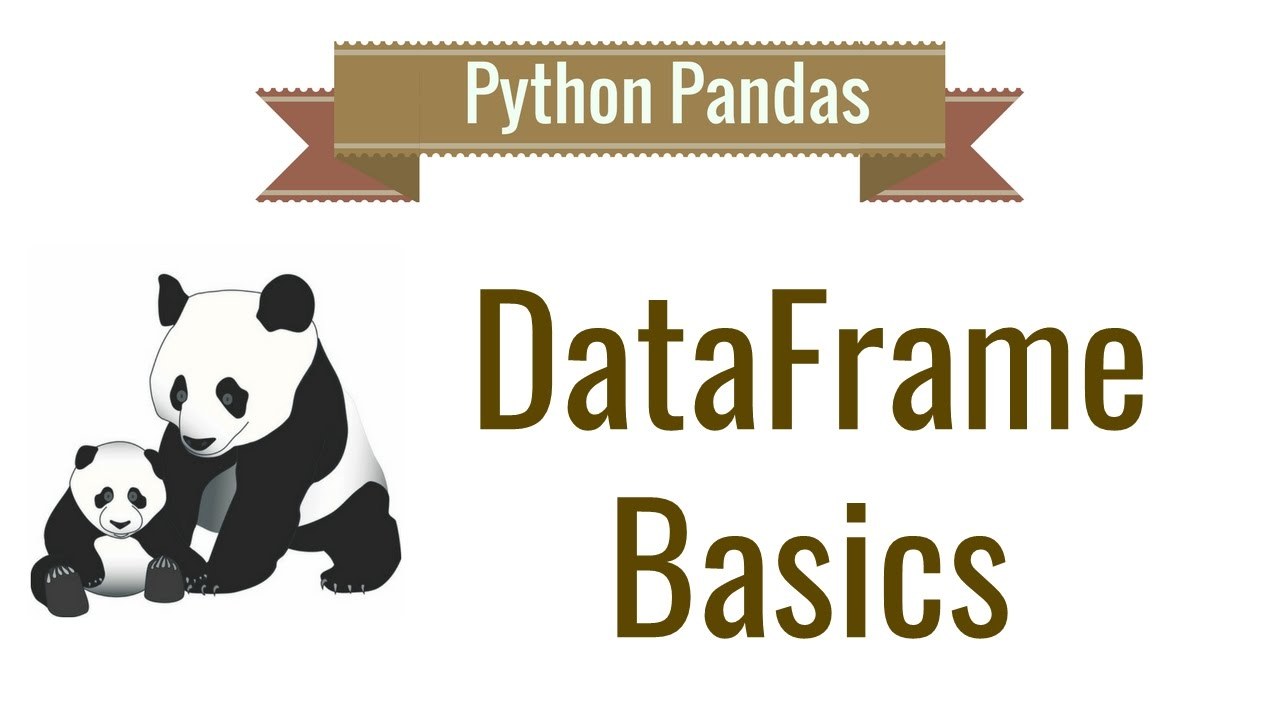
每台电脑内存都是有限的,当某些DataFrame的size较大时,很可能多载入了几个df内存就吃不消了,除了及时用gc清理不再需要的变量以外,作者最近还在kaggle论坛看到一个kernel,提供了一个减少每个df自身占用内存容量的方法,具体链接见:
Reducing DataFrame memory size by ~65%www.kaggle.com其实简单来说,这个kernel的原作者编写了一个函数可以实现以下功能
- 循环每列
- 判断是否该列类型为numeric
- 判断是否该列类型为int
- 找到最小最大值
- 找到一个最节省内存的datatype去fit这一列
具体函数代码如下:
import numpy as np # linear algebra
import pandas as pd # data processing, CSV file I/O (e.g. pd.read_csv)
def reduce_mem_usage(props):
start_mem_usg = props.memory_usage().sum() / 1024**2
print("Memory usage of properties dataframe is :",start_mem_usg," MB")
NAlist = [] # Keeps track of columns that have missing values filled in.
for col in props.columns:
if props[col].dtype != object: # Exclude strings
# Print current column type
print("******************************")
print("Column: ",col)
print("dtype before: ",props[col].dtype)
# make variables for Int, max and min
IsInt = False
mx = props[col].max()
mn = props[col].min()
# Integer does not support NA, therefore, NA needs to be filled
if not np.isfinite(props[col]).all():
NAlist.append(col)
props[col].fillna(mn-1,inplace=True)
# test if column can be converted to an integer
asint = props[col].fillna(0).astype(np.int64)
result = (props[col] - asint)
result = result.sum()
if result > -0.01 and result < 0.01:
IsInt = True
# Make Integer/unsigned Integer datatypes
if IsInt:
if mn >= 0:
if mx < 255:
props[col] = props[col].astype(np.uint8)
elif mx < 65535:
props[col] = props[col].astype(np.uint16)
elif mx < 4294967295:
props[col] = props[col].astype(np.uint32)
else:
props[col] = props[col].astype(np.uint64)
else:
if mn > np.iinfo(np.int8).min and mx < np.iinfo(np.int8).max:
props[col] = props[col].astype(np.int8)
elif mn > np.iinfo(np.int16).min and mx < np.iinfo(np.int16).max:
props[col] = props[col].astype(np.int16)
elif mn > np.iinfo(np.int32).min and mx < np.iinfo(np.int32).max:
props[col] = props[col].astype(np.int32)
elif mn > np.iinfo(np.int64).min and mx < np.iinfo(np.int64).max:
props[col] = props[col].astype(np.int64)
# Make float datatypes 32 bit
else:
props[col] = props[col].astype(np.float32)
# Print new column type
print("dtype after: ",props[col].dtype)
print("******************************")
# Print final result
print("___MEMORY USAGE AFTER COMPLETION:___")
mem_usg = props.memory_usage().sum() / 1024**2
print("Memory usage is: ",mem_usg," MB")
print("This is ",100*mem_usg/start_mem_usg,"% of the initial size")
return props, NAlist
使用方法很简单,df是我们想要精简的DataFrame,那么直接使用下面这行代码即可:
df = reduce_mem_usage(df)[0]
简单易用,并且可以提升内存管理效率,值得一试。