ML in CAE (ML IN CAE)
We all know that CAD is for designing a product and CAE is for testing and simulating it.
众所周知,CAD用于设计产品,CAE用于测试和模拟。
Computer-Aided Engineering (CAE) is a tool that supports finding the outcome by applying a discrete solution of partial differential equations for the phenomena to be analyzed.
计算机辅助工程(CAE)是一种工具,它通过对要分析的现象应用偏微分方程的离散解来支持找到结果。
CAE reduces the potential for errors in design, users avoid over-engineering, and the effect of altering a few parameters on the product can be studied.
CAE减少了设计错误的可能性,用户避免了过度设计,并且可以研究更改产品上几个参数的效果。
CAE在产品开发过程和PEA中的未来 (The future of CAE in the product development process and PEA)
Even though CAE has built a strong reputation as a verification, troubleshooting and analysis tool, there is still a perception that sufficiently accurate results come rather late in the design cycle to really drive the design. Smart systems lead to an increased need for multi-physics analysis including controls and contain new materials, to which engineers are often less familiar.
尽管CAE在验证,故障排除和分析工具方面享有很高的声誉,但仍然有人认为足够准确的结果会在设计周期的后期出现,从而真正推动了设计。 智能系统导致对包括控制和包含新材料在内的多物理场分析的需求增加,而工程师通常对此不太熟悉。
By integrating Machine Learning we facilitate powerful problem solving, by ensuring better use of computer resources and including ML based optimizations on pre- and post-processing side of things. Our products can better integrate CAE in the overall product lifecycle management. In this way, we allow connecting simulations with new upcoming product use cases, which is a must for smart products. Such an enhanced engineering process is also referred to as predictive engineering analytics.
通过集成机器学习,我们可以确保更好地利用计算机资源,并在事物的预处理和后处理方面包括基于ML的优化,从而促进强大的问题解决。 我们的产品可以更好地将CAE集成到整个产品生命周期管理中。 这样,我们允许将仿真与即将推出的新产品用例相连接,这对于智能产品而言是必须的。 这种增强的工程过程也称为预测工程分析。
Predictive engineering analytics (PEA) is the refinement of simulation and testing processes to improve collaboration between analysis teams that handle different applications.
预测工程分析(PEA)是对模拟和测试流程的改进,以改善处理不同应用程序的分析团队之间的协作。
CAE中机器学习的一个非常基本的示例: (A Very Basic Example of Machine Learning in CAE:)
ML systems improve the performance of an existing system based on their learning from past experience.
机器学习系统基于对过去经验的学习,可以提高现有系统的性能。
Consider a design system that proposes the dimensions of a car bumper beam using a set of equations. The program outputs the beam dimensions using input data that is provided by the designer. If, for example, the span-to-depth ratio is not satisfactory from an aesthetic viewpoint, the designer must manually introduce modifications. Most conventional programs provide no mechanism for the treatment of feedback. Therefore the design program continues to suggest the same dimensions for the same input, no matter how many times the designer rejects the design.
考虑一个设计系统,该系统使用一组方程式提出汽车保险杠横梁的尺寸。 程序使用设计人员提供的输入数据输出光束尺寸。 例如,如果从美学的角度来看跨度与深度的比率不令人满意,则设计人员必须手动进行修改。 大多数常规程序都没有提供处理反馈的机制。 因此,无论设计人员拒绝设计多少次,设计程序都会继续为相同的输入建议相同的尺寸。
What if the CAE software asked the user for feedback on the generated simulation? The code generalizes and adapts to the identified weaknesses and by incorporating this experience in its Machine Learning core, the system can then avoid similar weak output in the future. In turn, improving performance.
如果CAE软件要求用户提供有关生成的模拟的反馈怎么办? 该代码概括并适应了已发现的弱点,并且通过将这种经验纳入其机器学习核心中,系统可以避免将来出现类似的弱输出。 反过来, 提高性能。
This is one simple way of how ML algorithms can learn through their weaknesses to provide stronger better CAE Simulations later.
这是ML算法如何学习其弱点以在以后提供更强大,更好的CAE仿真的一种简单方法。
机器学习技术通常如何工作? (How Machine Learning Techniques work in general?)
If you already know Machine Learning, skip this section and scroll down to the next sections which relate Simulation to ML techniques.
