opencv 分割边界
This is part of a series describing the development of Moviegoer, a multi-disciplinary data science project with the lofty goal of teaching machines how to “watch” movies and interpret emotion and antecedents (behavioral cause/effect).
这是描述 Moviegoer 开发的系列文章的一部分, Moviegoer 是一个跨学科的数据科学项目,其崇高目标是教学机器如何“观看”电影以及解释情感和前因(行为因果)。
Films are divided into individual scenes, a self-contained series of shots which may contain dialogue, visual action, and more. Being able to programmatically identify specific scenes is key to turning a film into structured data. We attempt to identify the start and end frames for individual scenes by using Keras’ VGG16 image model to group similar frames (images) into clusters known as shots. Then an original algorithm, rooted in film editing expertise, is applied to partition individual scenes.
电影分为各个场景,一系列独立的镜头,其中可能包含对话,视觉动作等。 能够以编程方式识别特定场景是将电影转换为结构化数据的关键。 我们尝试通过使用Keras的VGG16图像模型将相似的帧(图像)分组为称为镜头的簇,来确定单个场景的开始和结束帧。 然后,将以电影编辑专业知识为基础的原始算法应用于分割各个场景。
Overview
总览
Our goal is to, given a set of input frames, identify the start frame and end frame for individual scenes. (This is completely unsupervised, but for the purposes of explanation, I’ll comment on our progress, as well as provide visualization.) In this example, 400 frames, one taken every second from The Hustle (2019) are being fed into the algorithm. Keras’ VGG16 image model is used to vectorize these images, and then unsupervised HAC clustering is applied to group similar frames into clusters. Frames with equal cluster values are similar, so a set of three consecutive frames with the same cluster value could represent a three-second shot of a character.
我们的目标是在给定一组输入帧的情况下,确定各个场景的开始帧和结束帧。 (这是完全不受监督的,但出于解释的目的,我将评论我们的进度并提供可视化效果。)在此示例中,将400帧(每秒从The Hustle(2019)中获取)输入到算法。 使用Keras的VGG16图像模型对这些图像进行矢量化,然后应用无监督的HAC聚类将相似的帧分组为聚类。 具有相同簇值的帧是相似的,因此一组具有相同簇值的三个连续帧可以表示一个角色的三秒钟拍摄。
Here is the vectorization of our sample 400 frames from The Hustle.
这是The The Hustle的样本400帧的向量化。
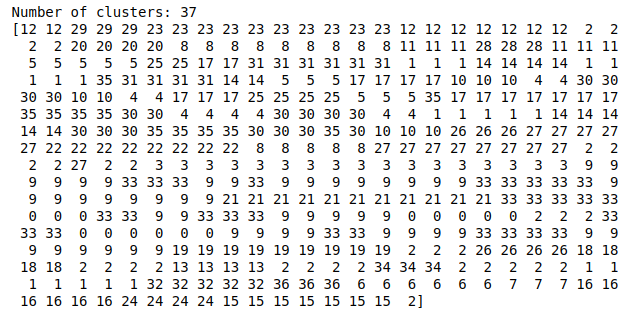
Target visualization
目标可视化
In this example, we have two partial scenes and two complete scenes. Our goal is to identify the scene boundaries of each scene; in this example, we’ll try and identify the boundaries of the blue scene. I’ve colored in this visualization manually, to illustrate our “target”.
在此示例中,我们有两个局部场景和两个完整场景。 我们的目标是确定每个场景的场景边界; 在此示例中,我们将尝试确定蓝色场景的边界。 我已在此可视化中手动上色,以说明我们的“目标”。
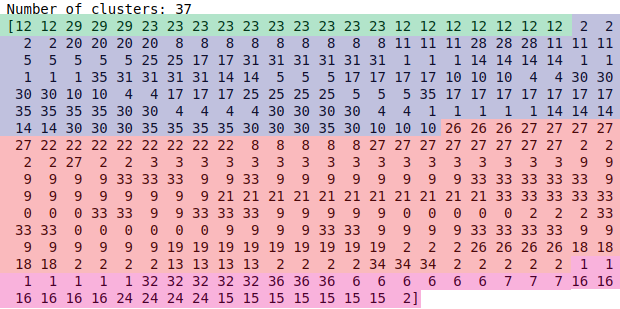
五步算法 (Five-step algorithm)
Step 1: Finding the A/B/A/B Shot Pattern
步骤1:找出A / B / A / B射击图案
Among all 400 frames, we look for any pairs of shots that form an A/B/A/B pattern.
