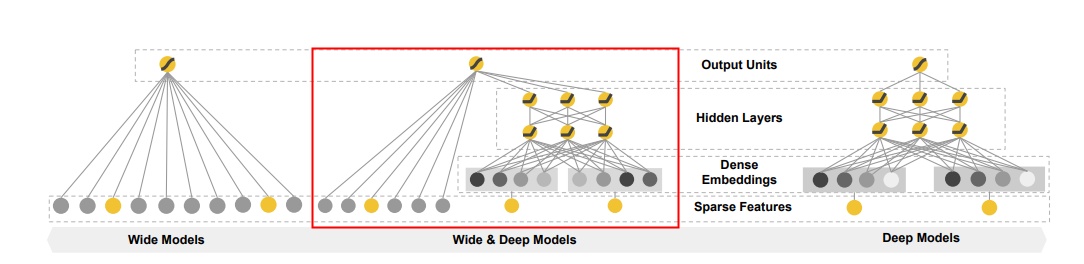
What
推荐系统广泛使用的一个组合模型,将LR与DNN模型融入到一个结构中,如下中间部分。它是2016年,Google针对Google Play的业务场景提出的,论文地址https://arxiv.org/pdf/1606.07792.pdf。
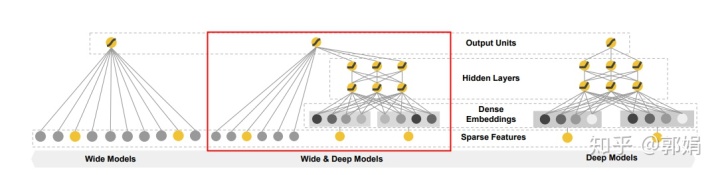
Why
Generalized linear models with nonlinear feature transformations are widely used for large-scale regression and classification problems with sparse inputs. Memorization of feature interactions through a wide set of cross-product feature transformations are effective and interpretable, while generalization requires more feature engineering effort.With less feature engineering, deep neural networks can generalize better to unseen feature combinations through low-dimensional dense embeddings learned for the sparse features. However, deep neural networks with embeddings can over-generalize and recommend less relevant items when the user-item interactions are sparse and high-rank. In this paper, we present Wide & Deep learning—jointly trained wide linear models and deep neural networks—to combine the benefits of memorization and generalization for recommender systems. 简单说就是优势互补,并且效果也更好。
论文里的一个效果对比:
- 离/在线推荐效果
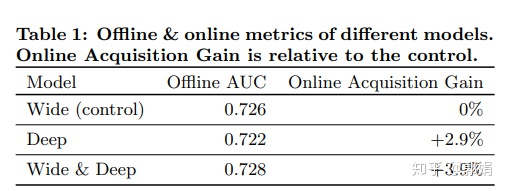
- 性能
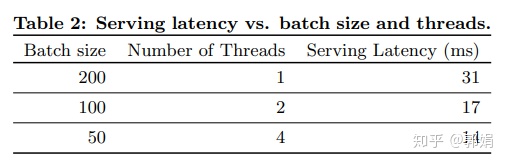
构成
- 组合公式

where Y is the binary class label, σ(·) is the sigmoid function, φ(x) are the cross product transformations of the original features x, and b is the bias term. Wwide is the vector of all wide model weights, and Wdeep are the weights applied on the final activations a (lf ) .