如果您已经了解了机器学习,请跳过本节,然后向下滚动至将Simulation与ML技术相关的下一部分。
参数调优: (Parameter Tuning:)
A Machine Learning task can be formulated to find the best combination of parameters. For example, Linear Regression is at the heart of the Machine Learning algorithms. The Linear Regression algorithm helps us find the best values for parameters by minimizing the error between the points obtained through linear approximations.
可以制定机器学习任务以找到参数的最佳组合。 例如,线性回归是机器学习算法的核心。 线性回归算法通过最小化通过线性近似获得的点之间的误差,可以帮助我们找到最佳参数值。
决策树: (Decision Trees:)
Most inductive Machine Learning Algorithms attempt to construct decision trees instead of inferring raw rules themselves. DTs work by looking for regularities in data and identifying discriminating features.
大多数归纳机器学习算法都试图构造决策树,而不是自己推断原始规则。 DT通过寻找数据规律性并识别出区别特征来工作。
Every leaf in a decision tree can be seen as a traditional IF-THEN rule, where the IF-part is describing the subspace with Boolean conditions(e.g. “IF0.12 < WALL_YAW < 2.39”), whereas the THEN-part is just our deformation classname (e.g. “THEN denting_mid”).
决策树中的每个叶子都可以视为传统的IF-THEN规则,其中IF部分使用布尔条件(例如,“ IF0.12 <WALL_YAW <2.39”)描述子空间,而THEN部分只是我们的变形类名(例如“ THEN denting_mid”)。
Selecting ‘good’ rules in terms of engineering is the major difficulty here and can be reformulated as a node selection problem within the decision tree. A fully automatic rule selection can be summarized as follows: As a first step, the algorithm ranks all rules within a decision tree in order to find the most meaningful and reliable design-subspaces. In a second step, the algorithm determines how many significant rules actually exist within the dataset, so that an engineer is only confronted with the most reliable and important knowledge within the dataset.
在工程方面选择“好的”规则是这里的主要困难,可以重新构造为决策树中的节点选择问题。 全自动规则选择可以总结如下:第一步,该算法对决策树中的所有规则进行排序,以找到最有意义和最可靠的设计子空间。 第二步,算法确定数据集中实际存在多少个重要规则,以便工程师仅面对数据集中最可靠和最重要的知识。
A machine learning process can be used for example for analysis of crash simulation results. Dimensionality Reduction helps engineers to quickly identify all major deformation modes of a component in a large dataset with thousands of simulations. Our geometry-based dimensionality reduction technique has the advantage that all simulations may be processed entirely independent. Also, a normalized similarity value between simulations results can be computed very easily. The following question, what actually causes certain deformation modes to occur is related to the task of finding large design-subspaces which trigger the specific deformation mode. We found Decision –Tree-based Rule Mining to be an appropriate choice since it matches the properties of data in the field of CAE.
机器学习过程可以例如用于碰撞仿真结果的分析。 降维可帮助工程师通过数千次仿真快速识别大型数据集中零部件的所有主要变形模式。 我们基于几何的降维技术的优势在于,可以完全独立地处理所有模拟。 而且,可以非常容易地计算出模拟结果之间的归一化相似度值。 以下问题实际上导致某些变形模式发生的原因与寻找触发特定变形模式的大型设计子空间的任务有关。 我们发现基于决策树的规则挖掘是合适的选择,因为它与CAE字段中的数据属性相匹配。
梯度提升机 (Gradient Boosting Machines)
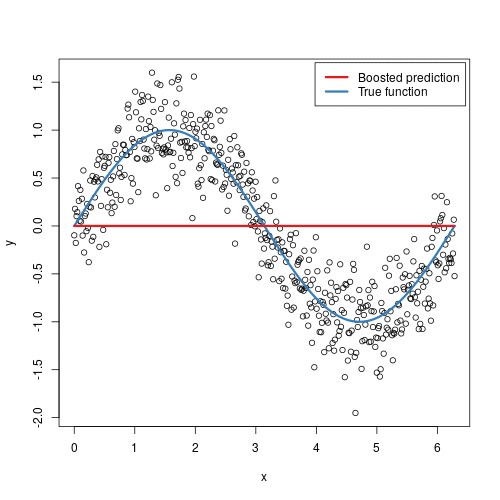
The most frequent approach to data-driven modelling is to build only a single strong predictive model. A different approach would be to build a bucket or an ensemble/tree of models for some particular learning task.
