数据科学与大数据是什么意思
重点 (Top highlight)
What is data science? Is a simple question, but the answers are often confusing. I regularly hear folks say that data science is nothing more than statistics dressed up in fancy clothes. Data science has jokingly been called statistics on a Mac. And a data scientist has been called a data analyst who lives in California. 😂
什么是数据科学? 这是一个简单的问题,但答案常常令人困惑。 我经常听到人们说,数据科学不过是打扮得很漂亮的统计数据。 数据科学在Mac上被戏称为统计学 。 一位数据科学家被称为住在加利福尼亚的数据分析师 。 😂
While these statements are humorous, it’s not at all obvious what data science encompasses. There have been many data science Venn diagrams and many definitions over the years. However, in my research, the ones I found were either convoluted or missing one of the three core data science functions.
这些陈述虽然很幽默,但数据科学包含的内容一点也不明显。 多年来,已经有许多数据科学的维恩图和许多定义。 但是,在我的研究中,发现的结果要么令人费解,要么就缺少了三个核心数据科学功能之一。
In this article, you’ll learn about the three primary parts of data science. You’ll also learn about an emerging type of data science project. Finally, you’ll see two other areas that are important to data science, but not quite part of the core.
在本文中,您将学习数据科学的三个主要部分。 您还将了解新兴的数据科学项目类型。 最后,您将看到另外两个对数据科学重要的领域,但并不是核心领域的一部分。
A data science project has one of three goals — either to provide insight, establish causality, or make predictions. These three goals are associated with the domains of data analysis, statistics, and machine learning.
数据科学项目具有三个目标之一- 提供见解 , 建立因果关系或做出预测 。 这三个目标与数据分析 , 统计和机器学习的领域有关 。
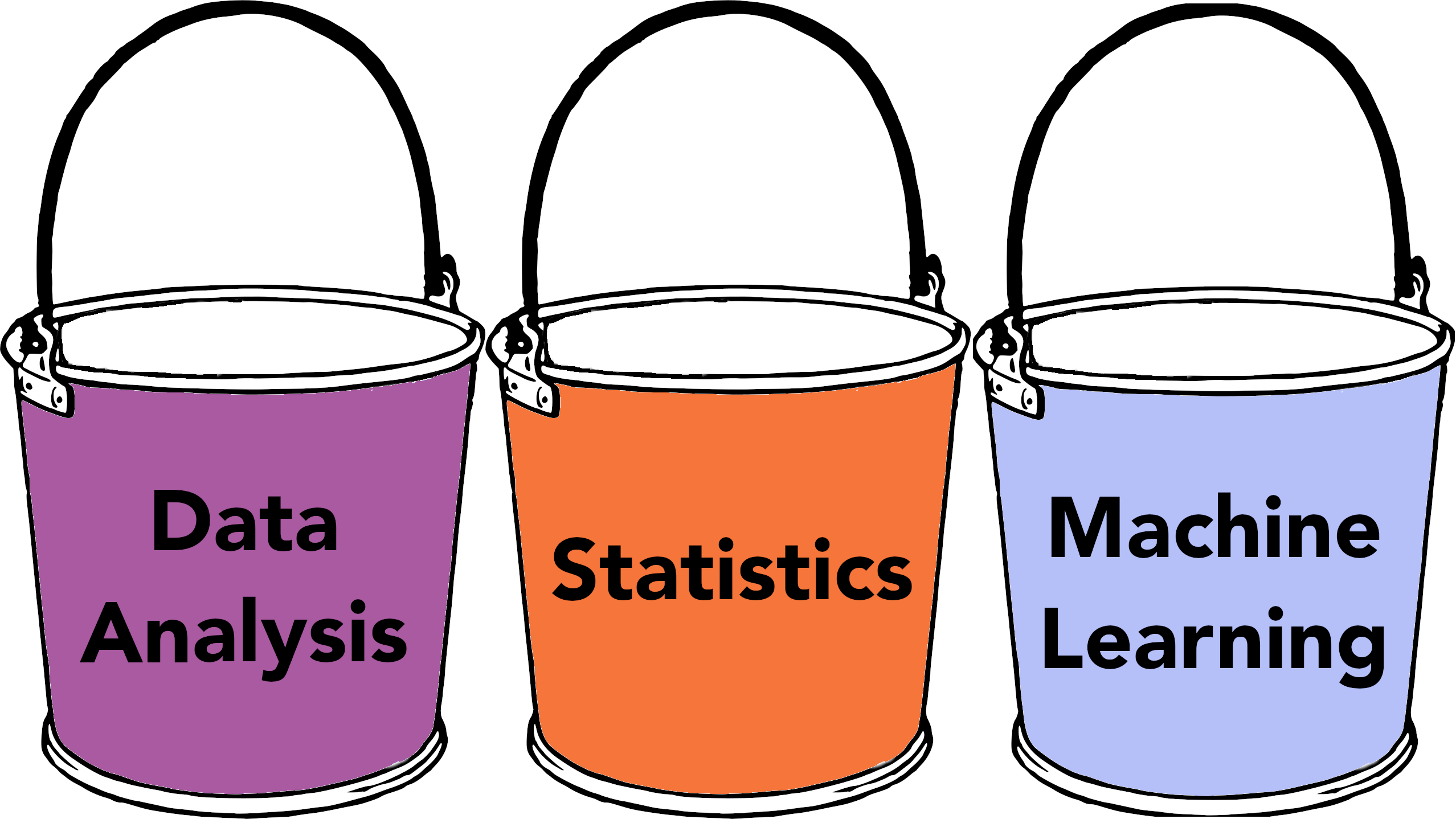
Analysis is used to extract and convey insights from existing data.
分析 用于从现有数据中提取和传达见解。
Statistics is used to establish causality.
统计用于确定因果关系。
Machine learning has prediction as its goal.
机器学习以预测为目标。
数据科学的定义 (A Definition of Data Science)
Here’s my definition that encompasses all three domains:
这是我的定义,涵盖了所有三个领域:
Data science is using data to make better decisions with analysis for insight, statistics for causality, and machine learning for prediction.
数据科学正在使用数据做出更好的决策,其中包括对见解的分析,对因果关系的统计以及对预测的机器学习 。
It’s possible to be solely a data analyst, statistician, or machine learning engineer. However, a data scientist is a person who can do all three. 👍
可能完全可以是数据分析师,统计学家或机器学习工程师。 但是, 数据科学家是可以做到这三者的人。 👍
After drafting this article, I saw that the insightful and eloquent Cassie Kozyrkov had come to a similar conclusion— she defines data science as “the discipline of making data useful” and then breaks it into statistics, machine learning, and data-mining/analytics. Her similar breakdown convinced me that it’s valuable to try to help folks understand what data science is by focussing on these three core aspects. 🎉
在起草本文之后,我看到有见地和雄辩的Cassie Kozyrkov得出了类似的结论 -她将数据科学定义为“使数据有用的学科”,然后将其分为统计,机器学习和数据挖掘/分析。 。 她的类似故障使我相信,通过专注于这三个核心方面,尝试帮助人们了解数据科学是很有价值的。 🎉
Also, I was humbly reminded that most any half-way decent thought I’ve had, Cassie probably had years ago. 😀
另外,我很谦虚地提醒我,卡西大概在几年前就已经经历过大多数中庸的想法。 😀
数据科学背景 (Data Science Context)
Here’s my depiction of how data flows through an organization. The core data science roles are highlighted in peach.