在所有400帧中,我们寻找形成A / B / A / B模式的任何镜头对。
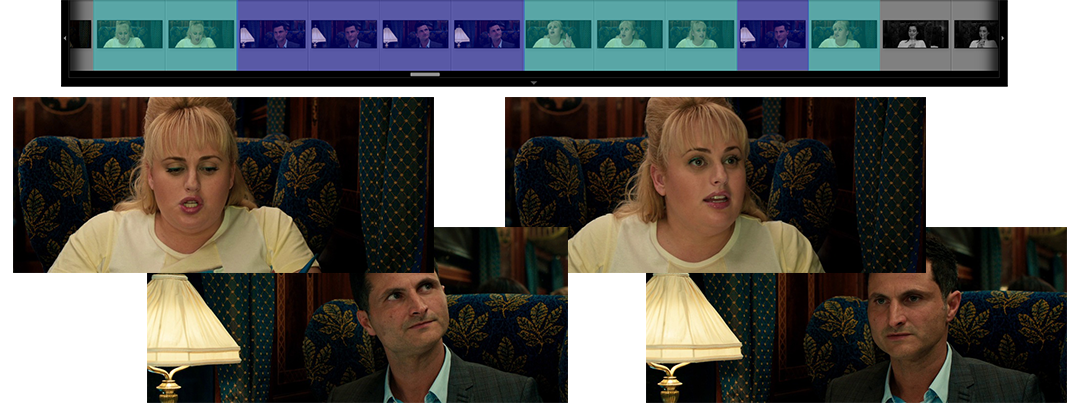
Step 2: Checking for MCUs
步骤2:检查MCU
Finding four A/B/A/B patterns, we run each shot through the MCU image classifier. Two of the patterns were rejected because they contain a shot that doesn’t pass the MCU check. In the below image, the top shot-pair represents our example scene.
找到四个A / B / A / B模式,我们通过MCU图像分类器运行每个镜头。 其中两个模式被拒绝,因为它们包含未通过MCU检查的镜头。 在下图中,最上面的镜头对代表我们的示例场景。
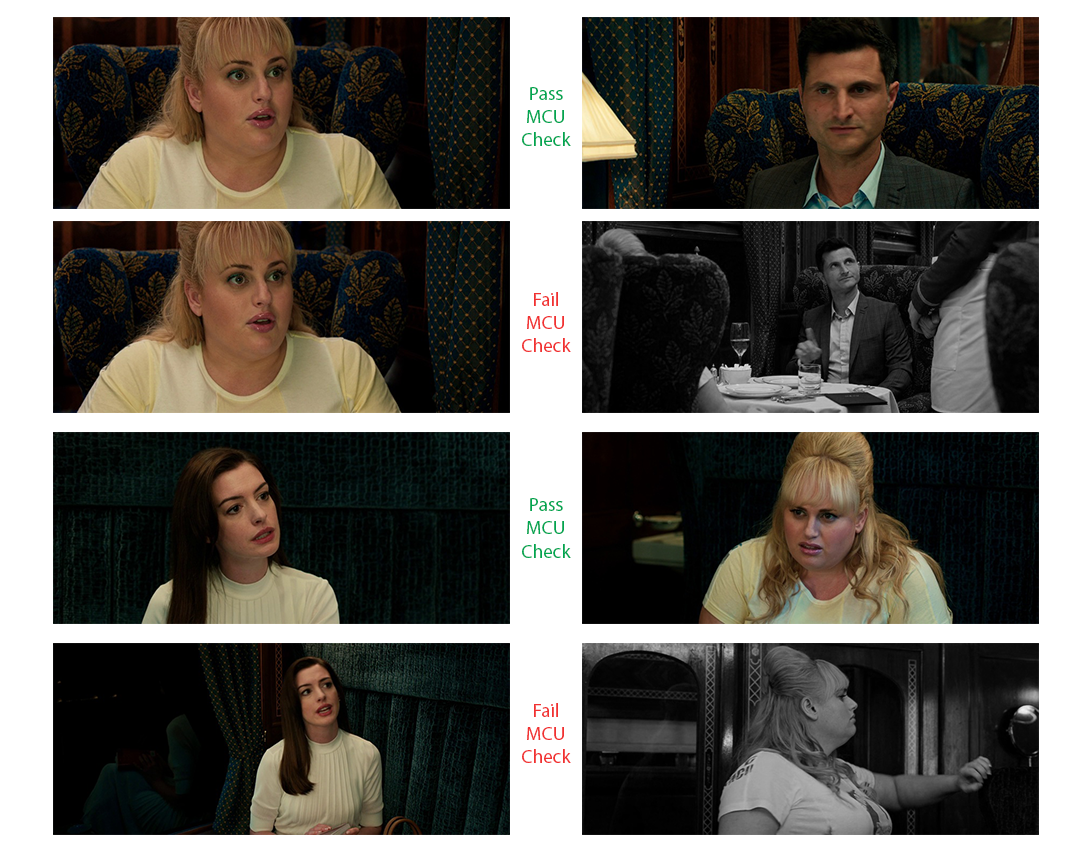
Step 3: Designating a Preliminary Scene Boundary: Anchor Start/End
步骤3:指定初步的场景边界:锚点开始/结束
Once we’ve confirmed that we’re looking at Medium Close-Up shots, we can reasonably believe that we’re looking at a two-character