Note on nominal classes: If you're interested in the distribution over all the classes, use the method distributionForInstance(Instance). This method returns a double array with the probability for each class. The returned double value from classifyInstance (or the index in the array returned by distributionForInstance) is just the index for the string values in the attribute. I.e., if you want the string representation for the above returned class label clsLabel, then you can, e.g., print it like this: System.out.println(clsLabel + " -> " + unlabeled.classAttribute().value((int) clsLabel)); Building a Clusterer Batch 和分类器用buildClassifier(Instances) 建立类似,聚类(器)用buildClusterer(Instances) 建立。下列代码片段(code snippet )实现了EM聚类算法,最大迭代次数为100 import weka.clusterers.EM; ... String[] options = new String[2]; options[0] = "-I"; // max. iterations options[1] = "100"; EM clusterer = new EM(); // new instance of clusterer clusterer.setOptions(options); // set the options clusterer.buildClusterer(data); // build the clusterer Building a Clusterer Incremental 实现了weka.clusterers.UpdateableClusterer接口的聚类器可以进行增量聚类。 训练增量式聚类器的过程: Call buildClusterer(Instances) with the structure of the dataset (may or may not contain any actual data rows). Subsequently call the updateClusterer(Instance) method to feed the clusterer one by one. Call updateFinished() after all Instance objects have been processed, for the clusterer to perform additional computations. 示例 // load data ArffLoader loader = new ArffLoader(); loader.setFile(new File("/some/where/data.arff")); Instances structure = loader.getStructure(); ? // train Cobweb Cobweb cw = new Cobweb(); cw.buildClusterer(structure); Instance current; while ((current = loader.getNextInstance(structure)) != null) cw.updateClusterer(current); cw.updateFinished(); Evaluating a clusterer 可用ClusterEvaluation 类评价一个聚类器。以下代码片段输出了算法发现的类的个数。 import weka.clusterers.ClusterEvaluation; import weka.clusterers.Clusterer; ... ClusterEvaluati
weka java 教程_WEKA教程完整版(新)详细分解.ppt
最新推荐文章于 2021-03-19 10:05:49 发布
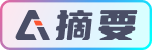