如何进行探索性数据分析
With the onset of my series on EDA, I decided to start it with a question. The answer to the above ‘Why’ lies in what exactly EDA has to give in to the world of data?
随着我关于EDA的系列文章的开始,我决定从一个问题开始。 上述“为什么”的答案在于,EDA究竟要对数据世界产生什么影响?
It’s a methodology introduced by John Tukey in 1969 to analyze data. It’s where researchers view the data from multiple angles, to make sense out of it.
这是John Tukey于1969年引入的一种用于分析数据的方法。 在这里,研究人员可以从多个角度查看数据,以使它们有意义。
A decade ago, there was a widespread misconception that EDA was said to be opposed to statistical modeling. That’s when Chong Ho Yu’s research came in defense of EDA. Now that this method came a long way since then statistics is a crucial element.
十年前,人们普遍误以为据说EDA反对统计建模。 那时Chong Ho Yu的研究是为EDA辩护的。 自从那时以来,这种方法已经走了很长一段路,然后统计才是关键要素。
Looking at aspects of Analytics and ML both, Data exploration is a backdrop for any predictive modeling or model deployment. Once the hypothesis testing is concluded and after the extraction of data, exploration comes in picture. If you consider reverse engineering this step gives you an intricate understanding of your problem statement. let’s look at what exactly EDA gives us.
从Analytics和ML的各个方面来看,数据探索是任何预测性建模或模型部署的背景。 假设检验结束后,在提取数据之后,便进行了探索。 如果您考虑进行逆向工程,则此步骤可以使您对问题陈述有更深入的了解。 让我们看看EDA到底给了我们什么。
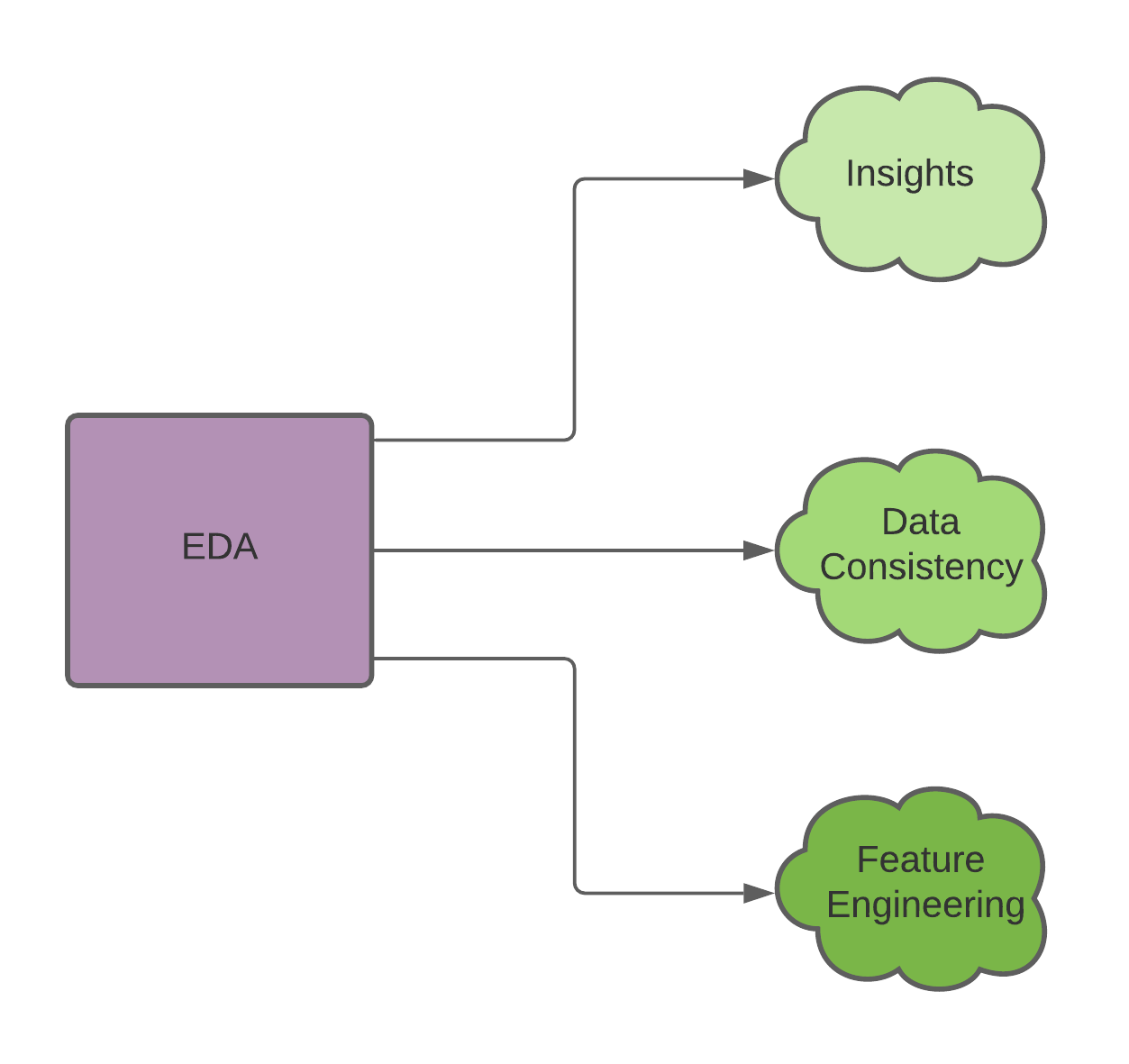
I believe the most crucial part of any analysis is questioning. In reference to the above outputs by EDA, we get to list down the following Questions.
我相信任何分析中最关键的部分是质疑。 参考EDA的上述输出,我们将列出以下问题。
见解 (Insights)
- Are we able to identify variables? 我们能够识别变量吗?
- What behavior do these variables possess? 这些变量具有什么行为?
- Is there a relationship between these variables? 这些变量之间有关系吗?
数据一致性 (Data Consistency)
- If all the data is present? 是否所有数据都存在?
- Are there any missing values? 是否有任何遗漏的值?
- If there are any outliers? 是否有异常值?
特征工程 (Feature Engineering)
- Checks on if any references are present? Majorly for ML purpose any ideas or reference models help in re-usage of the data to build new models. 检查是否存在任何参考? 主要出于ML目的,任何想法或参考模型都有助于重新使用数据以建立新模型。
Conclusion: It is an important component of the research process resulting in organized, well-reviewed, and thoroughly deciphered data.
结论: 它是研究过程中的重要组成部分,它可以产生有条理,经过全面审查和彻底解密的数据。
翻译自: https://medium.com/@deekshat04/why-exploratory-data-analysis-is-pre-eminent-f9ec9ab600b3
如何进行探索性数据分析