数据驱动建模的最常见方法是仅构建一个强大的预测模型。 一种不同的方法是为某个特定的学习任务构建一个存储桶或模型的集合/树。
GBMs build an ensemble of shallow and weak successive trees with each tree learning and improving on the previous. When combined, these many weak successive trees produce a powerful “committee” that are often hard to beat with other algorithms.
GBM在每棵树的学习和改进的基础上构建了浅,弱的连续树的集合。 当结合在一起时,这些许多弱小的连续树会产生一个强大的“委员会”,而这通常是其他算法所无法比拟的。
The main idea of boosting is to add new models to the ensemble sequentially. At each particular iteration, a new weak, base-learner model is trained with respect to the error of the whole ensemble learnt so far. The learning procedure consecutively fits new models to provide a more accurate estimate of the response variable.
增强的主要思想是按顺序向集合中添加新模型。 在每个特定的迭代中,针对到目前为止已学习的整个集成的错误,将训练一个新的弱基础学习器模型。 学习过程连续适合新模型,以提供对响应变量的更准确的估计。
Often provides predictive accuracy that cannot be beaten. They provide several hyperparameter tuning options that make the function fit very flexible. No data pre-processing required — often works great with categorical and numerical values as is. Handles missing data — imputation not required. In general, the choice of the loss function is up to the researcher with the possibility of implementing one’s own task-specific loss. This high flexibility makes the GBMs highly customizable to any particular data-driven task.
通常提供无法超越的预测准确性。 它们提供了几个超参数调整选项,使该函数非常灵活。 无需进行数据预处理-通常可以很好地使用分类值和数值。 处理丢失的数据-不需要插补。 通常,损失函数的选择取决于研究人员是否有可能实现自己的特定于任务的损失。 这种高度的灵活性使GBM可以高度自定义为任何特定的数据驱动任务。
Problems in GBMs:
GBM中的问题:
The most noticeable problem of the GBMs that arises in practice is their memory-consumption. GBMs often require many trees (>1000) which can be time and memory exhaustive. To use the fitted GBM model to obtain predictions, one has to evaluate all the base-learners in the ensemble. Despite the simplicity of each of the base-learners, when the ensemble is considerably large, obtaining predictions at a fast pace can become time-consuming.
实际上,GBM中最明显的问题是它们的内存消耗。 GBM通常需要许多树(> 1000),这可能会占用大量时间和内存。 为了使用拟合的GBM模型获得预测,必须评估集合中的所有基础学习者。 尽管每个基础学习者都很简单,但是当合奏相当大时,快速获取预测可能会很耗时。
知识网络 (KNNs)
KNN does not explicitly build any model, it simply predicts the new data based on the most similar historical data (i.e., majority class in the nearest neighbor). (Bishop, 2006; Duda et al., 2012)
KNN并未明确建立任何模型,它只是根据最相似的历史数据(即最近邻中的多数类)来预测新数据。 (Bishop,2006; Duda等,2012)
The KNN is a lazy learning approach which implements a simple and efficient non-linear decision rule. This approach often yields consistent results compared with other state-of-the-art machine learning approaches, such as neural networks and support vector machine. Additionally, we decided to go further exploring a weighted fashion of KNN classifier, named NCFS approach (Yang et al., 2012), which learns an optimal feature weighting by minimizing the regularized leave-one-out error.
KNN是一种懒惰的学习方法,它实现了简单而有效的非线性决策规则。 与其他最新的机器学习方法(例如神经网络和支持向量机)相比,该方法通常会产生一致的结果。 此外,我们决定进一步探索KNN分类器的加权方式,即NCFS方法(Yang等人,2012),该方法通过最小化正则化的留一法误差来学习最佳特征加权。
多层神经网络 (Multi-layered Neural Networks)
A multi-layered neural network can be used for approximation of a function f(x,y) which can then be used to assign weights and increasing and decreasing the weights by a constant called: the learning rate, to fine-tune the weights. We can for example use the Delta Rule here. This rule iteratively modifies the weights of connections to reduce the difference, the delta, between the desired output value and the actual output of a neuron. Thus reducing the mean squared error of the network. This is also known as the Widrow-Hoff learning rule and the least mean square (LMS) learning rule. We use Delta rule for backward propagation of errors and then call such networks as feedforward back-propagation networks.
