标题中的英文首字母大写比较规范,但在python实际使用中均为小写。
5.Pandas的数据运算和算术对齐
5.1 Series相加
from pandas import Series
s1 = Series([7.3, -2.5, 3.4, 1.5], index=['a', 'c', 'd', 'e'])
s2 = Series([-2.1, 3.6, -1.5, 4, 3.1], index=['a', 'c', 'e', 'f', 'g'])
s1 + s2
上面一段代码的运行结果如下图所示:
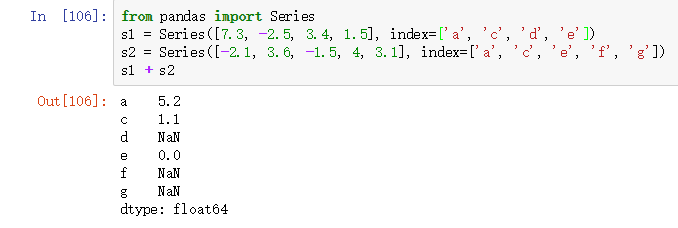
5.2 DataFrame相加
对于DataFrame,对齐会同时发生在行和列上,两个DataFrame对象相加后,其索引和列会取并集,缺省值用NaN。
from pandas import DataFrame
import numpy as np
kwargs = dict(
columns = list('bcd'),
index = ['北京','上海','广州']
)
df1 = DataFrame(np.arange(9).reshape(3,3),**kwargs)
kwargs = dict(
columns = list('bde'),
index = ['北京','上海','重庆','天津']
)
df2 = DataFrame(np.arange(12).reshape(4,3),**kwargs)
df1 + df2
上面一段代码的运行结果如下图所示:
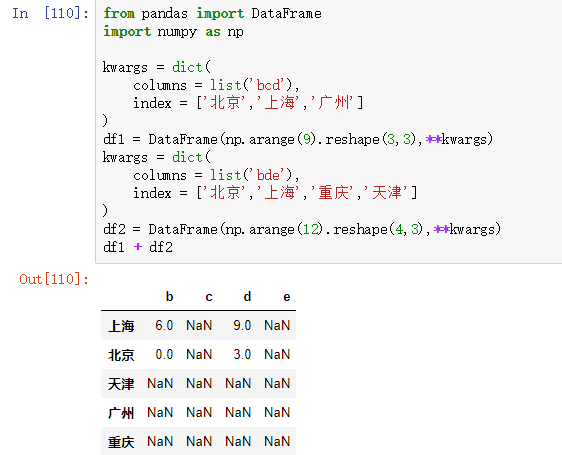
设置缺省时填充值
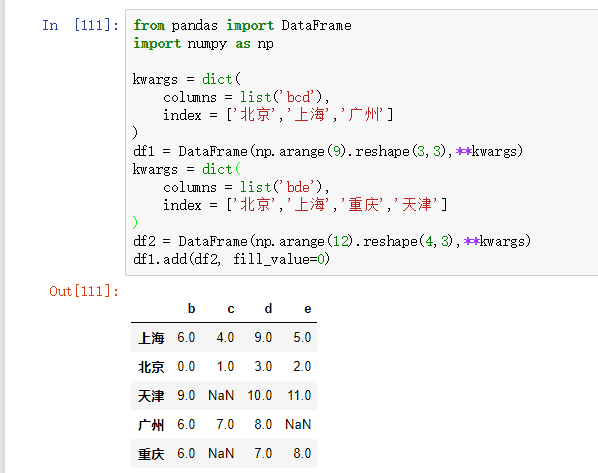
5.3 DataFrame和Series之间的运算
默认情况下,DataFrame和Series之间的算术运算会将Series的索引匹配到DataFram的列,然后沿着行一直向下广播,如下图所示:
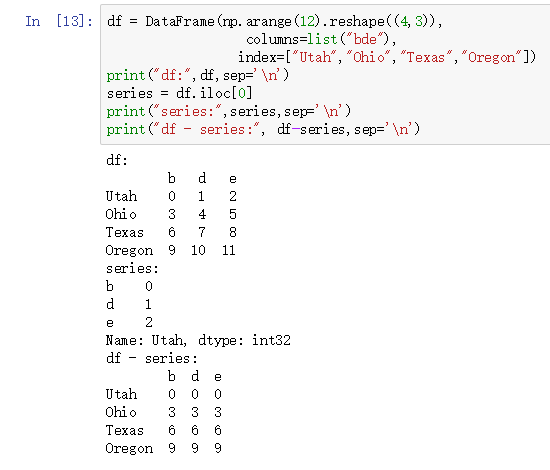
.读者可以复制下面代码运行,然后查看结果是否相同:
from pandas import Series,DataFrame
import numpy as np
df = DataFrame(np.arange(12).reshape((4,3)),
columns=list("bde"),
index=["Utah","Ohio","Texas","Oregon"])
print("df:",df,sep='\n')
series = df.iloc[0]