损失函数:逻辑损失,与LR类似。区别就是把Wide和Deep两部分加和再进行训练。
- 架构pipeline
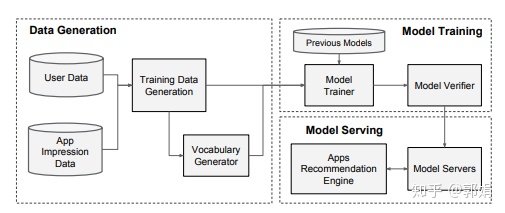
- 模型结构
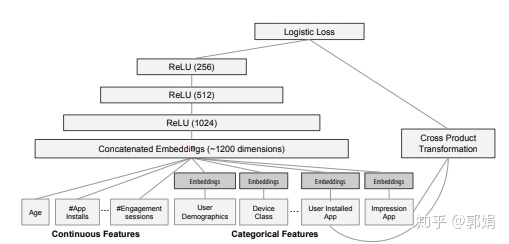
How
理论
- 特征处理
连续特征的处理: 如果要放入wide层,需要离散化(有监督和非监督方式,比如等频等宽、聚类、卡方等),如果放入deep层,可以是dense 特征,提前预计算或者提取, 也可以是sparse 特征,让模型自动进行dense vector的获取。
类别特征的处理:放入wide 层,可以进行一些组合处理。 也可以放入deep层,通常需要进行hash、onehot、字典等处理,转换成数值特征。
- 模型更新
Wide层使用FTRL进行优化,Deep层使用AdaGrad进行优化。
实践
环境准备:安装anoconda,配置jupyter, 创建python虚拟环境,安装TensorFlow。
模型训练通用步骤:1) 获得训练数据; 2) 特征处理 3) 模型训练 4) 模型评估 5) 模型部署。
样例数据
如下数据中心有14个特征x,一个标签y:
'age', 'workclass', 'fnlwgt', 'education', 'education_num','marital_status', 'occupation', 'relationship', 'race', 'gender','capital_gain', 'capital_loss', 'hours_per_week', 'native_country','income_bracket'
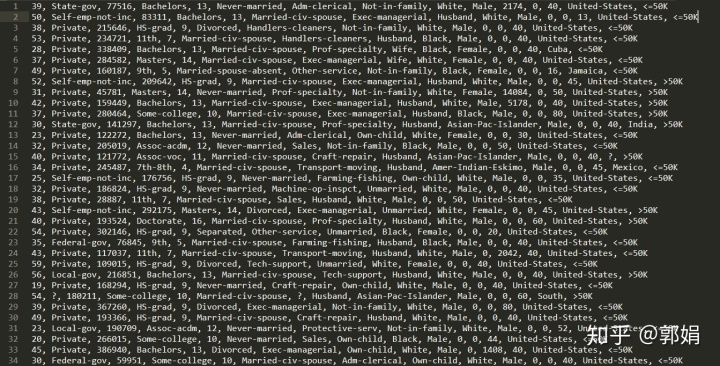
部分代码
from __future__ import absolute_import
from __future__ import division
from __future__ import print_function
import os
import sys
# pylint: disable=wrong-import-order
from absl import app as absl_app
from absl import flags
from six.moves import urllib
from six.moves import zip
import tensorflow as tf
# pylint: enable=wrong-import-order
from official.utils.flags import core as flags_core
DATA_URL = 'https://archive.ics.uci.edu/ml/machine-learning-databases/adult'
TRAINING_FILE = 'adult.data'
TRAINING_URL = '%s/%s' % (DATA_URL, TRAINING_FILE)
EVAL_FILE = 'adult.test'
EVAL_URL = '%s/%s' % (DATA_URL, EVAL_FILE)
_CSV_COLUMNS = [
'age', 'workclass', 'fnlwgt', 'education', 'education_num',
'marital_status', 'occupation', 'relationship', 'race', 'gender',
'capital_gain', 'capital_loss', 'hours_per_week', 'native_country',
'income_bracket'
]
# 缺失值的处理
_CSV_COLUMN_DEFAULTS = [[0], [''], [0], [''], [0], [''], [''], [''], [''], [''],
[0], [0], [0], [''], ['']]
_HASH_BUCKET_SIZE = 1000
_NUM_EXAMPLES = {
'train': 32561,
'validation': 16281,
}
def _download_and_clean_file(filename, url):
"""Downloads data from url, and makes changes to match the CSV format."""
temp_file, _ = urllib.request.urlretrieve(url)
with tf.gfile.Open(temp_file, 'r') as temp_eval_file:
with tf.gfile.Open(filename, 'w') as eval_file:
for line in temp_eval_file:
line = line.strip()
line = line.replace(', ', ',')
if not line or ',' not in line:
continue
if line[-1] == '.':
line = line[:-1]
line += 'n'
eval_file.write(line)
tf.gfile.Remove(temp_file)
def download(data_dir):
"""Download census data if it is not already present."""
tf.gfile.MakeDirs(data_dir)
training_file_path = os.path.join(data_dir, TRAINING_FILE)
if not tf.gfile.Exists(training_file_path):
_download_and_clean_file(training_file_path, TRAINING_URL)
eval_file_path = os.path.join(data_dir, EVAL_FILE)
if not tf.gfile.Exists(eval_file_path):
_download_and_clean_file(eval_file_path, EVAL_URL)
def build_model_columns():
"""Builds a set of wide and deep feature columns."""
