ai芯片fpga
Major tech companies invest billions in AI chip development. Even Microsoft and Facebook are onboard with Intel FPGA in accelerating their hardware infrastructures. There are a handful of startups that are already unicorns but there are sad stories like Wave Computing that filed for bankruptcy after raising 187 million in 3 years. In this series, we will cover about 30 companies. We will focus on the technology landscape with an emphasis on identifying future advancements and trends.
大型科技公司在AI芯片开发上投资了数十亿美元。 甚至Microsoft和Facebook也加入了Intel FPGA来加速其硬件基础架构。 有少数已经成为独角兽的初创公司,但有像Wave Computing这样的悲伤故事,它们在三年内筹集了1.87亿美元后申请破产。 在本系列中,我们将涵盖约30家公司。 我们将专注于技术领域,重点是确定未来的进步和趋势。
This series will be split into 3 parts. The first article looks at the development trends for GPU, TPU, FPGA, and Startups. The first three categories represent the largest market share in AI acceleration. We will focus on what vendors have been improving. Hopefully, it tells us where they may go next. In the second half of this article, we look at novel approaches popular by startups. In particular, many of them move away from instruction flow designs to dataflow designs. This is a major paradigm shift that can change the AI chip direction completely. So let’s spend some time studying it.
该系列将分为3部分。 第一篇文章介绍了GPU,TPU,FPGA和Startups的发展趋势。 前三个类别代表了AI加速的最大市场份额。 我们将重点关注供应商正在改进的方面。 希望它告诉我们他们下一步可能去哪里。 在本文的下半部分,我们将介绍初创公司流行的新颖方法。 特别是,它们中的许多都从指令流设计转移到了数据流设计。 这是一个重大的范式转换,可以完全改变AI芯片的方向。 因此,让我们花一些时间研究它。
英伟达GPU (Nvidia GPU)
GV100 (Volta architecture) is released in late 2017 while GA100 (Ampere architecture) is released in 2020.
GV100(Volta架构)于2017年末发布,而GA100(Ampere架构)于2020年发布。
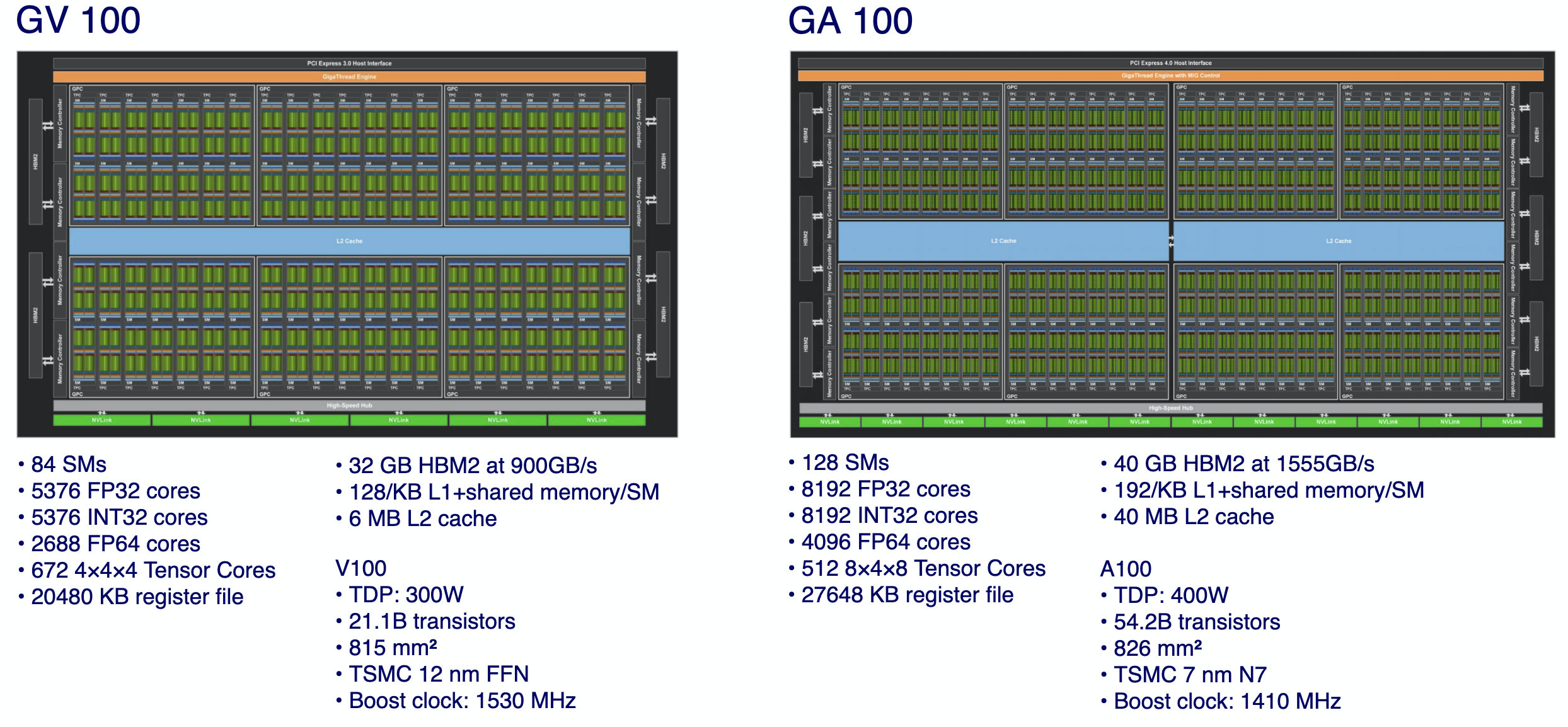
GA 100 GPU uses the TSMC 7 nm fabrication instead of the 12 nm process in GV 100. While their die sizes are about the same, the number of Streaming Multiprocessors (SMs) in GA100 is increased by 50% to 128 and the number of FP32 cores is increased from 5376 to 8192. The new Gen3 Tensor Cores perform 8×4 by 4×8 matrix multiplication instead of 4×4 by 4×4.(Details)
