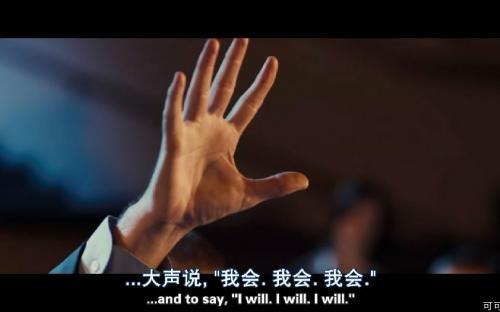
利用python对数据进行描述性统计分析-以鸢尾花数据集为例
学习前准备
- 下载Anaconda软件
主题
主要讲述数据分析中,数理统计基础中的描述性统计知识和python中的实现。
知识要点
数理统计基础
数理统计,以概率论为基础,研究大量随机现象的统计规律性,分为以下两类:
- 描述统计
- 推断统计
描述性统计分析概述
概念
描述性统计所提取的统计信息,称之为统计量,主要包括:
- 频数与频率
- 集中趋势分析:均值、中位数、众数、分位数
- 离散程度分析:极差、方差、标准差
- 分布形状:偏度、峰度
变量类型
- 类别变量:无序类别变量、有序类别变量‘
- 数值变量:连续变量、离散变量
统计量
频数与频率
import numpy as np
import pandas as pd
import matplotlib.pyplot as plt
import seaborn as sns
from sklearn.datasets import load_iris
import warnings
# 设置seaborn绘图的样式。
sns.set(style= "darkgrid", font_scale=1.2)
#设置中文字体
plt.rcParams["font.family"] = "SimHei"
#是否使用Unicode字符集中的负号
plt.rcParams["axes.unicode_minus"] = False
# 忽略警告信息。
warnings.filterwarnings("ignore")
#加载鸢尾花数据集
iris = load_iris()
#iris.data 鸢尾花数据集,
print(iris.data[:10])
#iris.target:每朵鸢尾花对应的类别。(取值为0,1,2)
print(iris.target[::20])
#iris.feature_names: 特征列的名称
print(iris.feature_names)
#iris.target_names: 鸢尾花类别的名称
print(iris.target_names)
[[5.1 3.5 1.4 0.2]
[4.9 3. 1.4 0.2]
[4.7 3.2 1.3 0.2]
[4.6 3.1 1.5 0.2]
[5. 3.6 1.4 0.2]
[5.4 3.9 1.7 0.4]
[4.6 3.4 1.4 0.3]
[5. 3.4 1.5 0.2]
[4.4 2.9 1.4 0.2]
[4.9 3.1 1.5 0.1]]
[0 0 0 1 1 2 2 2]
['sepal length (cm)', 'sepal width (cm)', 'petal length (cm)', 'petal width (cm)']
['setosa' 'versicolor' 'virginica']
# 将鸢尾花数据与对应的类型合并,组合成完整的记录。记得iris.data, iris.target.reshape()要带中括号
data = np.concatenate([iris.data, iris.target.reshape(-1,1)], axis = 1)
data = pd.DataFrame(data,
columns=["sepal length", "sepal width", "petal length", "petal width","type"])
data.sample(10)# sample(), 随机抽样