多层神经网络可用于函数f(x,y)的近似,然后可将其用于分配权重并通过称为学习率的常数来增加和减少权重,以对权重进行微调。 例如,我们可以在此处使用增量规则。 该规则迭代地修改连接的权重,以减小所需输出值与神经元实际输出之间的差异,即增量。 从而减少了网络的均方误差。 这也称为Widrow-Hoff学习规则和最小均方(LMS)学习规则。 我们使用Delta规则将错误向后传播,然后将此类网络称为前馈反向传播网络。
Other algorithms we can look at:
我们可以看看其他算法:
- Naive Bayes (NB) classifier employed in (Ogino, 2017) for interior design (Ogino,2017)在室内设计中使用了朴素贝叶斯(NB)分类器
- The support vector machine (SVM) employed in (Bilski, 2014) for the identification of motor parameters and in (Wang, 2011) for solving a regression task in order to predict the relationship between product form elements and product images; 为了预测产品形状元素和产品图像之间的关系,在(Bilski,2014)中使用支持向量机(SVM)来识别运动参数,在(Wang,2011)中使用支持向量机来解决回归任务;
- The deep belief network (DBN) employed in (Jin et al., 2017) for the estimation of motor data and parameters. (Jin等人,2017)中使用的深度信念网络(DBN)用于估计运动数据和参数。
Start of Section: PDMs and PLMs..
本节的开头:PDM和PLM。
产品数据管理PDM中的机器学习: (Machine Learning in Product Data Management PDMs:)
The CAE data model for PDMs can be very complex. Many CAE simulations require access to physical test data for input and correlation.
PDM的CAE数据模型可能非常复杂。 许多CAE模拟都需要访问物理测试数据以进行输入和关联。
The key feature that we look for when simulating the product life cycle is the breadth of a vendor’s portfolio.
我们在模拟产品生命周期时寻找的关键功能是供应商产品组合的广度。
A standard finite element analysis (FEA) is great for validation, and potentially some design as well. However, FEA will prove too slow once you reach the point of operations or early development.
标准的有限元分析(FEA)非常适合验证,也可能适用于某些设计。 但是,一旦您到达运营点或进行早期开发,FEA就会变得太慢。
So, you have a car part, or an operation, but now you need to ensure the day-to-day use and manufacturing of this product.
因此,您有汽车零件或手术,但现在您需要确保该产品的日常使用和制造。
Unfortunately, the high-fidelity simulations you have been working on during the validation and late design stages are too slow to adequately inform the quick pace of product operations and daily manufacturing.
不幸的是,您在验证和后期设计阶段一直在进行的高保真模拟太慢,无法充分反映产品操作和日常制造的快速步伐。
This is where we can make use of data-driven simulation facilitated by state-of-the-art machine learning models.
在这里,我们可以利用最先进的机器学习模型促进的数据驱动模拟。
The difference between data-driven simulation and model-based simulation is that data-driven simulation is based on machine learning and data collection, while a traditional model-based simulation is based on equations and finite elements.
数据驱动的仿真与基于模型的仿真之间的区别在于,数据驱动的仿真基于机器学习和数据收集,而传统的基于模型的仿真则基于方程和有限元。
For data-driven simulation, our software plans to take in the data and run it through a series of machine learning algorithms, such as least squares, neural networks and smart fit functions. We might build a different model with loading conditions based on real operations. Then we can pick the load cases we need to verify. From there, we can build statistical data models.
对于数据驱动的仿真,我们的软件计划接收数据并通过一系列机器学习算法(例如最小二乘法,神经网络和智能拟合函数)运行数据。 我们可能会基于实际操作构建带有加载条件的其他模型。 然后,我们可以选择需要验证的载荷工况。 从那里,我们可以建立统计数据模型。
In future, models can also be connected to the physical part using the Internet of Things (IoT).
将来,还可以使用物联网(IoT)将模型连接到物理部分。
Optimization is key to the quick product development and evolution of your products during each point of the development process.