这是我对数据如何在组织中流动的描述。 桃子中突出显示了核心数据科学角色。
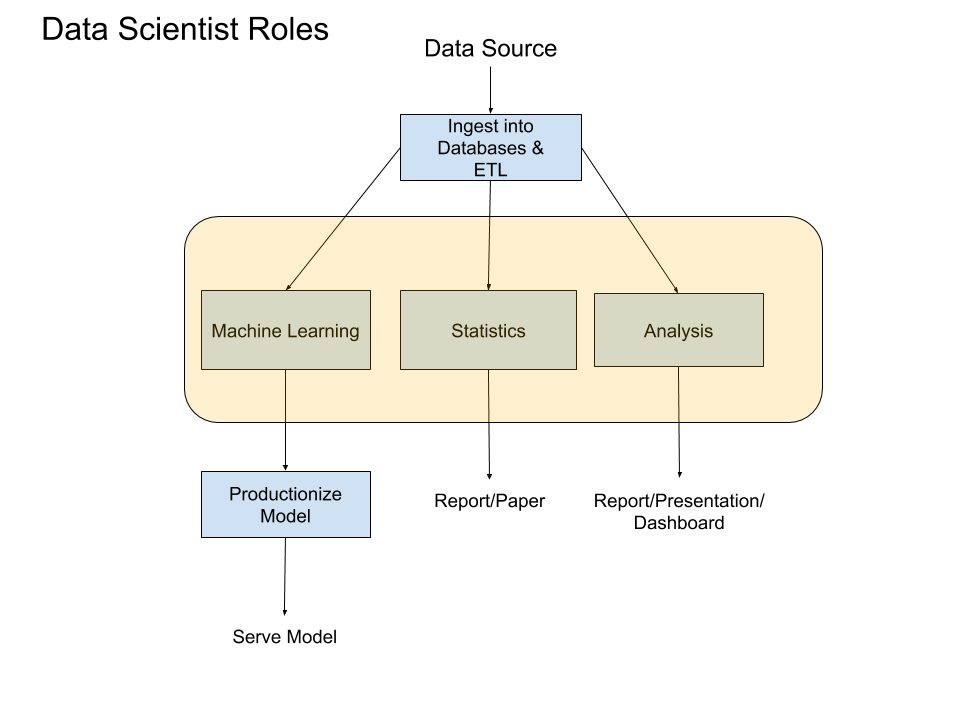
Let’s look at data analysis first.
首先让我们看一下数据分析。
数据分析📊 (Data Analysis 📊)
The goal of data analysis is to find insights in existing data.
数据分析的目的是在现有数据中寻找见解。
Example analysis question: What caused the sales drop last month?
示例分析问题: 是什么导致上个月的销售下降?
The person asking the question might want you to bring save them time by bringing relevant research. Hat tip to Cassie Kozyrkov for explaining this idea well in this article.
提出问题的人可能希望您通过进行相关研究来节省他们的时间。 致Cassie Kozyrkov的技巧文章很好地解释了这个想法。
Alternatively, the question asker might want you to answer their question and craft a narrative. You’ll then use the data as evidence for your argument as to why sales fell.
或者,提问者可能希望您回答他们的问题,并进行叙述。 然后,您将使用这些数据作为有关销售为什么下降的论据的证据。
A data analyst’s job requires exploring questions honestly. You need working hypotheses, but you must follow where the evidence leads and generate new hypotheses based on what you find.
数据分析师的工作需要诚实地探索问题。 您需要有效的假设,但您必须遵循证据的依据,并根据发现的内容生成新的假设。
Data analysis requires critical thinking and intellectual honesty.
数据分析需要批判性思维和理性的诚实。
Data analysis used poorly is obfuscation. It’s How to Lie with Statistics. It’s claiming the USA is doing well in terms of COVID-19 and supporting that claim with a chart showing the number of deaths as a proportion of cases. 🤦♂ ️A much more relevant metric is the number of deaths as a proportion of the population. You can see that chart for large developed nations below below.
数据分析使用不当是混淆。 这是如何撒谎统计 。 它声称美国在COVID-19方面表现良好 ,并通过图表显示死亡人数占案件总数的比例来支持这一说法。 relevant️更重要的指标是死亡人数占总人口的比例。 您可以在下面看到大型发达国家的图表。
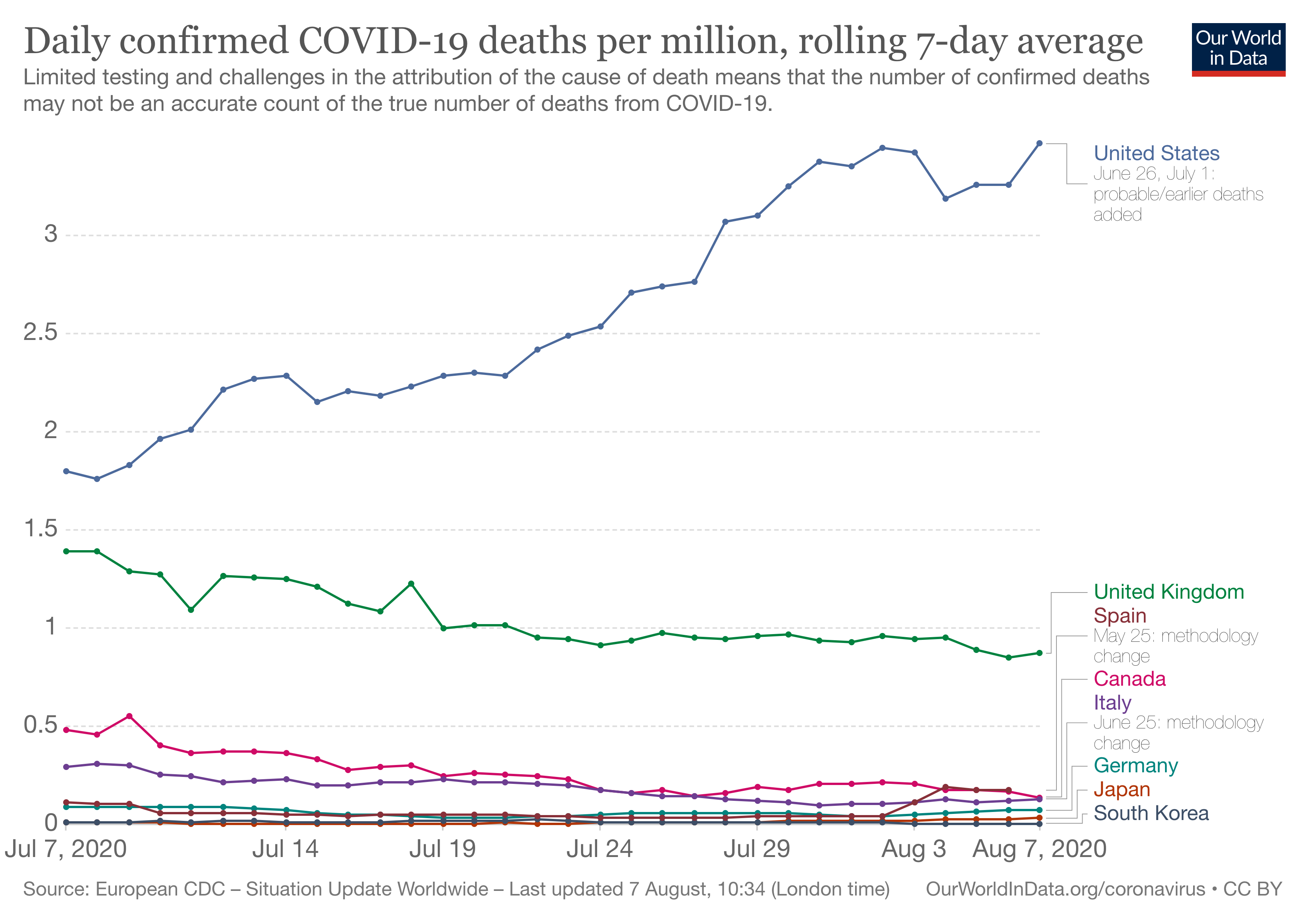
In data analysis you might be dipping your toes into the territory of causality, but you should be careful about making causal claims. You aren’t create experiments and using inferential statistics.