print("series:",series,sep='\n')
print("df - series:", df-series,sep='\n')
5.4 Pandas中的函数应用和映射
5.4.1 Numpy中的函数可以用于操作pandas对象
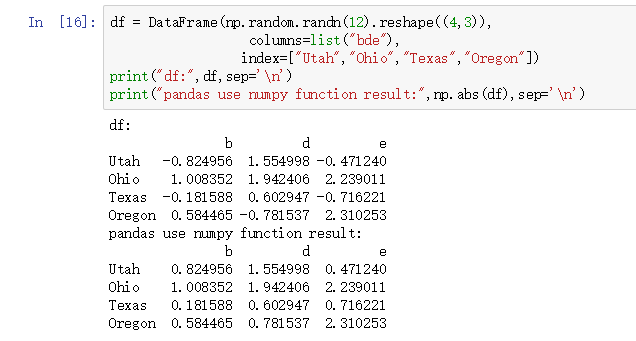
.读者可以复制下面代码运行,然后查看结果是否相同:
from pandas import Series,DataFrame
import numpy as np
df = DataFrame(np.random.randn(12).reshape((4,3)),
columns=list("bde"),
index=["Utah","Ohio","Texas","Oregon"])
print("df:",df,sep='\n')
print("pandas use numpy function result:",np.abs(df),sep='\n')
5.4.2 DataFrame对象的apply方法
需要2个参数:第1个参数的数据类型为函数对象,函数的返回值的数据类型为Series;第2个参数axis=1会得出行的结果,如下图所示,结果有4行。apply方法是对DataFram中的每一行或者每一列进行映射。
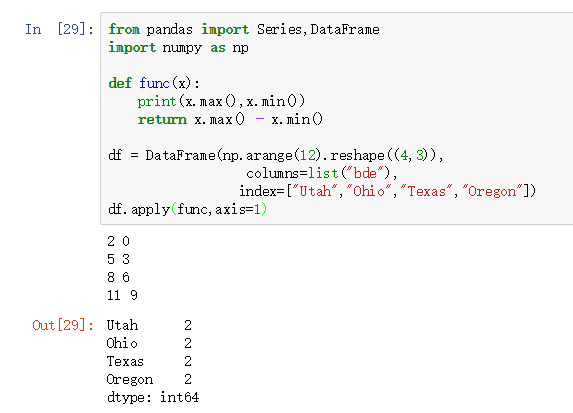
5.4.3 DataFrame对象的applymap方法
需要1个参数,参数的数据类型为函数对象,applymap方法的返回值的数据类型为DataFrame。applymap方法是对DataFram中的每一格进行映射,如下图所示:
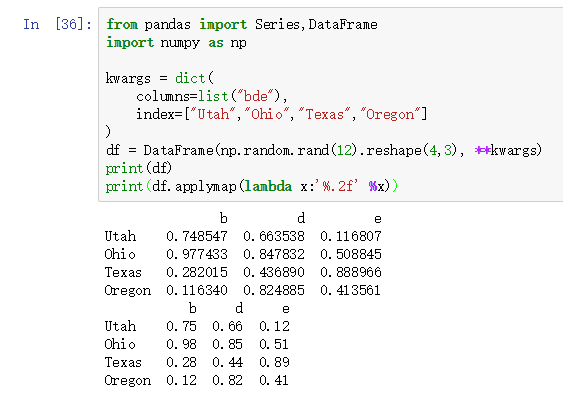
5.5 排序和排名
使用DataFrame对象的sort_valuse方法,需要两个参数:第1个参数by是根据哪一行或列排序;
第2个参数axis为0或1,默认为0,0为按列排序,1为按行排序。
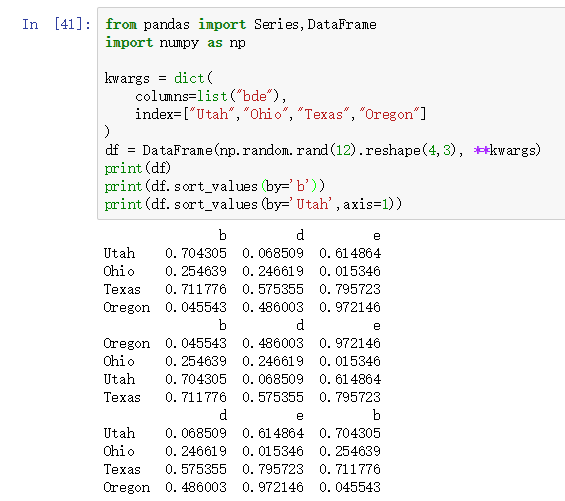
5.6 pandas的聚合函数
聚合函数包括:求和,最大值,最小值,计数、均值、方差、分位数
这些聚合函数都是基于没有缺失数据的情况。
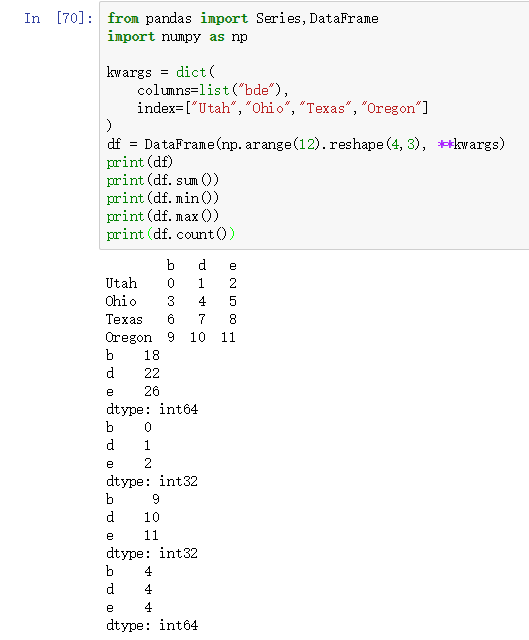
DataFrame对象的describe方法用于得出 统计信息。
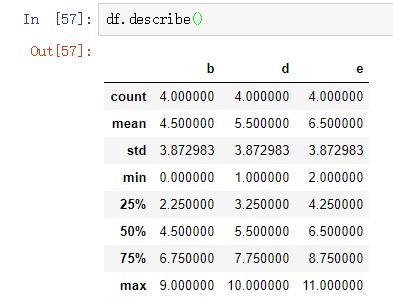
DataFrame对象的quantile函数可以得出分位数,
