from sklearn import datasets # 用于调用sklearn自带的数据集
from sklearn.model_selection import KFold
import matplotlib.pyplot as plt
%matplotlib inline
import numpy as np
import pandas as pd
datasets.load_wine
<function sklearn.datasets.base.load_wine(return_X_y=False)>
wine_data=datasets.load_wine()
print(wine_data.feature_names)
['alcohol', 'malic_acid', 'ash', 'alcalinity_of_ash', 'magnesium', 'total_phenols', 'flavanoids', 'nonflavanoid_phenols', 'proanthocyanins', 'color_intensity', 'hue', 'od280/od315_of_diluted_wines', 'proline']
data_input = wine_data.data # 输入输出数据
data_output = wine_data.target
data_input
array([[1.423e+01, 1.710e+00, 2.430e+00, ..., 1.040e+00, 3.920e+00,
1.065e+03],
[1.320e+01, 1.780e+00, 2.140e+00, ..., 1.050e+00, 3.400e+00,
1.050e+03],
[1.316e+01, 2.360e+00, 2.670e+00, ..., 1.030e+00, 3.170e+00,
1.185e+03],
...,
[1.327e+01, 4.280e+00, 2.260e+00, ..., 5.900e-01, 1.560e+00,
8.350e+02],
[1.317e+01, 2.590e+00, 2.370e+00, ..., 6.000e-01, 1.620e+00,
8.400e+02],
[1.413e+01, 4.100e+00, 2.740e+00, ..., 6.100e-01, 1.600e+00,
5.600e+02]])
data_output
array([0, 0, 0, 0, 0, 0, 0, 0, 0, 0, 0, 0, 0, 0, 0, 0, 0, 0, 0, 0, 0, 0,
0, 0, 0, 0, 0, 0, 0, 0, 0, 0, 0, 0, 0, 0, 0, 0, 0, 0, 0, 0, 0, 0,
0, 0, 0, 0, 0, 0, 0, 0, 0, 0, 0, 0, 0, 0, 0, 1, 1, 1, 1, 1, 1, 1,
1, 1, 1, 1, 1, 1, 1, 1, 1, 1, 1, 1, 1, 1, 1, 1, 1, 1, 1, 1, 1, 1,
1, 1, 1, 1, 1, 1, 1, 1, 1, 1, 1, 1, 1, 1, 1, 1, 1, 1, 1, 1, 1, 1,
1, 1, 1, 1, 1, 1, 1, 1, 1, 1, 1, 1, 1, 1, 1, 1, 1, 1, 1, 1, 2, 2,
2, 2, 2, 2, 2, 2, 2, 2, 2, 2, 2, 2, 2, 2, 2, 2, 2, 2, 2, 2, 2, 2,
2, 2, 2, 2, 2, 2, 2, 2, 2, 2, 2, 2, 2, 2, 2, 2, 2, 2, 2, 2, 2, 2,
2, 2])
from sklearn.linear_model import LogisticRegression # 逻辑回归模型
from sklearn.metrics import f1_score,log_loss,classification_report
kf=KFold(4,shuffle=True)
kf.get_n_splits(data_input)
lr=LogisticRegression()
for train_index,test_index in kf.split(data_input,data_output):
print(train_index,test_index)
[ 0 1 2 3 6 7 8 9 10 11 13 15 18 19 20 22 23 24
26 27 28 29 30 31 32 33 34 37 39 41 43 44 46 49 50 51
53 54 55 56 57 58 59 60 61 62 63 64 65 66 67 68 70 71
73 74 77 79 80 81 82 84 85 86 87 88 89 90 91 92 94 95
98 100 101 105 106 107 108 110 111 112 113 115 116 117 118 119 120 121
123 125 127 128 129 130 131 132 133 135 136 140 141 142 143 145 146 147
148 149 151 152 153 154 157 158 159 160 161 163 164 165 166 167 168 169
170 171 172 173 174 175 177] [ 4 5 12 14 16 17 21 25 35 36 38 40 42 45 47 48 52 69
72 75 76 78 83 93 96 97 99 102 103 104 109 114 122 124 126 134
137 138 139 144 150 155 156 162 176]
[ 0 1 4 5 6 8 9 10 12 13 14 16 17 19 21 23 24 25
26 28 29 30 31 32 34 35 36 38 39 40 42 45 46 47 48 49
50 52 56 57 58 59 60 63 64 65 66 67 68 69 70 71 72 74
75 76 77 78 79 80 81 82 83 85 86 87 88 89 90 92 93 94
95 96 97 98 99 100 102 103 104 105 106 107 108 109 111 112 113 114
115 116 117 121 122 124 125 126 128 130 131 132 133 134 135 136 137 138
139 142 143 144 147 148 150 152 154 155 156 157 158 159 160 161 162 164
166 167 170 172 174 176 177] [ 2 3 7 11 15 18 20 22 27 33 37 41 43 44 51 53 54 55
61 62 73 84 91 101 110 118 119 120 123 127 129 140 141 145 146 149
151 153 163 165 168 169 171 173 175]
[ 0 1 2 3 4 5 6 7 8 9 11 12 14 15 16 17 18 20
21 22 23 25 27 30 31 33 35 36 37 38 39 40 41 42 43 44