在数据分析中,您可能会涉足因果关系领域,但在提出因果关系声明时应格外小心。 您不会创建实验,也不会使用推理统计信息。
Stock market talking heads do analysis all day, and often use the language of causality. Their explanations are plausible, but they generally can’t be tested.
股市谈话负责人整天都进行分析,并经常使用因果关系语言。 他们的解释是合理的,但通常无法进行测试。
Why did the stock market go up today? Traders liked the jobs numbers.
为什么今天的股市上涨? 贸易商喜欢工作数量。
Why did it go down? Traders brushed off the bad jobs report.
为什么下降了? 交易员们清除了不良工作报告。
You should be skeptical. These causal statements are not disconfirmable.
您应该对此表示怀疑。 这些因果关系的陈述是不可接受的。
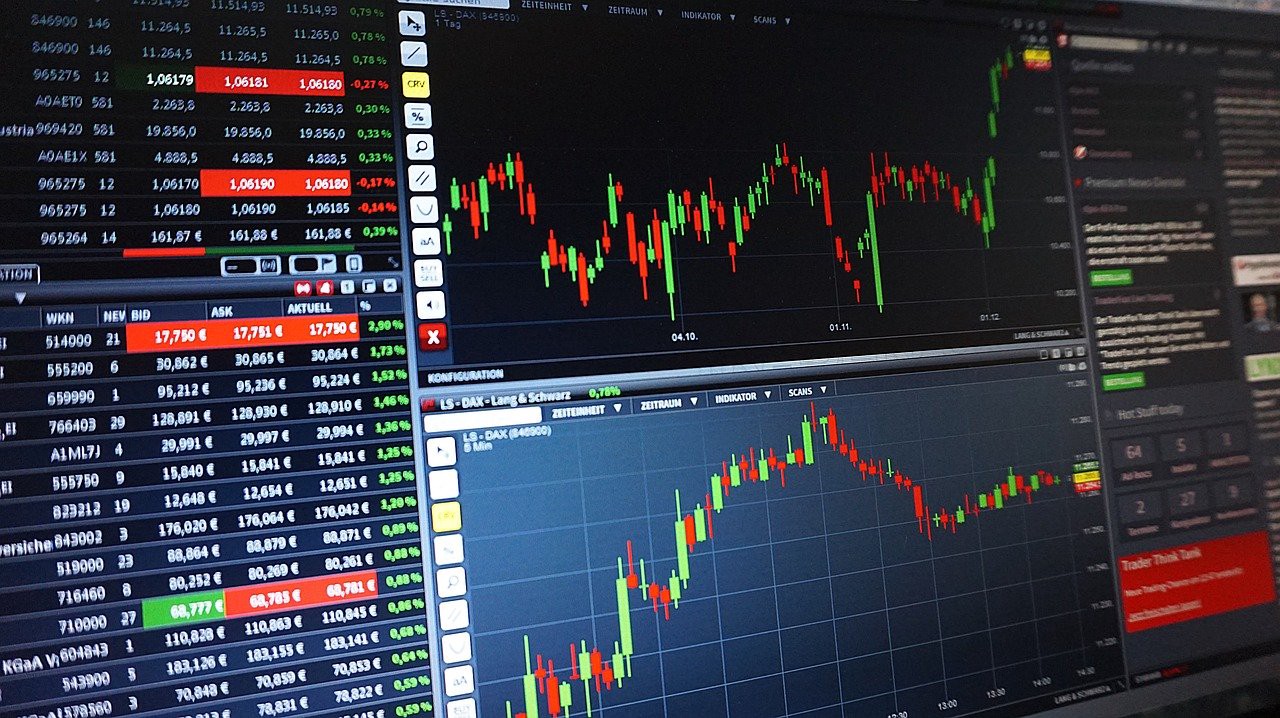
When doing data analysis reports and presentations are often one-off affairs. If you find yourself presenting the same metrics and charts repeatedly. It might be a good idea to set up a dashboard that will automatically grab the data and show those metrics and visualizations to stakeholders.
进行数据分析时,报告和演示文稿通常是一次性的事务。 如果您发现自己重复显示相同的指标和图表。 最好设置一个仪表板,该仪表板将自动获取数据并将这些指标和可视化内容显示给利益相关者。
The most common technology tools for data analysis are SQL, Excel, Tableau in that order. Programming with Python and R are next most common. See my analysis of the technologies for data analyst positions here.
依次进行数据分析的最常用技术工具是SQL,Excel和Tableau。 其次是使用Python和R进行编程。 见我的技术来进行数据分析职位分析在这里 。
Becoming competent with data analysis generally requires the least training time of the three areas. You need to understand how to query data, use descriptive statistics, and display data. You don’t necessarily need programming skills or advanced stats. However, programming skills are often useful to automate analyses.😀
要胜任数据分析,通常需要在这三个领域中花费最少的培训时间。 您需要了解如何查询数据,使用描述性统计信息以及显示数据。 您不一定需要编程技能或高级统计信息。 但是,编程技巧通常对于自动化分析很有用。
Now let’s look at the second core domain of data science: statistics.