GA 100 GPU使用台积电7纳米制造Craft.io代替了GV 100中的12纳米Craft.io。虽然它们的芯片尺寸大致相同,但GA100中的流式多处理器(SM)的数量增加了50%,达到128个,FP32的数量增加了核心从5376增加到8192。新的Gen3张量核心执行4×8矩阵乘法的8×4而不是4×4×4×4的矩阵。( 详细信息 )
So far, these trends are pretty expected in many AI chips — more cores with better matrix multiplication units targeted for deep learning (DL). But there are some noticeable design enhancements.
到目前为止,这些趋势在许多AI芯片中都是可预见的-更多的内核具有针对深度学习(DL)的更好的矩阵乘法单元。 但是,在设计上有一些明显的增强。
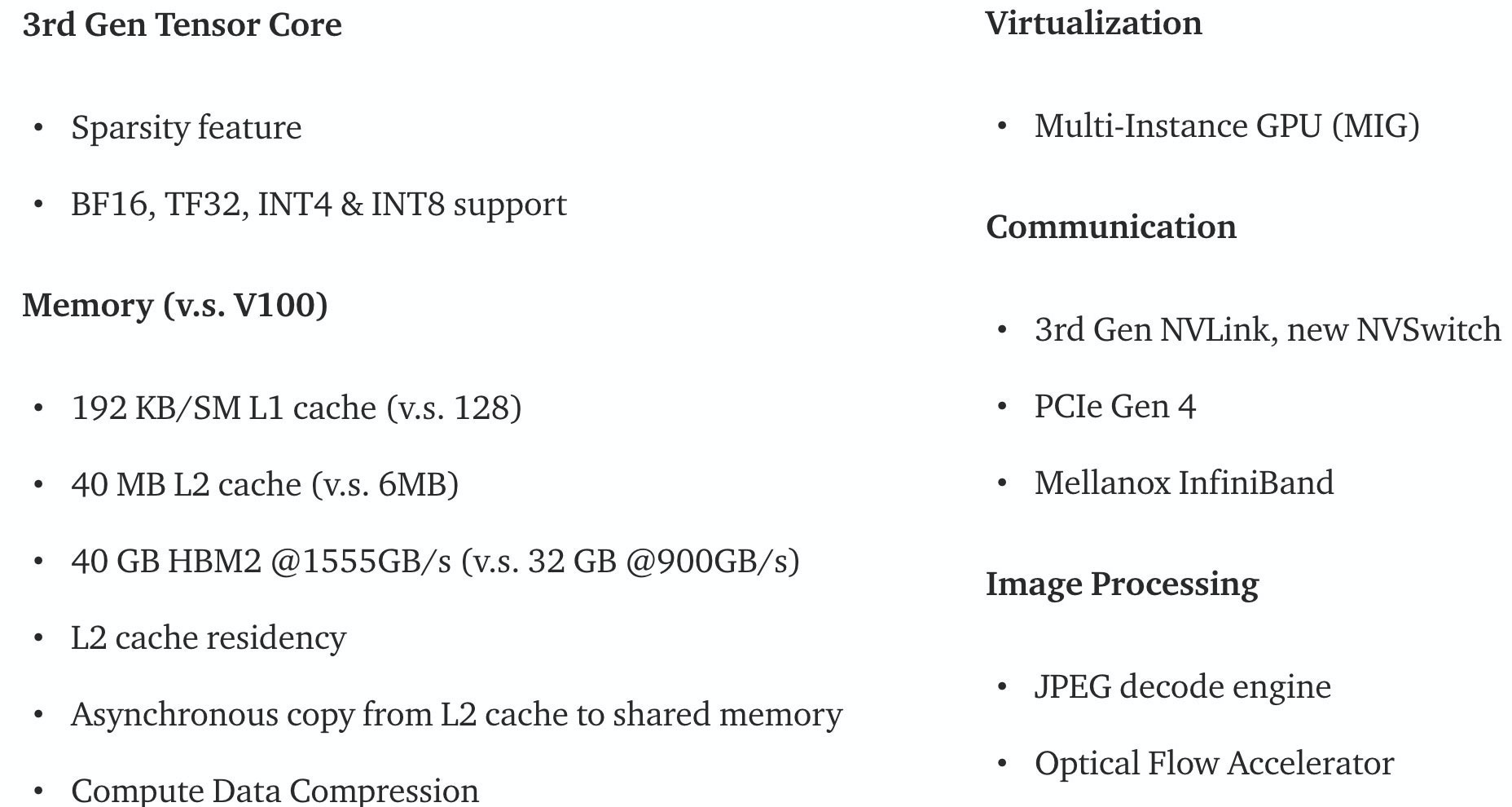
First, it addresses potential memory bottlenecks. The L2 level cache increases un-proportionally from 6MB to 40MB. The L1 cache per SM increases by 50%. In addition, developers have more control over what data to be cached on L2 and the flexibility of by-passing L1 in copying data directly to the SM shared memory.
首先,它解决了潜在的内存瓶颈。 L2级别的缓存从6MB不成比例地增加到40MB。 每个SM的L1缓存增加了50%。 此外,开发人员可以更好地控制要在L2上缓存哪些数据,以及在将数据直接复制到SM共享内存时绕过L1的灵活性。
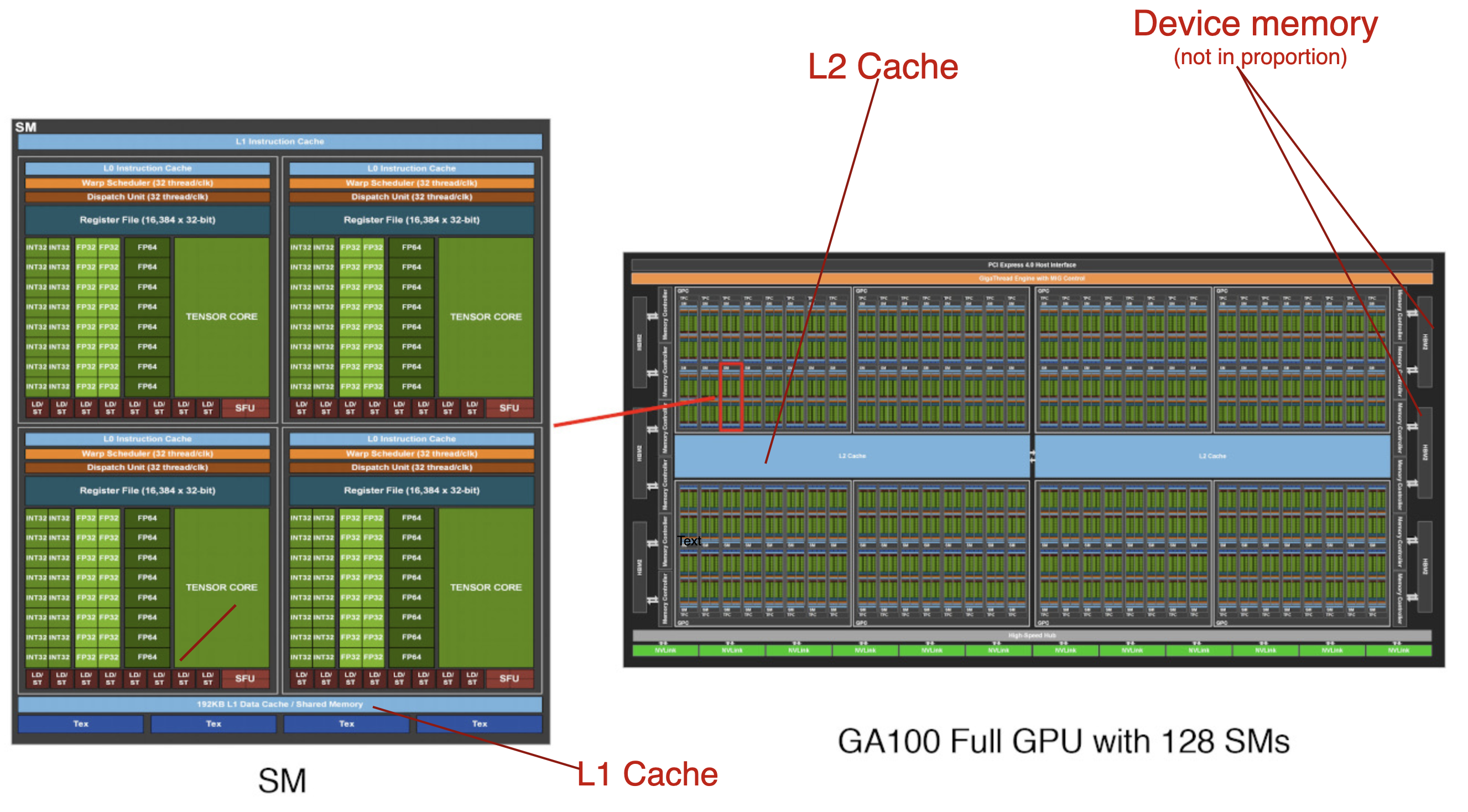
