时间序列图
带波峰波谷标记的时序图
自相关和部分自相关图
交叉相关图
时间序列分解图
多个时间序列
使用辅助Y轴来绘制不同范围的图形
带有误差带的时间序列
堆积面积图
未堆积的面积图
日历热力图
季节图
变化
35.时间序列图
时间序列图用于可视化给定指标如何随时间变化。在这里,您可以了解1949年至1969年之间的航空客运流量如何变化。
# Import Datadf = pd.read_csv('https://github.com/selva86/datasets/raw/master/AirPassengers.csv')# Draw Plotplt.figure(figsize=(16,10), dpi= 80)plt.plot('date', 'traffic', data=df, color='tab:red')# Decorationplt.ylim(50, 750)xtick_location = df.index.tolist()[::12]xtick_labels = [x[-4:] for x in df.date.tolist()[::12]]plt.xticks(ticks=xtick_location, labels=xtick_labels, rotation=0, fontsize=12, horizontalalignment='center', alpha=.7)plt.yticks(fontsize=12, alpha=.7)plt.title("Air Passengers Traffic (1949 - 1969)", fontsize=22)plt.grid(axis='both', alpha=.3)# Remove bordersplt.gca().spines["top"].set_alpha(0.0) plt.gca().spines["bottom"].set_alpha(0.3)plt.gca().spines["right"].set_alpha(0.0) plt.gca().spines["left"].set_alpha(0.3) plt.show()
36.带波峰波谷标记的时序图
下面的时间序列绘制了所有的波峰和波谷,并注释了选定特殊事件的发生。
# Import Datadf = pd.read_csv('https://github.com/selva86/datasets/raw/master/AirPassengers.csv')# Get the Peaks and Troughsdata = df['traffic'].valuesdoublediff = np.diff(np.sign(np.diff(data)))peak_locations = np.where(doublediff == -2)[0] + 1doublediff2 = np.diff(np.sign(np.diff(-1*data)))trough_locations = np.where(doublediff2 == -2)[0] + 1# Draw Plotplt.figure(figsize=(16,10), dpi= 80)plt.plot('date', 'traffic', data=df, color='tab:blue', label='Air Traffic')plt.scatter(df.date[peak_locations], df.traffic[peak_locations], marker=mpl.markers.CARETUPBASE, color='tab:green', s=100, label='Peaks')plt.scatter(df.date[trough_locations], df.traffic[trough_locations], marker=mpl.markers.CARETDOWNBASE, color='tab:red', s=100, label='Troughs')# Annotatefor t, p in zip(trough_locations[1::5], peak_locations[::3]): plt.text(df.date[p], df.traffic[p]+15, df.date[p], horizontalalignment='center', color='darkgreen') plt.text(df.date[t], df.traffic[t]-35, df.date[t], horizontalalignment='center', color='darkred')# Decorationplt.ylim(50,750)xtick_location = df.index.tolist()[::6]xtick_labels = df.date.tolist()[::6]plt.xticks(ticks=xtick_location, labels=xtick_labels, rotation=90, fontsize=12, alpha=.7)plt.title("Peak and Troughs of Air Passengers Traffic (1949 - 1969)", fontsize=22)plt.yticks(fontsize=12, alpha=.7)# Lighten bordersplt.gca().spines["top"].set_alpha(.0)plt.gca().spines["bottom"].set_alpha(.3)plt.gca().spines["right"].set_alpha(.0)plt.gca().spines["left"].set_alpha(.3)plt.legend(loc='upper left')plt.grid(axis='y', alpha=.3)plt.show()
37.自相关(ACF)和部分自相关(PACF)
图
ACF图显示了时间序列与其自身滞后的相关性。每条垂直线(在自相关图上)代表序列与从滞后0开始的滞后之间的相关性。图中的蓝色阴影区域是显着性水平。那些位于蓝线上方的滞后就是巨大的滞后。
那么如何解释呢?