现在,让我们看一下数据科学的第二个核心领域:统计。
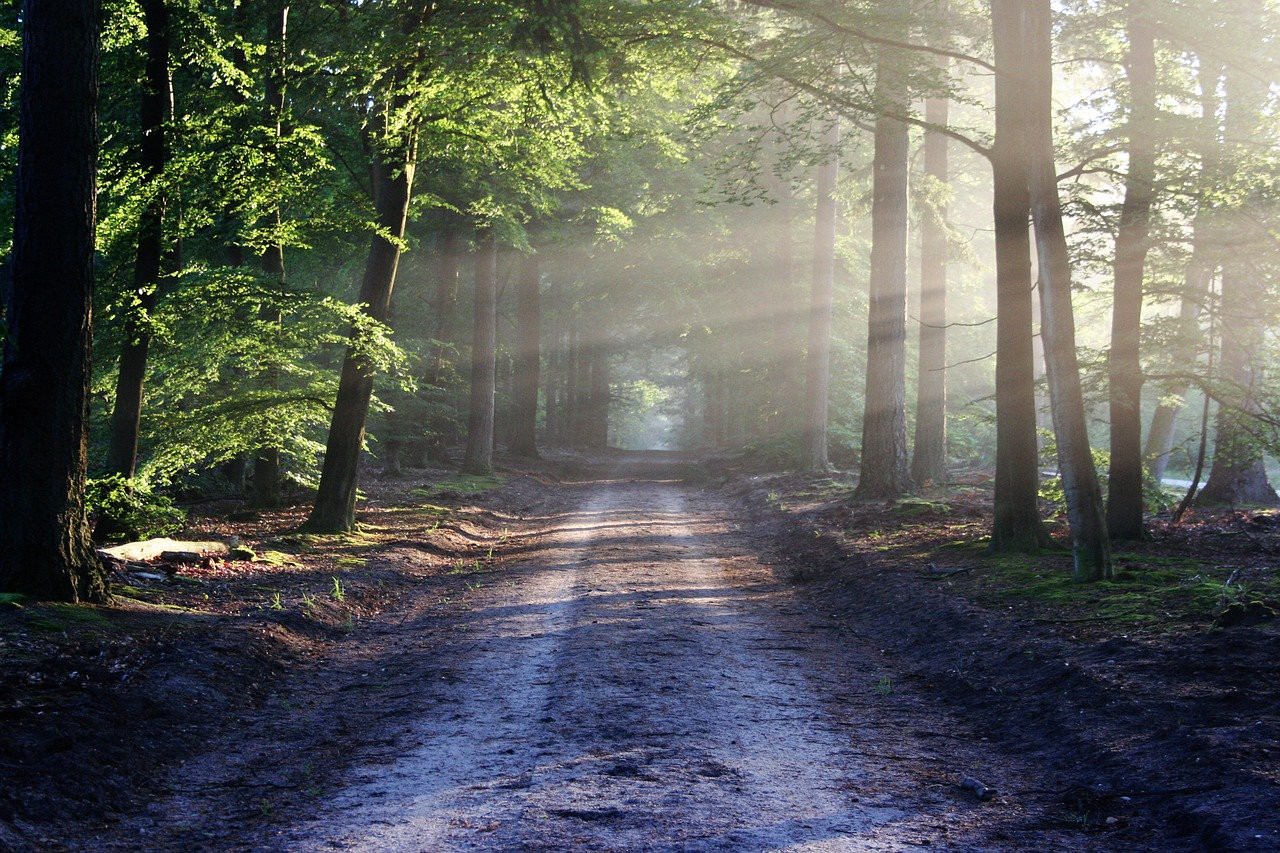
统计🔢 (Statistics 🔢)
Statistics is a key part of the scientific method. It’s how we take the data we have collected and apply probability and mathematical rigor to make causal claims.
统计是科学方法的关键部分。 这就是我们如何使用已收集的数据,并运用概率和数学严格度进行因果关系声明的方法。
Example statistics question: Which version of our website results in more sales?
统计问题示例:我们网站的哪个版本带来了更多的销售?
Let’s say you want to improve your website’s conversion rate. Of course you’ve used best practices in user experience and website design. 😀 Now you have two different website designs you want to test.
假设您要提高网站的转化率。 当然,您已经在用户体验和网站设计中使用了最佳实践。 😀现在,您有两个要测试的网站设计。
You want to to extrapolate to a population based on a randomized control experiment with a sample from that population. If you want to do this with an A/B test from frequentest statistics, you need to determine up front how much data to collect and what your cutoff will be to determine significance. No cheating! Statistics is all about rigor. ☝️
您想根据随机对照实验使用该人群的样本推断该人群。 如果要通过最频繁的统计信息进行A / B测试,则需要预先确定要收集多少数据以及确定的重要性。 别作弊! 统计数据是关于严谨性的。 ☝️
Alternatively, to answer this question you could use the multi-armed bandit test from Bayesian statistics. Both methods seek to draw inferences from a random sample of the population. See this blog post for more on the topic.
另外,要回答这个问题,您可以使用贝叶斯统计中的多臂强盗测试。 两种方法都试图从总体的随机样本中得出推论。 有关该主题的更多信息 ,请参见此博客文章 。
When doing statistics you care about effect sizes, p-values, and confidence intervals or credible intervals. You are down with the central limit theorem. You know statistical distributions and common statistical tests such as Chi-squared tests, ANOVA, linear regression, and logistic regression. You might do time series with ARIMA or Holt-Winters exponential smoothing.
在进行统计时,您会关注效果大小,p值以及置信区间或可信区间。 您不满意中心极限定理。 您知道统计分布和常见的统计检验,例如卡方检验,ANOVA,线性回归和逻辑回归。 您可以使用ARIMA或Holt-Winters指数平滑进行时间序列。
You might use R, Python (likely with pandas and statsmodels), or SAS as your tools.
您可以使用R,Python(可能带有pandas和statsmodels)或SAS作为工具。
Stats is often concerned with matching data to the underlying theoretical distribution of some phenomena. Here’s a great article on the history of data science vs. statistics.
统计数据通常涉及将数据与某些现象的基础理论分布进行匹配。 这是一篇有关数据科学与统计历史的精彩文章 。
Depending on your research problem you may need deep domain expertise or someone who has it on your team. 👍
根据您的研究问题,您可能需要深厚的专业知识或团队中的专业人才。 👍
Now let’s check out machine learning, the cool kid who made data science popular.