dialogue scene. We look for the first and last appearances of either shot (regardless of A or B). These frames define the Anchor Start and Anchor End Frames, a preliminary scene boundary.
一旦我们确认要查看中景特写镜头,就可以合理地认为我们正在查看两个字符的对话场景。 我们寻找镜头的第一和最后出现(无论A或B)。 这些框架定义了初始场景边界“锚定起点”和“锚定终点”。
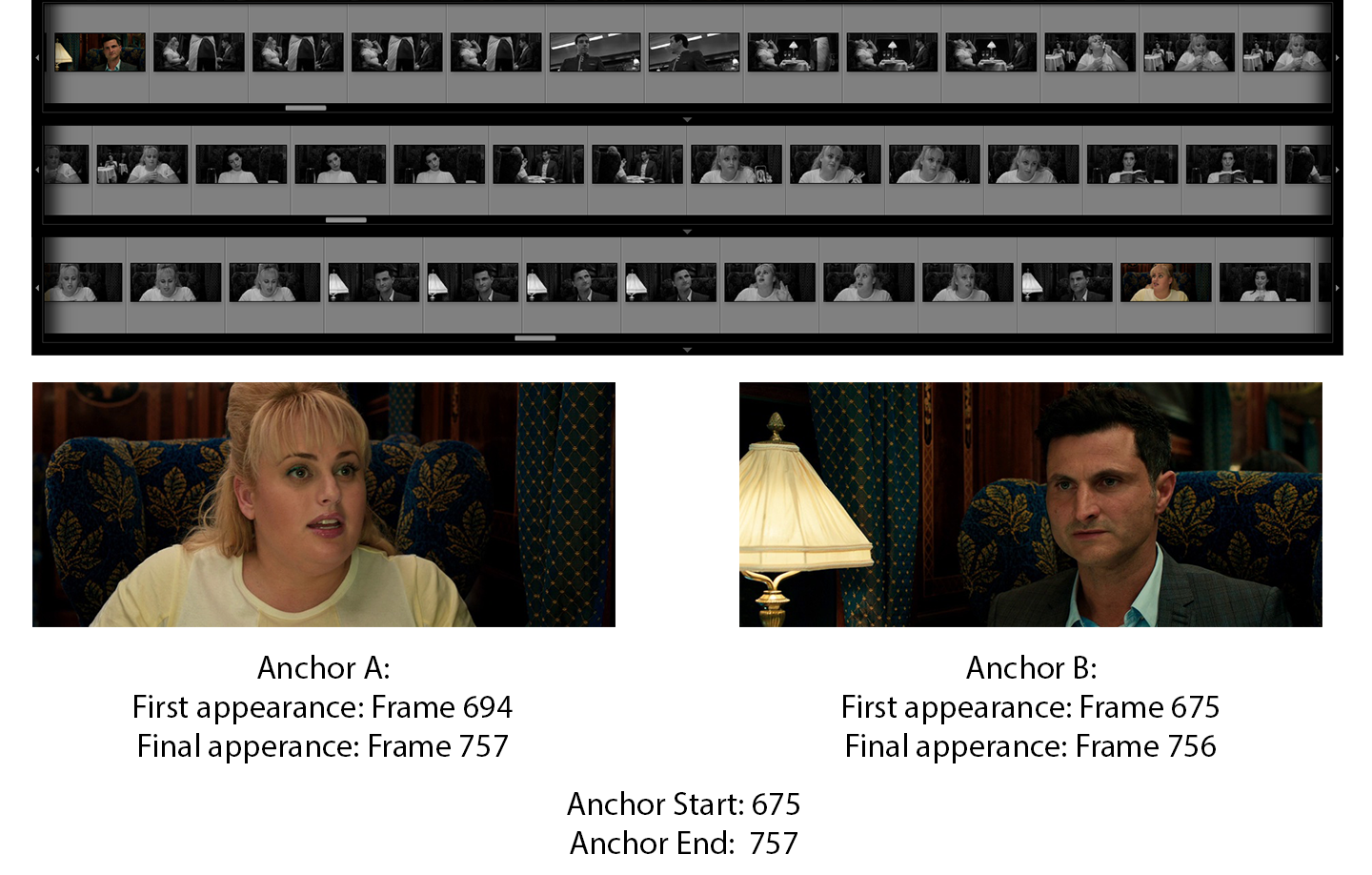
Step 4: Identify Cutaways
步骤4:确定切面
In between the Anchor Start and Anchor End are many other shots known as cutaways. These may represent any of the following:
在Anchor Start和Anchor End之间还有许多其他镜头,称为切面图。 这些可能代表以下任何一种:
- POV shots, showing what characters are looking at offscreen POV镜头,显示在屏幕外正在看什么角色
- Inserts, different shots of Speaker A or B, such as a one-off close-up 插入扬声器A或B的不同镜头,例如一次性特写镜头