# Continuous variable columns
age = tf.feature_column.numeric_column('age')
education_num = tf.feature_column.numeric_column('education_num')
capital_gain = tf.feature_column.numeric_column('capital_gain')
capital_loss = tf.feature_column.numeric_column('capital_loss')
hours_per_week = tf.feature_column.numeric_column('hours_per_week')
education = tf.feature_column.categorical_column_with_vocabulary_list(
'education', [
'Bachelors', 'HS-grad', '11th', 'Masters', '9th', 'Some-college',
'Assoc-acdm', 'Assoc-voc', '7th-8th', 'Doctorate', 'Prof-school',
'5th-6th', '10th', '1st-4th', 'Preschool', '12th'])
marital_status = tf.feature_column.categorical_column_with_vocabulary_list(
'marital_status', [
'Married-civ-spouse', 'Divorced', 'Married-spouse-absent',
'Never-married', 'Separated', 'Married-AF-spouse', 'Widowed'])
relationship = tf.feature_column.categorical_column_with_vocabulary_list(
'relationship', [
'Husband', 'Not-in-family', 'Wife', 'Own-child', 'Unmarried',
'Other-relative'])
workclass = tf.feature_column.categorical_column_with_vocabulary_list(
'workclass', [
'Self-emp-not-inc', 'Private', 'State-gov', 'Federal-gov',
'Local-gov', '?', 'Self-emp-inc', 'Without-pay', 'Never-worked'])
# To show an example of hashing:
occupation = tf.feature_column.categorical_column_with_hash_bucket(
'occupation', hash_bucket_size=_HASH_BUCKET_SIZE)
# Transformations.
age_buckets = tf.feature_column.bucketized_column(
age, boundaries=[18, 25, 30, 35, 40, 45, 50, 55, 60, 65])
# Wide columns and deep columns.
base_columns = [
education, marital_status, relationship, workclass, occupation,
age_buckets,
]
crossed_columns = [
tf.feature_column.crossed_column(
['education', 'occupation'], hash_bucket_size=_HASH_BUCKET_SIZE),
tf.feature_column.crossed_column(
[age_buckets, 'education', 'occupation'],
hash_bucket_size=_HASH_BUCKET_SIZE),
]
wide_columns = base_columns + crossed_columns
deep_columns = [
age,
education_num,
capital_gain,
capital_loss,
hours_per_week,
tf.feature_column.indicator_column(workclass),
tf.feature_column.indicator_column(education),
tf.feature_column.indicator_column(marital_status),
tf.feature_column.indicator_column(relationship),
# To show an example of embedding
tf.feature_column.embedding_column(occupation, dimension=8),
]
return wide_columns, deep_columns
def input_fn(data_file, num_epochs, shuffle, batch_size):
"""Generate an input function for the Estimator."""
assert tf.gfile.Exists(data_file), (
'%s not found. Please make sure you have run census_dataset.py and '
'set the --data_dir argument to the correct path.' % data_file)
def parse_csv(value):
tf.logging.info('Parsing {}'.format(data_file))
columns = tf.decode_csv(value, record_defaults=_CSV_COLUMN_DEFAULTS)
features = dict(list(zip(_CSV_COLUMNS, columns)))
labels = features.pop('income_bracket')
classes = tf.equal(labels, '>50K') # binary classification
return features, classes
# Extract lines from input files using the Dataset API.
dataset = tf.data.TextLineDataset(data_file)
if shuffle:
dataset = dataset.shuffle(buffer_size=_NUM_EXAMPLES['train'])
dataset = dataset.map(parse_csv, num_parallel_calls=5)
# We call repeat after shuffling, rather than before, to prevent separate
# epochs from blending together.
dataset = dataset.repeat(num_epochs)
dataset = dataset.batch(batch_size)
return dataset
def define_data_download_flags():
"""Add flags specifying data download arguments."""
flags.DEFINE_string(
name="data_dir", default="/tmp/census_data/",
help=flags_core.help_wrap(
"Directory to download and extract data."))
