1 说明:
=====
1.1 完整版:汽车车道动态视频检测讲解和注释版代码,小白秒懂。
1.2 python+OpenCV+moviepy+numpy为主的技术要点。
1.3 代码来源:
https://github.com/linghugoogle/CarND-Advanced-Lane-Lines #虽然感觉也是fork别人的,别忘了给他点个赞star
1.4 感谢原作者,并对文件进行修改和代码进行删减,注释等操作,便于操作和理解。
1.5 应用:无人驾驶汽车技术,热门!
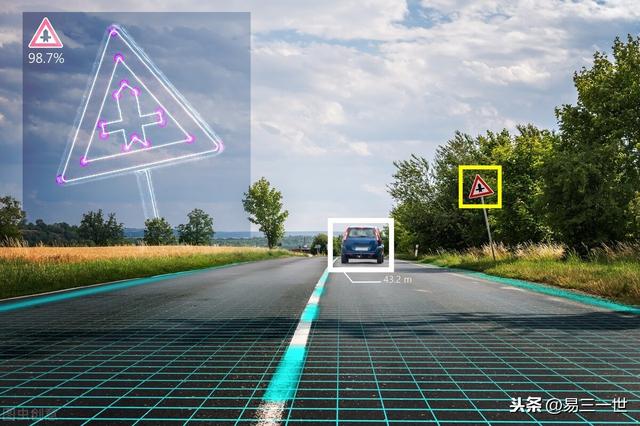
2 效果展示:由于gif≤10MB,所以是节选。
================================
2.1 原视频节选:
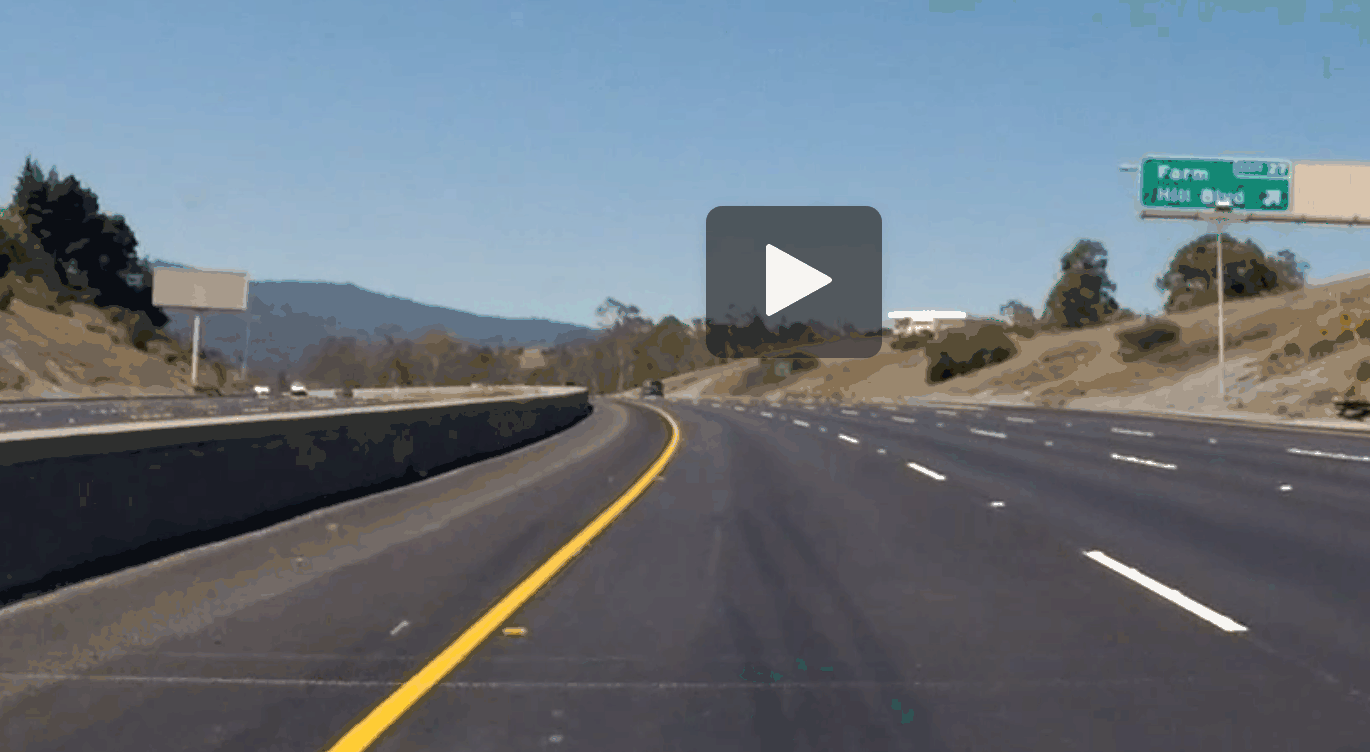
2.2 处理后视频节选:
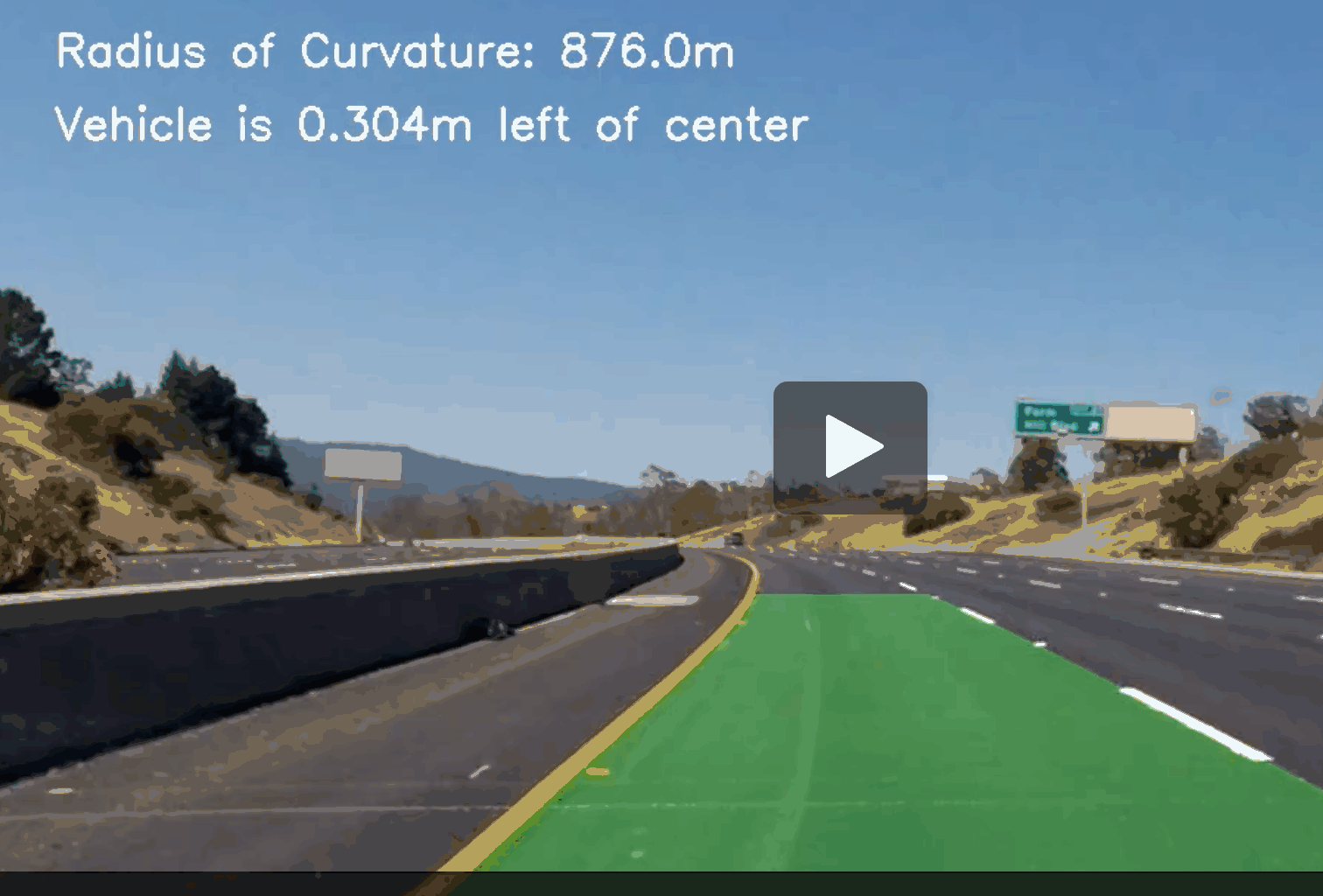
3 准备:
=====
3.1 环境:python3.8+OpenCV4.2.0+deepin-linux操作系统。
3.2 文件结构:
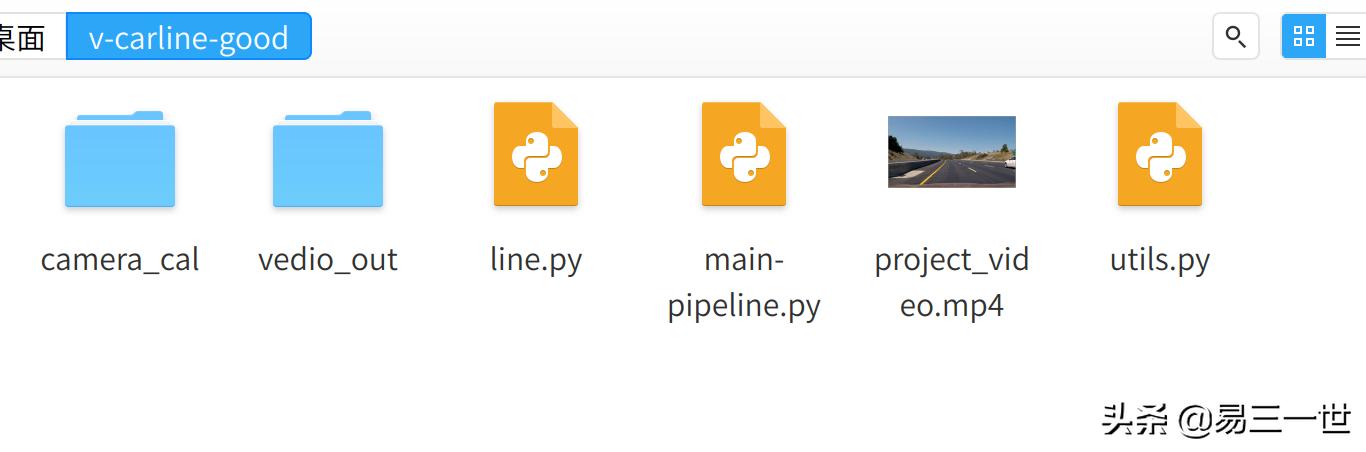
github下载下来
project_video.mp4 :原始视频,未进行标注
vedio_out:文件夹为输出被标注的视频(处理后的视频文件夹)
camera_cal:相机参数标定文件夹。
4 代码讲解:
=========
4.1 line.py代码:
# -*- coding: utf-8 -*-#导入模块import numpy as np#定义line这个类class Line(): #初始化参数 def __init__(self): # was the line detected in the last iteration? self.detected = False # x values of the last n fits of the line self.recent_fitted = [np.array([False])] #average x values of the fitted line over the last n iterations self.bestx = None #polynomial coefficients averaged over the last n iterations self.best_fit = None #polynomial coefficients for the most recent fit self.current_fit = [np.array([False])] #radius of curvature of the line in some units self.radius_of_curvature = None #distance