTM, I feel obligated to provide insight (or at least attempt to). Also, please delight yourself in this introductory machine learning course provided by MIT. Their laboratory example of using machine learning to toy with music was a key inspiration for this project.
但是,如果在缩略词VAE和LSTM周围存在一些合理的阴谋,我有义务提供见解(或至少尝试这样做)。 另外,请参加麻省理工学院提供的机器学习入门课程。 他们在实验室中使用机器学习来玩音乐的例子是该项目的主要灵感。
MusicVAE is short for Music Variational Auto-Encoder (VAE). Variational auto-encoders operate under the principle that something, say for example, a musical composition, can be modeled as a distribution of latent variables; this is the act of encoding. The distribution of latent variables (latent in that they have no meaning outside of our model) that encodes a musical score can then be decoded back into a musical score; and if your model is sufficiently accurate, the decoded score might be impossible to discern from the original. This notion lies at the heart of ‘deep fakes’…if you’re into that sort of thing.
MusicVAE是Music Variational Auto-Encoder(VAE)的缩写。 可变自动编码器的工作原理是,可以将诸如音乐作品之类的东西建模为潜在变量的分布。 这就是编码的行为。 然后可以将编码乐谱的潜变量的分布(潜变量,因为它们在我们的模型之外没有任何意义)可以解码回为乐谱。 如果您的模型足够准确,则解码后的分数可能无法与原始分数区分开。 这个概念是“深造假”的核心……如果您喜欢这种事情。
The model employed in this experiment is expanded upon by giving it ‘memory’. This is the LSTM aspect. LSTM stands for ‘Long Short-Term Memory’. When encoding a static object like an image, there is no need to account for time. Take all the pixels, map them to a set of distributions, and if you so desire, decode this mapped distribution to yield an image that is minimally different from the inputted image. With a dynamic object, such as a musical score, a single mapping will not suffice. Instead we map overlapping ‘chunks’ of our score at multiple points in time. The takeaway idea of an LSTM is that these overlapping chunks are not taken independently, but as a sequence. A great tool for working with data that contains periodic structure…such as a musical score!
通过为其提供“内存”来扩展此实验中使用的模型。 这是LSTM方面。 LSTM代表“长期短期记忆”。 对静态对象(如图像)进行编码时,无需考虑时间。 取所有像素,将它们映射到一组分布,如果需要,可以解码此映射的分布,以生成与输入图像差异最小的图像。 对于动态对象(例如乐谱),单个映射是不够的。 相反,我们在多个时间点映射分数的重叠“块”。 LSTM的外带想法是,这些重叠的块不是独立地取而代之,而是按顺序进行的。 一个伟大的工具,用于处理包含周期性结构(例如乐谱)的数据!
4a. (Option 1) Load the MIDI files that I have placed in the Github repo. This is done by running the cell beneath “Using stock MIDI files.”
4a。 (选项1)加载我在Github存储库中放置的MIDI文件。 这是通过运行“使用常规MIDI文件”下的单元格来完成的。
4b. (Option 2) Load files of your choosing. (This option assumes that you have MIDI files at your disposal. This is best done by running two cells, one cell for each MIDI track you wish to load)
4b。 (选项2)加载您选择的文件。 (此选项假定您可以使用MIDI文件。最好通过运行两个单元来完成,每个要加载的MIDI轨道一个单元)
5. Extract Trios by running cell beneath “Extract some Trios” heading. This step runs through the MIDI files and extracts trios contained within. A trio can be viewed as a score that contains three parts: drum, bass, lead (e.g. guitar, keys, and/or vocals). The trios extracted are all 16 bars in length. For a given track, multiple trios may be extracted.
5.通过运行“提取某些Trios”标题下的单元格来提取Trios。 此步骤将遍历MIDI文件并提取其中包含的三重奏。 三重奏可以看作是包含三个部分的乐谱:鼓,贝斯,主音(例如吉他,琴键和/或人声)。 提取的三重奏的长度均为16小节。 对于给定的曲目,可以提取多个三重奏。
N.B. For certain tracks, the ‘trio extractor’ can fail to extract any usable trios. In this event, you will have to choose a different track. I may ask the Magenta team to suggest a workaround here.
注意:对于某些曲目,“三重奏提取器”可能无法提取任何可用的三重奏。 在这种情况下,您将不得不选择其他曲目。 我可能会要求洋红色团队在这里提出解决方法。
6. Mash up the Trios! Accomplish this by running the cell below the heading “Trio Mashup Time!”. You can have some fun fooling around with the ‘temperature’ setting, which can change the feel of the mashup quite a bit!
6.混搭三重奏! 通过运行“ Trio Mashup Time!”标题下的单元格来完成此操作。 您可以在“温度”设置中找点乐子,这可以大大改变混搭的感觉!
7. Download your Mashup by running the cell below “Download the MIDI file for some mixing fun!”
7.通过运行“下载MIDI文件以获得一些混音乐趣!”下面的单元格,下载Mashup。
8. (Optional) Open your MIDI Mashup in a music editor of your choice.