对于AirPassengers,我们看到多达14个滞后时间已越过蓝线,因此意义重大。这意味着,距今已有14年之久的航空客运量对今天的客运量产生了影响。
另一方面,PACF显示了任何给定的(时间序列)滞后与当前序列之间的自相关,但是去除了两者之间的滞后的影响。
from statsmodels.graphics.tsaplots import plot_acf, plot_pacf# Import Datadf = pd.read_csv('https://github.com/selva86/datasets/raw/master/AirPassengers.csv')# Draw Plotfig, (ax1, ax2) = plt.subplots(1, 2,figsize=(16,6), dpi= 80)plot_acf(df.traffic.tolist(), ax=ax1, lags=50)plot_pacf(df.traffic.tolist(), ax=ax2, lags=20)# Decorate# lighten the bordersax1.spines["top"].set_alpha(.3); ax2.spines["top"].set_alpha(.3)ax1.spines["bottom"].set_alpha(.3); ax2.spines["bottom"].set_alpha(.3)ax1.spines["right"].set_alpha(.3); ax2.spines["right"].set_alpha(.3)ax1.spines["left"].set_alpha(.3); ax2.spines["left"].set_alpha(.3)# font size of tick labelsax1.tick_params(axis='both', labelsize=12)ax2.tick_params(axis='both', labelsize=12)plt.show()
38.交叉相关图
交叉相关图显示了两个时间序列之间的时滞。
import statsmodels.tsa.stattools as stattools# Import Datadf = pd.read_csv('https://github.com/selva86/datasets/raw/master/mortality.csv')x = df['mdeaths']y = df['fdeaths']# Compute Cross Correlationsccs = stattools.ccf(x, y)[:100]nlags = len(ccs)# Compute the Significance level# ref: https://stats.stackexchange.com/questions/3115/cross-correlation-significance-in-r/3128#3128conf_level = 2 / np.sqrt(nlags)# Draw Plotplt.figure(figsize=(12,7), dpi= 80)plt.hlines(0, xmin=0, xmax=100, color='gray') # 0 axisplt.hlines(conf_level, xmin=0, xmax=100, color='gray')plt.hlines(-conf_level, xmin=0, xmax=100, color='gray')plt.bar(x=np.arange(len(ccs)), height=ccs, width=.3)# Decorationplt.title('$Cross\; Correlation\; Plot:\; mdeaths\; vs\; fdeaths$', fontsize=22)plt.xlim(0,len(ccs))plt.show()
39.时间序列分解图
时间序列分解图显示了时间序列按趋势,季节和残差成分的分解。
from statsmodels.tsa.seasonal import seasonal_decomposefrom dateutil.parser import parse# Import Datadf = pd.read_csv('https://github.com/selva86/datasets/raw/master/AirPassengers.csv')dates = pd.DatetimeIndex([parse(d).strftime('%Y-%m-01') for d in df['date']])df.set_index(dates, inplace=True)# Decompose result = seasonal_decompose(df['traffic'], model='multiplicative')# Plotplt.rcParams.update({'figure.figsize': (10,10)})result.plot().suptitle('Time Series Decomposition of Air Passengers')plt.show()
40.多个时间序列
您可以在同一张图表上绘制测量同一值的多个时间序列,如下所示。
# Import Datadf = pd.read_csv('https://github.com/selva86/datasets/raw/master/mortality.csv')# Define the upper limit, lower limit, interval of Y axis and colorsy_LL = 100y_UL = int(df.iloc[:, 1:].max().max()*1.1)y_interval = 400mycolors = ['tab:red', 'tab:blue', 'tab:green', 'tab:orange'] # Draw Plot and Annotatefig, ax = plt.subplots(1,1,figsize=(16, 9), dpi= 80) columns = df.columns[1:] for i, column in enumerate(columns): plt.plot(df.date.values, df[column].values, lw=1.5, color=mycolors[i]) plt.text(df.shape[0]+1, df[column].values[-1], column, fontsize=14, color=mycolors[i])# Draw Tick lines for y in