df.quantile(.1)等同于df.quantile(0.1),可以取出从小到大排序第10%位置的数。
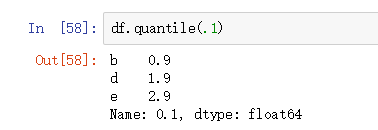
5.7 值集合、值计数
Series对象的unique方法可以得到值的集合,集合没有重复元素,相当于去除重复元素。
Series对象有value_counts方法可以得到值的集合,以及这些值出现的次数。
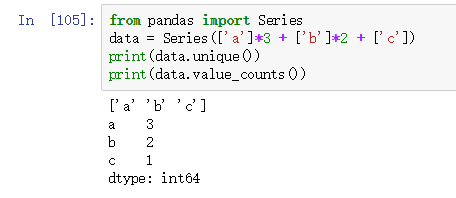
Series对象的value_counts方法类似于collections.Counter方法,如下图所示:
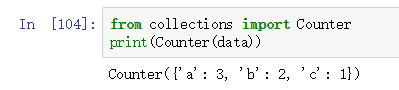
Series对象的isin方法可以获得元素数据类型为布尔bool的新Series,如下图所示:
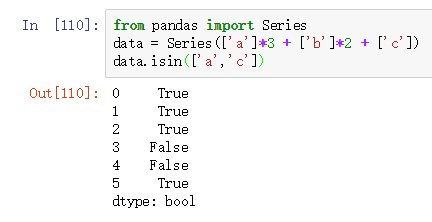
5.8 缺失值处理
缺失值数据在大部分数据分析应用中都很常见,pandas的设计目标之一就是让缺失数据的处理任务尽量轻松。
pandas对象上的所有描述统计都排除了缺失数据。
DataFrame对象和Series对象都有isnull方法,如下图所示:
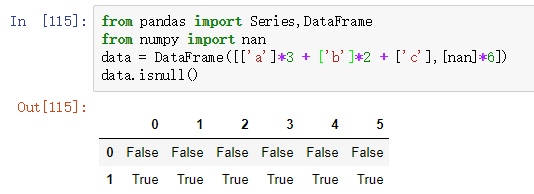
notnull方法为isnull方法结果的取反
fillna方法可以填充缺失值。
dropna方法可以根据行列中是否有空值进行删除。这个方法有2个参数:
关键字参数how,可以填入的值为any或all,any表示只要有1个空值则删除该行或该列,all表示要一行全为空值则删除该行。
关键字参数axis,可以填入的值为0或1,0表示对行进行操作,1表示对列进行操作
示例如下:
from pandas import Series,DataFrame
from numpy import nan
data = DataFrame([['a']*3 + ['b']*2 + ['c'],['b']*3 + [nan]*3])
print(data.dropna(axis=0,how='all'))
print(data.dropna(axis=0,how='any'))
print(data.dropna(axis=1,how='any'))
上面一段代码的运行结果如下图所示:
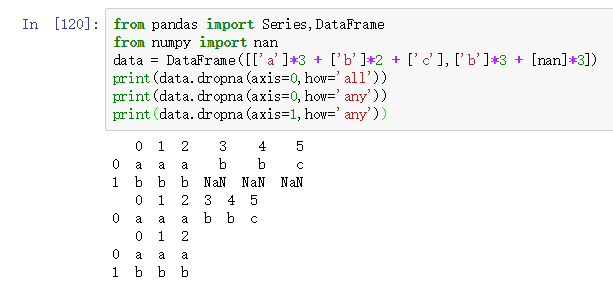
练习
练习作答文件下载链接: https://pan.baidu.com/s/1mQwBA3ZP1_EPBLGD5oRVAA 密码: xhc7