8.(可选)在您选择的音乐编辑器中打开MIDI Mashup。
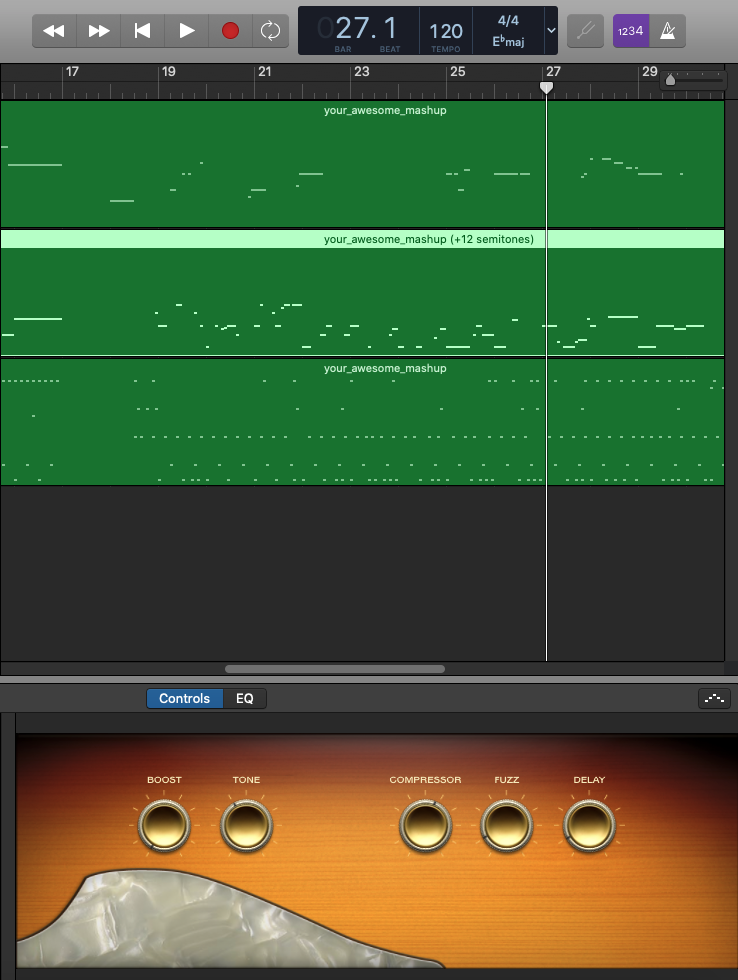
9.(可选)将您的MIDI Mashup转换为乐谱形式,并准备好进行视听乐趣!
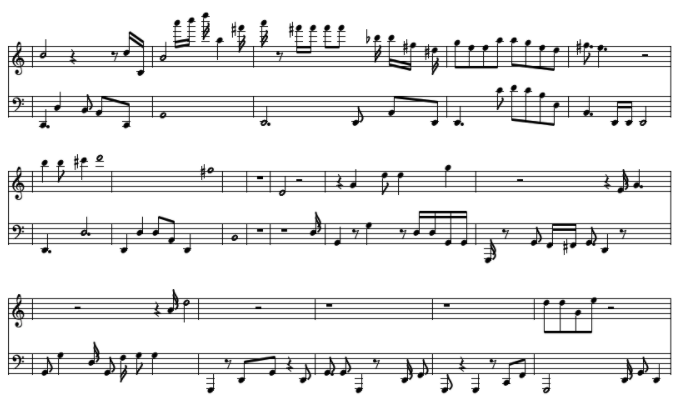
资源资源 (Resources)
My Project’s Github Repository
Blog post describing the MusicVAE notebook
The Magenta teams’s MusicVAE Notebook
Solid source of downloadable MIDI files (thank you Collin Raffel)
可下载MIDI文件的可靠来源(感谢Collin Raffel)
Drag-and-drop MIDI to sheet music web app
致谢(Acknowledgements)
Ava Soleimany and Alexander Amini, the instructors of the MIT course, whose lab inspired me to dig deeper into the intersection between AI and music.
麻省理工学院课程的讲师Ava Soleimany和Alexander Amini的实验室激发了我更深入地挖掘AI与音乐之间的交集的机会。
Christine Liu, and her really cool, AI-centric Instagram account, @ai.noodle. Follow her there for AI generated recipes that are more often than not, delicious. Seeing her ai.noodle blog inspired me to share my ML explorations, too!
克里斯汀·刘( Christine Liu )和她以AI为中心的非常酷的Instagram帐户@ ai.noodle 。 跟着她去那里,那里有AI制作的食谱,这些食谱通常不那么美味。 看到她的ai.noodle博客也启发了我分享我的机器学习探索!
Colin Raffel, who kindly made an awesome collection of MIDI files available.
Colin Raffel ,请提供许多很棒的MIDI文件。
引文 (Citations)
[1] Dinculescu,M.,Engel,J。,&Roberts,A。(2019年)。 MidiMe:使用用户数据个性化MusicVAE模型。
[2] Raffel,C.“基于学习的序列比较方法,及其在音频到MIDI对齐和匹配中的应用”。 博士学位论文,2016。
机器学习 生成