在开发过程的每个阶段,优化是快速产品开发和产品演进的关键。
PLM中的机器学习—产品生命周期管理: (Machine Learning in PLMs — Product Life Cycle Management:)
Today, the ability to apply machine learning to Product Lifecycle Management (PLM) systems can help car manufacturers better understand and drive insights from product data that has been collected over many years.
如今,将机器学习应用于产品生命周期管理(PLM)系统的能力可以帮助汽车制造商更好地理解和推动多年积累的产品数据的见解。
Product lifecycle intelligence (PLI) is an evolution of PLM that applies artificial intelligence and automation to help PLM users extract meaningful insights from product data, formulate predictions, recommend improvements, and automate actions within systems and processes.
产品生命周期智能(PLI)是PLM的演进,它应用了人工智能和自动化技术来帮助PLM用户从产品数据中提取有意义的见解,制定预测,建议改进并自动执行系统和流程中的操作。
By using PLI and machine learning together, car part manufacturers can proactively prevent delays and failures.
通过将PLI和机器学习一起使用,汽车零件制造商可以主动防止延迟和故障。
PLM is a strategic business approach that involves orchestrating the creation, maintenance, and reuse of intellectual assets, resulting from the innovative efforts of manufacturers, suppliers, and startups. These intellectual assets often engender large volumes of heterogeneous data, leading to the challenge of storage, communication, analysis, reporting, as well as, extracting intelligence from the data. Due to increased connectivity, driven largely by the Internet of Things (IoT), between the products and the enterprise systems used to develop them, the data volumes are growing at an explosive rate. Nevertheless, the technological advances for dealing with petabytes of structured and unstructured data are also surging, resulting in the expansion of the frontiers of analytics, machine learning (ML), and artificial intelligence (AI) in many business disciplines, including PLM.
PLM是一种战略性业务方法,涉及协调制造商,供应商和初创公司的创新努力而形成的知识资产的创建,维护和重用。 这些知识资产通常会产生大量的异构数据,从而带来存储,通信,分析,报告以及从数据中提取情报的挑战。 由于产品和用于开发产品的企业系统之间的连接在很大程度上是由物联网(IoT)驱动的,因此数据量正以爆炸性的速度增长。 尽管如此,处理PB级结构化和非结构化数据的技术进步也在Swift发展,导致包括PLM在内的许多业务领域的分析,机器学习(ML)和人工智能(AI)领域的扩展。
弥合机器学习和CAE之间的差距 机器学习的重要性。 (Bridging the gap between Machine Learning and CAE | Importance of Machine Learning.)
An innovative Design Support System (DSS) is similar to a Decision Support System and can be used for the prediction and estimation of machine specification data such as machine geometry and machine design on the basis of heterogeneous input parameters. An effective design support system can enhance the design process, and thus improve the design product.
创新的设计支持系统(DSS)与决策支持系统相似,可用于基于异构输入参数对机器规格数据(例如机器几何形状和机器设计)进行预测和估计。 有效的设计支持系统可以增强设计过程,从而改善设计产品。
The machine learning algorithms are able to propose the characteristics of the new possible versions of the product or service by learning, correlating, and interpreting the parameters of a database, which represents the characteristics of a product.
机器学习算法能够通过学习,关联和解释代表产品特性的数据库参数来提出产品或服务的新可能版本的特性。
Machine Learning-based Simulation systems are able to analyze every type of data: continuous variables, discrete variables and non-numerical classes. This feature makes these tools superior to other tools (e.g. operating on the mathematical basis), being more robust and allowing a broader and complete set of solutions. A machine learning-based system has the possibility to re-train the model every time new data are inserted, optimizing and refining predictive capacity over time.
基于机器学习的仿真系统能够分析各种类型的数据:连续变量,离散变量和非数值类。 此功能使这些工具优于其他工具(例如,在数学基础上进行操作),功能更强大并且可以提供更广泛和完整的解决方案。 基于机器学习的系统可以在每次插入新数据时重新训练模型,从而随着时间的推移优化和完善预测能力。
Machine learning-aided SDMs allows considerable savings in terms of time and costs as compared to classic design flow where the designer needs to do calculations and simulations in order to choose the specific product.