Second, DNNs (deep neural networks) weight sparsity is heavily exploited. After data compression, the data volume transmitted between the off-chip memories and the on-chip cache decrease significantly by 2x to 4x. With this sparsity, the Tensor Core is redesigned for even faster operations.
其次, DNN (深度神经网络)权重稀疏性被大量利用。 数据压缩后,在片外存储器和片上高速缓存之间传输的数据量显着减少了2倍至4倍。 由于这种稀疏性,Tensor Core被重新设计以实现更快的操作。
Third, more data type (BF16, INT4, TF32) is supported. In DL, the general trend is 16-bit matrix arithmetic with the value range equals FP32 for training and hopefully even 8 bit for inference. As shown below, some improvements are in one order of magnitude for lower precision arithmetics.
第三,支持更多数据类型(BF16,INT4,TF32)。 在DL中,一般趋势是16位矩阵算术,其值范围等于FP32进行训练,希望甚至达到8位进行推理。 如下所示,对于较低精度的算术,一些改进在一个数量级上。
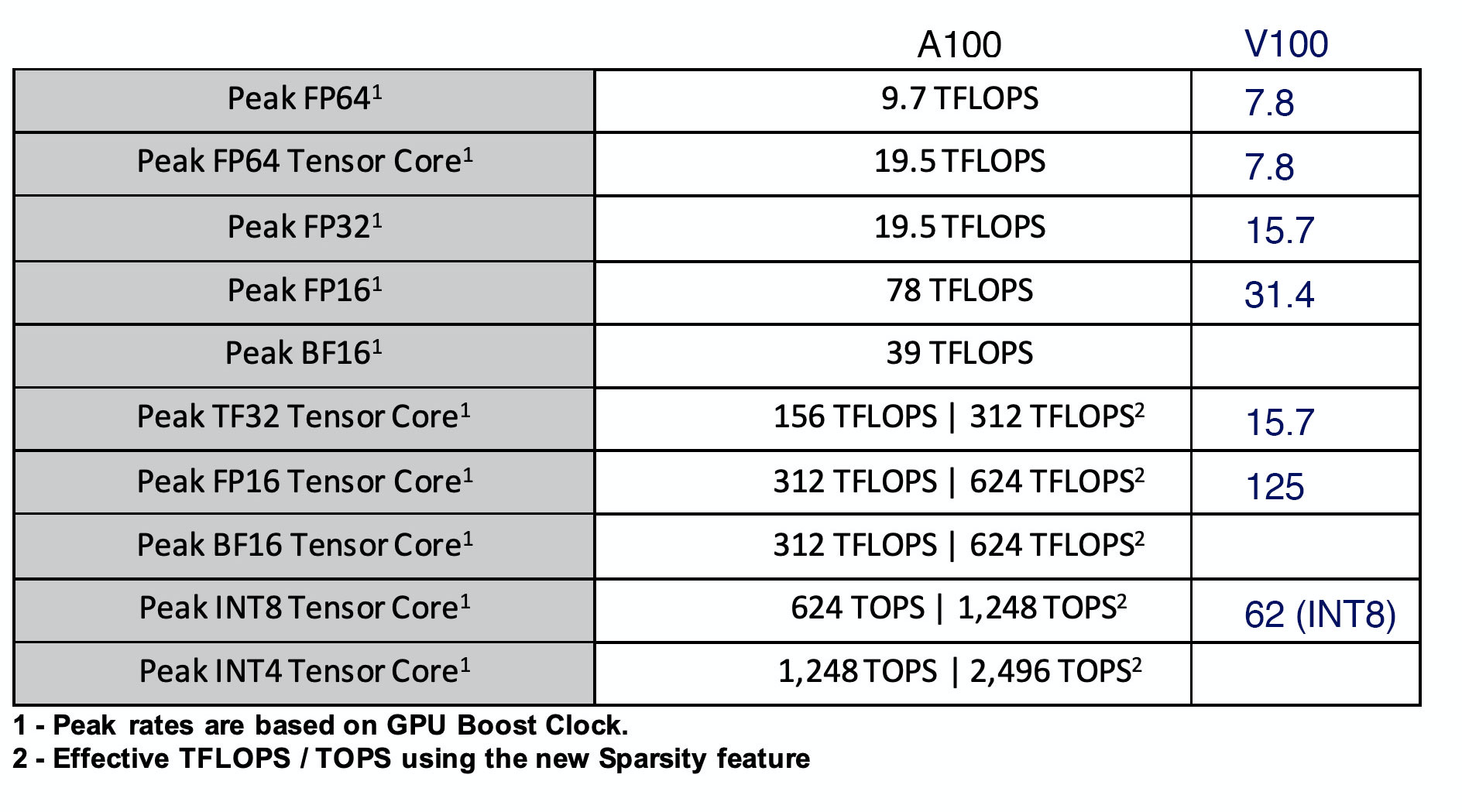
Fourth, faster inter-GPU communications to handle model training that cannot fit into one GPU.
第四,更快的GPU间通信以处理无法适合一个GPU的模型训练。
Fifth, new features are created, like video encoding/decoding, for end-to-end solutions.
第五,为端到端解决方案创建了新功能,例如视频编码/解码。
These trends are very important as we look at many AI companies. These are the same problems that chip designers are solving but just not necessarily the same solution. Sparsity and memory bandwidth will remain important for future development. But besides hardware support in weight sparsity, data sparsity, and weight updates will be heavily studied (more on this in part 2).
当我们关注许多AI公司时,这些趋势非常重要 。 这些是芯片设计人员正在解决的相同问题,但不一定是相同的解决方案。 稀疏性和内存带宽对于将来的开发将仍然很重要。 但是,除了对重量稀疏性的硬件支持之外,还将对数据稀疏性和重量更新进行大量研究(第2部分将对此进行详细介绍)。
In addition, NVidia is the most mature AI chips provider. As dealing with real customer needs, GPU virtualization (MIG feature turns a single GPU into multiple virtual GPUs) is added for better utilization in cloud-based inferencing.
此外,NVidia是最成熟的AI芯片提供商。 在满足实际客户需求时,添加了GPU虚拟化(MIG功能将单个GPU变成多个虚拟GPU),以更好地利用基于云的推理。
For reference, these are the magnitude of performance improvements made in A100 GPU over V100 for the specific enhancements.