range(y_LL, y_UL, y_interval): plt.hlines(y, xmin=0, xmax=71, colors='black', alpha=0.3, linestyles="--", lw=0.5)# Decorations plt.tick_params(axis="both", which="both", bottom=False, top=False, labelbottom=True, left=False, right=False, labelleft=True) # Lighten bordersplt.gca().spines["top"].set_alpha(.3)plt.gca().spines["bottom"].set_alpha(.3)plt.gca().spines["right"].set_alpha(.3)plt.gca().spines["left"].set_alpha(.3)plt.title('Number of Deaths from Lung Diseases in the UK (1974-1979)', fontsize=22)plt.yticks(range(y_LL, y_UL, y_interval), [str(y) for y in range(y_LL, y_UL, y_interval)], fontsize=12) plt.xticks(range(0, df.shape[0], 12), df.date.values[::12], horizontalalignment='left', fontsize=12) plt.ylim(y_LL, y_UL) plt.xlim(-2, 80) plt.show()
41.使用辅助Y轴来绘制不同范围的图形
如果要显示在同一时间点测量两个不同量的两个时间序列,则可以在右边的第二个Y轴上再次绘制第二个序列。
# Import Datadf = pd.read_csv("https://github.com/selva86/datasets/raw/master/economics.csv")x = df['date']y1 = df['psavert']y2 = df['unemploy']# Plot Line1 (Left Y Axis)fig, ax1 = plt.subplots(1,1,figsize=(16,9), dpi= 80)ax1.plot(x, y1, color='tab:red')# Plot Line2 (Right Y Axis)ax2 = ax1.twinx() # instantiate a second axes that shares the same x-axisax2.plot(x, y2, color='tab:blue')# Decorations# ax1 (left Y axis)ax1.set_xlabel('Year', fontsize=20)ax1.tick_params(axis='x', rotation=0, labelsize=12)ax1.set_ylabel('Personal Savings Rate', color='tab:red', fontsize=20)ax1.tick_params(axis='y', rotation=0, labelcolor='tab:red' )ax1.grid(alpha=.4)# ax2 (right Y axis)ax2.set_ylabel("# Unemployed (1000's)", color='tab:blue', fontsize=20)ax2.tick_params(axis='y', labelcolor='tab:blue')ax2.set_xticks(np.arange(0, len(x), 60))ax2.set_xticklabels(x[::60], rotation=90, fontdict={'fontsize':10})ax2.set_title("Personal Savings Rate vs Unemployed: Plotting in Secondary Y Axis", fontsize=22)fig.tight_layout()plt.show()
42.带有误差带的时间序列
如果您有一个在每个时间点(日期/时间戳)具有多个观测值的时间序列数据集,则可以构建带有误差带的时间序列。您可以在下面看到几个示例,这些示例基于一天中不同时间的订单。另一个例子是在45天的时间内到达的订单数量。
在这种方法中,订单数量的平均值由白线表示。然后计算出95%的置信带并围绕均值绘制。
from scipy.stats import sem# Import Datadf = pd.read_csv("https://raw.githubusercontent.com/selva86/datasets/master/user_orders_hourofday.csv")df_mean = df.groupby('order_hour_of_day').quantity.mean()df_se = df.groupby('order_hour_of_day').quantity.apply(sem).mul(1.96)# Plotplt.figure(figsize=(16,10), dpi= 80)plt.ylabel("# Orders", fontsize=16) x = df_mean.indexplt.plot(x, df_mean, color="white", lw=2) plt.fill_between(x, df_mean - df_se, df_mean + df_se, color="#3F5D7D") # Decorations# Lighten bordersplt.gca().spines["top"].set_alpha(0)plt.gca().spines["bottom"].set_alpha(1)plt.gca().spines["right"].set_alpha(0)plt.gca().spines["left"].set_alpha(1)plt.xticks(x[::2], [str(d) for d in x[::2]] , fontsize=12)plt.title("User Orders by Hour of Day (95% confidence)", fontsize=22)plt.xlabel("Hour of Day")s, e = plt.gca().get_xlim()plt.xlim(s, e)# Draw Horizontal Tick lines for y in range(8, 20, 2): plt.hlines(y, xmin=s, xmax=e, colors='black', alpha=0.5, linestyles="--", lw=0.5)plt.show()