- Other characters, both silent and speaking 其他字符,包括静音和说话
After we identify these cutaways, we may be able to expand the scene’s start frame backward, and the end frame forward. If we see these cutaways again, but before the Anchor start or after the Anchor end, they must still be part of the scene.
确定这些切点后,我们可以向后扩展场景的开始帧,向后扩展结束帧。 如果再次看到这些切角,但在“锚点”开始之前或“锚点”结束之后,它们仍必须是场景的一部分。
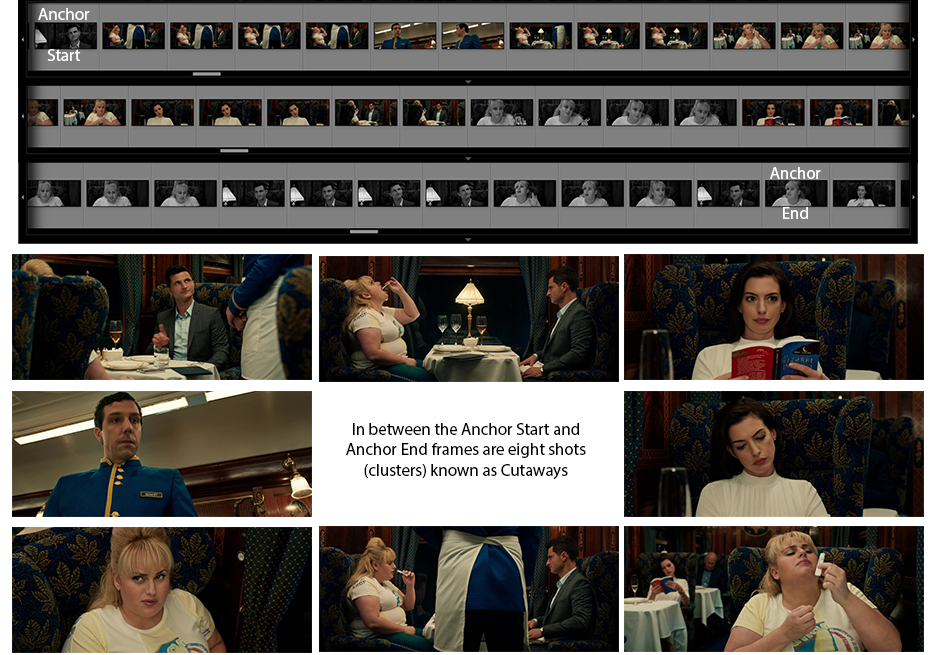
Step 5a: Extending the Scene End
步骤5a:扩展场景结束
After the Anchor End are three frames with a familiar shot (cluster). Since we saw this cluster earlier, as a Cutaway, we incorporate these three frames into our scene. The following frames are unfamiliar, and are indeed not part of this scene.