作为参考,这些是A100 GPU在V100上针对特定增强所做的性能改进幅度。

Google TPU (Google TPU)
A general GPU provides the programmability that many projects may consider as “fat”. So it comes back to the question of whether this hurts or not. For Google, many servers are allocated to solving specific problems. Small improvements can save millions. Specifically, GPU consumes more energy than ASIC (Application-specific integrated circuit) and has higher latency.
通用GPU提供了许多项目可能认为是“胖”的可编程性。 因此,这又回到了是否有伤害的问题。 对于Google,分配了许多服务器来解决特定问题。 小小的改进可以节省数百万美元。 具体而言,GPU比ASIC(专用集成电路)消耗更多的能量,并且具有更高的延迟。
Chip companies can design ASIC chips for maximum efficiency. But development costs are expensive and not adaptable to changes. Since the breakout success of AI in 2012, Google has gathered abundant experience in narrow down these design requirements. In fact, it concludes that Google TPU just needs high throughput for matrix multiplication, activation, normalization, and pooling.
芯片公司可以设计ASIC芯片以实现最高效率。 但是开发成本昂贵,并且无法适应变化。 自2012年AI取得突破性成功以来,Google在缩小这些设计要求方面积累了丰富的经验。 实际上,得出的结论是,Google TPU仅需要高吞吐量即可进行矩阵乘法,激活,归一化和池化。
TPU (details) takes away many control logic, in particular, dealing with instruction fetching and scheduling. It shifts this work to the CPU host. TPU simply acts as a coprocessor and provides vertical instructions.
TPU( 详细信息 )取消了许多控制逻辑,尤其是处理指令提取和调度的逻辑。 它将工作转移到CPU主机。 TPU只是充当协处理器并提供垂直指令。
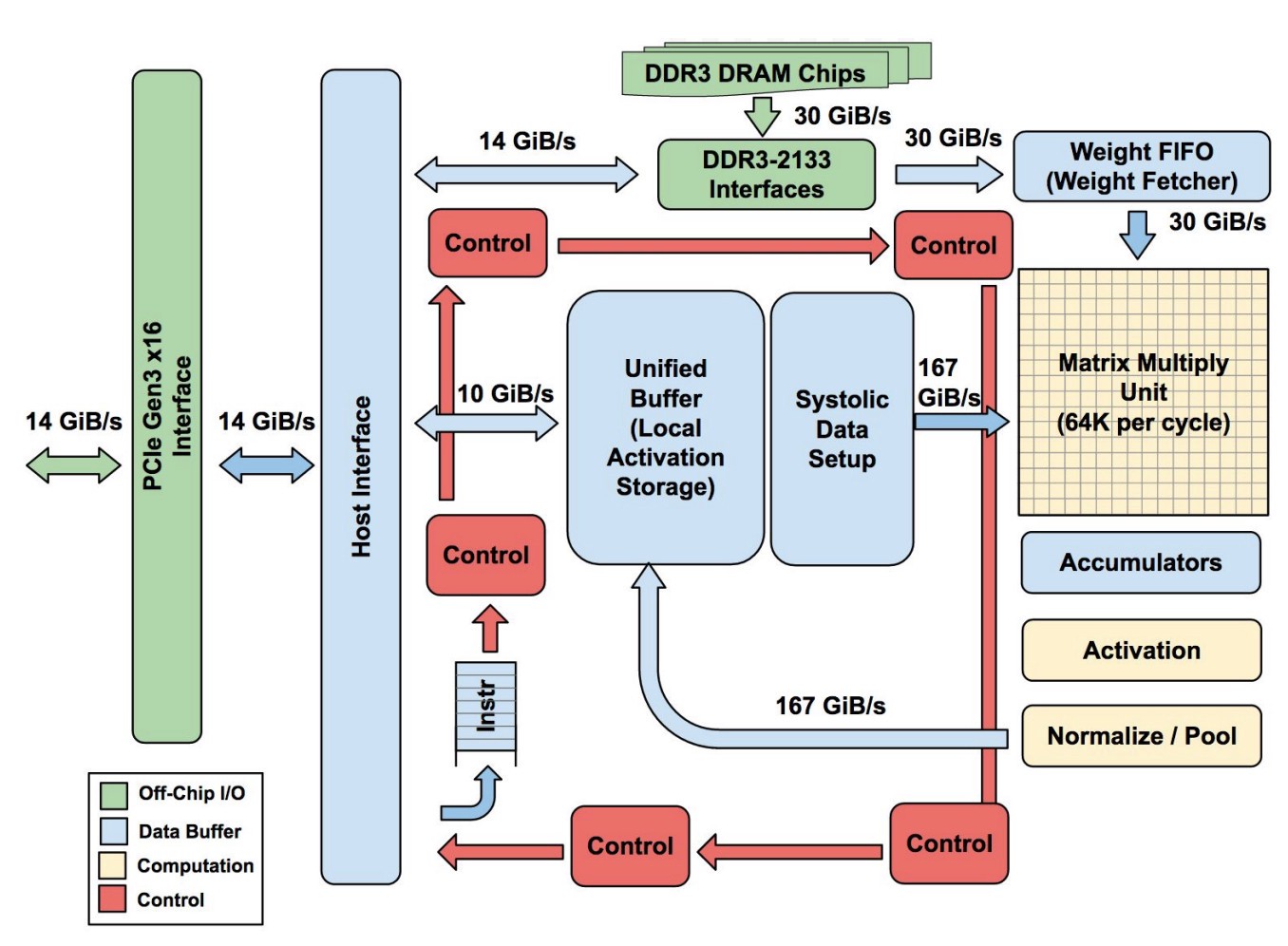
This leads to a very important design trend in general.