"Data Source: https://www.kaggle.com/olistbr/brazilian-ecommerce#olist_orders_dataset.csv"from dateutil.parser import parsefrom scipy.stats import sem# Import Datadf_raw = pd.read_csv('https://raw.githubusercontent.com/selva86/datasets/master/orders_45d.csv', parse_dates=['purchase_time', 'purchase_date'])# Prepare Data: Daily Mean and SE Bandsdf_mean = df_raw.groupby('purchase_date').quantity.mean()df_se = df_raw.groupby('purchase_date').quantity.apply(sem).mul(1.96)# Plotplt.figure(figsize=(16,10), dpi= 80)plt.ylabel("# Daily Orders", fontsize=16) x = [d.date().strftime('%Y-%m-%d') for d in df_mean.index]plt.plot(x, df_mean, color="white", lw=2) plt.fill_between(x, df_mean - df_se, df_mean + df_se, color="#3F5D7D") # Decorations# Lighten bordersplt.gca().spines["top"].set_alpha(0)plt.gca().spines["bottom"].set_alpha(1)plt.gca().spines["right"].set_alpha(0)plt.gca().spines["left"].set_alpha(1)plt.xticks(x[::6], [str(d) for d in x[::6]] , fontsize=12)plt.title("Daily Order Quantity of Brazilian Retail with Error Bands (95% confidence)", fontsize=20)# Axis limitss, e = plt.gca().get_xlim()plt.xlim(s, e-2)plt.ylim(4, 10)# Draw Horizontal Tick lines for y in range(5, 10, 1): plt.hlines(y, xmin=s, xmax=e, colors='black', alpha=0.5, linestyles="--", lw=0.5)plt.show()
43.堆积面积图
堆积面积图直观地表示了多个时间序列的贡献程度,因此可以轻松地进行相互比较。
# Import Datadf = pd.read_csv('https://raw.githubusercontent.com/selva86/datasets/master/nightvisitors.csv')# Decide Colors mycolors = ['tab:red', 'tab:blue', 'tab:green', 'tab:orange', 'tab:brown', 'tab:grey', 'tab:pink', 'tab:olive'] # Draw Plot and Annotatefig, ax = plt.subplots(1,1,figsize=(16, 9), dpi= 80)columns = df.columns[1:]labs = columns.values.tolist()# Prepare datax = df['yearmon'].values.tolist()y0 = df[columns[0]].values.tolist()y1 = df[columns[1]].values.tolist()y2 = df[columns[2]].values.tolist()y3 = df[columns[3]].values.tolist()y4 = df[columns[4]].values.tolist()y5 = df[columns[5]].values.tolist()y6 = df[columns[6]].values.tolist()y7 = df[columns[7]].values.tolist()y = np.vstack([y0, y2, y4, y6, y7, y5, y1, y3])# Plot for each columnlabs = columns.values.tolist()ax = plt.gca()ax.stackplot(x, y, labels=labs, colors=mycolors, alpha=0.8)# Decorationsax.set_title('Night Visitors in Australian Regions', fontsize=18)ax.set(ylim=[0, 100000])ax.legend(fontsize=10, ncol=4)plt.xticks(x[::5], fontsize=10, horizontalalignment='center')plt.yticks(np.arange(10000, 100000, 20000), fontsize=10)plt.xlim(x[0], x[-1])# Lighten bordersplt.gca().spines["top"].set_alpha(0)plt.gca().spines["bottom"].set_alpha(.3)plt.gca().spines["right"].set_alpha(0)plt.gca().spines["left"].set_alpha(.3)plt.show()
44.未堆积的面积图
未堆积的面积图用于可视化两个或多个系列相对于彼此的进度(涨跌)。在下面的图表中,您可以清楚地看到随着失业时间的中位数增加,个人储蓄率如何下降。未堆积面积图很好地显示了这种现象。