锚端之后是三帧,并带有熟悉的镜头(群集)。 由于我们较早地看到了该群集,因此它是Cutaway,因此将这三个帧合并到场景中。 以下帧是陌生的,并且确实不是该场景的一部分。
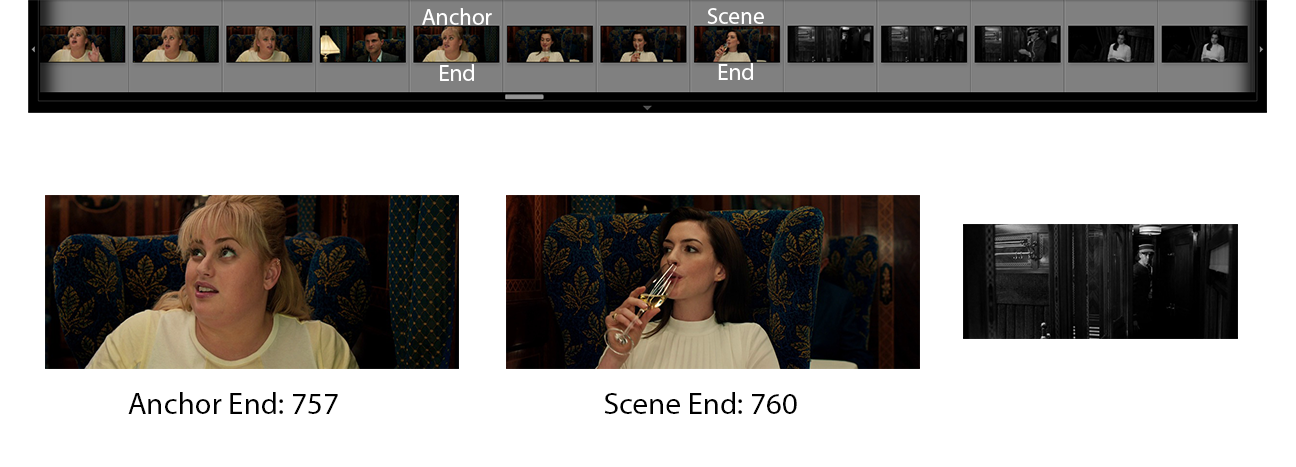
Step 5b: Extending the Scene Start
步骤5b:扩展场景开始
We apply this same technique to the scene’s beginning, in the opposite direction. We find many Cutaways, so we keep progressing earlier and earlier until no more Cutaways are found.