通常,这导致非常重要的设计趋势。
Works, like instruction scheduling, optimization and resource assignments is shifted to the runtime library in CPU and the compiler.
指令调度,优化和资源分配等工作已转移到CPU和编译器中的运行时库中。
As shown in the diagram below, TPU v3 doubles the number of cores than v2, and TFLOPs is a little bit more than double. The coming TPU v4 will double in performance again (2.7x). The throughput of its matrix multiplication units has been more than double. Not much else is known yet but it will not be surprising if the number of cores is double or the matrix multiplication size has increased. But this has increased the memory bandwidth requirement significantly which is solved with unspecified advances in interconnect technology.
如下图所示,TPU v3的内核数量是v2的两倍,而TFLOP则是两倍多。 即将面世的TPU v4的性能将再次翻倍(2.7倍)。 它的矩阵乘法单元的吞吐量已增加了一倍以上。 到目前为止,还没有多少其他信息,但是如果核心数增加一倍或矩阵乘法大小增加,也就不足为奇了。 但这极大地增加了内存带宽需求,而互连技术的未指定进步解决了这一需求。

Google has the monetary resource, powers, and wills to build a vertical solution. Their own datacenters and cloud services already provide a mature market for TPUs. Keep things focus, keep it simple, and optimize what matters seem to be the key focus for TPU.
Google拥有金钱资源,能力和意愿来建立纵向解决方案。 他们自己的数据中心和云服务已经为TPU提供了成熟的市场。 保持重点,使其简单,并优化问题,这似乎是TPU的重点。
英特尔FPGA (Intel FPGA)
However, the rigidity of ASIC bothers companies like Facebook or Microsoft. For better flexibility in designs and options, they are looking into solutions like FPGA. The hardware design for GPU and TPU cannot be changed. But for decades, FPGA has allowed hardware designers for reconfigurable ASIC design that can be reprogrammed in ∼20ms.
但是,ASIC的僵化困扰着Facebook或Microsoft等公司。 为了使设计和选项具有更好的灵活性,他们正在研究诸如FPGA之类的解决方案。 GPU和TPU的硬件设计无法更改。 但是几十年来,FPGA允许硬件设计人员进行可重配置的ASIC设计,该设计可在约20ms内重新编程。
FPGA Overview (Optional)
FPGA概述(可选)
Software engineers are less familiar with FPGA. So let’s have a simple overview. FPGA contains an array of blocks for logic and arithmetic functions. FPGA has put many thoughts on its reconfigurability in creating custom functions in each block. In addition, these blocks can be connected through programmable interconnect to build custom features with specific concurrency, latency, I/O throughput, and power consumption.
软件工程师对FPGA不太熟悉。 因此,让我们进行简单的概述。 FPGA包含一系列用于逻辑和算术功能的模块。 FPGA在每个块中创建自定义函数时已经考虑了其可重新配置性。 此外,这些模块可以通过可编程互连进行连接,以构建具有特定并发性,延迟,I / O吞吐量和功耗的自定义功能。

FPGA also provides blocks, like those for local memory and IP blocks (a.k.a. reusable hardware cell design