in meters of vehicle center from the line self.line_base_pos = None #difference in fit coefficients between last and new fits self.diffs = np.array([0,0,0], dtype='float') #x values for detected line pixels self.allx = None #y values for detected line pixels self.ally = None #检测侦测 def check_detected(self): if (self.diffs[0] < 0.01 and self.diffs[1] < 10.0 and self.diffs[2] < 1000.) and len(self.recent_fitted) > 0: return True else: return False #更新 def update(self,fit): if fit is not None: if self.best_fit is not None: self.diffs = abs(fit - self.best_fit) if self.check_detected(): self.detected =True if len(self.recent_fitted)>10: self.recent_fitted = self.recent_fitted[1:] self.recent_fitted.append(fit) else: self.recent_fitted.append(fit) self.best_fit = np.average(self.recent_fitted, axis=0) self.current_fit = fit else: self.detected = False else: self.best_fit = fit self.current_fit = fit self.detected=True self.recent_fitted.append(fit)
4.2 utils.py代码省略。
4.3 main-pipeline.py(就是代码为:pipeline.py)
# -*- coding: utf-8 -*-#第1步:导入模块import osimport cv2import matplotlib.pyplot as pltimport numpy as npfrom moviepy.editor import VideoFileClipimport line #自定义模块import utils #自定义模块#第2步:图片阈值处理def thresholding(img): #setting all sorts of thresholds x_thresh = utils.abs_sobel_thresh(img, orient='x', thresh_min=10 ,thresh_max=230) mag_thresh = utils.mag_thresh(img, sobel_kernel=3, mag_thresh=(30, 150)) dir_thresh = utils.dir_threshold(img, sobel_kernel=3, thresh=(0.7, 1.3)) hls_thresh = utils.hls_select(img, thresh=(180, 255)) lab_thresh = utils.lab_select(img, thresh=(155, 200)) luv_thresh = utils.luv_select(img, thresh=(225, 255)) #Thresholding combination threshholded = np.zeros_like(x_thresh) threshholded[((x_thresh == 1) & (mag_thresh == 1)) | ((dir_thresh == 1) & (hls_thresh == 1)) | (lab_thresh == 1) | (luv_thresh == 1)] = 1 return threshholded#第3步:视频拟合和图片纠正def processing(img,object_points,img_points,M,Minv,left_line,right_line): #camera calibration, image distortion correction undist = utils.cal_undistort(img,object_points,img_points) #get the thresholded binary image thresholded = thresholding(undist) #perform perspective transform thresholded_wraped = cv2.warpPerspective(thresholded, M, img.shape[1::-1], flags=cv2.INTER_LINEAR) #perform detection if left_line.detected and right_line.detected: left_fit, right_fit, left_lane_inds, right_lane_inds = utils.find_line_by_previous(thresholded_wraped,left_line.current_fit,right_line.current_fit) else: left_fit, right_fit, left_lane_inds, right_lane_inds = utils.find_line(thresholded_wraped) left_line.update(left_fit) right_line.update(right_fit) #draw the detected laneline and the information area_img = utils.draw_area(undist,thresholded_wraped,Minv,left_fit, right_fit) curvature,pos_from_center = utils.calculate_curv_and_pos(thresholded_wraped,left_fit, right_fit) result = utils.draw_values(area_img,curvature,pos_from_center) return result#第4步:步骤:划线-校正-读取原视频和生成修改后的视频#划线left_line = line.Line() #左线right_line = line.Line() #右线#获取棋盘格图片#使用提供的一组棋盘格图片计算相机校正矩阵(camera calibration matrix)和失真系数(distortion coefficients).cal_imgs = utils.get_images_by_dir('/home/xgj/Desktop/v-carline-good/camera_cal')#计算object_points,img_pointsobject_points,img_points = utils.calibrate(cal_imgs,grid=(9,6))M,Minv = utils.get_M_Minv()#需要修改的视频:原视频project_video_clip = VideoFileClip("/home/xgj/Desktop/v-carline-good/project_video.mp4")#输出修改后的视频:完成视频project_outpath = '/home/xgj/Desktop/v-carline-good/vedio_out/project_video_out.mp4'#制作视频project_video_out_clip = project_video_clip.fl_image(lambda clip: processing(clip,object_points,img_points,M,Minv,left_line,right_line))project_video_out_clip.write_videofile(project_outpath, audio=False)
5 完结:
=====
5.1 以上代码完整,但制作视频估计花20分钟,我也是将代码最简化跑起来。
5.2 如果逐步深入分析,可能要从基本开始。
5.3 可以参考这篇文章:
https://zhuanlan.zhihu.com/p/46146266