现在,让我们看看机器学习,这是使数据科学流行的好孩子。
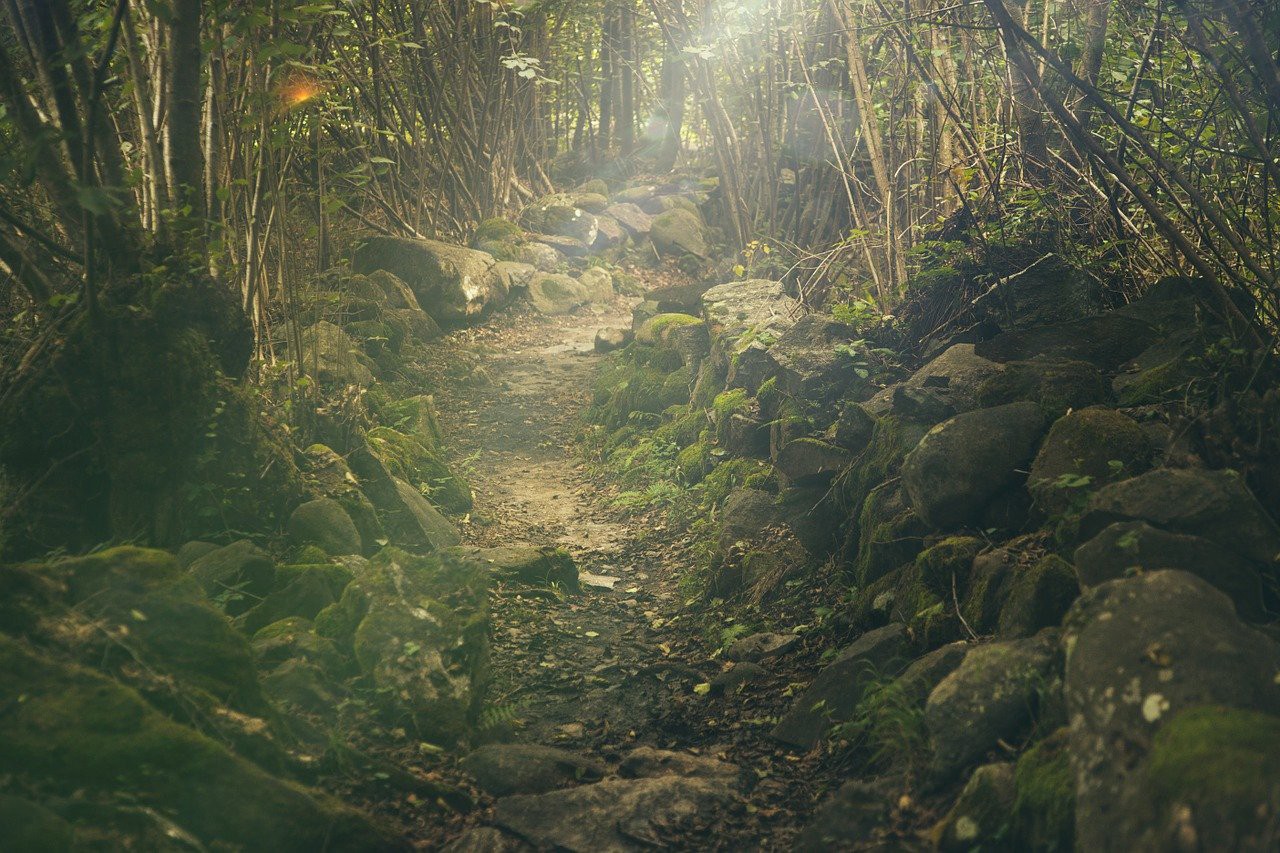
机器学习🖥 (Machine Learning 🖥)
The key feature of machine learning is that prediction dominates.
机器学习的关键特征是预测占主导地位。
Example machine learning question: What will customer churn be next month?
机器学习问题示例:什么 将 客户下个月会流失吗?
When doing machine learning, you don’t care that much about the assumptions of statistical distributions, you care about what works. You might not care which variables led to the results.
在进行机器学习时,您并不在乎统计分布的假设,而在乎什么有效。 您可能不在乎哪个变量导致了结果。
Did the homoscedacity of variance assumption of linear regression get violated? If you only care about how well the model predicts the outcome variable, then “Who cares, my model predicts well”, is a reasonable response. Machine learning folks are pragmatists.
是否违反了线性回归的方差同质性假设? 如果您仅关心模型对结果变量的预测程度,那么“谁在乎,我的模型预测的结果就很好”,这是一个合理的回答。 机器学习专家是实用主义者。
However, many machine learning problems share a fair amount of overlap with statistics.
但是,许多机器学习问题与统计数据有很多重叠之处。
- Sometimes explainability is key — for example with a bank loan decision. When you need to be able to say why someone was denied a loan the statistical requirements for interpretability matter. In that case you care about predictive validity and interpretability. 👍 有时,可解释性是关键-例如,通过银行贷款决定。 当您需要说出某人为什么被拒绝贷款时,有关可解释性的统计要求就很重要。 在那种情况下,您需要关注预测的有效性和可解释性。 👍
- Machine learning shares some algorithms with statistics, such as linear and logistic regression. 机器学习与统计共享一些算法,例如线性和逻辑回归。
- Like statisticians, machine learning researchers want the simplest model that works well. That saves time and money for training and inference. 像统计学家一样,机器学习研究人员也希望使用最简单的模型。 这样可以节省时间和金钱进行训练和推理。
There are also some fundamental differences with statistics, in addition to machine learning being more concerned with prediction.
除了机器学习更关注预测之外,统计信息还存在一些根本差异。
- Folks doing machine learning are expected to be more adept with programming than folks who strictly do statistics. 与严格进行统计的人们相比,从事机器学习的人们被认为更擅长编程。
- In machine learning you train your models and evaluate your model type and hyperparameters based on data that they haven’t seen before. This hold-out test set isn’t generally found in traditional statistics. 在机器学习中,您可以训练模型,并根据之前从未见过的数据评估模型类型和超参数。 传统统计中通常找不到这种保留测试集。
- Machine learning is designed to take advantage of bigger data and more processing power. Its sophisticated algorithms became useful when processing power became cheap and data became plentiful. 机器学习旨在利用更大的数据和更多的处理能力。 当处理能力变得廉价且数据变得丰富时,其复杂的算法变得很有用。
Let’s now drill down into deep learning — a family of machine learning algorithms where amazing breakthroughs are occurring. ☀️
现在让我们深入研究深度学习-一系列正在发生惊人突破的机器学习算法。 ☀️
深度学习🧠 (Deep learning 🧠)
Deep learning, also known as artificial neural networks, requires many layers of hidden nodes. The nodes’ weights are iteratively updated as the model is trained with the goal of optimizing a loss function.
深度学习 (也称为人工神经网络)需要多层隐藏节点。 为了优化损失函数,在训练模型时迭代地更新节点的权重。
Deep learning is eating more and more of the kinds of problems that used to be tackled with more traditional machine learning algorithms.
深度学习正在解决越来越多的传统机器学习算法曾经解决的问题。
If the relationship between the predictors variables and outcome variable is complex, deep learning is often the tool of choice. For example, image classification is one area where deep learning excels. Natural language processing (NLP) is another area where deep learning is being used for many types of artificial intelligence breakthroughs.
如果预测变量与结果变量之间的关系复杂,则深度学习通常是选择的工具。 例如, 图像分类是深度学习擅长的领域之一。 自然语言处理 (NLP)是深度学习被用于许多类型的人工智能突破的另一个领域。
Although insights, causality, and prediction are the types of tasks data scientists usually tackle, they have begun using deep learning for a fourth task: creation.
尽管洞察力,因果关系和预测是科学家通常处理的任务类型,但他们已开始将深度学习用于第四项任务: 创造。
Creative breakthroughs are happening by using generative adversarial networks (GANs). The importance of this domain for the future of humanity is large. Check out the latest GPT-3 NLP model to see what’s possible. 😲
通过使用生成对抗网络 (GAN),实现了创造性的突破。 这个领域对人类未来的重要性很大。 查看最新的GPT-3 NLP模型,以了解可能的情况。 😲
Most data scientists aren’t using deep learning for creation, although its prevalence is increasing. In the future, creation might become a core component of the data scientist’s role.