与传统的设计流程相比, 机器学习辅助的SDM可以节省大量的时间和成本 ,而经典的设计流程则需要设计师进行计算和仿真以选择特定的产品。
Machine Learning can be divided into two: Supervised learning (where we know expected output labels), Unsupervised learning (Autonomous data arrangement and clustering).
机器学习可以分为两种:监督学习(我们知道预期的输出标签),监督学习(自主数据排列和聚类)。
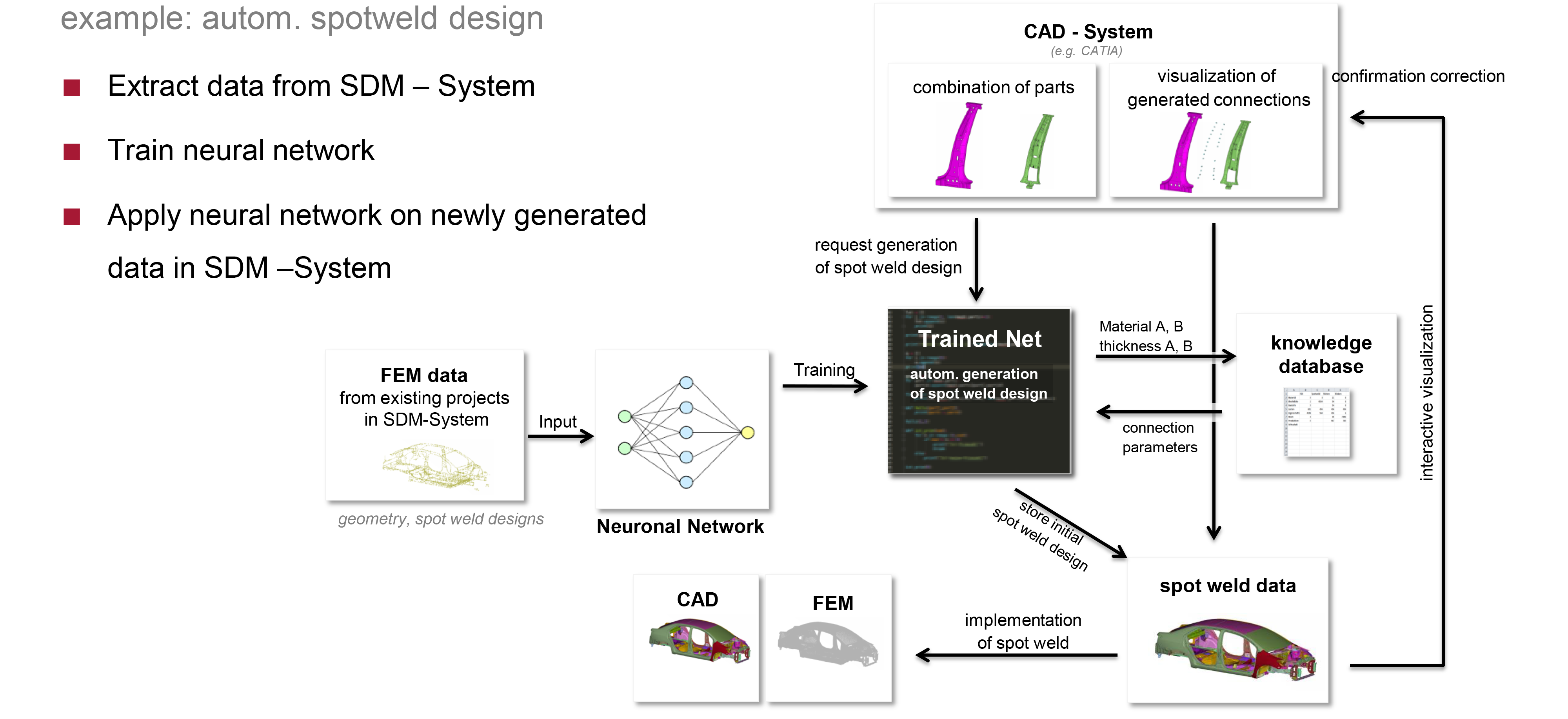
From the above diagrams, the relationship between ML and SDM is clear.