练习需要csv文件下载链接: https://pan.baidu.com/s/1SHs3-2yG7ofvi5etav2Dpg 密码: dfq3
Step 1. 导入相关的模块
from pandas import Series,DataFrame
import pandas as pd
import numpy as np
import matplotlib.pyplot as plt
Step 2. 导入数据,并赋值给变量df,输出前10行
df = pd.read_csv("Student_Alcohol.csv")
df.head(10)
Step 3. 为了便由于分析,请获取到数据集中从列名为school到列名guardian之间的所有数据
start_column = np.where(df.columns == 'school')[0][0]
end_column = np.where(df.columns == 'guardian')[0][0] + 1
selected_columns = df.columns[start_column:end_column]
df[selected_columns]
1行代码解答:
df.loc[:,'school':'guardian']
Step 4.创建一个能实现字符串的首字母大写的lambda匿名函数,应用到guardian 数据列实现如下效果
df['guardian'].apply(lambda x:x.title())
相同效果,不用lambda解答:
df['guardian'].apply(str.title)
Step 5. 将数据列 Mjob 和 Fjob中所有数据实现首字母大写
df[['Mjob','Fjob']].applymap(str.title)
Step 6. 经过第6步之后,为什么原来的dataframe数据中Mjob和Fjob列的数据仍然是小写的?简单说明原因,并修改原始dataframe中的数据使得Mjob和Fjob列变为首字母大写
函数操作不影响原数据,返回值的新数据要赋值给原数据,如下面代码所示:
df[['Mjob','Fjob']] = df[['Mjob','Fjob']].applymap(str.title)
Step 7.创建一个名为majority函数,并根据age列数据返回一个布尔值添加到新的数据列,列名为 legal_drinker (根据年龄这一列数据,大于17岁为合法饮酒)
df['legal_drinker'] = df['age'] > 17
6. Python中的字符串处理
对于大部分应用来说,python中的字符串应该已经足够。