尽管深度学习的普及率在上升,但大多数数据科学家并未将其用于深度学习。 将来,创建可能会成为数据科学家角色的核心组成部分。
You’ve seen how a data scientist uses data analysis, statistics, and machine learning. Now let’s briefly look at two areas that are important to data science, but not quite part of the core.
您已经了解了数据科学家如何使用数据分析,统计信息和机器学习。 现在,让我们简要地看一下对数据科学重要的两个领域,但并不是核心领域的一部分。
数据工程 (Data engineering)
Data collection, storage, and preparation are vitally important for data science. You might have heard the statistic that 80% of a data scientist’s job is data cleaning. However, this number appears to be the result of something akin to a game of telephone. 📞 See great research digging into the sources here.
数据收集,存储和准备对于数据科学至关重要。 您可能已经听说过统计数据科学家的工作中有80%是数据清理。 但是,该数字似乎是类似于电话游戏的结果。 📞在这里可以看到大量的研究资料。
Below is a chart from from the Kaggle 2018 Developer Survey showing how respondents report spending their time. The survey had nearly 24,000 responses from around the world. Note that it includes professions beyond data scientists and a good number of students.
以下是来自Kaggle 2018开发者调查的图表,该图表显示了受访者如何报告自己的时间花费。 这项调查收到了来自世界各地的近24,000份回复。 请注意,它包括数据科学家以外的专业和大量的学生。
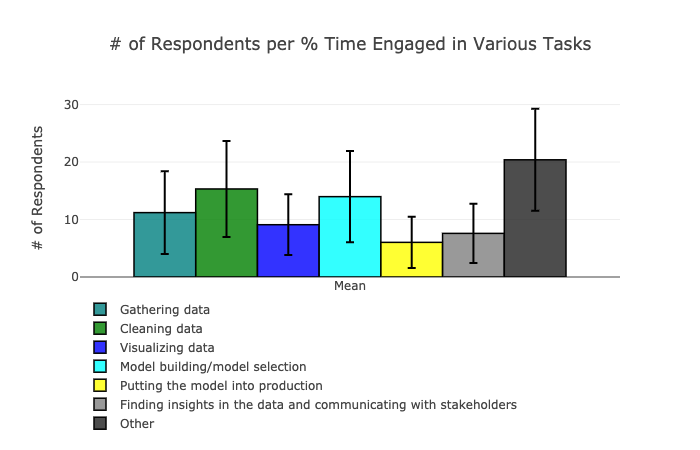
For what it’s worth, gathering and cleaning data took more than 25% of the respondents’ time, on average. That’s not insignificant, but it’s also not 80%. 😉
就其价值而言,收集和清理数据平均花费了受访者25%以上的时间。 这并不重要,但也不是80%。 😉
The last several years have seen the rise of the data engineer as a separate job title. Many organizations now have dedicated data engineers to build pipelines to injest, transform, and store data for use by other folks. However, in a small organization the data engineering and machine learning might be done by the same person.
在过去的几年中,数据工程师的崛起是一个单独的职位。 现在,许多组织都有专门的数据工程师来构建管道,以注入,转换和存储数据以供其他人使用。 但是,在小型组织中,数据工程和机器学习可能是由同一个人完成的。
All this is to say that many data scientists do some data gathering and cleaning, too — it just isn’t their core function.
这就是说,许多数据科学家也进行了一些数据收集和清理工作,这并不是他们的核心职能。
Next, let’s shift our focus to what happens to machine learning models after they leave the the data scientist. 🚀
接下来,让我们将重点转移到机器学习模型离开数据科学家之后所发生的事情。 🚀
机器学习工程 (Machine learning engineering)
Machine learning engineers ask “What can we build with these models, and how can we do it?” — Caleb Kaiser here.
机器学习工程师问道:“我们可以用这些模型构建什么,以及如何做到?” — 卡莱布·凯泽 ( Caleb Kaiser) 在这里 。
Machine learning engineers productionize a model. Machine learning engineers have to ensure models will be continually updated and that they will reliably make fast, high quality predictions at scale. See my article on in-demand tech skills for machine learning engineers here.
机器学习工程师生产模型。 机器学习工程师必须确保模型会不断更新,并能够可靠地大规模进行快速,高质量的预测。 查看有需求的技术技能机器学习的工程师我的文章在这里 。
Data engineering and machine learning engineering are important to data science, but not in its primary domain.
数据工程和机器学习工程对数据科学很重要,但在其主要领域中并不重要。
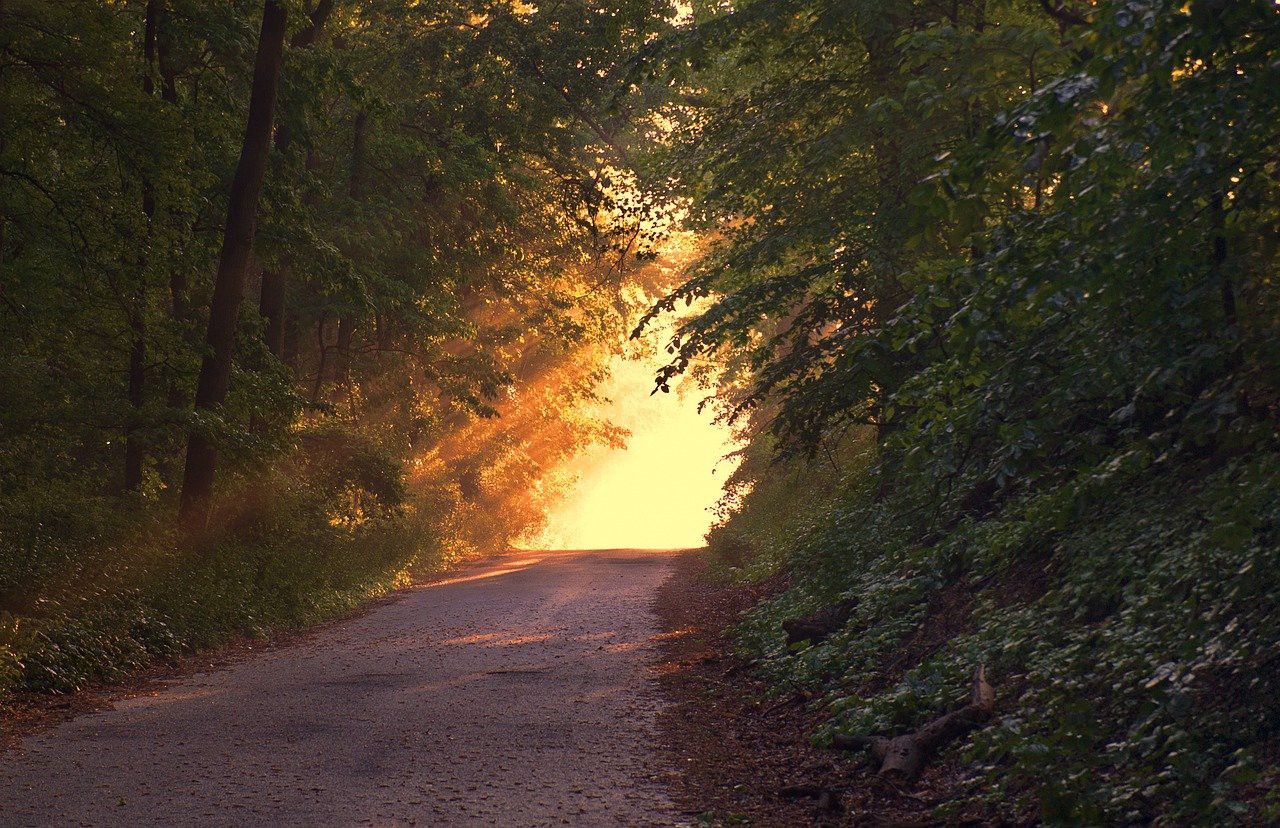
回顾 (Recap)
The major goals of a data science project are either insight, causality, or prediction.