从上图中可以清楚地看到ML和SDM之间的关系。
在SDM系统中实现基于ML的助手所面临的挑战 (Challenges in implementing ML-based assistants in SDM systems)
- Capturing the relationship between data 捕获数据之间的关系
- Gathering the relevant data from the user 收集用户的相关数据
Verdict: Machine Learning will help to further automate tasks in SDM systems.
结论:机器学习将有助于进一步自动化SDM系统中的任务。
Machine learning and simulation have a similar goal: To predict the behaviour of a system with data analysis and mathematical modelling.
机器学习和模拟具有相似的目标:通过数据分析和数学建模来预测系统的行为。
classical simulation computations can not handle the available dimensionality of the data
经典的模拟计算无法处理数据的可用维数
a key challenge in developing a hybrid modelling approach is to bridge the knowledge gap between the two individual communities, which are mostly either experts for machine learning or experts for simulation
开发混合建模方法的关键挑战是弥合两个单独社区之间的知识鸿沟,这两个社区大多数是机器学习专家或仿真专家
为什么要在仿真中使用机器学习? (Why Machine Learning in Simulation?)
“Simulation results are not fully exploited: Especially in the industrial practice, simulations are run with a very specific analysis goal based on expert-designed quantities of interest. This ignores that the simulation result might reveal more patterns and regularities, which might be irrelevant for the current analysis goal but useful in other contexts. Here machine learning can be used.”
“ 仿真结果并未得到充分利用 :尤其是在工业实践中,基于专家设计的感兴趣数量,以非常具体的分析目标运行仿真。 这忽略了模拟结果可能揭示更多的模式和规律性,这可能与当前的分析目标无关,但在其他情况下很有用。 这里可以使用机器学习。”
“Selective surrogate modelling: Even if modern machine learning approaches are used, surrogate models are built for very specific purposes and the decision when and where to use a surrogate model is left to domain experts. In this way, it is exploited too little that similar underlying systems might lead to similar surrogate models and in consequence, too many costly high-fidelity simulations are run to generate the data basis, although parts of the learned surrogate models could be transferred”
“ 选择性代理建模:即使使用了现代机器学习方法,替代模型也会为非常特定的目的而构建,何时以及在何处使用代理模型的决策留给领域专家。 通过这种方式,很少利用相似的基础系统可能导致相似的替代模型,因此,尽管可以转移部分学习的替代模型,但运行太多昂贵的高保真模拟来生成数据基础。
Parameter studies and simulation engines: Parameter and design studies are well-established tools in many fields of engineering. Surprisingly, the frameworks to conduct these studies and to build the surrogate models are third-party solutions that are separated from the core simulation engines. For the parameter study framework, the simulation engine is a black box, which does not know that it is currently used for a parameter study. In turn, the standard rules to generate sampling points in the parameter space are not aware about the internals of the simulation engine. This raises the question how much more efficient parameter studies could be conducted so that both software systems were stronger connected to each other.
参数研究和仿真引擎:参数和设计研究是许多工程领域中公认的工具。 令人惊讶的是,进行这些研究和建立替代模型的框架是与核心仿真引擎分离的第三方解决方案。 对于参数研究框架,模拟引擎是一个黑匣子,它不知道它当前用于参数研究。 反过来,在参数空间中生成采样点的标准规则并不了解模拟引擎的内部。 这就提出了一个问题,即可以进行多少次更有效的参数研究,以使两个软件系统之间的连接更牢固。
A learning simulation engine is a hybrid system that combines machine learning and simulation in an optimal way. Such an engine can automatically decide when and where to apply learned surrogate models or high-fidelity simulations.
一种 学习仿真引擎是一个混合系统,结合机器学习和仿真以最佳的方式。 这样的引擎可以自动决定何时和何处应用学习的代理模型或高保真模拟。
machine learning is a bottom-up approach that generates an inductive, data-based model and simulation as a top-down approach that applies a deductive, knowledge-based model.
机器学习是一种自下而上的方法,可生成基于数据的归纳模型,而模拟则是自上而下的方法,可应用基于知识的演绎模型。
The advanced pairing of machine learning and simulation aligns with the goals of Industry 4.0.
机器学习和仿真的高级配对符合工业4.0的目标。
翻译自: https://medium.com/swlh/bridging-the-gap-between-machine-learning-and-cae-cf5cf4fd4017