# Import Datadf = pd.read_csv("https://github.com/selva86/datasets/raw/master/economics.csv")# Prepare Datax = df['date'].values.tolist()y1 = df['psavert'].values.tolist()y2 = df['uempmed'].values.tolist()mycolors = ['tab:red', 'tab:blue', 'tab:green', 'tab:orange', 'tab:brown', 'tab:grey', 'tab:pink', 'tab:olive'] columns = ['psavert', 'uempmed']# Draw Plot fig, ax = plt.subplots(1, 1, figsize=(16,9), dpi= 80)ax.fill_between(x, y1=y1, y2=0, label=columns[1], alpha=0.5, color=mycolors[1], linewidth=2)ax.fill_between(x, y1=y2, y2=0, label=columns[0], alpha=0.5, color=mycolors[0], linewidth=2)# Decorationsax.set_title('Personal Savings Rate vs Median Duration of Unemployment', fontsize=18)ax.set(ylim=[0, 30])ax.legend(loc='best', fontsize=12)plt.xticks(x[::50], fontsize=10, horizontalalignment='center')plt.yticks(np.arange(2.5, 30.0, 2.5), fontsize=10)plt.xlim(-10, x[-1])# Draw Tick lines for y in np.arange(2.5, 30.0, 2.5): plt.hlines(y, xmin=0, xmax=len(x), colors='black', alpha=0.3, linestyles="--", lw=0.5)# Lighten bordersplt.gca().spines["top"].set_alpha(0)plt.gca().spines["bottom"].set_alpha(.3)plt.gca().spines["right"].set_alpha(0)plt.gca().spines["left"].set_alpha(.3)plt.show()
45.日历热图
日历地图是与时间序列相比可视化基于时间的数据的替代方法,而不是首选方法。尽管可以在视觉上吸引人,但数值并不十分明显。但是,它可以有效地很好地描绘出极端值和假日效果。
import matplotlib as mplimport calmap# Import Datadf = pd.read_csv("https://raw.githubusercontent.com/selva86/datasets/master/yahoo.csv", parse_dates=['date'])df.set_index('date', inplace=True)# Plotplt.figure(figsize=(16,10), dpi= 80)calmap.calendarplot(df['2014']['VIX.Close'], fig_kws={'figsize': (16,10)}, yearlabel_kws={'color':'black', 'fontsize':14}, subplot_kws={'title':'Yahoo Stock Prices'})plt.show()
46.季节图
季节性图可用于比较上一个季节的同一天(年/月/周等)的时间序列执行情况。
from dateutil.parser import parse # Import Datadf = pd.read_csv('https://github.com/selva86/datasets/raw/master/AirPassengers.csv')# Prepare datadf['year'] = [parse(d).year for d in df.date]df['month'] = [parse(d).strftime('%b') for d in df.date]years = df['year'].unique()# Draw Plotmycolors = ['tab:red', 'tab:blue', 'tab:green', 'tab:orange', 'tab:brown', 'tab:grey', 'tab:pink', 'tab:olive', 'deeppink', 'steelblue', 'firebrick', 'mediumseagreen'] plt.figure(figsize=(16,10), dpi= 80)for i, y in enumerate(years): plt.plot('month', 'traffic', data=df.loc[df.year==y, :], color=mycolors[i], label=y) plt.text(df.loc[df.year==y, :].shape[0]-.9, df.loc[df.year==y, 'traffic'][-1:].values[0], y, fontsize=12, color=mycolors[i])# Decorationplt.ylim(50,750)plt.xlim(-0.3, 11)plt.ylabel('$Air Traffic$')plt.yticks(fontsize=12, alpha=.7)plt.title("Monthly Seasonal Plot: Air Passengers Traffic (1949 - 1969)", fontsize=22)plt.grid(axis='y', alpha=.3)# Remove bordersplt.gca().spines["top"].set_alpha(0.0) plt.gca().spines["bottom"].set_alpha(0.5)plt.gca().spines["right"].set_alpha(0.0) plt.gca().spines["left"].set_alpha(0.5) # plt.legend(loc='upper right', ncol=2, fontsize=12)plt.show()
申明:原文来源网络,由《计算机视觉社区》公众号整理分享大家,仅供参考学习使用,不得用于商用,引用或转载请注明出处!如有侵权请联系删除!
点一下 在看 会更好看哦
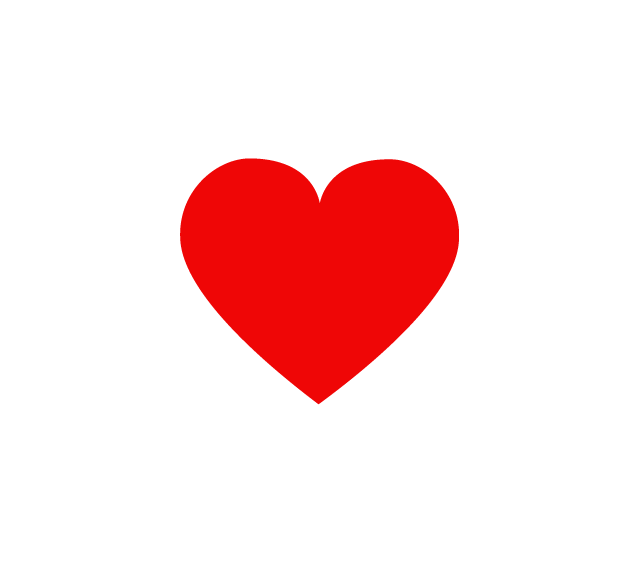