我们将相同的技术应用于相反方向的场景开始。 我们发现了很多剖面图,因此我们会越来越快地进行开发,直到找不到更多剖面图为止。
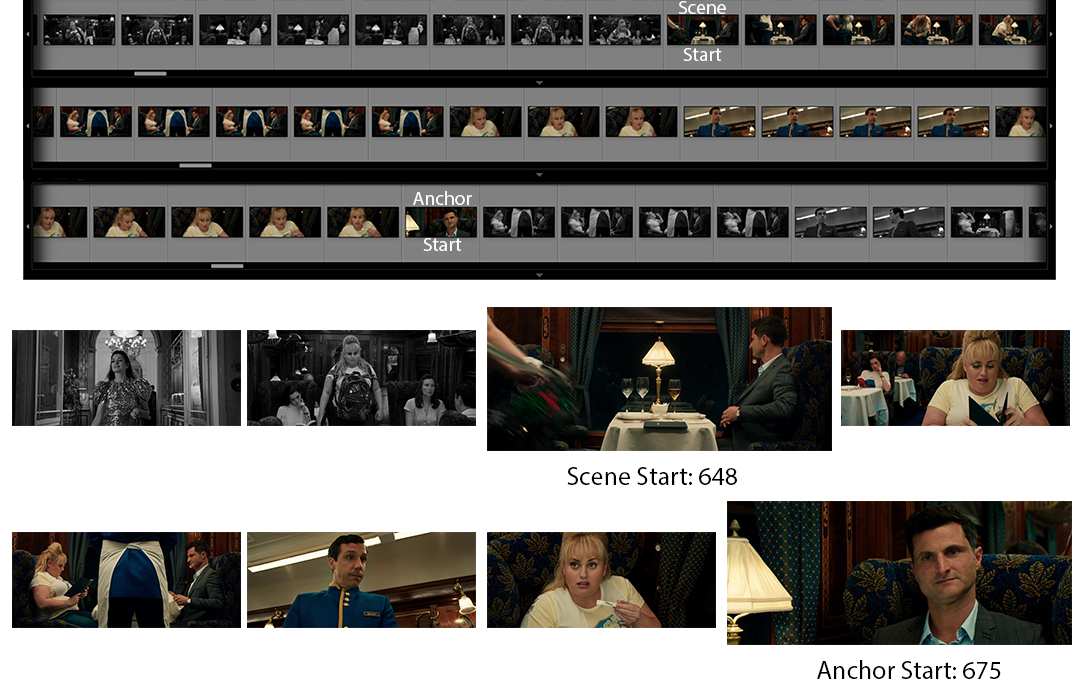
Evaluation
评价
Below is a visualization of the total frames in the scene, with the blue highlighted frames included in our prediction, and the orange highlighted frames not included in our prediction. This algorithm managed to label most frames of the scene. Although some frames were missed at the scene’s beginning, these are non-speaking introductory frames. The scene takes some time to get started, and we’ve indeed captured all frames containing dialogue, the most important criteria.
以下是场景中所有帧的可视化,蓝色的突出显示的帧包含在我们的预测中,橙色的突出显示的帧不包含在我们的预测中。 该算法设法标记了场景的大多数帧。 尽管在场景开始时就错过了一些框架,但这些都是非言语性的入门框架。 场景需要一些时间才能开始,我们确实捕获了所有包含对话(最重要的条件)的帧。
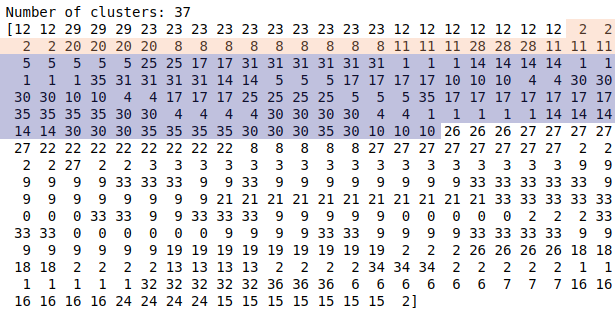
Wanna see more?
想看更多吗?
Repository: Moviegoer
资料库: Moviegoer
Part 1: Can a Machine Watch a Movie?
第1部分: 机器可以看电影吗?
Part 2: Cinematography Shot Modeling
第2部分: 摄影镜头建模
Part 3: Scene Boundary Partitioning
第3部分: 场景边界分区
Part 4: Dialogue Attribution
第4部分: 对话归因
Part 5: Four Categories of Comprehension
第五部分: 四类理解
Part 6: Vision Features
第6部分: 视觉功能
翻译自: https://medium.com/swlh/moviegoer-scene-boundary-partitioning-95a0192baf1e
opencv 分割边界