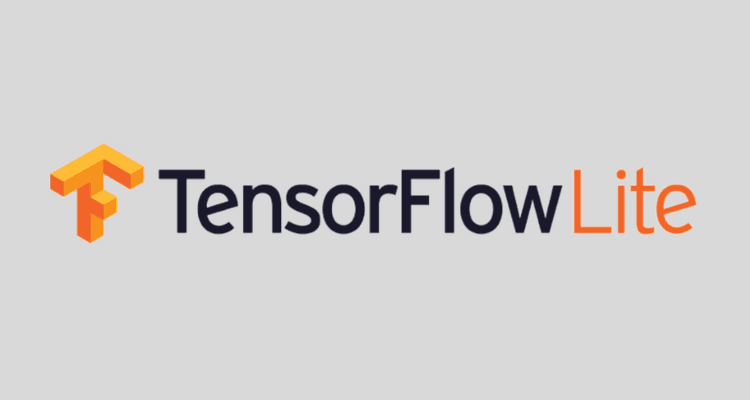
准备条件:
- 树莓派Pi4B & Buster系统
- 安装了python virtualenv :参考我的上一篇 马马虎虎:树莓派raspberry4B入坑指南 part-1
准备python 3.7环境
$ mkdir tflite & cd tflite/
$ virtualenv ENV
$ source ENV/bin/activate
$ python --version
Python 3.7.3
安装TensorFlow Lite
以下操作所需要的文件我都已经下载好了,具体参考我的github:https://github.com/whgreate/pi4b_tensorflow_lite
TensorFlow lite官网 https://www.tensorflow.org/lite/guide/python ,提供了多个版本,我们根据自己的版本,应该是选择Python 3.7 & ARM32 这个:
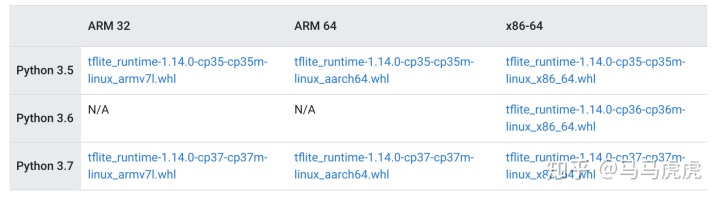
# 可选,克隆我的github地址,包含所有的安装文件和训练模型
$ git clone https://github.com/whgreate/pi4b_tensorflow_lite && cd pi4b_tensorflow_lite
# 备用下载链接 git clone https://gitee.com/whgreate/pi4b_tensorflow_lite.git
# 安装tensorflow lite
$ pip install tflite_runtime-1.14.0-cp37-cp37m-linux_armv7l.whl
Looking in indexes: https://pypi.org/simple, https://www.piwheels.org/simple
Processing ./tflite_runtime-1.14.0-cp37-cp37m-linux_armv7l.whl
Installing collected packages: tflite-runtime
Successfully installed tflite-runtime-1.14.0
# numpy pillow库需要的一些依赖
$ sudo apt-get install libatlas-base-dev
$ sudo apt-get install libjpeg-dev
# 安装 numpy pillow
$ pip install -r requirements.txt
# 备用
# pip install -r requirements.txt -i https://mirrors.aliyun.com/pypi/simple
修改测试文件(可选!!!)
本节都是可选操作,我在github中已经把label_image.py修改好了。
如果参考官网安装(https://www.tensorflow.org/lite/guide/python)的话,需要注意修改label_image.py
,因为我们使用的tensorflow lite,而非大而全的TensorFlow,所以包名有所出入,以下两处修改:
import tensorflow as tf
换成:
import tflite_runtime.interpreter as tflite
interpreter = tf.lite.Interpreter(model_path=args.model_file)
换成:
interpreter = tflite.Interpreter(model_path=args.model_file)
运行图像分类模型
选取TensorFlow Example的图片,执行以下命令:
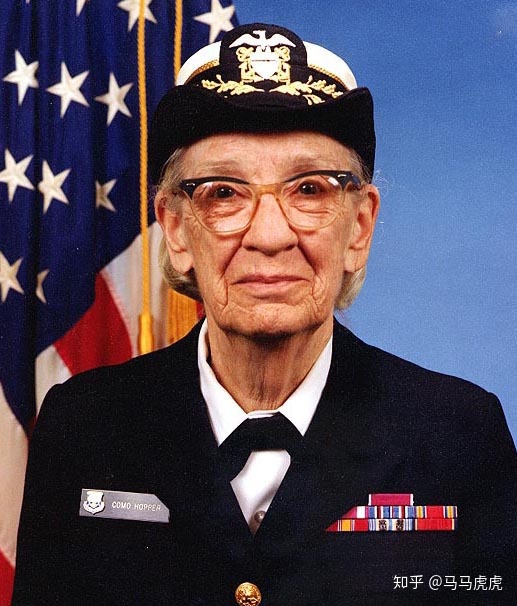
$ python label_image.py -m mobilenet_v1_1.0_224_quant.tflite -l labels_mobilenet_quant_v1_224.txt -i grace_hopper.bmp
INFO: Initialized TensorFlow Lite runtime.
0.658824: military uniform
0.149020: Windsor tie
0.039216: bow tie
0.027451: mortarboard
0.019608: bulletproof vest
可以看出65%概率识别出了服装,可以试试各种大小的图片,都可以识别出来。
后续
对象检测模型(需要摄像头);Coral USB Accelerator加速
参考链接:
TensorFlow python Quickstart
Tensorflow Lite examples