数据科学项目的主要目标是洞察力,因果关系或预测。
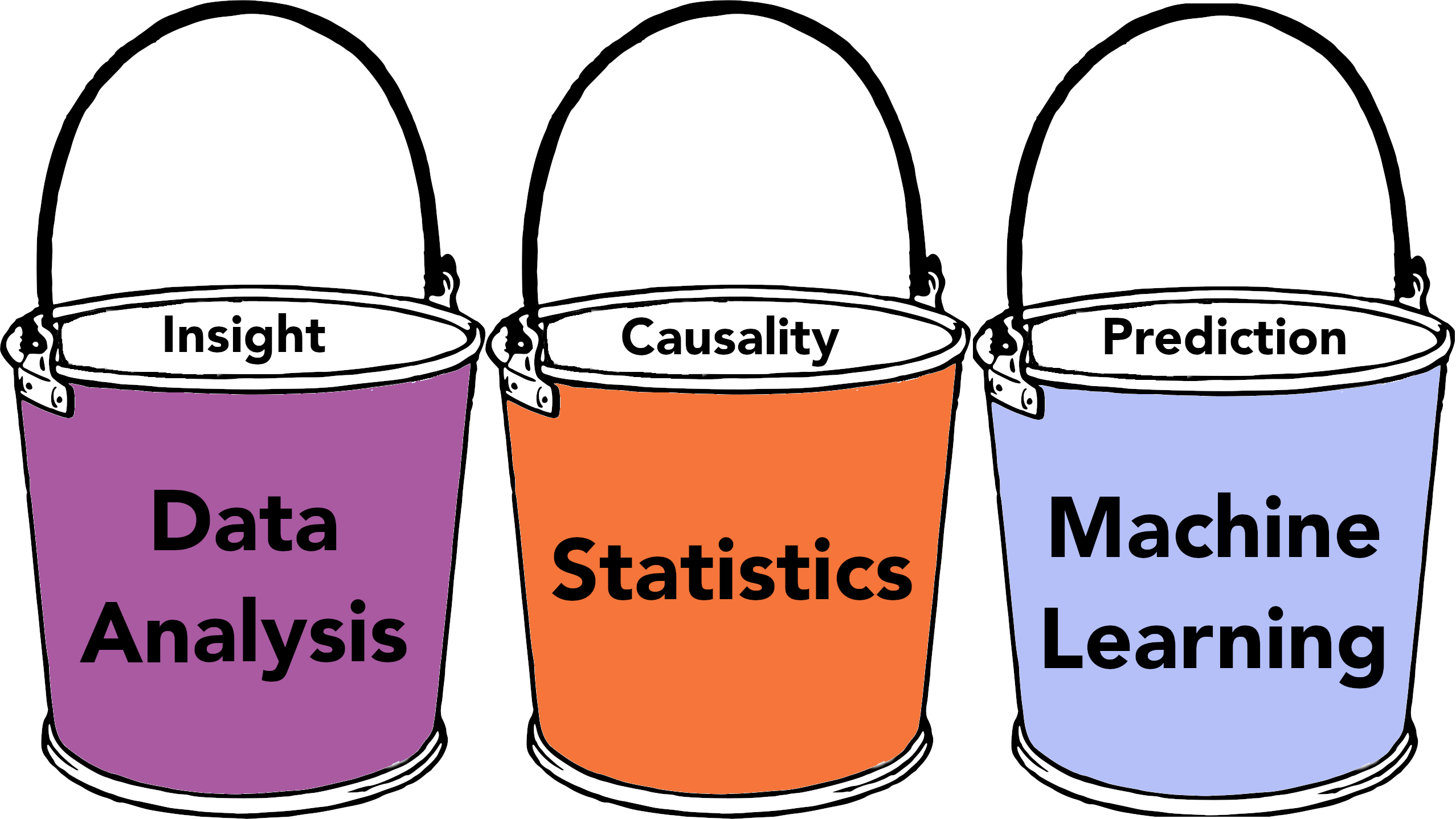
Doing data science well requires a foundation in coding, ethics, communication, math, and critical thinking. It also requires an understanding of the problem context. Although this skill set is large, data scientists are not unicorns. 🦄
做好数据科学需要具备编码,道德,沟通,数学和批判性思维的基础。 它还需要对问题上下文的理解。 尽管这种技能非常丰富,但数据科学家并不是独角兽。 🦄
Data scientists need an understanding of the analysis, stats, and machine learning processes. Understanding data engineering and machine learning engineering is valuable, too. However, you don’t need to be a pro at everything. That’s what teammates are for. 😀
数据科学家需要了解分析,统计数据和机器学习过程。 了解数据工程和机器学习工程也很有价值。 但是,您不需要在所有方面都成为专家。 那就是队友的目的。 😀
Above all, data scientists are continually learning. I suggest you focus on your own learning journey and embrace the humility that comes with feeling like there is always more to learn. It’s better than feeling overwhelmed. 😂