如split()函数对字符串拆分,strip()函数对字符串去除两边空白字符。
复习字符串对象的4个方法:join方法连接字符串、 find方法寻找子字符串出现的索引位置、count方法返回子字符串出现的次数、 replace方法用来替换。
练习
练习所需csv文件下载链接: https://pan.baidu.com/s/1XZfldauALhGcyRr8EcUVVg 密码: hk67
Step1.Import the necessary libraries
import pandas as pd
from pandas import Series,DataFrame
Step2. Import the dataset from this address
Step3. Assign it to a variable called chipo
chipo = pd.read_csv("chipotle.csv",sep='\t')
Step4. See the first 10 entries
chipo.head(10)
Step5. What is the number of observations in the dataset?
len(chipo)
Step6. What is the number of columns in the dataset?
len(chipo.columns)
Step7. Print the name of all columns
print(list(chipo.columns))
Step8. How is the dataset indexed
chipo.index
Step9. Which was the most ordered item?
item_ordered_quantity = []
for name,group in chipo.groupby('item_name'):
item_ordered_quantity.append([name,group.quantity.sum()])
sorted(item_ordered_quantity,key = lambda x:x[1], reverse=True)[:5]
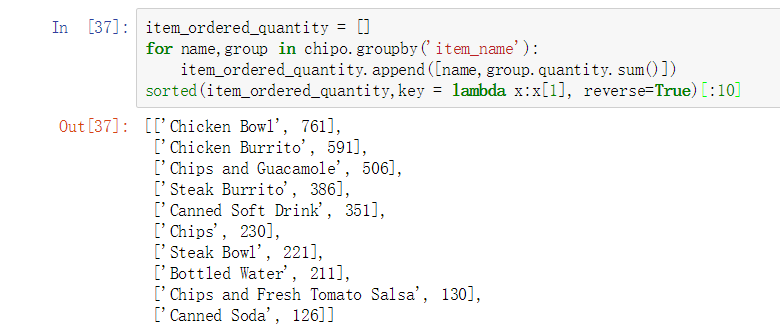
从上图可以看出 Chicken Bowl是购买最多的商品。
Step10. How many items were ordered?
chipo.quantity.sum()
Step11.What was the most ordered item in the choice_description column?