def main(_):
download(flags.FLAGS.data_dir)
if __name__ == '__main__':
tf.logging.set_verbosity(tf.logging.INFO)
define_data_download_flags()
absl_app.run(main)
def build_estimator(model_dir, model_type, model_column_fn, inter_op, intra_op):
"""Build an estimator appropriate for the given model type."""
wide_columns, deep_columns = model_column_fn()
hidden_units = [100, 75, 50, 25]
# Create a tf.estimator.RunConfig to ensure the model is run on CPU, which
# trains faster than GPU for this model.
run_config = tf.estimator.RunConfig().replace(session_config=tf.compat.v1.ConfigProto(device_count={'GPU': 0},inter_op_parallelism_threads=inter_op,intra_op_parallelism_threads=intra_op))
if model_type == 'wide':
return tf.estimator.LinearClassifier(model_dir=model_dir,feature_columns=wide_columns,config=run_config)
elif model_type == 'deep':
return tf.estimator.DNNClassifier(model_dir=model_dir,feature_columns=deep_columns,hidden_units=hidden_units,config=run_config)
else:
return tf.estimator.DNNLinearCombinedClassifier(model_dir=model_dir,linear_feature_columns=wide_columns, dnn_feature_columns=deep_columns,dnn_hidden_units=hidden_units,config=run_config)
def run_loop(name, train_input_fn, eval_input_fn, model_column_fn,
build_estimator_fn, flags_obj, tensors_to_log, early_stop=False):
"""Define training loop."""
# model_helpers.apply_clean(flags.FLAGS)
model = build_estimator_fn(model_dir=model_dir, model_type=model_type,
model_column_fn=model_column_fn,
inter_op=inter_op_parallelism_threads,
intra_op=intra_op_parallelism_threads)
run_params = {
'batch_size': batch_size,
'train_epochs': train_epochs,
'model_type': model_type,
}
logger.info('wide_deep', name, run_params,test_id)
loss_prefix = LOSS_PREFIX.get(model_type, '')
tensors_to_log = {k: v.format(loss_prefix=loss_prefix)
for k, v in tensors_to_log.items()}
# train_hooks = hooks_helper.get_train_hooks(hooks, model_dir=model_dir,batch_size=batch_size, tensors_to_log=tensors_to_log)
# Train and evaluate the model every `flags.epochs_between_evals` epochs.
for n in range(train_epochs // epochs_between_evals):
# model.train(input_fn=train_input_fn, hooks=train_hooks)
model.train(input_fn=train_input_fn)
results = model.evaluate(input_fn=eval_input_fn)
# Display evaluation metrics
logger.info('Results at epoch %d / %d',(n + 1) * epochs_between_evals,train_epochs)
logger.info('-' * 60)
for key in sorted(results):
logging.info('%s: %s' % (key, results[key]))
logger.info(results)
if early_stop:
break
def run_census(flags_obj):
"""Construct all necessary functions and call run_loop.
Args:
flags_obj: Object containing user specified flags.
"""
# if flags_obj.download_if_missing:
# census_dataset.download(flags_obj.data_dir)
train_file = os.path.join(data_dir, TRAINING_FILE)
test_file = os.path.join(data_dir, EVAL_FILE)
# Train and evaluate the model every `flags.epochs_between_evals` epochs.
def train_input_fn():
return input_fn(
train_file, epochs_between_evals, True, batch_size)
def eval_input_fn():
return input_fn(test_file, 1, False, batch_size)
tensors_to_log = {
'average_loss': '{loss_prefix}head/truediv',
'loss': '{loss_prefix}head/weighted_loss/Sum'
}
run_loop(name="Census Income", train_input_fn=train_input_fn,
eval_input_fn=eval_input_fn,
model_column_fn=build_model_columns,
build_estimator_fn=build_estimator,
flags_obj=flags_obj,
tensors_to_log=tensors_to_log,
early_stop=True)
参考文档
tensorflow 安装:https://www.tensorflow.org/install/index
wide&deep 模型:https://arxiv.org/pdf/1606.07792.pdf
anaconda 以及jupyter配置:https://www.cnblogs.com/faramita2016/p/7512471.html
论文解读:https://zhuanlan.zhihu.com/p/53361519