最重要的是,数据科学家正在不断学习。 我建议您专注于自己的学习历程,并怀着谦卑的感觉,好像总有更多的东西要学习。 总比不知所措好。 😂
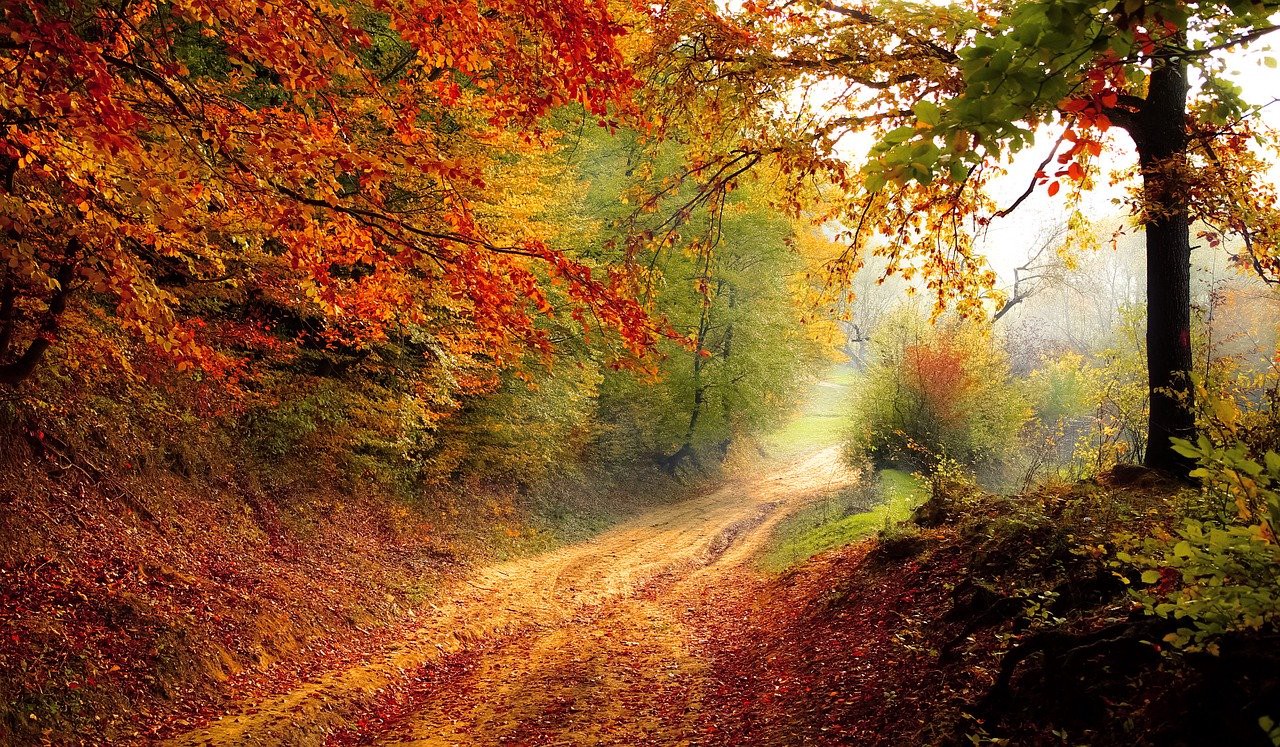
包 (Wrap)
I hope you’ve enjoyed this article and found it helpful. If you did, please share it on your favorite social media so other folks can find it, too. 😀
希望您喜欢这篇文章并发现对您有所帮助。 如果您这样做了,请在您喜欢的社交媒体上分享它,以便其他人也可以找到它。 😀
I write about Python, SQL, Docker, and other tech topics. If any of that’s of interest to you, sign up for my mailing list of awesome data science resources and read more to help you grow your skills here. 👍
我撰写有关Python , SQL , Docker和其他技术主题的文章。 如果您有任何兴趣,请注册我的超棒数据科学资源邮件列表,并在此处阅读更多内容以帮助您提高技能。 👍
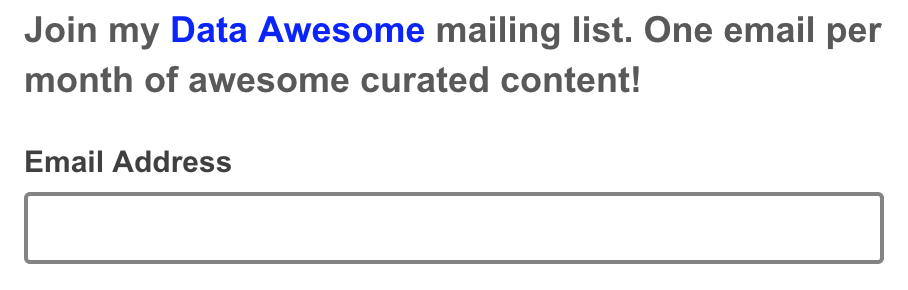
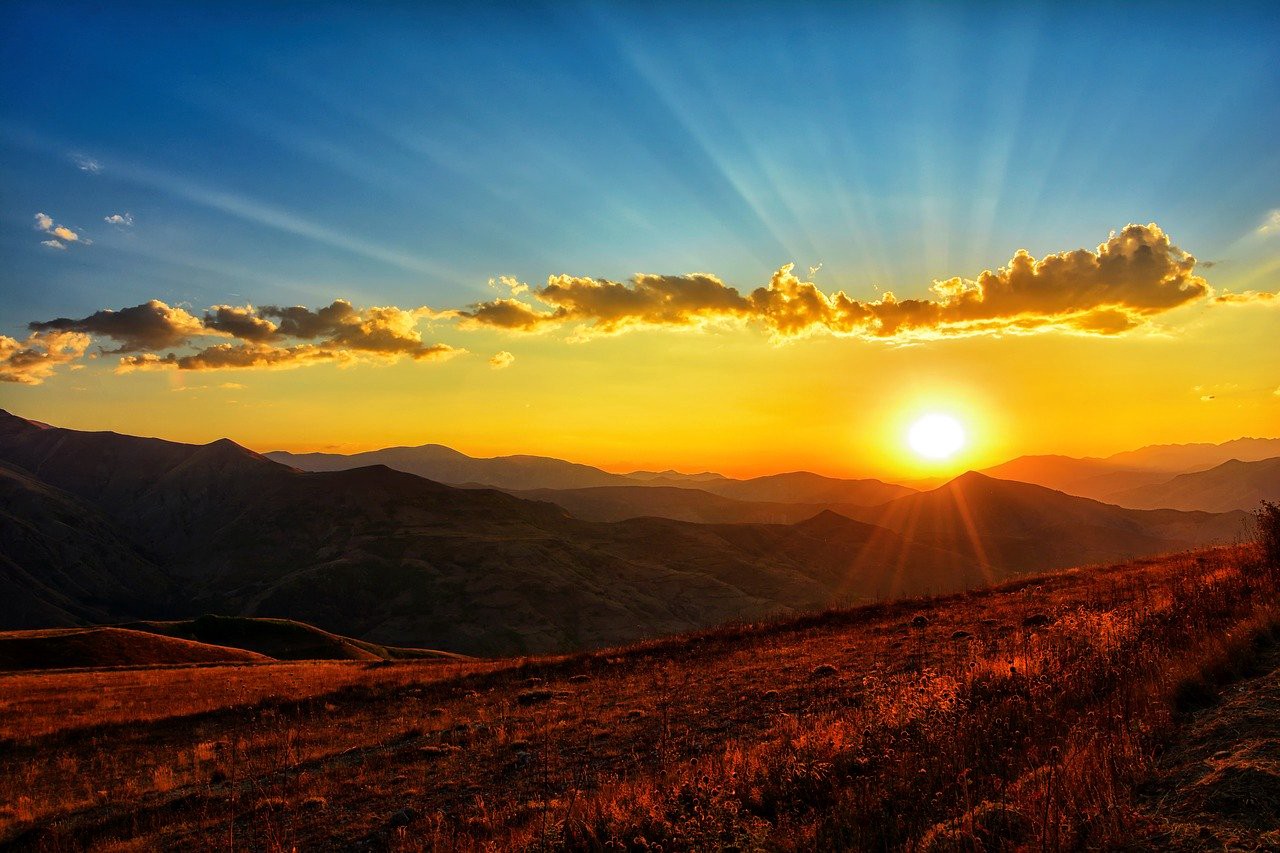
Happy data-sciencing! 😀
快乐的数据科学! 😀
翻译自: https://towardsdatascience.com/what-is-data-science-8c8fbaef1d37
数据科学与大数据是什么意思