choice = chipo.choice_description.str.replace('[','').str.replace(']','').dropna()
choice_list = []
for item in choice:
choice_list.extend(item.split(','))
pd.value_counts(choice_list)[:10]
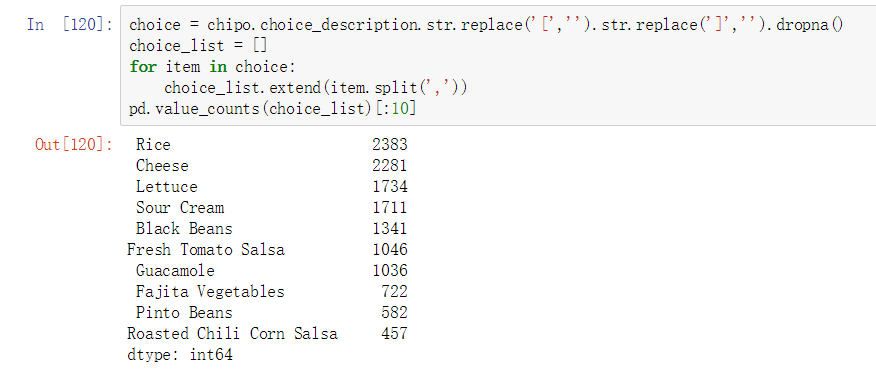
从上图中可以看出Rice是choice_description列中买的最多的商品
Step12.Turn the item price into a float
chipo.item_price.str.strip('$').astype('f')
Step13. How much was the revenue for the period in the dataset?
chipo['price'] = chipo.quantity * chipo.item_price.str.strip('$').astype('f')
chipo.price.sum()
Step14. How many orders were made in the period?
chipo.order_id.unique().shape[0]
第2种解法
chipo.order_id.value_counts().count()
Step15. What is the average amount per order?
chipo['price'] = chipo.quantity * chipo.item_price.str.strip('$').astype('f')
order_number = chipo.order_id.unique().shape[0]
chipo.price.sum()/order_number
Step16. How many different items are sold?
chipo.item_name.unique().shape[0]
7. Pandas中的时间序列
不管在哪个领域中(如金融学、经济学、生态学、神经科学、物理学等),时间序列数据都是一种重要的结构化数据形式。在多个时间点观察或者测量到的任何事物都是可以形成一段时间序列。很多时间序列是固定频率的,也就是说,数据点是根据某种规律定期出现的。时间序列也可以是不定期的。时间序列数据的意义取决于具体的应用场景,主要有以下几种:
1.时间戳,特定的时间
2.固定时期(period),如2017年1月或2017年
3.时间间隔(interval),由开始时间和结束时间戳表示,时期可以被看为时间间隔的特例。
7.1 Python标准库
包含用于日期(date)和时间(time)数据的数据类型,而且还有日历方面的功能。主要使用datetime、 time、 calendar模块。datetime.datetime也是用的最多的数据类型。
datetime以毫秒形式存储日期和时间,datetime.timedelta表示两个datetime对象之间的时间差。


7.2 日期时间类与字符串相互转换
使用datetime模块中的datatime对象的strftime方法将时间转换为字符串,需要1个参数,参数为字符串格式。方法的返回值的数据类型是字符串。
另外,其实time模块中有strftime方法,需要1个参数,参数为字符串格式。可以将现在的时间转换为字符串。
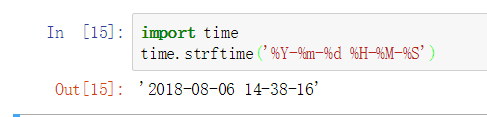
使用datetime模块中的striptime方法,需要2个参数,第1个参数是字符串,第2个参数是字符串格式。方法返回值的数据类型是datetime对象。
字符串转换为datetime对象,其实有1个更简单的方法,使用dateutil包中parser文件的parse方法。
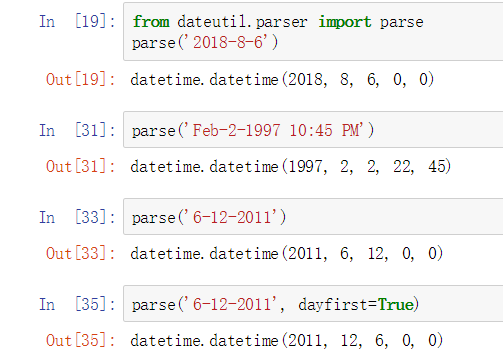
7.3 Pandas中的时间序列
pandas通常是用于处理成组日期的,不管这个日期是DataFrame的轴索引还是列。to_datetime方法可以解析多种不同的日期表示形式。对标准日期形式的解析非常快。
to_datetime方法可以处理缺失值,缺失值会被处理为NaT(not a time)。
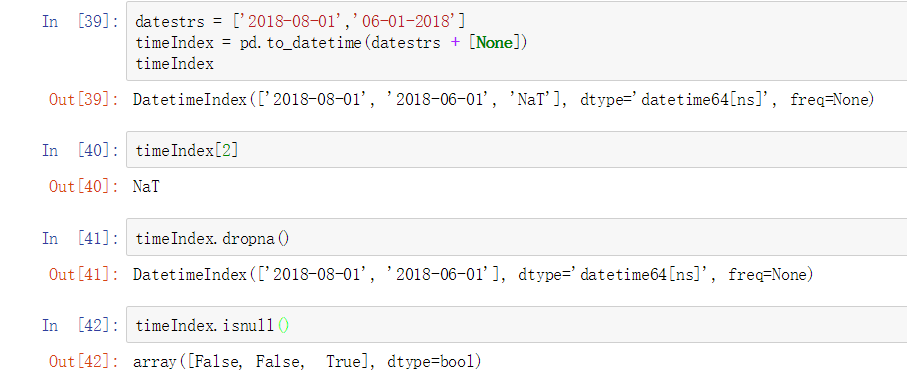
7.4 时间序列切片索引
对于较长的时间序列,只需传入“年”或者“年-月”即可轻松选取数据的切片。
pandas库中的date_range方法可以产生时间日期索引,关键字periods可以指定有多少天。
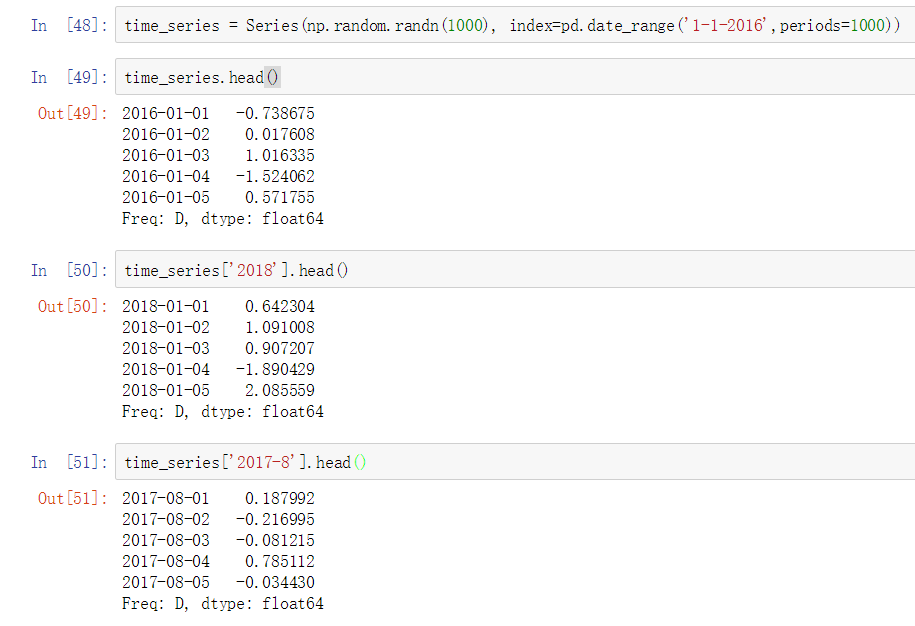