45 47 48 49 50 51 52 53 54 55 56 57 58 59 60 61 62 67
68 69 70 71 72 73 75 76 78 80 81 83 84 85 89 91 93 94
95 96 97 99 100 101 102 103 104 109 110 114 116 118 119 120 121 122
123 124 125 126 127 129 130 131 134 135 136 137 138 139 140 141 143 144
145 146 147 149 150 151 152 153 155 156 158 159 161 162 163 165 167 168
169 170 171 173 174 175 176 177] [ 10 13 19 24 26 28 29 32 34 46 63 64 65 66 74 77 79 82
86 87 88 90 92 98 105 106 107 108 111 112 113 115 117 128 132 133
142 148 154 157 160 164 166 172]
[ 2 3 4 5 7 10 11 12 13 14 15 16 17 18 19 20 21 22
24 25 26 27 28 29 32 33 34 35 36 37 38 40 41 42 43 44
45 46 47 48 51 52 53 54 55 61 62 63 64 65 66 69 72 73
74 75 76 77 78 79 82 83 84 86 87 88 90 91 92 93 96 97
98 99 101 102 103 104 105 106 107 108 109 110 111 112 113 114 115 117
118 119 120 122 123 124 126 127 128 129 132 133 134 137 138 139 140 141
142 144 145 146 148 149 150 151 153 154 155 156 157 160 162 163 164 165
166 168 169 171 172 173 175 176] [ 0 1 6 8 9 23 30 31 39 49 50 56 57 58 59 60 67 68
70 71 80 81 85 89 94 95 100 116 121 125 130 131 135 136 143 147
152 158 159 161 167 170 174 177]
for train_index,test_index in kf.split(data_input,data_output):
#print(train_index,test_index)
lr.fit(data_input[train_index],data_output[train_index])
y_pre_lr=lr.predict(data_input[test_index])
y=data_output[test_index]
print(f1_score(y,y_pre_lr,average=None))
[1. 1. 1.]
[0.96296296 0.97297297 1. ]
[1. 0.94444444 0.90909091]
[0.9375 0.88235294 0.90909091]
/anaconda3/envs/tensorflow/lib/python3.7/site-packages/sklearn/linear_model/logistic.py:433: FutureWarning: Default solver will be changed to 'lbfgs' in 0.22. Specify a solver to silence this warning.
FutureWarning)
/anaconda3/envs/tensorflow/lib/python3.7/site-packages/sklearn/linear_model/logistic.py:460: FutureWarning: Default multi_class will be changed to 'auto' in 0.22. Specify the multi_class option to silence this warning.
"this warning.", FutureWarning)
/anaconda3/envs/tensorflow/lib/python3.7/site-packages/sklearn/linear_model/logistic.py:433: FutureWarning: Default solver will be changed to 'lbfgs' in 0.22. Specify a solver to silence this warning.
FutureWarning)
/anaconda3/envs/tensorflow/lib/python3.7/site-packages/sklearn/linear_model/logistic.py:460: FutureWarning: Default multi_class will be changed to 'auto' in 0.22. Specify the multi_class option to silence this warning.
"this warning.", FutureWarning)
/anaconda3/envs/tensorflow/lib/python3.7/site-packages/sklearn/linear_model/logistic.py:433: FutureWarning: Default solver will be changed to 'lbfgs' in 0.22. Specify a solver to silence this warning.
FutureWarning)
/anaconda3/envs/tensorflow/lib/python3.7/site-packages/sklearn/linear_model/logistic.py:460: FutureWarning: Default multi_class will be changed to 'auto' in 0.22. Specify the multi_class option to silence this warning.
"this warning.", FutureWarning)
/anaconda3/envs/tensorflow/lib/python3.7/site-packages/sklearn/linear_model/logistic.py:433: FutureWarning: Default solver will be changed to 'lbfgs' in 0.22. Specify a solver to silence this warning.
FutureWarning)
/anaconda3/envs/tensorflow/lib/python3.7/site-packages/sklearn/line
python十折交叉验证_机器学习】交叉验证、正则化实例Python代码实现
最新推荐文章于 2022-05-05 20:12:45 发布
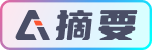